Revolutionizing IR Spectroscopy: Unleashing the Power of Gemini in Technological Advancements
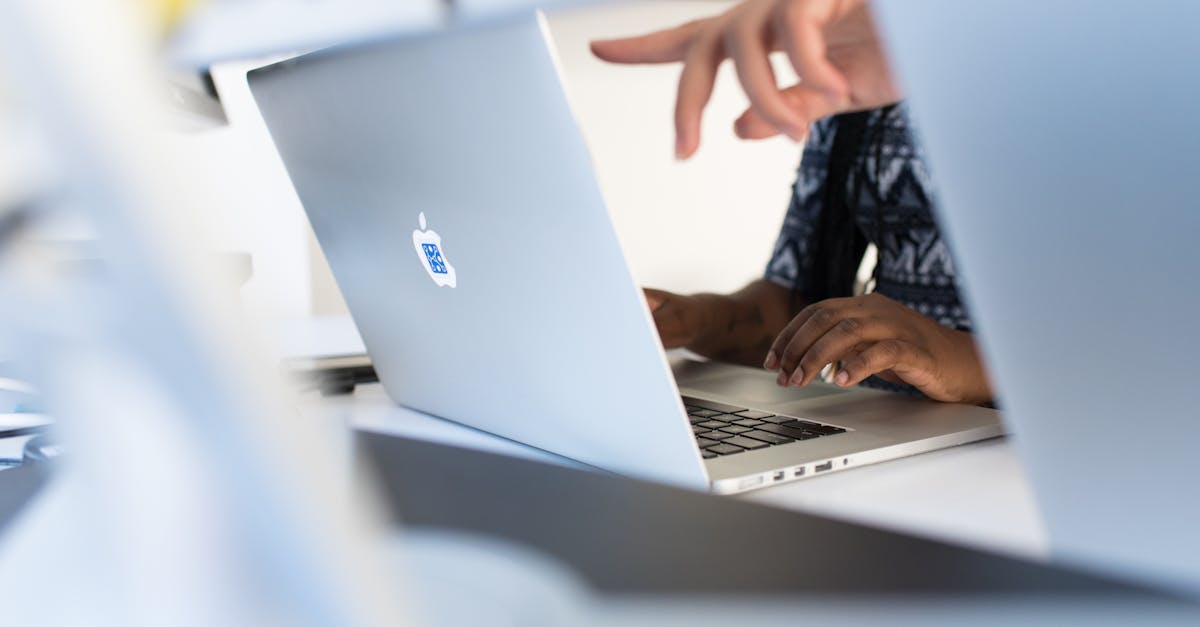
In the ever-evolving field of technology, breakthroughs are constantly being made to push the boundaries of what is possible. One such breakthrough has occurred in the realm of IR spectroscopy, thanks to the advent of Gemini.
IR spectroscopy, or infrared spectroscopy, is a technique widely used to identify and analyze chemical compounds based on the frequencies of infrared light absorbed by the given substance. Traditionally, data interpretation in IR spectroscopy relied heavily on manual analysis and human expertise. This process could be time-consuming, prone to human error, and limited by the vast amount of spectral data that needed to be analyzed.
Enter Gemini, a cutting-edge language model developed by Google. Powered by state-of-the-art machine learning algorithms, Gemini is designed to generate human-like text, respond to prompts, and perform various language processing tasks. Leveraging its capabilities, Gemini has been successfully integrated into IR spectroscopy to revolutionize the field.
Technological Advancements
The integration of Gemini in IR spectroscopy has led to several technological advancements. One of the key areas where Gemini shines is in the automation of spectral analysis. By training the model on a vast dataset of spectral information and patterns, it has learned to identify and interpret these patterns automatically. This significantly reduces the need for manual analysis, accelerating the analytical process and increasing productivity.
Furthermore, Gemini's ability to generate text and respond to prompts allows researchers to ask questions or seek insights from the model. Through a user-friendly interface, scientists can interact with Gemini and obtain relevant information about the spectroscopic data. This interactive feature enhances collaboration and facilitates knowledge sharing among researchers.
Usage and Applications
The utilization of Gemini in IR spectroscopy opens up numerous possibilities for its applications. With its automated analysis capabilities, researchers can now analyze large sets of spectral data more efficiently, enabling them to identify trends, anomalies, or correlations that were previously challenging to uncover manually.
