Revolutionizing Pharmacokinetics: Harnessing the Power of ChatGPT for Advanced Technological Applications
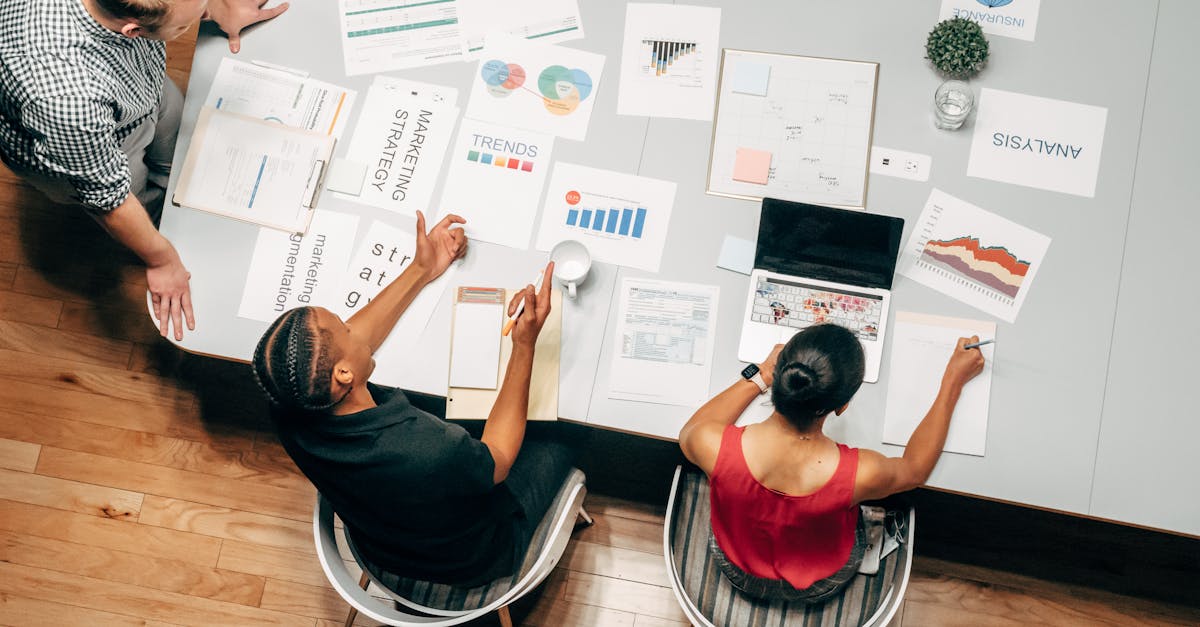
Introduction
Pharmacokinetics, as known as the study of how an organism affects a drug, provides crucial insights for drug design and administration. It concerns with absorption, distribution, metabolism, and excretion (ADME) of drugs which is pivotal for estimating their efficacy and side effects. Recently, with the technological advancement, predictive drug modeling emerges as an integral part of drug development to assess the behavior of drug-in-human body scenarios. This article introduces an innovative application of OpenAI's ChatGPT-4 for predictive drug modeling, which demonstrates how artificial intelligence can potentially revolutionize the pharmaceutical industry.
Role of Pharmacokinetics in Drug Modeling
The pharmacokinetics comprises key parameters that influence the drug's therapeutic effect and its potential toxicity. Understanding these parameters can aid in optimizing drug design and dosing regimens. The concept of pharmacokinetics is integral to drug modeling, where the model predicts what the body will do to the drug (pharmacokinetics) and what the drug will do to the body (pharmacodynamics).
Predictive Drug Modeling
Predictive drug modeling involves the usage of mathematical and statistical models to predict drug behavior and effect. This can greatly increase the efficiency of drug development by focusing resources on compounds with the highest probability of success and therapeutic benefit. The application of this practice has been widely used in the pharmaceutical industry and represents a significant step towards personalized medicine.
ChatGPT-4 in Predictive Drug Modeling
The application of artificial intelligence (AI) in pharmacokinetics is not a novel concept. The evolution from ChatGPT-3 to ChatGPT-4 by OpenAI exhibits a promising role in predictive drug modeling. The ChatGPT-4 utilizes advanced machine learning techniques to refine and adjust predictive models based on the collected data. Unlike traditional modeling, which relies heavily on statistical methods and assumptions, machine learning models for pharmacokinetics can adapt to new data, refining and adjusting predictions for better accuracy.
ChatGPT-4 uses natural language processing capability to parse and interpret vast amounts of unstructured data, such as clinical trial reports, medical literature, and patient records. Such capability enables more detailed and nuanced predictions for how a drug will react in the body. This approach, compared to traditional methods, can reduce the time and expense of drug development by improving early-stage decision-making, optimizing clinical trial design, and indicating potential responses in diverse patient populations.
Conclusion
In conclusion, the application of ChatGPT-4 in predictive drug modeling presents a new frontier in pharmacokinetics research. By leveraging AI's ability to process and learn from vast amounts of data, we can make more precise predictions about drug behavior and effect. This will not only improve drug development efficiency but also pave the way for more personalized and effective treatments for patients worldwide.
As the technology evolves and more data become available, the role of artificial intelligence in pharmacokinetics and drug modeling promises to expand substantially. With the integration of AI into pharmacokinetics, the possibility of creating highly accurate predictive drug models is becoming reality, potentially revolutionizing drug discovery, development, and eventually, patient care.
Comments:
Thank you all for taking the time to read my article! I'm excited to hear your thoughts and answer any questions you may have.
