Revolutionizing Organizational Budget Planning: Harnessing the Power of ChatGPT for Compensation Structure Design
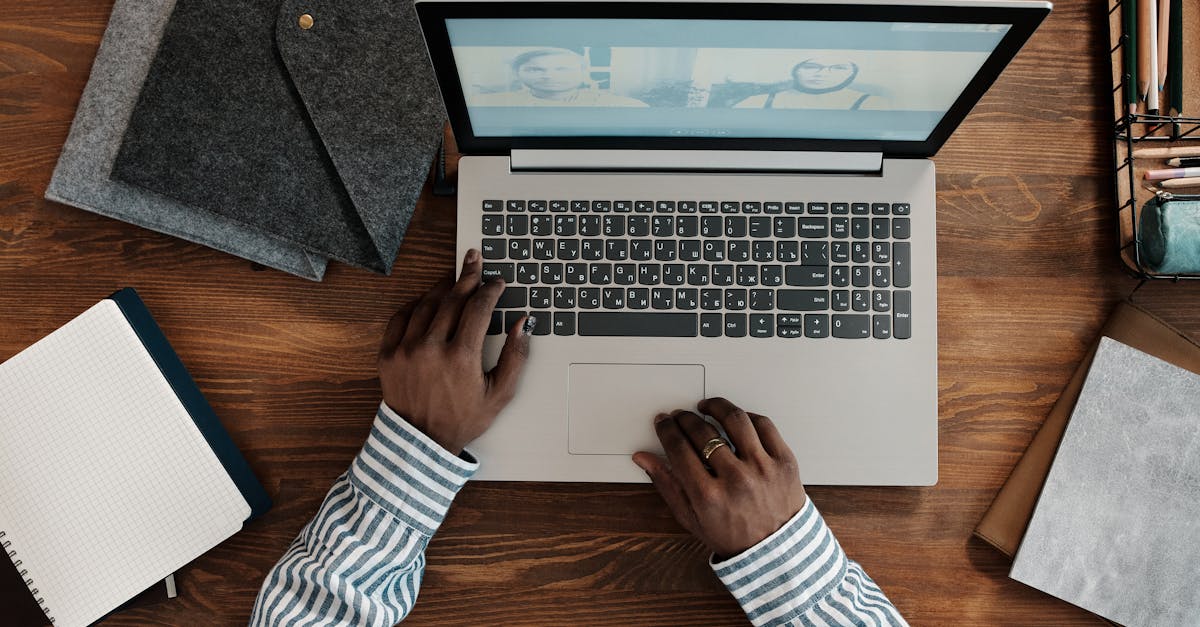
In today's competitive job market, attracting and retaining top talent is crucial for the success of any organization. One key aspect of achieving this goal is to have a well-designed compensation structure that aligns with the company's financial capabilities and ensures fairness among employees. With the advancement in technology, specifically the chatbot technology like ChatGPT-4, organizations now have an efficient tool to help them analyze company data and create realistic and financially healthy compensation budgets.
Technology: ChatGPT-4
ChatGPT-4 is an AI-powered chatbot developed by OpenAI. It is trained on a vast amount of data to understand and respond to natural language inputs. This technology allows organizations to interact with the bot, ask questions related to compensation structure design, and analyze company data to make informed decisions.
Area: Organizational Budget Planning
Organizational budget planning involves the allocation of financial resources to various areas within a company. It is an essential process that impacts the overall financial health of the organization. Compensation expenses typically account for a significant portion of the budget, and having an effective compensation structure is critical to ensure that the allocated budget aligns with the company's goals and values.
Usage: Analyzing Company Data
With the assistance of ChatGPT-4, organizations can leverage its data analysis capabilities to evaluate and understand various aspects of their company data. It can assist in identifying patterns and trends related to compensation, such as salary ranges, employee benefits, bonuses, and performance-based incentives. By analyzing this data, organizations can make more informed decisions when designing their compensation structure and budget allocation.
ChatGPT-4 can provide insights into factors that influence compensation, such as job roles, market trends, geographic location, and employee performance. It can perform complex calculations and simulations to help organizations create compensation budgets that are financially sustainable and competitive in the market.
Furthermore, ChatGPT-4 can also assist in scenario planning, allowing organizations to evaluate the financial implications of different compensation structures. By inputting various parameters and variables, the chatbot can generate projections and recommendations that align with the organization's financial goals and constraints.
Overall, the usage of technology like ChatGPT-4 in compensation structure design for organizational budget planning offers several benefits. It enhances the accuracy and efficiency of data analysis, provides valuable insights, and helps organizations create compensation budgets that are realistic, financially healthy, and competitive in the job market.