Additionally, Gemini can aid in the identification and classification of chemical compounds. By using its natural language processing abilities, the model can provide accurate information about the composition, structures, and functional groups present in spectroscopic data. This can be invaluable in various fields, including pharmaceuticals, forensics, environmental science, and materials research.
The Future of IR Spectroscopy
The integration of Gemini in IR spectroscopy represents a significant leap forward in the field. The enhanced automation and analysis capabilities brought about by Gemini hold immense potential for improving the accuracy, efficiency, and scalability of spectroscopic analysis.
As AI and machine learning algorithms continue to advance, we can expect further refinements and enhancements in the integration of Gemini in IR spectroscopy. The combination of human expertise and AI-driven automation has the power to unlock new insights, advance scientific discoveries, and drive technological innovations in the field of IR spectroscopy and beyond.
Comments:
Thank you all for reading my article on revolutionizing IR spectroscopy with Gemini! I'm excited to hear your thoughts and feedback.
Great article, Galen! I'm fascinated by the potential of Gemini in advancing IR spectroscopy. It could revolutionize data analysis and interpretation.
I agree, Erika! The applications of Gemini in IR spectroscopy are promising. It could save researchers a lot of time and help discover new insights.
Indeed, Daniel! The ability of Gemini to quickly process large datasets and provide real-time analysis is a game-changer for IR spectroscopy research.
As an IR spectroscopy researcher, I'm curious about the integration process of Gemini. How would we ensure its accuracy and reliability for our specific applications?
That's a valid concern, Sophia. To ensure accuracy, the integration would involve training the Gemini specifically on IR spectroscopy data. Additionally, continuous fine-tuning and validation with expert input would be essential.
I'm impressed by the potential impact of Gemini on IR spectroscopy, but what about the limitations? Can it handle the complexity and nuances present in spectra?
Valid point, Michael. While Gemini shows promise, it indeed has limitations. It's essential to assess its accuracy, especially in complex spectra analysis, through rigorous testing and benchmarking against existing methods.
I see the potential, but I'm concerned about the ethics. How can we ensure responsible use of Gemini in IR spectroscopy while avoiding biased outcomes?
Ethical considerations are critical, Olivia. Transparency in training data, fine-tuning, and regular audits help mitigate biases. We must also ensure human oversight and establish guidelines to avoid any unintended consequences.
I wonder about the possible integration challenges for laboratories already using traditional methods. Would they need significant infrastructure upgrades?
Good question, Lucas. Integrating Gemini would require proper infrastructure and computational resources. However, leveraging cloud-based solutions could diminish the need for extensive upgrades at individual laboratories.
Gemini seems promising, but I'm concerned about the cost. Would it be affordable for smaller research institutions or individuals?
Affordability is a valid concern, Emily. While cost considerations would need to be addressed, cloud-based platforms and potential collaborations with institutions could help mitigate the financial burden for smaller research entities.
I can see how Gemini can streamline data analysis, but what about the interpretability of results? Will the AI be able to explain its conclusions to researchers?
Excellent point, Liam. Explainability is important. While AI models like Gemini might not provide direct explanations, efforts can be made to build on interpretability techniques and design systems that provide insights into the model's decision-making processes.
Gemini's potential is exciting, but I'm concerned about the learning curve for researchers unfamiliar with AI. How user-friendly would the integration be?
User-friendliness is crucial, Sarah. The integration should aim to be intuitive and provide clear interfaces for researchers with different levels of AI expertise. User feedback and iterative improvements will be essential in making it accessible.
Galen, have there been any preliminary trials or practical implementations of Gemini in the field of IR spectroscopy?
Great question, Erika. While Gemini in IR spectroscopy is still at an early stage, there have been some small-scale trials demonstrating its potential. Broader practical implementations and further research are needed to fully assess its capabilities.
I appreciate the potential of Gemini, but what about its scalability? Can it handle large-scale data analysis required in industrial applications?
Scalability is crucial, David. While Gemini has limitations in handling vast amounts of data, cloud-based solutions and parallel computation can enhance its scalability for industrial applications. Further optimization and research are necessary.
I'm concerned about possible biases in the training data impacting the AI's output. How can we address and minimize biased results in Gemini?
Addressing biases is crucial, Sophie. Data selection, augmentation, and validation must be carefully performed to minimize biased outcomes. Regular audits and diverse input from experts can help identify and rectify any biases that arise.