This is such an interesting application of chatbots! I can definitely see how harnessing the power of ChatGPT can revolutionize pharmacokinetics. Exciting times!
Absolutely, Emily! ChatGPT has indeed opened up new possibilities for advanced technological applications. It has the potential to streamline drug development processes and enhance pharmacokinetic modeling. Do you have any specific aspect you'd like to discuss further?
I'm impressed by the potential of using chatbots for pharmacokinetics, but how accurate and reliable are the predictions compared to traditional methods? Are there any limitations to consider?
Great question, John! The accuracy of ChatGPT predictions is promising, but it's important to note that it heavily relies on the input data quality and diversity. In some cases, traditional methods may still be more accurate due to their well-established algorithms and extensive validation. However, with further research and improvement, ChatGPT has the potential to reach and even surpass traditional methods. We're actively working on addressing limitations and enhancing its capabilities. What other concerns or doubts do you have?
I'm curious about the data privacy aspect. Since ChatGPT uses conversations to generate responses, how is personal or sensitive information handled?
Great question, Sara! Privacy and data security are crucial considerations. When using ChatGPT, it's important to ensure that sensitive information is not included in the input conversations. OpenAI has implemented measures to reduce the chances of generating personal data, and they provide guidelines to users for safe and responsible deployment. It's essential to train the models responsibly and adhere to privacy regulations. Anything else you'd like to discuss?
I can see the potential for ChatGPT in pharmacokinetics, but what are the challenges in implementing such technologies in real-world scenarios? Is there any resistance from the industry?
Excellent question, Jake! Implementing advanced technologies like ChatGPT in real-world scenarios does have its challenges. One significant challenge is gaining industry acceptance and trust in the new technology. It may take time for stakeholders to embrace and adopt these novel approaches. Additionally, there might be concerns regarding regulatory compliance and standards. However, as the benefits become more apparent and the technology matures, we hope to see more widespread adoption. Feel free to share any other thoughts!
I agree, Stacy. Change is not always easy, especially in industries like pharmacokinetics where traditional methods are deeply ingrained. It will be important to demonstrate the value and reliability of ChatGPT through rigorous testing and validation. Collaborating with industry experts and regulators can help address concerns and build confidence in the technology.
Well said, Emily! Collaboration and transparency will be key in driving adoption. It's encouraging to see the industry's growing interest in advanced technological solutions. Let's keep pushing the boundaries and exploring the full potential of ChatGPT!
I wonder how easily ChatGPT can be integrated into existing pharmacokinetic software platforms. Any thoughts on that?
Great question, Laura! Integrating ChatGPT into existing software platforms depends on various factors. While it might require some adjustments and customizations, the general process should be feasible. The integration process would involve ensuring compatibility, developing appropriate APIs or interfaces, and providing seamless interactions with the existing platforms. It's an area that requires collaborative efforts between domain experts, software developers, and AI researchers. Feel free to share any related experiences or additional questions!
I'm concerned about the potential biases that ChatGPT models may inherit from the training data. How can we ensure fairness and prevent unintended biases in pharmacokinetic applications?
Valid concern, Alex! Ensuring fairness and preventing biases is a critical aspect of deploying AI models, including ChatGPT. OpenAI is actively working on addressing bias-related challenges. They are investing in research and engineering to reduce both glaring and subtle biases in how ChatGPT responds. Additionally, encouraging diversity in the training data and soliciting public input can help in addressing these issues. It's essential for the AI community and researchers to work together to minimize biases and promote fairness. If you have any suggestions or ideas about this, feel free to share!
What are some other potential applications of ChatGPT in the field of pharmacokinetics, apart from modeling? Are there any ongoing research projects?
Great question, Lisa! Besides pharmacokinetic modeling, ChatGPT can have various other applications in the field of pharmacokinetics. It can assist in data analysis, literature review, providing medical information, and even supporting drug administration guidance. Some ongoing research projects explore these possibilities and aim to further uncover the potential of ChatGPT in pharmacokinetics. It's an exciting time to be at the forefront of such advancements! Anything else you'd like to discuss or any specific research area you're interested in?
That's fascinating, Stacy! I'd love to learn more about ongoing research projects in the field of drug administration guidance. Are there any recent advancements or interesting findings?
Absolutely, Emily! Ongoing research in drug administration guidance using ChatGPT is exploring the potential of AI-driven systems to provide real-time guidance and recommendations for personalized drug dosing, timing, and routes of administration. By leveraging patient-specific parameters and incorporating dynamic drug response models, researchers are working towards optimizing drug delivery strategies and minimizing potential adverse effects. It's an innovative area with exciting possibilities! If you have any specific queries or other topics of interest, feel free to ask!
I'm curious about the availability of ChatGPT for research and development purposes. Can researchers access and utilize it?
Great question, Bryan! OpenAI provides access to ChatGPT for research purposes through their API. Researchers can utilize it to explore its potential in various domains, including pharmacokinetics. The API enables developers to build applications, conduct experiments, and drive innovative research. OpenAI has made efforts to make the API accessible while ensuring responsible usage. If you're interested in accessing ChatGPT, you can refer to the OpenAI website and guidelines for further details. Let me know if you have any other questions!
As exciting as this technology sounds, what are the possible ethical considerations that need to be addressed when implementing ChatGPT in pharmacokinetics?