It is important to note that while ChatGPT-4 can provide valuable assistance, human expertise and judgment are still vital in the compensation structure design process. The chatbot should be seen as a tool to support decision-making, rather than a replacement for human involvement.
In conclusion, technology like ChatGPT-4 offers organizations the opportunity to leverage AI-powered chatbots to analyze company data and assist in creating realistic and financially healthy compensation budgets. By utilizing such tools, organizations can enhance their compensation structures, attract and retain top talent, and ensure the overall financial success of the organization.
Comments:
Thank you all for joining the discussion on my blog article! I'm excited to hear your thoughts and insights.
ChatGPT has indeed revolutionized various aspects of organizational processes. I can see the potential it holds for compensation structure design as well. What are the specific advantages you envision, Ken?
Great question, Mark! One major advantage is the ability to leverage ChatGPT's natural language processing capabilities to analyze vast amounts of data efficiently. This can help in understanding compensation trends, employee feedback, and market insights to inform better decisions.
Ken, I'm curious about the ethical considerations of using AI for compensation design. How can we ensure fairness and avoid biases in the system?
Valid concern, Julia. Transparency and careful modeling are essential. ChatGPT can assist in identifying potential biases by providing alternative perspectives or flagging any patterns in the data. Additionally, involving diverse stakeholders in the design process can help mitigate biases.
It's fascinating to think about the possibilities. But won't the use of ChatGPT for compensation design remove the human touch and understanding?
Good point, Samuel. The goal isn't to replace humans but to augment and enhance their decision-making process. ChatGPT can provide valuable insights, but ultimately, it's the people who will consider and make the final decisions based on a range of factors.
I'm skeptical about the accuracy and reliability of AI models for such critical tasks. How do we ensure that the recommendations provided by ChatGPT are trustworthy and dependable?
Trust is crucial, Sarah. Validating and fine-tuning the models extensively is essential. Combining AI with human expertise and validation processes can help build reliable systems. It's important to remember that AI models like ChatGPT are tools that need to be carefully utilized.
Ken, have there been any real-world instances where ChatGPT has successfully contributed to compensation structure design? I'd love to learn more about practical applications.
Absolutely, Mark! Several organizations have already started experimenting with chat-based AI systems to assist in compensation planning. They found that it helped them save time, gain deeper insights, and make more informed decisions. I believe it's a promising avenue for innovation.
Ken, do you see any potential downsides or challenges to consider when adopting ChatGPT for compensation structure design?
Certainly, Emma. One challenge is data privacy. Ensuring confidentiality and protection of employees' information is vital. Additionally, the interpretability of AI models can be a concern. Organizations must be able to understand and explain the reasoning behind the model's recommendations.
I'm intrigued by the idea of using AI to handle compensation. Ken, what would be the expected impact on reducing biases and bridging pay gaps within organizations?
Great question, Thomas. While AI can be a valuable tool, it's important to approach it with caution. AI models need to be carefully designed and trained to be unbiased. With proper implementation and considering relevant variables, AI can help reduce biases and bridge pay gaps.
Ken, what kind of challenges should organizations anticipate when implementing ChatGPT for compensation design?
Thanks for the question, Megan. One challenge is managing expectation. AI models like ChatGPT are not magic solutions, but tools to enhance decision-making. Organizations may also face resistance from employees who fear AI replacing humans in such critical matters. Addressing these concerns through effective communication and training is crucial.
Ken, if an organization decides to implement ChatGPT, what steps would you suggest for ensuring a smooth transition and maximizing the benefits?
Excellent question, Mark. Firstly, clearly defining the goals and expectations is important. Organizations should invest sufficient time in training and familiarizing employees with ChatGPT. Gradual implementation, continuous monitoring, and soliciting feedback are effective strategies for a successful transition.
Do you think ChatGPT can also help adapt compensation structures in response to changing market conditions or organizational goals?
Absolutely, Julia! Market trends and organizational goals are dynamic. ChatGPT's ability to analyze data and provide insights can assist in adapting compensation structures accordingly, ensuring they align with both internal and external factors.
I'm still concerned about the potential biases. Even with diverse stakeholders involved, can we guarantee complete impartiality when using ChatGPT for compensation planning?
Great point, Samuel. It's challenging to guarantee complete impartiality, but by involving diverse stakeholders, balancing their perspectives, and proactively monitoring the AI system, we can minimize biases and strive for fairness. Continuous assessment and feedback loops are essential.
Ken, what kind of resources and training would be necessary for organizations to effectively adopt and utilize ChatGPT for compensation design?
