Revolutionizing Predictive Analysis: Harnessing ChatGPT's Highly Organized and Strong Analytical Abilities
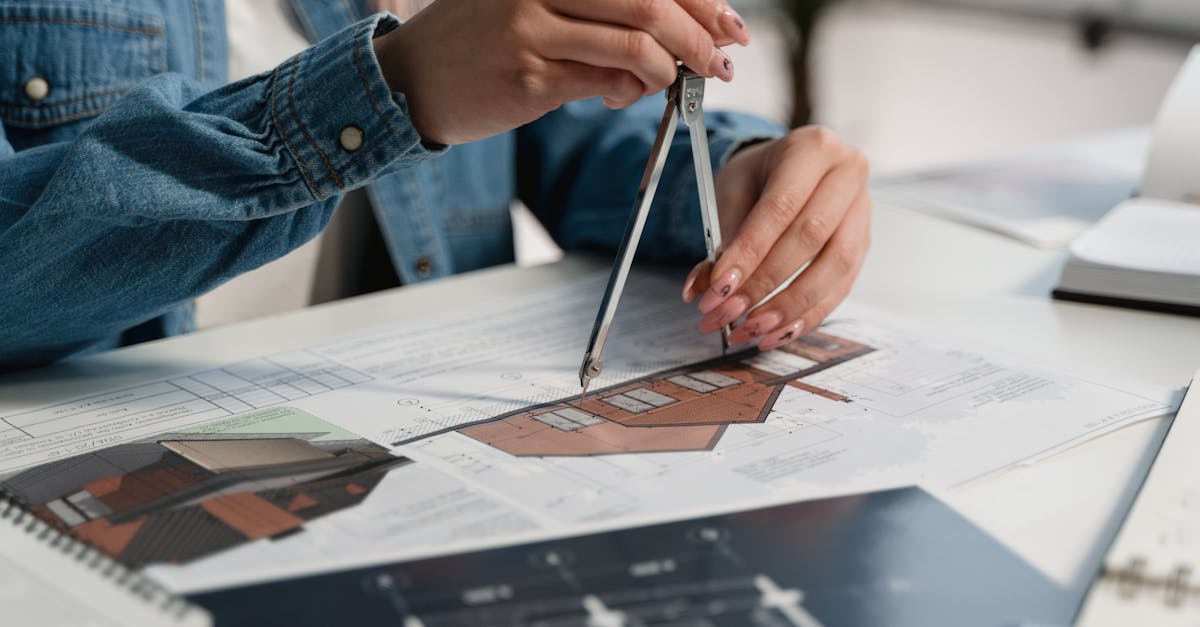
With the advent of advanced artificial intelligence technologies, predictive analysis has reached new heights. One such groundbreaking technology is ChatGPT-4, a chatbot powered by highly organized and strong analytical abilities, which enables it to make accurate predictions about future events. This article explores how ChatGPT-4 leverages historical data and its analytical skills to provide valuable insights for various domains.
Understanding Predictive Analysis
Predictive analysis refers to the process of using historical data, statistical algorithms, and machine learning techniques to forecast future outcomes based on patterns and trends. It has found applications in a wide range of areas, such as finance, marketing, healthcare, and more. The primary goal of predictive analysis is to identify potential trends, risks, and opportunities, and make informed decisions based on the predictions.
Introducing ChatGPT-4
ChatGPT-4, developed by OpenAI, is an advanced conversational AI model that possesses highly organized and strong analytical abilities. Building on the success of its predecessors, ChatGPT-4 takes predictive analysis to the next level by leveraging vast amounts of historical data and utilizing complex algorithms for accurate predictions.
Learning from Historical Data
One key aspect of ChatGPT-4 is its ability to learn from large datasets containing historical information. By analyzing this data, including past events, trends, and outcomes, the model can identify patterns and correlations that might not be immediately apparent to human observers. This comprehensive understanding of historical data serves as the basis for its predictive capabilities.
Applying Analytical Skills
ChatGPT-4's strong analytical abilities allow it to process and interpret complex data sets with ease. It can detect subtle patterns, correlations, and dependencies, enabling it to make accurate predictions. The model applies various statistical algorithms and machine learning techniques to analyze the data and extract meaningful insights, facilitating predictive analysis in a variety of domains.