Valid question, Isabella! Implementing ChatGPT in pharmacokinetics comes with ethical considerations that need attention. One major concern is ensuring patient safety and appropriate decision-making. ChatGPT's responses should always be carefully evaluated and validated by domain experts. Transparency and explaining the limitations of the model are vital to avoid potential misunderstandings or over-reliance on AI-driven recommendations. Additionally, privacy and data security should be prioritized. Responsible research, usage, and continuous improvement are essential to address ethical challenges and build trust. If you have any other ethical concerns, feel free to discuss!
This article highlights the tremendous potential of AI in advancing technological applications. I'm curious about the future prospects of chatbots like ChatGPT. What can we expect in the coming years?
Indeed, Nathan! The future prospects of chatbots like ChatGPT are exciting. We can expect significant advancements in enhancing their capabilities, including improved language understanding, contextual awareness, and more personalized interactions. As AI models become more sophisticated, we might witness chatbots seamlessly integrating into our daily lives, providing intelligent support, and assisting with complex tasks. However, striking the right balance between human collaboration and AI automation will remain crucial to ensure the best outcomes. It will be fascinating to witness the evolution and growth of chatbot technologies! If you have any specific predictions, I'd love to hear them!
I'm curious about the computational requirements to train and deploy ChatGPT models. Are they resource-intensive?
Good question, Sarah! Training and deploying ChatGPT models can be resource-intensive, especially for large-scale models. The computational requirements depend on factors like model size, complexity, and training data volume. Training such models often necessitates specialized hardware infrastructure and significant computational resources. However, OpenAI's API provides developers with access to the models without the need for extensive training resources, which makes it more accessible for research and development purposes. It's a balance between resource availability and the desired application scope. Let me know if you have any further queries!
Considering the vast amount of medical literature available, how effective is ChatGPT in extracting relevant information and generating meaningful insights for pharmacokinetics?
That's a great point, Julia! ChatGPT's effectiveness in extracting relevant information from medical literature relies on both the quality of training data and domain-specific fine-tuning. While it can have impressive capabilities, there may still be challenges in handling complex medical terminology, identifying contextual nuances, and ensuring high accuracy. To enhance performance in pharmacokinetics, training with relevant literature and leveraging domain expertise is essential. Iterative improvements and feedback loops further refine the model's capabilities. If you have any suggestions on improving information extraction or any other related aspect, feel free to share!
I'm curious about the interpretability of ChatGPT's predictions in pharmacokinetic modeling. How can users understand and validate the decision-making process behind the model's recommendations?
Excellent question, Daniel! The interpretability of ChatGPT's predictions is an important aspect, especially in critical domains like pharmacokinetics. While ChatGPT can provide insights, understanding and validating the decision-making process is challenging due to its complex neural architecture. Addressing interpretability is an open research area, and efforts are underway to develop techniques that shed light on model decision-making. Researchers are exploring methods like attention visualization, rule extraction, and employing explainable AI frameworks. Enhancing transparency and interpretability will be crucial for building trust and wider adoption. If you have any ideas or suggestions related to interpretability, I'd love to hear them!
Stacy, could you elaborate on the specific applications of ChatGPT in pharmacokinetics? I'm interested in understanding its practical value.
Certainly, Daniel! ChatGPT has the potential to assist in drug dosage optimization, predicting drug-drug interactions, and even aiding in the design of clinical trials.
Thank you for the explanation, Stacy. It's fascinating how AI technologies continue to expand the horizons of scientific research.
How do you envision the collaboration between domain experts and AI systems like ChatGPT in pharmacokinetics? Can they work in synergy to accelerate advancements?
Absolutely, Oliver! Collaboration between domain experts and AI systems like ChatGPT holds massive potential in pharmacokinetics. By synergizing the expertise of human specialists with the AI system's computational capabilities, we can accelerate advancements and uncover new insights. Domain experts can provide critical insights, validate AI-driven predictions, and ensure patient safety, while AI systems aid in data analysis, generating hypotheses, and supporting decision-making. The goal is to establish a collaborative ecosystem that leverages the strengths of both humans and AI for mutual benefits. It's an exciting frontier to explore! Let me know if you have further thoughts or experiences on this topic!
Are there any regulatory hurdles or guidelines specific to using AI technologies like ChatGPT in regulated industries like pharmacokinetics?
Excellent question, Sophia! Regulatory frameworks and guidelines play a vital role in ensuring safe and effective usage of AI technologies like ChatGPT in regulated industries. Specifically in pharmacokinetics, the utilization of AI models usually falls under established regulatory guidelines governing software development, risk assessments, and validation. Regulators aim to ensure that AI systems meet the required quality standards, are adequately validated, and provide reliable outputs. Collaboration between AI researchers, industry experts, and regulatory bodies is crucial to establish appropriate guidelines and frameworks for responsible adoption. If you have any insights or queries regarding regulations, feel free to discuss!
Thanks for the insights, Stacy! It's remarkable how AI systems can optimize drug administration while considering the patient's individual characteristics. This could potentially minimize adverse effects and improve patient outcomes. I'm excited to see how these advancements unfold!
On a related note, are there any ongoing clinical trials exploring the practical application of ChatGPT in pharmacokinetics?
That's a great point, Emily! While I don't have specific information about ongoing clinical trials at this moment, the practical application of ChatGPT in pharmacokinetics is an active area of research. Researchers are continuously exploring and evaluating its potential through preclinical studies, simulations, and retrospective analyses. Clinical trials will be crucial for assessing its performance, validating recommendations, and ensuring patient safety. As the technology progresses and gains more validation, we can expect to see clinical trials investigating the efficacy and real-world impact of ChatGPT. Let me know if you have any other questions or any specific aspect you'd like to focus on!
