Revolutionizing Laboratory Automation: Leveraging ChatGPT for Automated Pipetting
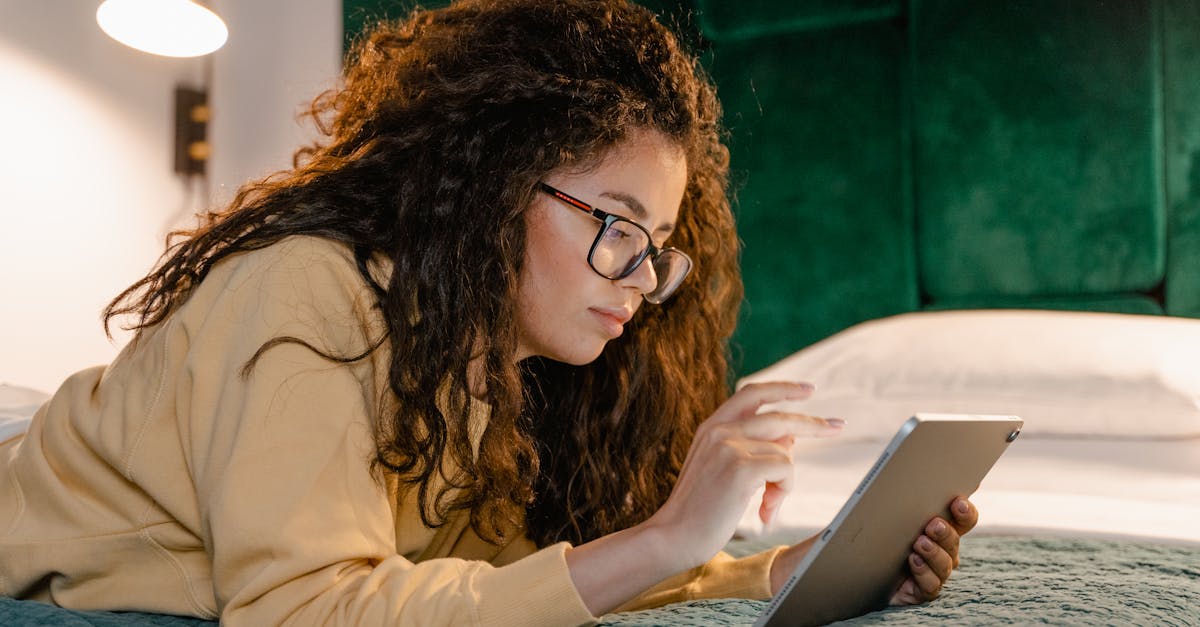
Laboratory automation has revolutionized various processes in scientific research, making them more efficient, accurate, and reliable. One critical area of laboratory automation is automated pipetting, which involves the precise transfer of small liquid volumes. This area has significantly benefited from advancements in technology, and one promising development is the use of ChatGPT-4 to control pipetting processes.
What is ChatGPT-4?
ChatGPT-4 is an advanced natural language processing model developed by OpenAI. It utilizes state-of-the-art machine learning techniques to generate human-like responses based on the given input. It has been trained on a vast amount of text data and can understand and generate coherent and contextually relevant sentences.
Automated Pipetting and ChatGPT-4 Integration
Automated pipetting systems have been around for many years, streamlining various laboratory workflows. However, integrating ChatGPT-4 with automated pipetting systems takes this automation to a whole new level.
With ChatGPT-4's language capabilities, it can interpret and respond to complex instructions given by researchers or technicians. This means that instead of relying solely on pre-programmed pipetting protocols, which may not cover every experimental scenario, researchers can communicate their specific requirements directly to ChatGPT-4.
For example, a researcher may need to perform a series of dilutions in a particular experiment. They can interact with ChatGPT-4 and provide the required dilution factors, volumes, and target concentrations. ChatGPT-4, in turn, can generate the appropriate pipetting commands and send them to the automated pipetting system, controlling the process with precision.
