Revolutionizing Network Provisioning: Harnessing the Power of ChatGPT in Network Design Technology
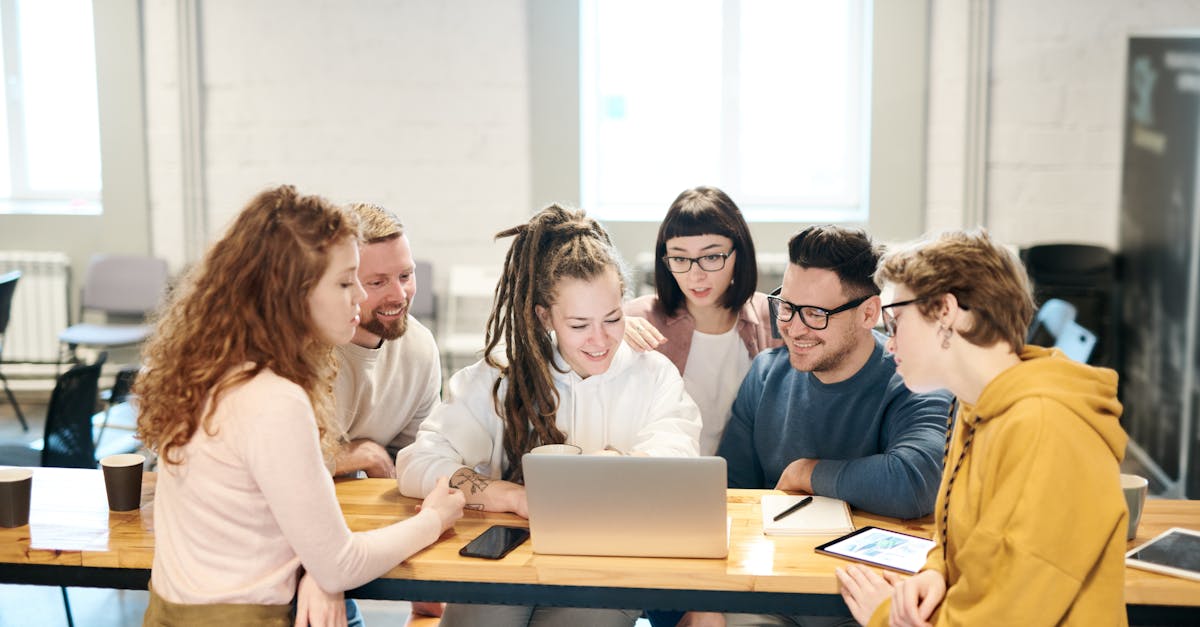
ChatGPT-4, an advanced AI-powered assistant, is capable of providing guidance on various network provisioning techniques. In today's rapidly evolving network landscape, organizations seek reliable, scalable, and efficient network designs to meet their growing connectivity needs. ChatGPT-4 can assist in understanding and implementing network provisioning techniques, including virtualization, software-defined networking (SDN), and network function virtualization (NFV).
Virtualization
Virtualization is a technology that enables the creation of virtual instances or representations of physical resources such as servers, networks, operating systems, and storage devices. In the context of network provisioning, virtualized networks allow multiple logical networks to coexist and operate securely on the same physical infrastructure. ChatGPT-4 can offer insights into implementing network virtualization, explaining its benefits, such as increased flexibility, improved resource utilization, and simplified network management through centralized control.
Software-Defined Networking (SDN)
Software-Defined Networking (SDN) is an architectural approach that separates the network's control plane from the data plane, enabling centralized control and programmability. Through SDN, network administrators can dynamically manage and configure network resources through software applications, rather than relying on traditional manual configuration. ChatGPT-4 can provide guidance on SDN implementation methodologies, discuss its advantages like enhanced network agility, improved scalability, and simplified network automation.