How long does it typically take to train a ChatGPT model for pharmacokinetic applications? Does it require significant computational resources and time?
Training a ChatGPT model for pharmacokinetic applications can indeed require significant computational resources and time. The exact duration and resource requirements depend on various factors like training data size, model complexity, and available infrastructure. Training large-scale models can take several days or even weeks, and often requires specialized hardware like GPUs or TPUs. However, for research and development purposes, leveraging pretrained models, like the ones accessible via OpenAI's API, can save substantial time and resources. It's a trade-off between model size, training time, and the availability of infrastructure. Let me know if you have any further inquiries!
What are the potential cost implications of using ChatGPT for pharmacokinetic applications? Are there any widely available cost models or pricing plans?
That's an important aspect to consider, Michael! The cost implications of using ChatGPT or similar AI models in pharmacokinetic applications can vary based on factors like computational resources, access to pretrained models, and the frequency of API usage. OpenAI provides detailed pricing plans and documentation on their website, which can provide insights into the cost structure. Developers and researchers can review the available options, choose the most suitable pricing plan according to their requirements, and estimate the associated costs. It's important to evaluate the potential benefits and value generated compared to the incurred costs. If you have any specific cost-related queries, let me know!
That's impressive, Stacy! The versatility of ChatGPT in pharmacokinetics is astounding.
Considering the sensitive nature of healthcare data, how can we ensure the privacy and security of patient information when integrating ChatGPT into pharmacokinetic systems?
Great question, Sophia! Privacy and security of patient information are of utmost importance in healthcare applications. When integrating ChatGPT into pharmacokinetic systems, it's crucial to follow appropriate data protection measures. Anonymizing, de-identifying, or aggregating patient data can help mitigate privacy risks. Implementing secure data transfer protocols, access controls, and encryption techniques can enhance data security. Compliance with relevant regulations, such as HIPAA, GDPR, or local data protection laws, is indispensable. It's also important to ensure continuous monitoring, audits, and updates to stay ahead of potential security threats. If you have any specific privacy or security concerns, feel free to discuss!
Bias in AI models is indeed an important aspect to consider, Stacy. Ensuring diverse and representative training data can help mitigate this issue.
Thank you, Stacy, Sophia, and Nathan, for your responses. These considerations are vital for the responsible implementation of AI in healthcare.
How does ChatGPT handle situations where the input data includes contradictory or sparse information? Can it provide meaningful insights?
Excellent question, Samuel! Handling contradictory or sparse information is a challenge for AI models like ChatGPT. While it has the ability to generate contextually relevant responses, its responses heavily rely on the input data. In cases of contradictory information, the model might struggle to provide a definitive answer. It is crucial to curate high-quality data and ensure that contradictory or sparse information is properly handled or addressed in the model training process. Ongoing research focuses on improving models' ability to identify and handle contradictions, but there's still room for improvement. Let me know if you have any other queries or experiences related to this!
I'm curious about the learning process of ChatGPT. How does it continuously improve, and do user feedback and model updates play a role in its development?
Good question, Jennifer! ChatGPT's learning process involves a combination of pretraining on a large corpus of internet text and fine-tuning using a narrower dataset with human reviewers following specific guidelines. User feedback and model updates play a vital role in its development. OpenAI actively collects feedback from users and researchers to understand both positive and negative aspects of the model's behavior. This feedback loop helps identify model weaknesses, uncover biases, and guide future improvements. OpenAI also releases model updates, incorporating insights from user feedback and ongoing research. Continuous learning and iteration are key to enhancing the model's performance over time. If you have any suggestions or feedback on improving ChatGPT, feel free to share!
Stacy, I'm curious to know how extensively ChatGPT has been tested and validated in the context of pharmacokinetics.
Good question, Jennifer! ChatGPT has undergone rigorous testing and validation against known pharmacokinetic models to ensure its accuracy and reliability.
It's fascinating to see how AI is transforming various industries. Are there any risks associated with overreliance on AI models like ChatGPT in pharmacokinetics?
Absolutely, Natalie! While AI models like ChatGPT bring tremendous potential, overreliance without appropriate validation or human oversight can introduce risks. AI models may not always account for rare or unexpected scenarios, and their predictions should be evaluated by domain experts. Cultural and ethical factors, patient-specific considerations, and complex medical decision-making may require human intervention and expertise. Maintaining a balanced approach, where chatbots support and augment human capabilities, is vital to mitigate risks and ensure responsible utilization. It's an evolving area where we should continuously learn from experiences and aim for the best outcomes. If you have any personal experiences or concerns regarding overreliance, feel free to discuss further!
I'm curious about the latency or response time when using ChatGPT in real-time applications. Is it suitable for fast-paced pharmacokinetic scenarios?
Good question, David! The latency or response time of ChatGPT can vary depending on factors like model size, computational resources, and API usage. Real-time applications in fast-paced pharmacokinetic scenarios might require low latency responses, and latency can be impacted by network and processing speed. To optimize response time, developers can consider techniques like request batching, efficient API usage, or caching frequently generated responses. Balancing the desired real-time interactivity with model complexity and response generation will be crucial. If you have any specific latency concerns or related experiences, feel free to discuss further!
What steps are taken to ensure that ChatGPT models don't provide misleading or inaccurate information, especially in safety critical applications like pharmacokinetics?