Are there any plans to develop a user community/platform around Gemini in IR spectroscopy for knowledge sharing and collaboration?
Absolutely, Samuel! Creating a user community and fostering collaboration is essential for the success of Gemini in IR spectroscopy. Establishing platforms for knowledge sharing, troubleshooting, and collective improvement would be highly beneficial.
Do you foresee any challenges in gaining acceptance and trust from the scientific community for the integration of Gemini in IR spectroscopy?
Trust and acceptance are indeed challenges, Nora. Open communication, peer-reviewed studies, and replicability of results will be crucial in gaining the trust of the scientific community. Collaboration with experts and involving the community in the development process will also help.
Galen, could we get access to a pre-trained Gemini model specifically tailored for IR spectroscopy, or would we have to train it ourselves?
Good question, Daniel. While pre-trained Gemini models could provide a starting point, adapting the model to the specific requirements of IR spectroscopy would likely involve fine-tuning or retraining on relevant datasets. Tailoring it to specific needs is crucial for accuracy.
How long do you think it will be before Gemini becomes mainstream in IR spectroscopy research laboratories?
Predicting exact timelines is challenging, Lucas. However, with continued research, refining the technology, addressing limitations, and proving its potential, we can expect broader adoption within the next decade.
Galen, are there any privacy concerns associated with using Gemini in IR spectroscopy research?
Privacy concerns should be taken seriously, Sophia. Implementations of Gemini must ensure data confidentiality and compliance with privacy regulations. Researchers should have control over the data shared and communicate transparently about any potential risks.
I'm intrigued by the potential applications of Gemini in IR spectroscopy. Galen, what do you think are the most exciting possibilities?
There are several exciting possibilities, Michael! Real-time analysis, automated spectral interpretation, enhanced data processing, and predictive modeling are just a few. Gemini has the potential to accelerate the pace of discoveries and make IR spectroscopy more efficient.
How would you propose involving domain experts while training Gemini to ensure accuracy and prevent errors?
Involving domain experts is vital, Emily. Collaborations between AI researchers and spectroscopy experts can ensure accurate training data, provide insights into spectral analysis nuances, and detect potential errors during development. Their input is essential in achieving reliable results.
Galen, have you considered the potential impact of Gemini integration on reducing costs and time investments in IR spectroscopy research?
Absolutely, Sarah! The potential cost and time savings are significant. Faster analysis, reduced manual effort, and AI-enabled optimization can make IR spectroscopy research more cost-effective and allow researchers to focus on further advancements and discoveries.
I'm concerned about the black-box nature of AI models. How can we ensure transparency and avoid blindly relying on Gemini's predictions?
Transparency is important, Samuel. While complete transparency in AI systems may be challenging, we can encourage research in explainability techniques, interpretability tools, and robust validation procedures. Striving for transparency will strengthen trust in Gemini's predictions.
I'm worried about potential job displacement for researchers. Could Gemini replace the need for manual interpretation and analysis in IR spectroscopy?
Job displacement is a valid concern, Olivia. Gemini can automate certain tasks, but it's important to view it as a valuable tool for researchers rather than a total replacement. It can enhance efficiency, allowing researchers to focus on more complex challenges and scientific advancements.
Have there been any instances where Gemini has produced unexpected or unreliable results in IR spectroscopy research?
While there have been instances of AI models producing unexpected results, David, early trials with Gemini in IR spectroscopy have shown promise. However, further validation, testing, and addressing model limitations are necessary to ensure reliable and robust outcomes.
Galen, what kind of computational resources or hardware would be required to effectively use Gemini for IR spectroscopy analysis?
Using Gemini effectively would require computational resources, Daniel. Depending on the scale of data and tasks, adequate hardware setups could include GPUs or cloud-based solutions to handle the processing and analysis demands efficiently.
Given the potential of Gemini, are there any plans to integrate it with existing IR spectroscopy software tools?
Integrating Gemini with existing software tools is indeed a possibility, Lucas. Collaboration with software developers and seamless compatibility with different platforms would be beneficial, fostering wider adoption and usability of Gemini in the IR spectroscopy community.
Thank you all for engaging in this discussion! I'm thrilled to see so much interest in the potential of Gemini in advancing IR spectroscopy. I would love to hear your thoughts and opinions on this topic.
This article presents an exciting use case for Gemini. The ability to have interactive conversations with the AI model opens up new possibilities in the field of spectroscopy.