Network Function Virtualization (NFV)
Network Function Virtualization (NFV) is a technique that involves replacing traditional dedicated hardware network functions with virtualized instances running on standard servers or cloud infrastructure. By virtualizing network functions such as firewalls, load balancers, and intrusion detection systems, NFV empowers organizations to reduce hardware costs, improve network flexibility, and rapidly deploy new services. ChatGPT-4 can assist in understanding the deployment and management of NFV, and explain how it aligns with virtualization and SDN to create a more dynamic and cost-effective network infrastructure.
Conclusion
Network provisioning techniques such as virtualization, software-defined networking, and network function virtualization play a crucial role in modern network design. By adopting these techniques, organizations can achieve greater flexibility, scalability, and operational efficiency. ChatGPT-4, as an AI-powered assistant, is well-equipped to provide guidance on implementing these network provisioning methodologies. Whether you are exploring the benefits of network virtualization, seeking advice on SDN implementation, or looking into the advantages of NFV, ChatGPT-4 can assist in your journey toward designing and provisioning cutting-edge network infrastructures.
Comments:
Thank you all for taking the time to read my article on 'Revolutionizing Network Provisioning: Harnessing the Power of ChatGPT in Network Design Technology'. I'm excited to discuss this topic with you!
This is a fascinating article, Robyn. The potential of leveraging AI and chatbots in network design sounds promising. Can you share some specific use cases where ChatGPT has made a significant impact?
Thanks for your question, Derek. ChatGPT has been successfully used in network provisioning for tasks like automating configuration changes, troubleshooting network issues, and even optimizing network performance in real-time. Its ability to understand network infrastructure and provide tailored recommendations has proved invaluable to network engineers.
I'm a bit skeptical about using AI for such critical tasks. How reliable is ChatGPT in making accurate decisions? Are there any limitations or challenges?
Valid concerns, Jennifer. ChatGPT is highly reliable due to its extensive training on vast amounts of network-related data. However, it's important to note that it still requires human validation and oversight to ensure accuracy. Limitations include potential biases in training data, handling complex scenarios, and the need for continuous improvement to address evolving network challenges.
The concept is interesting, but what happens when ChatGPT encounters a situation it hasn't been trained for? Can it adapt and provide effective solutions in real-time?
Great question, Gregory. While ChatGPT is trained on a wide range of network scenarios, it may encounter unfamiliar situations. In such cases, it can fall back to suggesting general best practices or escalate the issue to a human operator. ChatGPT's real-time learning capabilities also allow it to improve its knowledge base over time, so it becomes more proficient at handling diverse scenarios.
I'm curious about the implementation process of ChatGPT in network design technology. How does one integrate it into existing network infrastructure without disruption?
That's a valid concern, Emily. Implementing ChatGPT requires carefully planning and testing to ensure a smooth integration. The initial step involves training the model on a diverse set of network data. Then, it can be integrated into existing network management systems or deployed as a separate chatbot with appropriate APIs. Continuous monitoring and feedback loops are necessary to refine its performance and ensure compatibility with the existing infrastructure.
I see the benefits of using AI in network provisioning, but what about security concerns? Are there any vulnerabilities associated with integrating ChatGPT into network design technology?
Security is a critical consideration, Mark. When implementing ChatGPT, it's essential to follow industry best practices and ensure robust security measures. Access controls, data encryption, secure APIs, and thorough vulnerability testing should be in place. Regular updates and patches to ChatGPT's software are also necessary to address any potential security vulnerabilities that may arise.
This article raises interesting possibilities for network design. In your opinion, Robyn, how soon do you think widespread adoption of ChatGPT and similar technologies in network provisioning will occur?
Good question, Linda. While adoption of AI technologies varies across industries, the growing demand for efficient network management solutions indicates a promising future for tools like ChatGPT. We can expect a gradual increase in adoption over the next few years as organizations recognize the benefits and successfully overcome any implementation challenges.
I'm impressed with the potential capabilities of ChatGPT in network design. Are there any other emerging AI technologies that could contribute to network provisioning in the future?