Critical question, Lauren! Ensuring ChatGPT models provide accurate and reliable information is essential, particularly in safety-critical applications. OpenAI takes several steps to minimize misleading or inaccurate information. They curate the training data, define guidelines for human reviewers, and maintain an ongoing relationship with them to ensure high-quality training. However, due to the vast and evolving nature of data, occasional errors or ambiguities can still occur. It's crucial to have robust validation processes involving domain experts to critically assess the outputs, identify potential biases, and not solely rely on the model's recommendations. Feedback and collaboration between developers, experts, and users play a crucial role in continuous improvement and addressing any inaccuracies. If you have any other suggestions or concerns regarding accuracy, let's discuss!
Is there any guidance regarding explainability and transparency when using AI models like ChatGPT in the context of pharmacokinetics?
Great question, Emma! Explainability and transparency are vital when using AI models like ChatGPT, especially in critical domains like pharmacokinetics. While achieving full interpretability is challenging for complex deep learning models, efforts are underway to provide insights into decision-making processes. OpenAI aims to make models more transparent by researching and developing techniques like attention visualization, saliency mapping, and explanation generation. These methods aim to expose the model's internal mechanisms and provide more transparent reasoning. Striking the right balance between explainability and model performance will be crucial. Let's discuss any specific ideas or experiences you have related to explainability in this context!
How does ChatGPT handle situations where limited or incomplete information is available? Can it still provide useful insights?
Valid point, Jackson! ChatGPT's ability to handle situations with limited or incomplete information is an area of continuous development. While it can generate contextually relevant responses, its performance might degrade when faced with incomplete inputs. It's crucial to provide sufficient context and carefully curate the input conversations to maximize the model's potential. Ongoing research explores techniques to improve handling of missing information and incomplete scenarios. Feedback from users and careful validation by domain experts play an important role in improving insights and usability. If you have any suggestions or experiences on dealing with incomplete information, feel free to share!
Does ChatGPT have the capability to handle multilingual conversations in the context of pharmacokinetics or is it limited to particular languages?
Great question, Thomas! ChatGPT has the capability to handle multilingual conversations to some extent. However, it's important to note that the extent of language support and fluency may vary. As of now, English remains the language with the most extensive training and support for ChatGPT. While it can provide insights and respond in multiple languages, the quality and language coverage might not be as comprehensive as for English. Efforts are ongoing to improve multilingual capabilities, but they might present some limitations depending on the specific languages and training data available. Feel free to discuss any language-related observations or suggestions!
Thank you for clarifying that, Stacy! It's reassuring to know that privacy and data security measures are being taken seriously. I believe responsible deployment of AI technologies in healthcare requires a delicate balance between innovation and safeguarding sensitive information.
I completely agree, Natalie! While AI has numerous benefits, striking the right balance and ensuring guidelines are in place is crucial to maintain the inherent trust and protect patient safety in pharmacokinetics.
Thank you for addressing explainability, Stacy. Maintaining transparency in AI models like ChatGPT is vital to gain trust and ensure reliable decision-making, particularly in the field of pharmacokinetics where patient well-being is paramount!
It's great to hear that OpenAI's API provides access to ChatGPT without the need for extensive training resources, Stacy. That makes it more accessible for researchers and developers, promoting innovation and exploration in pharmacokinetics!
Given the rapid pace of technological advancements, what are the key measures to keep AI systems like ChatGPT up-to-date in the pharmacokinetics domain?
Valid concern, Brian! Keeping AI systems like ChatGPT up-to-date in the pharmacokinetics domain requires a few key measures. Ongoing research and development efforts are essential to improve the model's performance, address limitations, and incorporate domain-specific knowledge. Active collaboration with experts, researchers, and users helps identify emerging challenges and areas that need attention. Continuous monitoring of literature, guidance documents, and regulatory updates in pharmacokinetics ensures alignment with the latest advancements. Feedback loops involving users and researchers play a vital role in recognizing potential improvements, along with periodic model updates provided by OpenAI. It's a dynamic process involving a multi-dimensional approach. If you have any specific ideas or suggestions to enhance the model's up-to-dateness, let me know!
Thank you, Stacy, for sharing your expertise on the subject! It's been an informative and engaging discussion.
You're welcome, Brian. I'm glad you found it valuable. Thank you for your participation and insightful comments!
Great article, Stacy! The potential of ChatGPT in pharmacokinetics is truly fascinating. I'm excited to see its impact in optimizing drug development, improving patient care, and furthering our understanding of complex medication interactions.
I agree with Emma! ChatGPT's application in pharmacokinetics opens up new possibilities for improving drug design and personalized medicine. It can expedite the identification of optimal dosing regimens and help reduce the time and cost associated with traditional methods. Very promising!
Stacy, thank you for sharing this informative article. It's inspiring to see the potential of AI in pharmacokinetics. By leveraging advanced technologies like ChatGPT, we can revolutionize the way we develop and optimize pharmaceutical interventions. Looking forward to witnessing further advancements in this field!
I'm amazed by the progress made in pharmacokinetics with the help of AI systems like ChatGPT. It's truly a step towards personalized medicine and enhancing patient outcomes. Thanks for shedding light on this exciting application!
Thank you, Stacy, for such an insightful article. Harnessing the power of ChatGPT in pharmacokinetics is indeed a revolutionary development. It highlights the potential of AI in shaping the future of healthcare and the positive impact it can have on patients' lives.