Precision and Accuracy
By using ChatGPT-4 to control automated pipetting, precision and accuracy can be greatly enhanced. ChatGPT-4 has the ability to analyze complex experimental requirements and generate precise pipetting commands accordingly. It takes into account factors such as liquid volumes, dilution factors, multiple steps, and specific instructions provided by the researcher.
Human error is minimized as ChatGPT-4 ensures adherence to the requested experiment parameters and instructions. Mistakes such as incorrect volumes, mix-ups, or missed steps can be significantly reduced, leading to more reliable and reproducible experimental results.
Benefits and Future Implications
The integration of ChatGPT-4 with automated pipetting in laboratory automation has several notable benefits. Firstly, it saves valuable time for researchers and technicians by automating the process of generating pipetting commands.
Secondly, it reduces the reliance on pre-programmed pipetting protocols and allows for more flexibility in experimental design. Researchers can interact with ChatGPT-4 and easily adapt the pipetting process to meet their specific requirements, without the need for extensive programming or manual intervention.
Furthermore, the precision and accuracy achieved through ChatGPT-4's control over pipetting processes contribute to more robust and reproducible scientific studies. The reduction of human error ensures that experimental outcomes are reliable and consistent, enabling better scientific insights and conclusions.
In the future, the integration of advanced language models like ChatGPT-4 with laboratory automation technologies may extend beyond automated pipetting. Such models can potentially be applied to control various other laboratory processes, ranging from sample preparation to data analysis, further enhancing the efficiency and accuracy of scientific research.
Conclusion
Laboratory automation, particularly in the area of automated pipetting, continues to leverage technological advancements to simplify complex scientific workflows. With the integration of ChatGPT-4, researchers can now control pipetting processes more precisely and accurately, effectively minimizing human error.
The collaboration between advanced language models and laboratory automation technologies opens up new avenues for improving experimental efficiency, adaptability, and reliability. As we move forward, we can expect to see further advancements and applications in laboratory automation, driven by the continuous evolution of both technology and artificial intelligence.
Comments:
Thank you all for your comments and feedback on my article! I appreciate your time and insights.
This article is fascinating! I never imagined that chatbots could be used in laboratory automation. The potential for improving efficiency is remarkable.
I agree, Sandra! Chatbot technology has come a long way, and its application in laboratory automation is truly revolutionary.
I'm curious about the accuracy of automated pipetting using ChatGPT. Can the system handle delicate and precise experiments?
That's a valid concern, Emily. It would be great to know more about the accuracy and precision of this technology.
From what I understand, the accuracy of automated pipetting using ChatGPT is very high. The system can be trained on vast amounts of data, making it capable of achieving precise pipetting.
Additionally, the article mentions that ChatGPT can learn from user feedback, meaning it can improve its accuracy over time.
That's reassuring, Sandra. I'm amazed at the progress made in incorporating AI into laboratory processes.
I wonder if ChatGPT can be integrated with other laboratory automation systems to create a more comprehensive solution?
Absolutely, Mark! This article suggests that ChatGPT can be integrated with existing software and hardware, making it adaptable to various laboratory setups.
That's impressive, Sandra. The versatility of ChatGPT opens up possibilities for optimizing different lab workflows.
Thanks for the info, Sandra! It's reassuring to know that this technology has high accuracy and flexibility.
I wonder if there are any limitations to using ChatGPT in laboratory automation?
Yes, Daniel, there are limitations. ChatGPT relies heavily on the data it is trained on, so if there are gaps in the training data, it may struggle to provide accurate suggestions.
Furthermore, it's important to keep in mind that ChatGPT is a language model and may not have a deep understanding of the specific tasks it is assisting with.
That's a valid point, Sandra. It's crucial to carefully train and fine-tune a ChatGPT model to fit the specific laboratory requirements.
Great exchange of thoughts, everyone! Sandra, you raised a crucial point about the limitations of ChatGPT.