Absolutely, Michael. AI technologies like deep learning, reinforcement learning, and generative adversarial networks (GANs) show promise for network provisioning. These technologies can further enhance network automation, anomaly detection, and predictive maintenance. As AI continues to evolve, we can expect even more sophisticated tools to revolutionize network provisioning beyond ChatGPT.
I can see how ChatGPT can simplify network design tasks, but do you think it will eventually replace the need for human network engineers?
That's an important consideration, Diana. While AI technologies like ChatGPT can significantly augment and automate network design tasks, human network engineers will continue to play a vital role. Their expertise, creativity, and ability to handle complex scenarios are irreplaceable. Instead, ChatGPT and similar tools empower engineers by providing them with valuable insights and automating repetitive tasks, allowing them to focus on higher-level decision-making and innovation.
The potential of ChatGPT in network design is exciting, but how accessible is this technology? Will it be limited to large organizations, or can smaller businesses also benefit from it?
Great question, Peter. While large organizations may have the resources to adopt and develop AI technologies like ChatGPT in-house, there are also emerging solutions and cloud services that can make it accessible to smaller businesses. As the technology matures and adoption increases, we can expect more user-friendly implementations and offerings that cater to businesses of various sizes.
I'm curious about the training process of ChatGPT. How do you ensure it understands complex network scenarios and provides accurate recommendations?
Good question, Laura. The training process involves feeding ChatGPT with diverse network-related data, including configuration files, network diagrams, and historical performance data. By exposing the model to a broad range of scenarios, it develops a comprehensive understanding of network design principles and best practices. Human validation and feedback loops are crucial for ensuring accuracy and refining the model's recommendations over time.
I can see the benefits of using ChatGPT for network provisioning, but what about the costs associated with implementation and ongoing support and maintenance? Are there any estimates available?
Financial considerations are significant, David. Implementing ChatGPT involves costs such as model training, infrastructure setup, and integration efforts. Ongoing support, maintenance, and regular updates are also necessary. However, it's challenging to provide precise estimates as costs vary depending on factors like the scale of deployment, complexity of the network infrastructure, and custom requirements. Organizations interested in implementing ChatGPT should consult with AI solution providers to get accurate cost assessments.
How does ChatGPT handle real-time network changes? Can it adapt quickly and provide immediate recommendations?
Good question, Sarah. ChatGPT's real-time learning capabilities enable it to adapt to network changes swiftly and provide immediate recommendations. It continually updates its knowledge base based on new data and feedback. However, real-time adaptation might have some limitations depending on the complexity and novelty of the specific network change. In such cases, human intervention or further analysis might be required before implementing recommendations.
What kind of data is needed to train ChatGPT effectively? Are there any privacy concerns associated with using sensitive network data in the training process?
Valid concerns, Adam. ChatGPT requires network-related data such as configuration files, network logs, and performance metrics for effective training. While privacy is essential, organizations can work with expert AI solution providers who prioritize data security and privacy. Anonymizing sensitive data, using secure storage and transfer methods, and complying with relevant regulations are best practices that minimize privacy risks.
This technology could be a game-changer for network provisioning. However, are there any ethical considerations when leveraging AI for decision-making in network design?
Ethical considerations are crucial, Oliver. While AI technologies like ChatGPT can bring immense benefits, it's important to ensure transparency, accountability, and fairness in their decision-making processes. Measures should be in place to prevent biases, address potential discrimination, and allow human oversight. Organizations adopting AI should adhere to ethical guidelines and establish frameworks that promote responsible AI usage and decision-making in network design.
What are the key challenges organizations might face when implementing ChatGPT in network design technology?
Good question, Sophia. One of the key challenges is ensuring the availability and quality of relevant network data for training the model effectively. Integrating ChatGPT into existing network infrastructure without disruption can also be complex. Additionally, addressing security concerns, providing proper oversight, and managing expectations regarding the limitations of AI automation are important aspects to consider during implementation.
How does ChatGPT handle non-technical users who might not have in-depth knowledge of network design and terminology?