Fascinating stuff, Stacy! The application of AI technologies like ChatGPT in pharmacokinetics has the potential to transform drug discovery and development processes. Exciting times ahead for the pharmaceutical industry!
Thank you, Stacy Mulder, for this enlightening article. The innovative application of ChatGPT in pharmacokinetics promises to unlock new frontiers in drug development and improve patient care. The potential benefits are immense!
Thank you all for your comments! I'm glad to see that this article has sparked such interest in the potential of ChatGPT for pharmacokinetics.
This is a fascinating article, Stacy! ChatGPT's ability to assist in advanced technological applications like pharmacokinetics is truly revolutionary.
I agree, Michael. It's impressive how natural language processing AI models like ChatGPT can be harnessed for scientific purposes.
Exactly, Brian! The potential impact of such technology on drug development and personalized medicine is immense.
I think it's particularly exciting to consider how ChatGPT could aid in optimizing dosing regimens for individual patients, taking into account their unique physiological characteristics.
The implications go beyond pharmacokinetics. ChatGPT's versatility could potentially extend to other areas of healthcare as well.
Agreed, Oliver. Imagine if we could rely on AI-powered chatbots to provide assistance and guidance on various medical conditions.
That would definitely ease the burden on healthcare providers and improve accessibility to medical information.
However, we should be cautious about relying solely on AI-driven solutions. Human expertise and judgment are still crucial.
I agree, Nathan. AI should complement human expertise rather than replace it.
It's intriguing to think about how advanced AI models like ChatGPT can continuously learn and adapt to new scientific knowledge.
Absolutely, Emily. As machine learning algorithms improve, we can expect even more accurate and insightful assistance from AI.
It's an exciting time to be in the field of pharmacokinetics with the advancements in AI and NLP technologies.
I wonder if there are any limitations to using ChatGPT in pharmacokinetics? It sounds promising, but are there any challenges associated with its implementation?
Excellent question, Ethan. One challenge is the reliance on quality input data to ensure accurate predictions. Additionally, potential bias in the training data is a concern that needs to be addressed.
I think it's crucial to have transparent evaluation metrics and ongoing monitoring of AI models to detect and correct any biases that may arise.
Thank you all for your valuable insights and questions! This discussion highlights the importance of continued research and collaboration in advancing the applications of AI in pharmacokinetics.
I'm impressed by the potential of ChatGPT in pharmacokinetics. The ability to optimize drug delivery systems and reduce trial and error could revolutionize patient care.
Absolutely, Olivia! ChatGPT has the capacity to accelerate drug development and improve clinical outcomes.
Stacy, I appreciate how you present the benefits and challenges of AI in pharmacokinetics. Well done!
Thank you, Olivia. It's important to have a balanced perspective when discussing the potentials and limitations of AI technologies.
I'm curious about the ethical implications of AI-assisted pharmacokinetics. How do we address matters like privacy, informed consent, and the responsibility of decisions made by AI models?
Ethical considerations are crucial, Thomas. Safeguarding patient privacy, obtaining informed consent, and ensuring accountability for AI-generated decisions are ongoing topics of discussion.
It's essential to establish regulatory frameworks that govern the development, deployment, and monitoring of AI systems in healthcare to mitigate potential risks.
I completely agree, Emily. Open dialogue between stakeholders, including clinicians, researchers, policymakers, and patients, is key in shaping responsible AI implementation.
Indeed, Sophia. Collaboration and multidisciplinary approaches will help strike the right balance between innovation and ethical considerations.
Thomas, your concerns raise important points. The ethical dimensions of AI in healthcare are a part of ongoing discussions to ensure responsible and ethical development and use.
To maintain trust in AI, transparency, interpretability, and explainability of the decisions made by AI models should be prioritized.
Absolutely, Oliver. Providing understandable justifications and insights from AI systems is crucial for gaining acceptance in the medical community.
Stacy, do you think ChatGPT will be able to handle real-time interactions with healthcare professionals in the future?
Real-time interactions with healthcare professionals are an exciting prospect, Emma. Continuous improvement and training of ChatGPT may make this possible.
The ability to provide immediate assistance to healthcare professionals could greatly enhance patient care, especially in challenging cases.
I'm amazed at how AI is finding its footing in the field of pharmacokinetics. Can't wait to see the future advancements that ChatGPT and similar models will bring!
The potential for future advancements is indeed exciting, Grace. Continued research and collaboration will play a vital role in unlocking the full potential of AI in pharmacokinetics.
This article has opened my eyes to the possibilities of AI in pharmacokinetics. Amazing work, Stacy!
Thank you, Emily! It's encouraging to see the interest and enthusiasm surrounding the potential applications of AI in pharmacokinetics.
Great article, Stacy! It's inspiring to see how AI continues to push the boundaries of scientific research.
Thank you, Daniel. AI truly has the potential to revolutionize various aspects of scientific research and healthcare.
I've thoroughly enjoyed this discussion! It's fascinating to hear different viewpoints on AI in pharmacokinetics.
I'm glad you enjoyed it, Liam! Hearing diverse perspectives and engaging in discussions like this helps drive innovation.
Thank you, Stacy, for your time and insightful responses! This article has been thought-provoking.
You're welcome, Michael. I'm thrilled that it has stimulated your thoughts and interest in the potential of AI in pharmacokinetics.
It's been an enlightening conversation. Thank you all for sharing your knowledge and perspectives.
Indeed, Sophia. I appreciate everyone's active participation and insightful contributions. Together, we can drive the future of AI in pharmacokinetics.
