Revolutionizing Predictive Maintenance in Manufacturing: Harnessing the Power of ChatGPT
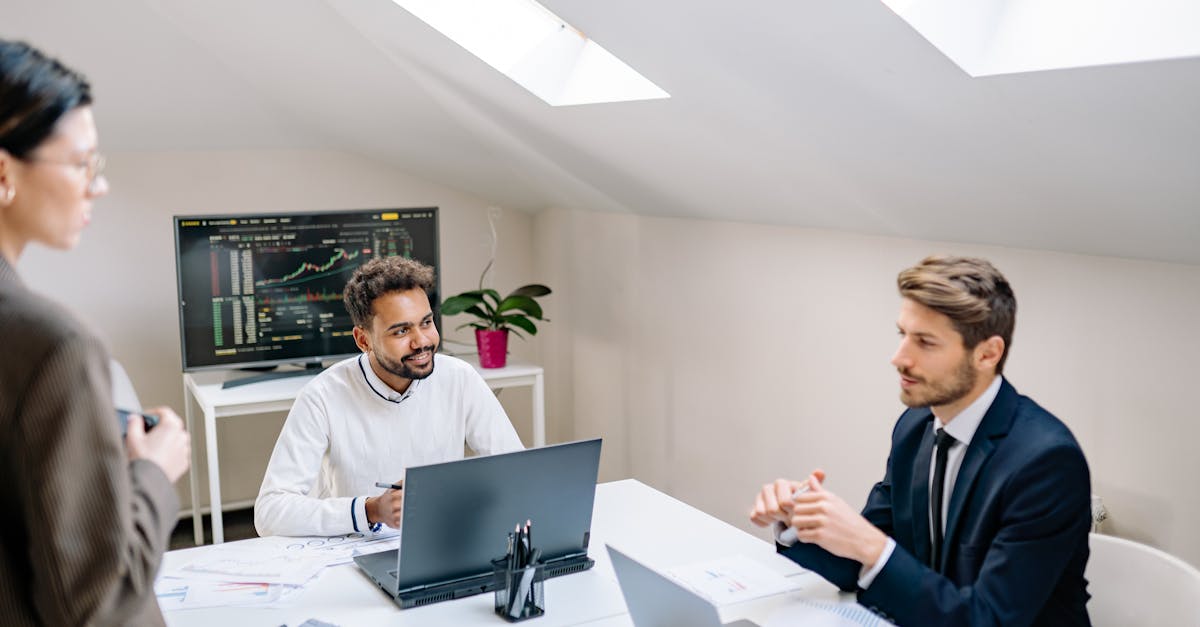
Introduction
In the manufacturing industry, equipment failure can lead to significant financial losses, production delays, and safety hazards. Predictive maintenance provides a proactive approach to equipment maintenance by utilizing technology to analyze data and predict equipment failures before they occur. This article explores how predictive maintenance is used in the manufacturing sector to enhance operational efficiency and reduce downtime.
Technology: Predictive Maintenance
Predictive maintenance relies on advanced technologies such as Internet of Things (IoT), sensor networks, and artificial intelligence (AI) algorithms to collect and analyze data from various equipment. These technologies enable real-time monitoring of critical parameters such as temperature, vibration, pressure, and power consumption. By continuously monitoring equipment, predictive maintenance systems can detect anomalies, identify patterns, and predict potential failures.
Area: Manufacturing
Predictive maintenance is particularly relevant in the manufacturing industry due to the criticality of equipment uptime and the potential impact of failures. Manufacturing facilities often rely on complex machinery, production lines, and automated systems, making it essential to ensure their proper functioning. By implementing predictive maintenance, manufacturers can minimize unplanned downtime, improve production capacity, and reduce maintenance costs.
Usage: Equipment Failure Prediction
One of the primary uses of predictive maintenance in manufacturing is the prediction of equipment failures. By continuously monitoring equipment performance and analyzing historical data, predictive maintenance systems can identify indicators of potential failures. These indicators can include abnormal patterns, deviations from normal operating conditions, or specific events that precede failures. Based on these predictions, maintenance teams can plan and schedule preventive maintenance activities to address issues before they escalate.