Good question, Sarah. Adequate resources for training employees and ensuring data quality are crucial. Organizations should collaborate with AI experts and provide ongoing support to users of ChatGPT. Effective training programs and documentation can also facilitate a smooth adoption process.
Are there any limitations to consider when using ChatGPT for compensation design? What are the boundaries of its capabilities in this context?
Excellent question, Emma. ChatGPT's capabilities are impressive, but it's important to recognize its limitations. It relies on the data it's trained on, and if that data is biased or incomplete, it may inadvertently perpetuate those biases. Additionally, the complexity of compensation design may require other factors beyond ChatGPT's scope.
Ken, how can organizations strike the right balance between AI-driven decision-making and human judgment in compensation structure design?
Finding the right balance is crucial, Thomas. AI can provide valuable insights, but ultimate decision-making should involve human judgment. Organizations should create frameworks that combine AI recommendations with human expertise, allowing final decisions to consider a range of factors beyond what AI models can currently capture.
Ken, is there a risk of overreliance on ChatGPT's recommendations, potentially leading to a loss of critical thinking and creativity in compensation structure design?
Valid concern, Megan. Overreliance can be a risk with any technology. Organizations should ensure that ChatGPT's recommendations are reviewed critically and evaluated alongside human expertise. Encouraging creative thinking and maintaining the humans-in-the-loop approach is essential.
Would small organizations benefit as much as large ones from implementing ChatGPT for compensation design? Or are there specific challenges for smaller entities?
Excellent question, Samuel. While smaller organizations may have different scaling challenges, they can still benefit from ChatGPT. Adjusting the implementation to suit their specific needs, involving key stakeholders, and seeking support from AI experts can help small organizations leverage AI for compensation design.
Ken, when it comes to feedback loops, how often should organizations evaluate and update the ChatGPT model used for compensation planning to ensure continuous improvement?
Regular evaluation and updates are crucial, Julia. The frequency depends on various factors, including the evolving needs of the organization, changing market dynamics, and technological advancements. Establishing a feedback loop and assessing the model's performance periodically can lead to continuous improvement.
In your experience, Ken, what are the key success factors or best practices for organizations adopting ChatGPT for compensation structure design?
Great question, Mark. Clearly defining goals, fostering collaboration between HR, data science, and other relevant teams, maintaining transparency, validating data, and involving stakeholders are some of the key success factors. A proactive approach to address concerns, continuous learning, and iterative improvements also contribute to successful adoption.
Ken, what do you think the future holds for AI in compensation planning? Can we expect more advanced models to emerge?
Absolutely, Sarah. AI is a rapidly evolving field, and we can expect more advanced models tailored for compensation planning. As technology progresses, models like ChatGPT will become even more sophisticated, incorporating better language understanding, data interpretation, and addressing current limitations.
I've enjoyed this discussion. Thanks for sharing your insights, Ken, and thank you all for the valuable exchange of ideas.
Thank you, Emma! It was a pleasure engaging with all of you. Your questions and perspectives have given me further food for thought.
Ken, your article has shed light on an intriguing implementation of AI. Thank you for sharing your knowledge and experience.
You're most welcome, Thomas! I'm glad you found the article informative.
Thank you, Ken, for addressing our questions and providing valuable insights. It was a stimulating discussion.
You're welcome, Megan! I'm delighted that the discussion sparked your curiosity.
Thanks, Ken, for taking the time to share your expertise with us. Looking forward to future discussions on emerging technologies!
Thank you, Samuel! I appreciate your engagement and enthusiasm. Absolutely, let's continue exploring innovations together.
Ken, you've provided valuable insights into AI adoption for compensation design. Thank you for sharing your knowledge.
You're most welcome, Julia! I'm happy to have shared my thoughts with all of you.
Thank you, Ken, for your comprehensive responses. It was a highly informative discussion.
I agree with Samuel's concern. The human touch is crucial in compensation design as it involves understanding individual circumstances and motivations.
I'm interested to know how the implementation of ChatGPT can impact the efficiency of compensation structure design processes.
Can ChatGPT also assist in visualizing and presenting compensation structures to stakeholders, along with the analysis and design phases?
Besides resistance, how can organizations manage the fear that employees may have about AI involvement in compensation design?
Considering external factors like market conditions seems necessary, but how can ChatGPT effectively incorporate internal factors specific to different organizations?
To enhance transparency, should organizations publish the rationale behind the compensation decisions made based on ChatGPT's recommendations?
How can organizations strike the right balance between being data-driven and considering subjective factors like employee morale and experience when using AI for compensation design?