Usage of ChatGPT-4 for Predictive Analysis
ChatGPT-4's powerful predictive analysis capabilities can be utilized in numerous applications across various domains. Here are a few examples:
- Financial Forecasting: Using historical financial data, ChatGPT-4 can analyze market trends and predict future stock prices, exchange rates, and investment opportunities, helping financial institutions and individual investors make informed decisions.
- Marketing Strategy: By analyzing consumer behavior, purchasing patterns, and marketing campaign data, ChatGPT-4 can provide insights into customer preferences, predict market trends, and recommend targeted marketing strategies.
- Healthcare Planning: Leveraging historical patient data, medical records, and epidemiological information, ChatGPT-4 can predict disease outbreaks, identify at-risk populations, and facilitate resource planning for healthcare providers.
- Risk Management: ChatGPT-4's predictive analysis capabilities can be applied to assess risks in different domains, such as insurance, cybersecurity, and climate change. By analyzing historical data on incidents and vulnerabilities, it can predict potential future risks and aid in developing risk mitigation strategies.
Conclusion
With its highly organized and strong analytical abilities, ChatGPT-4 opens up new possibilities in the field of predictive analysis. By learning from historical data and applying complex algorithms, it can make accurate predictions about future events in diverse domains. As this technology continues to evolve, we can expect ChatGPT-4 to play a significant role in helping individuals and organizations make informed decisions based on data-driven insights.
Comments:
Thank you all for your comments on my article! I'm glad to see such interest in the potential of ChatGPT for predictive analysis.
ChatGPT's capabilities are indeed impressive. I can definitely see it revolutionizing predictive analysis in various industries.
While ChatGPT shows promise, it's important to consider potential biases in the data used for training. How can we ensure unbiased and ethical analysis?
John, you raise a valid concern. It is crucial to address biases in the data. A comprehensive data selection and preprocessing approach, along with regular audits, can help mitigate bias and maintain ethical analysis.
I'm curious about the scalability of using ChatGPT for predictive analysis. Can it handle large datasets efficiently?
Sarah, excellent question! ChatGPT can handle large datasets, but it's important to consider computational resources and model optimization to ensure efficient processing.
Can ChatGPT be applied to real-time prediction tasks, or is it better suited for offline analysis?
Michael, great point. While ChatGPT works well for offline analysis, adapting it for real-time prediction tasks may require additional considerations, such as optimizing response time and model deployment strategies.
Michael, while real-time prediction is possible, it requires infrastructure setup to handle continuous input and output. Latency and resource allocation become important factors to consider.
I'm concerned about potential privacy issues when using ChatGPT for predictive analysis. How can we ensure the protection of sensitive data?
Emma, privacy is indeed a crucial aspect. Implementing appropriate security measures, such as data anonymization, secure storage, and access controls, can help safeguard sensitive data when using ChatGPT for predictive analysis.
Emma, apart from technological safeguards, legal frameworks like GDPR play a crucial role in ensuring privacy when using ChatGPT for predictive analysis.
Emma, implementing a comprehensive user consent framework ensures individuals have control over how their data is used in ChatGPT-based predictive analysis.
What are the major challenges in integrating ChatGPT into existing predictive analysis pipelines?
Peter, integrating ChatGPT into existing pipelines may require adapting data formats, addressing model integration complexities, and ensuring compatibility with downstream applications. It's important to carefully plan and test the integration process.
How does ChatGPT compare to traditional predictive analysis methods in terms of accuracy and interpretability?
Thomas, ChatGPT's accuracy can be impressive, but it's important to note that interpretability can be a challenge. Traditional methods often provide more explainability, but research efforts are ongoing to enhance the interpretability of AI models like ChatGPT.
ChatGPT's strong analytical abilities are exciting, but how can we ensure the model's predictions are reliable and trustworthy?
Amy, ensuring reliability and trustworthiness requires rigorous evaluation, validation, and benchmarking of ChatGPT's performance. Regular monitoring and continuous improvement are essential to maintain predictive accuracy.
What are the potential applications of ChatGPT in the field of predictive analysis? Are there any specific industries that can benefit the most?
Oliver, ChatGPT has broad applications in industries like finance, healthcare, marketing, and customer support. Any industry that requires predictive analysis can benefit from the powerful capabilities of ChatGPT.