Usage: Preventive Maintenance Scheduling
Another key application of predictive maintenance is the optimization of preventive maintenance schedules. Traditionally, manufacturers follow fixed maintenance intervals, which can result in unnecessary maintenance and downtime. Predictive maintenance systems, on the other hand, can provide insights into the actual condition of equipment, allowing maintenance activities to be scheduled based on real-time data rather than pre-defined time intervals. This approach reduces maintenance costs, extends equipment lifespan, and maximizes operational efficiency.
Conclusion
Predictive maintenance is revolutionizing the manufacturing industry by enabling proactive equipment maintenance and improving operational efficiency. By leveraging advanced technologies and analyzing data in real-time, manufacturers can predict equipment failures, plan preventive maintenance, and reduce unplanned downtime. The adoption of predictive maintenance can lead to significant cost savings, increased productivity, and enhanced competitiveness in the fast-paced manufacturing sector.
Comments:
Thank you all for your interest in my article! I'm glad to see a discussion starting here. Please feel free to share your thoughts and ask any questions you may have about predictive maintenance in manufacturing and the potential of ChatGPT.
Great article, Lois! I think the use of ChatGPT in predictive maintenance could revolutionize the manufacturing industry. The ability to analyze and interpret data in real-time can significantly improve efficiency and reduce downtime.
I agree, Melissa. It's exciting to see how advanced AI technologies like ChatGPT can bring more accuracy and speed to maintenance operations. This will definitely have a huge impact on productivity.
While I agree that predictive maintenance has immense potential, I'm concerned about the reliability of ChatGPT. Sometimes AI models can make mistakes or miss important issues. How can we ensure that ChatGPT is trustworthy enough to rely on for critical decisions?
Hi Samantha, that's a valid concern. While AI models like ChatGPT are continually improving, it's important to have safety measures in place. The best approach would be to use ChatGPT as a tool to assist human experts rather than relying solely on it. Human oversight and verification will ensure more reliable outcomes.
Absolutely, human oversight is crucial. It's also worth considering that we should continuously train and fine-tune ChatGPT using real-world data to improve its accuracy and reliability as it operates in different manufacturing environments.
I'm curious about the implementation process. Can anyone share insights on how easy or challenging it is to integrate ChatGPT into existing predictive maintenance systems?
Hi Emily, integrating ChatGPT into existing systems can have its challenges, but it is feasible with proper planning and coordination. It requires training the model on relevant maintenance data and developing an API or interface for seamless integration. It's best to involve experts from both AI and maintenance domains throughout the process.
I've been involved in a similar integration process, Emily. It can be complex, particularly when dealing with legacy systems. However, the potential benefits outweigh the initial challenges. The key is having a well-defined strategy and involving all stakeholders from the beginning.
Indeed, the collaboration between AI and maintenance experts is vital. It ensures that the system understands the unique requirements and constraints of predictive maintenance in different manufacturing contexts.
I'm interested in the potential cost savings with predictive maintenance. Has anyone conducted a cost-benefit analysis to determine the return on investment?
Katherine, we conducted a cost-benefit analysis before implementing predictive maintenance using ChatGPT. While the initial investment can be significant, the long-term savings in terms of reduced maintenance costs, increased equipment lifespan, and minimized downtime outweigh the expenses.
You're right, David. We saw a considerable decrease in emergency repairs and unplanned downtime after implementing predictive maintenance. That alone made the investment worth it.
Oliver, I completely agree. Real-world data helps us train the model to be more reliable and adaptable to different manufacturing environments.
Katherine, I can offer my experience with cost-benefit analysis. It helped us justify the implementation of predictive maintenance with ChatGPT, and the return on investment has been remarkable.
Thanks for sharing, Kara! It's encouraging to hear about the positive return on investment.
That's true, Katherine. While AI has great potential, it's important to be aware of its limitations and consider them when implementing predictive maintenance solutions.
Thanks, David and Kara! Sounds like conducting a cost-benefit analysis is a crucial step in ensuring successful implementation.
What about the workforce impact? Will predictive maintenance with ChatGPT lead to job losses in the manufacturing sector?
Daniel, that's an important concern. While AI may automate certain tasks, it can also augment the workforce by providing data-driven insights and enabling better decision-making. The shift would be towards more skilled roles, such as AI system operators and maintenance strategists.