Great question, Daniel. ChatGPT can handle non-technical users by using natural language processing techniques to understand user queries in plain language. It can provide explanations, suggestions, and actionable recommendations in a user-friendly way. By simplifying complex network design concepts, it becomes accessible to a broader range of users without extensive technical knowledge.
Is the implementation of ChatGPT more suitable for specific types of networks, such as enterprise networks, or can it be applied to various network sizes and types?
Good question, Joshua. While enterprise networks can benefit significantly from ChatGPT, the technology is applicable to various network sizes and types. Its scalability and flexibility make it adaptable to different network architectures and infrastructures. Whether it's a small business network or a large-scale enterprise environment, ChatGPT can be tailored and integrated accordingly.
As with any AI technology, ethics and responsible AI usage are important. How can organizations ensure they are using ChatGPT ethically and avoiding any unintended negative consequences?
You're absolutely right, Michelle. To ensure ethical AI usage, organizations should follow established guidelines and frameworks. They should actively work to identify and mitigate biases in training data and decision-making, provide human oversight and intervention, and establish transparency around the involvement of AI in network design. Continuously monitoring and addressing any unintended negative consequences is crucial to ensure ethical and responsible adoption of ChatGPT.
What are some potential benefits of using ChatGPT in network provisioning compared to traditional methods?
Great question, William. Compared to traditional methods, ChatGPT offers several benefits. It enables faster and more accurate network configuration changes, improves troubleshooting efficiency, provides real-time recommendations for optimizing network performance, and reduces manual efforts required for routine tasks. ChatGPT's ability to understand network context and user requirements empowers engineers with actionable insights, resulting in improved network design and efficient provisioning processes.
Given the accelerating pace of technological advancements, how does ChatGPT ensure continuous learning and keep up with evolving network challenges?
Valid point, Amy. ChatGPT has continual learning capabilities. It can incorporate new network data, updated best practices, and user feedback to expand its knowledge base and improve its recommendations. AI solution providers and network engineers work together to ensure ChatGPT remains up-to-date with evolving network challenges. Ongoing training, monitoring, and periodic retraining help keep the model relevant and adaptive.
I'm interested in the implementation timelines for ChatGPT. How long does it typically take to integrate and deploy this technology in a network design environment?
Implementation timelines can vary, Samuel. Factors like the organization's network complexity, availability of training data, infrastructure readiness, and the integration approach affect the timeline. While it's challenging to provide an exact estimate, organizations can expect implementation to take several months. It involves phases like data preparation, model training, integration testing, and gradual deployment with proper monitoring and validation to ensure smooth adoption.
As AI continues to evolve, what areas of network provisioning do you think will benefit the most from future advancements in this field?
Exciting possibilities lie ahead, Natalie. Future advancements in AI can greatly benefit network provisioning in areas like predictive maintenance, automated network security, intelligent capacity planning, and proactive anomaly detection. As AI becomes more proficient at understanding complex network behaviors and optimizing performance, it can revolutionize these aspects, resulting in more efficient and resilient network infrastructure.
What kind of resources and expertise are required to implement ChatGPT effectively? Does it require a highly specialized team?
Good question, Dan. Implementing ChatGPT effectively requires a combination of AI expertise and network design knowledge. Organizations would benefit from a dedicated team that includes AI researchers, data scientists, network engineers, and domain experts. Collaborating with AI solution providers who specialize in network provisioning can also help organizations leverage their specific expertise and ensure a successful implementation.
In the context of network design, can ChatGPT also interact with other systems or network management tools?
Absolutely, Jason. ChatGPT can interact with other systems or network management tools through appropriate APIs and integrations. It can retrieve relevant network data, provide recommendations for specific tools, and even automate certain actions through integration with network automation platforms. Seamless interaction with existing network management infrastructure is a vital aspect of ChatGPT's implementation in network design technology.
Thank you all for your engaging questions and insights on the topic of revolutionizing network provisioning with ChatGPT. It has been a pleasure discussing this exciting technology with you. If you have any more questions, feel free to ask!