Oliver, ChatGPT can have significant applications in the insurance industry. It can aid in risk assessment, fraud detection, and customer sentiment analysis, among other use cases.
I'm curious about the training process of ChatGPT. How much data and computational resources are typically required for effective predictive analysis?
David, the training process involves using large-scale datasets and substantial computational resources. The specific requirements depend on the complexity of the analysis task and desired accuracy, but it generally requires significant data and computational power.
David, the effectiveness of predictive analysis depends not only on the model but also on the quality and relevance of the data used. Collecting and curating high-quality data is essential.
In what ways can ChatGPT be fine-tuned for domain-specific predictive analysis?
Kelly, fine-tuning can be valuable for domain-specific analysis. By training ChatGPT on data relevant to a particular domain, it can learn specialized knowledge and improve predictive performance in that specific area.
Kelly, fine-tuning includes steps like adjusting hyperparameters, training on a domain-specific dataset, and performing iterative evaluation to optimize ChatGPT's performance.
Are there any risks or limitations associated with relying heavily on ChatGPT for predictive analysis?
Laura, there are risks to consider. Heavy reliance on ChatGPT without appropriate validation and human oversight may result in erroneous predictions. It's crucial to strike a balance between leveraging AI capabilities and maintaining human involvement for critical decision-making.
What level of technical expertise is required to implement ChatGPT for predictive analysis?
Nathan, implementing ChatGPT for predictive analysis requires a solid understanding of machine learning, natural language processing, and relevant programming skills. Additionally, experience with data preprocessing and model deployment is important.
Nathan, although technical expertise is important, collaboration between domain experts, data scientists, and software engineers can lead to successful implementation of ChatGPT in predictive analysis.
Could you provide some examples where ChatGPT has already made a significant impact in predictive analysis?
Sophia, ChatGPT is relatively new, but it has shown promise in various areas such as sentiment analysis, demand forecasting, and anomaly detection. Ongoing research and applications are continuously expanding its impact in predictive analysis.
What are some important factors to consider when selecting a suitable model for predictive analysis, especially in relation to ChatGPT?
Mark, selecting a suitable model involves considering factors like task complexity, available data, interpretability requirements, computational resources, and integration feasibility. ChatGPT can be a strong option for many use cases, but these considerations are crucial.
I'm curious about the interaction between qualitative and quantitative analysis with ChatGPT. How well does it handle both aspects?
Grace, ChatGPT's versatility allows for handling both qualitative and quantitative analysis. Its ability to understand and generate natural language makes it particularly suited for qualitative aspects, while its analytical capabilities enable quantitative analysis as well.
Grace, the combination of qualitative and quantitative analysis with ChatGPT enables richer insights, as it can grasp underlying context and patterns while processing large amounts of data.
Are there any ongoing developments to enhance ChatGPT's capabilities for predictive analysis?
Jason, yes, there are continuous efforts to enhance ChatGPT's capabilities. Research focuses on areas like interpretability, reducing biases, handling unstructured data, and improving performance on specific tasks. The AI community is actively working on these developments.
How does the cost of utilizing ChatGPT for predictive analysis compare to traditional methods?
Liam, the cost of utilizing ChatGPT for predictive analysis involves considerations like computational resources, model training, and ongoing maintenance. While initial costs may be higher, ChatGPT can potentially offer cost-efficiency in the long term, especially for complex analysis tasks.
What precautions should organizations take in terms of data governance and legal compliance when using ChatGPT for predictive analysis?
Emily, organizations should establish comprehensive data governance policies, ensure compliance with relevant laws, and prioritize user privacy. It's important to engage legal and compliance experts in the process of implementing ChatGPT to meet regulatory requirements.
Emily, organizations should also establish transparent data processing practices and provide clear guidelines on the use of ChatGPT, especially when it comes to sensitive data.
Can ChatGPT be combined with other AI models or techniques to enhance predictive analysis?
Robert, absolutely! Combining ChatGPT with other AI models or techniques can lead to more powerful predictive analysis solutions. Ensemble methods and hybrid approaches often bring complementary strengths to enhance overall performance.
I agree with John's concern on data biases. In addition to audits, can post-deployment monitoring help identify and address any biases that may arise?