I agree with Lois. Predictive maintenance frees up time for technicians to focus on more complex and valuable tasks, resulting in upskilling opportunities and increasing job satisfaction.
How scalable is ChatGPT for large-scale manufacturing operations? Can it handle the enormous amounts of data generated by sensors and machines?
Sophia, scalability is definitely a consideration. ChatGPT can handle a substantial volume of data, but the infrastructure needs to support the processing requirements efficiently. Distributed systems and optimized architectures can help ensure smooth scalability.
Thanks, Nathan. Involving stakeholders from different domains sounds like a crucial step in ensuring successful integration.
Cloud-based solutions and distributed computing platforms are indeed essential for scalability. Thanks for the clarification, Lois and Nathan.
To add to Nathan's point, leveraging cloud-based solutions or distributed computing platforms can enhance scalability. That way, ChatGPT can analyze vast amounts of data in parallel, enabling it to cater to the needs of large-scale manufacturing.
What are the potential limitations of ChatGPT in the context of predictive maintenance?
One limitation of ChatGPT is that it relies on the data it was trained on. If it encounters uncommon scenarios or edge cases, it might struggle to provide accurate predictions. Continuous monitoring and fine-tuning are important to address such limitations.
You're right, Melissa. Understanding the unique requirements and constraints of different manufacturing contexts is vital for effective implementation and utilization of ChatGPT in predictive maintenance.
Absolutely, Melissa. Continuous monitoring and adaptation are key to ensuring accurate predictions in diverse scenarios.
Another limitation is the interpretability of ChatGPT's decisions. Sometimes, it's hard to understand or explain why it reached a particular prediction. That's where human expertise is invaluable in verifying and validating the AI model's outputs.
Absolutely, David. The transparency and interpretability of AI systems will play a significant role in building trust and addressing any concerns.
Indeed, Oliver. The interpretability of AI decisions is critical, especially when making important maintenance-related decisions based on ChatGPT's output.
Thank you all for your valuable insights and questions! The potential of predictive maintenance with ChatGPT is indeed significant, but it's important to address concerns and limitations while realizing its benefits. Feel free to continue the discussion and I'll be here to provide further input.
Lois, your point about upskilling opportunities is crucial. It's important to view AI as a tool that complements and empowers the workforce rather than replacing them.
Thank you, Lois, for sharing your expertise with us through this article and engaging in this conversation. It has been enlightening and thought-provoking.
You're welcome, Sophia. I'm glad to have sparked interesting discussions. This kind of exchange allows us to explore different perspectives and drive innovation in the field of predictive maintenance.
Indeed, thank you, Lois. Your article has shed light on the potential of ChatGPT in predictive maintenance and the importance of collaborative approaches.
Thanks for clarifying, Lois. Proper planning and involving experts from both AI and maintenance fields will definitely help overcome integration challenges.
You're welcome, Sophia! It's been a pleasure discussing these topics with all of you. The collective insights from this conversation will surely contribute to the advancement of predictive maintenance practices.
So, the integration process requires a combination of technical expertise and collaboration with domain experts. That makes sense considering the complexity involved.
I think AI adoption would lead to new job roles and a shift in the skillset required. It's an opportunity for workers to adapt and acquire new skills instead of facing job losses.
I completely agree, Liam. Rather than job losses, AI adoption can lead to the evolution of jobs and create new opportunities.
Continuous monitoring and fine-tuning are indeed crucial to address the limitations. It ensures that the AI model remains accurate in real-world scenarios.
Upskilling can also lead to more job satisfaction as employees are given the opportunity to take on more interesting and challenging tasks.
Human oversight and verification are indeed necessary to ensure the reliability of predictive maintenance systems. ChatGPT should be used as a valuable tool in combination with human expertise.
Collaborating with domain experts throughout the integration process is essential to ensure that the AI system understands the nuances of predictive maintenance.
Cloud-based solutions and distributed computing platforms indeed offer the scalability needed to process vast amounts of data generated by manufacturing operations.
Predictive maintenance not only reduces costs but also improves overall equipment reliability, which positively impacts production efficiency.