I'm curious how ChatGPT compares to traditional laboratory automation systems in terms of speed and efficiency.
Regarding speed and efficiency, Mark, ChatGPT can provide real-time assistance, reducing the time spent on manual pipetting tasks.
That's impressive, Laslo! It seems ChatGPT has the potential to speed up laboratory workflows.
However, I wonder if ChatGPT's response time can be affected by large amounts of concurrent user interactions?
You have a valid concern, Mark. Although the article doesn't specifically address that, it's possible that high user load could impact response times.
Thank you for the clarification, Laslo. I agree that scalability is an important factor to consider.
Do you have any examples, Laslo?
Understandable, Laslo. I appreciate your time and valuable insights in this discussion.
You're welcome, Mark! Thank you for being a part of this conversation. If you have any further questions, feel free to reach out.
Will do, Laslo! Thanks again, and have a great day.
Thank you for providing valuable information, Laslo. The potential use cases for ChatGPT in laboratory automation are indeed exciting.
You're welcome, Emily! I'm glad you find the potential applications exciting. It's an exciting time for laboratory automation.
To ensure optimal performance, it might be necessary to consider system scalability and load balancing techniques.
Laslo, your insights would be valuable.
I'm excited to see the future developments and advancements in this field. The potential benefits for laboratories are immense!
Another aspect to consider is the cost of implementing and maintaining the ChatGPT system for laboratory automation.
It would be interesting to know if the benefits outweigh the financial investment.
I agree, Emily. Cost is always a significant consideration, especially for research institutions with limited budgets.
The article suggests that implementing ChatGPT can lead to cost savings in the long run by reducing the need for manual pipetting.
Additionally, there may be potential cost benefits associated with improved accuracy, reducing experimental errors.
Thank you for the insights, Sandra. It's reassuring to know that this technology can offer long-term cost savings.
I also wonder if ChatGPT requires specialized training for laboratory personnel to operate effectively.
That's a great question, Emily. The article mentions that ChatGPT is designed to be user-friendly and can provide guidance to users without extensive technical expertise.
However, depending on the complexity of the laboratory procedures, some level of training or familiarization might still be beneficial.
I believe the integration process with existing laboratory systems would require collaboration between technical experts and the laboratory staff.
Understanding the usability and training requirements is crucial for successful adoption of any new technology.
It's encouraging to know that ChatGPT aims to be user-friendly, as this could facilitate its integration into different laboratory settings.
I'm impressed with the potential of this technology to improve lab workflows and enhance productivity.
Are there any security concerns associated with using ChatGPT in laboratory automation?
Especially when considering sensitive or confidential research data.
Security is an important aspect, Daniel. As with any AI system, it's crucial to take measures to protect sensitive data and ensure compliance with data privacy regulations.
The users, especially in research settings, might need to evaluate any potential risks and take appropriate precautions.
It's possible that certain laboratories with highly sensitive data might prefer to limit the use of ChatGPT within their internal networks.
Having strong security measures in place, such as data encryption and access controls, can mitigate potential risks.
Thank you for addressing those concerns, Sandra. Security is definitely a top priority, especially when dealing with confidential research data.
You're welcome, Emily. I'm glad I could help. Are there any other questions or thoughts on this topic?
I'd love to hear more about real-world use cases where ChatGPT has been successfully implemented for laboratory automation.
I'm also interested in hearing about any practical applications of ChatGPT in laboratory environments.
Thank you all for your questions and engagement! Unfortunately, I'm not able to disclose specific real-world use cases at this moment, but the potential applications are vast.
The article emphasizes the flexibility of ChatGPT, allowing it to be adaptable to various laboratory automation scenarios.
Each laboratory can assess their specific needs and explore the feasibility of implementing ChatGPT into their workflows.
Thank you for your response, Laslo. I understand the need to protect proprietary information.
Thank you all once again for your participation and insightful comments! This has been a stimulating discussion.