I agree, Margot. Gemini's capacity to understand and respond in a conversational manner could greatly enhance the user experience and speed up the analysis process in IR spectroscopy.
The potential applications of Gemini in various scientific fields are vast. I'm curious, Galen, have there been any studies demonstrating the reliability and accuracy of Gemini in spectroscopic analysis?
Anjali, excellent question. While Gemini shows promise, there is ongoing research to further validate its reliability in spectroscopic analysis. Initial results suggest high accuracy, but further investigation is needed to establish its robustness.
AI has revolutionized several industries, and it's exciting to see its potential in spectroscopy. However, I wonder how Gemini handles complex and specialized queries that may arise during analysis.
Paul, that's a valid concern. While Gemini has shown impressive capabilities, it may encounter limitations when faced with extremely specialized queries. It's crucial to understand the model's scope and potential constraints.
I'm curious about the integration process of Gemini into existing IR spectroscopy systems. Galen, can you elaborate on how it can be seamlessly incorporated?
Certainly, Elena. Integrating Gemini into IR spectroscopy systems involves creating an interface that allows users to interact in real-time. The model needs to be trained on relevant spectroscopy data to ensure its understanding of specific requirements.
Thank you for the clarification, Galen. It sounds like an exciting development that can greatly enhance the capabilities of existing spectroscopy systems.
While Gemini shows promise in spectroscopy, I wonder about its potential vulnerability to adversarial attacks. Are there any measures in place to ensure the model's security?
Good point, Mark. Security is a crucial aspect to consider, especially when incorporating AI models into sensitive scientific domains. Galen, are there any security measures being explored for Gemini in spectroscopy?
Mark and Harold, you raise an important concern. The research team behind Gemini is actively investigating potential security vulnerabilities and working on measures to mitigate any risks associated with adversarial attacks in spectroscopy applications.
I can certainly see the potential of using Gemini in IR spectroscopy, but what about the cost implications? Will integrating this technology significantly increase the expenses for laboratories and research facilities?
Sophie, that's a valid concern. While integrating Gemini may involve additional costs initially, the reduced need for human experts and the potential efficiency gains could offset those expenses in the long run.
Thank you for addressing my question, Margot. It's important to carefully consider both the immediate and long-term financial implications of adopting this technology.
Galen, how does Gemini handle uncertainties and ambiguities in spectral analysis, where human judgment often plays a crucial role?
Great question, Adam. Gemini can provide insights based on learned patterns and knowledge, but it also recognizes its limitations. Human judgment remains essential in cases where uncertainties and ambiguities arise, acting as a valuable complement to the AI model.
It's fascinating to witness the integration of AI into fields like spectroscopy. Galen, what are the steps involved in training Gemini for spectroscopy tasks?
Megan, training Gemini for spectroscopy tasks involves providing it with a large dataset of spectral data along with relevant annotations. The model learns to understand the patterns and correlations within the data, enabling it to provide more accurate responses during analysis.
I'm excited about incorporating AI into spectroscopy, but what about the ethical aspects of relying on AI models for scientific analysis? Are there any guidelines or ethical frameworks being discussed in this context?
Nikhil, ethical considerations are crucial when embracing AI in scientific domains. Many researchers are actively working on establishing guidelines and ethical frameworks to ensure responsible and unbiased usage of AI models like Gemini in spectroscopic analysis.
Thank you for addressing my concern, Margot. It's reassuring to know that the scientific community is actively addressing the ethical implications of utilizing AI in fields like spectroscopy.
I'm curious to know if Gemini can handle real-time data analysis without any significant delays. Galen, could you share some insights regarding the computational requirements and speed of the model?
Grace, Gemini can achieve real-time analysis when properly optimized and deployed. The computational requirements may vary based on the complexity of the task, but with appropriate hardware configurations, the model can provide fast and efficient results.
I'm intrigued by the potential of Gemini in advancing spectroscopy. Can you elaborate on any specific technological advancements enabled by this integration?
Certainly, Daniel. The integration of Gemini in spectroscopy opens up possibilities for real-time interactive analysis, automatic report generation, and enhanced accessibility for researchers and users with varying levels of expertise. It can democratize access to spectroscopic knowledge and drive further innovations.
I'm glad to see AI facilitating scientific progress. Galen, are there any specific limitations or challenges that researchers should keep in mind when considering the adoption of Gemini in their spectroscopy workflows?