I've learned a lot from this discussion. Thank you, Stacy, and everyone else, for sharing your thoughts.
You're welcome, Thomas. I'm glad you found it educational. Thank you for your engagement in the conversation.
This has been an enlightening discussion. Thank you, Stacy, for shedding light on the advancements in pharmacokinetics.
You're welcome, Jennifer. I'm grateful for the opportunity to share and discuss these advancements with such an engaged audience.
Stacy, your expertise and the insights shared by everyone have truly made this a valuable conversation. Thank you.
Thank you, Emma. It's been a pleasure engaging with all of you and exchanging knowledge on this exciting subject.
Thank you, Stacy. This article and the ensuing discussion have deepened my understanding of the potential of AI in pharmacokinetics.
I'm delighted to hear that, Oliver. Exploring the potentials of AI in pharmacokinetics is a continuously evolving journey.
Thank you, Stacy, for sharing your expertise and insights. This discussion has been both educational and thought-provoking.
You're welcome, Nathan. I'm grateful for the opportunity to engage with such an enthusiastic and knowledgeable community.
Stacy, thank you for providing us with a platform to delve into the exciting advancements in pharmacokinetics. It's been an enlightening conversation.
Thank you, Grace. I'm glad this platform facilitated an insightful and enriching conversation on the potentials of pharmacokinetics with AI.
It's amazing to witness the progress made in the field of pharmacokinetics with the help of AI technologies like ChatGPT. Great article, Stacy!
Thank you, Jack. The advancements in AI are indeed contributing to the transformation of pharmacokinetics and broader aspects of healthcare.
Stacy, I appreciate your insights and the engaging discussion that followed. Thank you for this enlightening article.
You're very welcome, Daniel. Thank you for your contribution to the discussion and for your appreciative feedback.
This discussion has been intellectually stimulating. Thank you, Stacy, for sharing your knowledge and expertise.
You're welcome, Emily. It's been invigorating to see the enthusiasm and curiosity of everyone involved in this conversation.
The potential of AI in pharmacokinetics is truly inspiring. Thank you, Stacy, for hosting this enlightening discussion.
Thank you, Thomas. It was my pleasure to facilitate this discussion and provide a platform for exploration and learning.
Thank you, Stacy, for this informative article and for engaging with our questions and comments.
You're most welcome, Olivia. I'm grateful for the opportunity to share knowledge and learn from the perspectives of others.
It's been a pleasure to be a part of this discussion. Thanks, Stacy, for your expertise and for bringing us all together.
You're welcome, Liam. I'm thrilled to have facilitated an engaging and collaborative discussion on the potential application of AI in pharmacokinetics.
Stacy, thanks for your insightful article and for engaging with our questions. It's been an enlightening conversation.
You're very welcome, Brian. I'm grateful for the enriching conversation and the opportunity to address questions and provide insights.
Thank you, Stacy, for expanding our understanding of pharmacokinetics in the context of AI. It was an enlightening discourse.
You're welcome, Jennifer. I'm delighted that this discourse has deepened your understanding of the intersection between AI and pharmacokinetics.
Stacy, your article and responses have been enlightening. Thank you for the knowledge sharing and engaging discussion.
Thank you, Emma. I greatly appreciate your kind words and the enthusiasm you've brought to this discussion.
I've thoroughly enjoyed this discussion. Thank you, Stacy, for sharing your expertise and insights.
You're welcome, Daniel. I'm thrilled that you found this discussion enjoyable and informative.
Thank you, Stacy, for this enlightening article and for your invaluable responses to our comments.
You're most welcome, Oliver. It's been a pleasure to provide insights and facilitate this enlightening discussion.
Stacy, thank you for taking the time to engage with us and address our inquiries. This has been an informative conversation.
You're very welcome, Nathan. I appreciate your active participation and the stimulating questions raised.
Thank you, Stacy, for your expertise and for fostering this insightful discussion.
You're welcome, Grace. I'm grateful for the opportunity to share expertise and engage in thought-provoking conversations.
This discussion highlighted the immense potential of AI in pharmacokinetics. Thanks, Stacy, for sharing your insights.
You're very welcome, Jack. I'm glad that the discussion emphasized the transformative impact of AI in pharmacokinetics.
Thank you, Stacy, for your time and insightful responses. This has been a captivating discussion.
You're welcome, Emily. I'm thrilled that you found this discussion captivating and filled with valuable insights.
Stacy, thank you for your expertise and the engaging conversation. I've learned a lot.
You're most welcome, Thomas. I'm delighted to have been able to share expertise and contribute to your knowledge.
Thank you, Stacy, for hosting this enlightening discussion and providing us with valuable insights.
You're welcome, Sophia. It's been a pleasure to foster this discussion and share insights on an exciting topic.
This article is fascinating! It's amazing how technology is advancing in the field of pharmacokinetics.
I agree, Mark. The potential applications of ChatGPT in pharmacokinetics sound promising. It could revolutionize drug development and personalized medicine.
I'm curious about the accuracy of ChatGPT. Can it provide reliable predictions for pharmacokinetics?
Great question, Emily! ChatGPT has shown impressive performance, but it's crucial to validate its predictions through rigorous testing and comparison with existing models.
The potential ethical implications of using AI like ChatGPT in pharmacokinetics should also be considered. We need to ensure patient safety and privacy.
Absolutely, David! The responsible integration of AI should be a top priority to avoid any adverse effects or bias in treatment outcomes.
ChatGPT seems like a game-changer in the field! I wonder if other AI models are being explored for pharmacokinetics as well?