Victoria, researchers should consider that Gemini's responses are generated based on learned patterns from data, which may lead to inaccuracies in certain cases. Additionally, domain-specific expertise is still crucial to ensure the model's outputs are interpreted correctly.
Gemini's potential in spectroscopy is undoubtedly exciting. However, I wonder about the long-term support and maintenance needed for the model. Galen, could you provide some insights into this aspect?
Eric, maintaining and updating AI models like Gemini is an ongoing process. The research team behind Gemini is dedicated to improving and refining the model based on user feedback and new insights. Long-term support will be provided to ensure the model remains reliable and up-to-date.
It's exciting to witness the potential impact of Gemini in the field of spectroscopy. Galen, how does the model handle rare or novel spectral patterns that may not be well-represented in the training data?
Liam, while Gemini can generalize based on patterns learned from the training data, it may struggle with rare or novel spectral patterns that deviate significantly from its training set. In such cases, the involvement of human experts becomes crucial to ensure accurate analysis.
Galen, what data security measures are being implemented when using Gemini in spectroscopy? It's essential to protect sensitive spectral data from unauthorized access.
Oliver, data security is of utmost importance. When using Gemini, measures like encryption, access controls, and secure data handling protocols should be implemented to safeguard sensitive spectroscopic data from unauthorized access or breaches.
Gemini seems like a fascinating tool! Galen, how well can it adapt to the specific requirements of different spectroscopic techniques? Is it equally effective for infrared, Raman, and other types of spectroscopy?
Michelle, Gemini can be trained on data from various spectroscopic techniques, including infrared, Raman, and others. However, specialized training on specific techniques can further enhance its effectiveness in addressing technique-specific requirements and nuances.
I'm curious about the potential applications of Gemini in the pharmaceutical industry. Galen, are there any specific use cases being explored for the integration of AI in pharmaceutical spectroscopic analysis?
Samuel, the pharmaceutical industry has tremendous potential for utilizing AI, including Gemini, in spectroscopic analysis. Applications include drug development, quality control, and identification of impurities. It can speed up processes and improve overall efficiency in pharmaceutical analysis.
The article highlights the potential of Gemini in spectroscopy, but it's essential to remember that AI models are tools that assist human experts. They should augment, not replace, expert knowledge and experience.
Emily, you make an excellent point. AI models like Gemini should be seen as valuable tools that enhance and support human experts in their analyses. The combination of AI and human expertise has the potential to drive significant advancements in spectroscopy.
The incorporation of Gemini in spectroscopy is undoubtedly intriguing. Galen, what are the anticipated future developments in this field, and where do you see the integration of AI models heading?
Alexandra, the integration of AI models like Gemini opens up exciting possibilities for the future of spectroscopy. We can anticipate improved model capabilities, enhanced user interfaces, and expanded use cases. The collaborative efforts of researchers and user feedback will shape the future direction of AI integration in spectroscopy.
The potential advancements in spectroscopy with Gemini are impressive. Galen, what are the current challenges in deploying AI models like Gemini in real-world spectroscopic analysis?
Robert, deploying AI models like Gemini in real-world spectroscopic analysis faces challenges such as data preprocessing, performance optimization, and integration with existing systems. Additionally, ensuring reliable and accurate outputs while comprehending model limitations remains an active area of research.
The field of spectroscopy is constantly evolving, and the integration of AI models brings new possibilities. Galen, how do you envision the collaboration between AI models and human experts shaping the future of spectroscopic analysis?
Jennifer, I see a synergistic collaboration between AI models and human experts greatly shaping the future of spectroscopy. AI can augment human expertise, assist in rapid analysis, and enhance accessibility, enabling experts to focus on more intricate aspects of scientific exploration. The combination of AI and human insights will drive breakthroughs in this field.
Gemini's potential in advancing IR spectroscopy is evident. However, it's essential to ensure transparency in the decision-making process of the model to build trust and reliability. Galen, how is this aspect being addressed?
Matthew, ensuring transparency is a crucial aspect of AI model development. Efforts are being made to understand and interpret the decision-making processes of models like Gemini. Researchers are working on techniques to provide explanations for model outputs, building trust and fostering reliable usage in fields like spectroscopy.
Thank you all for your engaging comments and questions. It has been an insightful discussion, and your input is invaluable in shaping the future of AI in spectroscopy. If you have any further thoughts or questions, feel free to share them!