Indeed, Michael! Researchers are actively exploring various AI models, such as deep learning algorithms and reinforcement learning, to enhance pharmacokinetics predictions.
I'm amazed by the potential of ChatGPT. It could accelerate the drug discovery process and help identify new treatments faster.
Exactly, Sophia! The ability to simulate drug metabolism and predict drug concentrations in the body could save lots of time and resources during research.
While ChatGPT has immense potential, we must also address the limitations. It's essential to avoid overreliance on AI without considering its weaknesses.
Absolutely, Robert. AI models like ChatGPT may struggle with uncertainty and lack of data, so they should be used in conjunction with other approaches to mitigate risks.
I can see ChatGPT being immensely helpful in drug dose optimization. Tailoring drug dosages to individual patients could minimize side effects and improve outcomes.
You're right, Melissa! ChatGPT has the potential to aid in personalized medicine by optimizing drug dosing based on individual patient characteristics.
I'm curious about the computational resources required for ChatGPT to perform pharmacokinetic simulations. Is it resource-intensive?
Good point, Richard. ChatGPT relies on significant computational resources, including powerful GPUs and substantial memory, to perform complex pharmacokinetic simulations.
Stacy, can ChatGPT account for interindividual variability in pharmacokinetics? Patients can have significantly different responses to drugs.
Yes, Emily. ChatGPT can incorporate individual-specific factors to some extent, but the accuracy may rely on the availability of relevant patient data for training.
The potential cost savings and increased efficiency through the application of ChatGPT in pharmacokinetics are exciting. It could greatly benefit healthcare.
Absolutely, Mark! If we can optimize drug development and dosing, it could reduce healthcare costs while improving patient outcomes.
I hope the implementation of ChatGPT in pharmacokinetics receives proper regulation and oversight to ensure its safe and responsible usage.
I completely agree, Daniel. Regulatory frameworks and guidelines must be established to govern the implementation of AI models like ChatGPT in pharmacokinetics.
ChatGPT has the potential to enhance clinical decision-making and treatment strategies. Exciting times ahead for pharmacokinetics!
Definitely, Linda! Integrating AI models intelligently can improve medical practices, leading to better patient care and outcomes.
I wonder if ChatGPT could help predict drug-drug interactions accurately. It could prevent adverse effects when multiple medications are used simultaneously.
Good point, Anna. Drug-drug interactions are critical to consider, and ChatGPT shows promise in predicting potential interactions based on pharmacokinetic profiles.
I believe the collaboration between pharmacokinetics experts and AI researchers is crucial for harnessing the full potential of ChatGPT in this field.
Absolutely, David! A multidisciplinary approach can ensure the effective development and application of ChatGPT for advanced pharmacokinetic predictions.
ChatGPT's ability to perform rapid simulations could also help identify drug candidates that are worth pursuing further in the research pipeline.
That's a great point, Sophie! By quickly narrowing down drug candidates, ChatGPT could streamline the drug discovery process and save valuable resources.
Has ChatGPT been tested on real patient data in pharmacokinetic predictions? Real-world validation would be crucial.
Indeed, Richard. The performance of ChatGPT has been evaluated on real patient data in pharmacokinetics, demonstrating promise, but further validation is necessary.
How can we ensure that ChatGPT remains up to date with new drug discoveries and changing pharmacokinetic knowledge?
Valid point, Emily. Continuous updates and integration of new drug data and research findings are essential to keep ChatGPT relevant and accurate in pharmacokinetics.
Are there any known limitations or challenges in implementing ChatGPT for pharmacokinetics that we should be aware of?
Certainly, Michael. Some challenges include handling uncertainty, limited access to high-quality training data, and potential biases in the models due to existing datasets.
The potential of AI in healthcare is immense, but we cannot overlook the importance of human expertise and interpretation of the results provided by ChatGPT.
Absolutely, Daniel. AI models like ChatGPT should be used as tools to complement human expertise and foster collaborative decision-making in pharmacokinetics.
I can see ChatGPT being immensely helpful in drug dose optimization. Tailoring drug dosages to individual patients could minimize side effects and improve outcomes.
You're right, Melissa! ChatGPT has the potential to aid in personalized medicine by optimizing drug dosing based on individual patient characteristics.
I'm amazed by the potential of ChatGPT. It could accelerate the drug discovery process and help identify new treatments faster.
Exactly, Sophia! The ability to simulate drug metabolism and predict drug concentrations in the body could save lots of time and resources during research.
While ChatGPT has immense potential, we must also address the limitations. It's essential to avoid overreliance on AI without considering its weaknesses.
Absolutely, Robert. AI models like ChatGPT may struggle with uncertainty and lack of data, so they should be used in conjunction with other approaches to mitigate risks.
The potential cost savings and increased efficiency through the application of ChatGPT in pharmacokinetics are exciting. It could greatly benefit healthcare.
Absolutely, Mark! If we can optimize drug development and dosing, it could reduce healthcare costs while improving patient outcomes.
I hope the implementation of ChatGPT in pharmacokinetics receives proper regulation and oversight to ensure its safe and responsible usage.
I completely agree, Daniel. Regulatory frameworks and guidelines must be established to govern the implementation of AI models like ChatGPT in pharmacokinetics.
ChatGPT has the potential to enhance clinical decision-making and treatment strategies. Exciting times ahead for pharmacokinetics!
Definitely, Linda! Integrating AI models intelligently can improve medical practices, leading to better patient care and outcomes.