Revolutionizing Public Opinion Polling: Harnessing ChatGPT for Social Network Analysis Technology
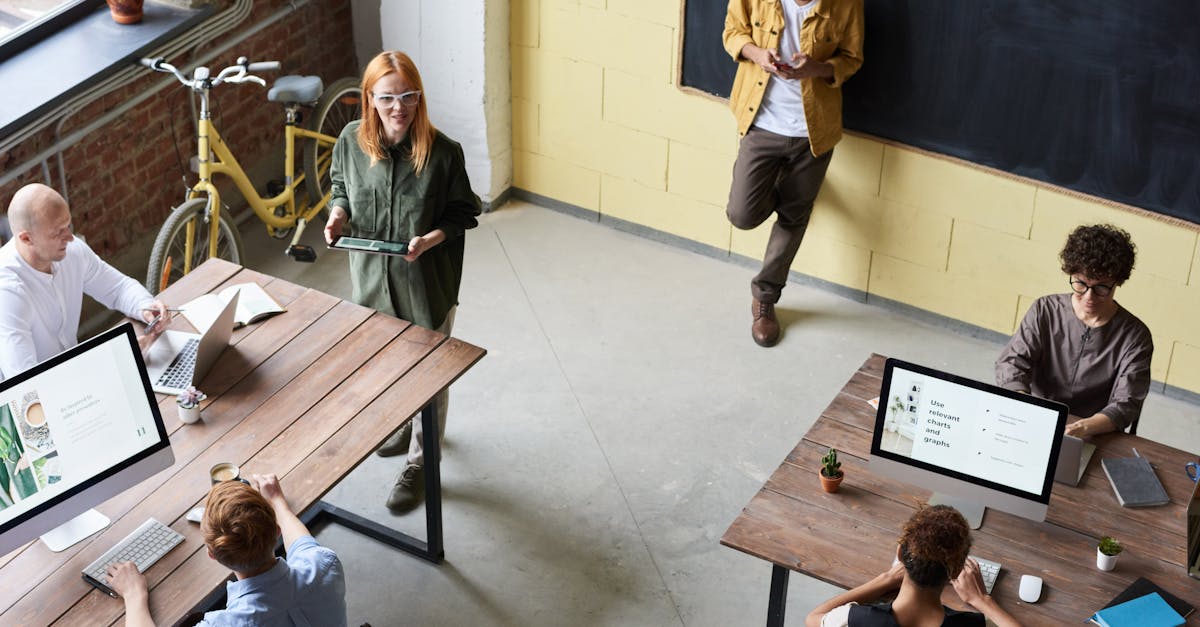
In the age of social media, understanding public opinion has become more important than ever. Social network analysis (SNA) provides a powerful tool to analyze sentiments and gather data for public opinion polling. This technology has revolutionized the way we conduct polls and enables us to accurately gauge the pulse of the public.
Social network analysis involves the study of social relationships and interactions among individuals or organizations. By examining these interactions, SNA allows us to understand the structure and dynamics of social networks. It provides valuable insights into how information spreads, influence flows, and opinions are formed within these networks.
One of the key applications of SNA is in public opinion polling. Traditionally, polling relied on surveys and questionnaires to gather data. However, this approach often faced challenges due to small sample sizes, limited representation, and response bias. With social network analysis, we can overcome these limitations and collect data on a much larger scale.
By analyzing sentiments on social networks, researchers can identify trends, measure attitudes, and evaluate public sentiment towards various topics. This information can be used to understand public opinion and make informed decisions. SNA allows us to go beyond traditional polling techniques and gather data from a diverse range of sources.
There are several ways in which SNA can be utilized for public opinion polling:
1. Sentiment Analysis
Social network analysis enables sentiment analysis, which involves the categorization and measurement of opinions expressed in social media posts. By analyzing the sentiment associated with particular topics or keywords, researchers can understand the public's perception and sentiment towards those topics.
2. Trend Identification
SNA helps identify emerging trends and topics of interest. By tracking the spread of information and opinions within social networks, analysts can identify key influencers and monitor the buzz around specific issues. This information can be invaluable in understanding the public's preferences and concerns.
3. Targeted Sampling
SNA allows for targeted sampling by identifying specific communities or groups within the social network. This ensures that the polling data represents a diverse range of opinions and demographics. Researchers can reach out to specific clusters and communities to obtain a more accurate representation of public opinion.
4. Real-time Updates
Unlike traditional polling methods, SNA provides real-time insights and updates. By continuously monitoring social media platforms, researchers can stay up-to-date with the changing public sentiment. This allows for timely analysis and informed decision-making.
Social network analysis has transformed the field of public opinion polling. Its ability to analyze sentiments on a large scale, capture real-time data, and provide insights into social networks has made it an invaluable tool for understanding public opinion. By harnessing the power of SNA, we can obtain a more accurate and comprehensive understanding of the public's sentiment and preferences.
It is important to note that while SNA provides valuable insights, it also has its limitations. The data collected from social media platforms may not be representative of the entire population, as not everyone is active on these platforms. Additionally, SNA relies on publicly available data and may not capture private sentiments or opinions.
Nevertheless, social network analysis has revolutionized public opinion polling and continues to shape the way we gather and analyze data. With its ability to capture real-time sentiments, identify trends, and target specific communities, SNA offers a more comprehensive and accurate approach to understanding public opinion.
In conclusion, social network analysis has emerged as a powerful technology for public opinion polling. By harnessing the capabilities of SNA, researchers can analyze sentiments on social networks, identify trends, target specific groups, and obtain real-time updates. This enables a more nuanced understanding of public opinion and informs decision-making processes. While SNA has its limitations, its numerous advantages make it an invaluable tool for gathering and analyzing data for public opinion polling.
Comments:
Thank you all for reading my blog post! I'm excited to see what you think about the potential of using chatbots for social network analysis. Your comments are greatly appreciated.
Great article, Jeff! I believe using ChatGPT for social network analysis could be a game-changer. The ability to analyze large amounts of conversation data in real-time can provide valuable insights into public opinion.
I agree, Sarah. This technology holds tremendous potential for understanding public sentiment. It could revolutionize the way we conduct opinion polls and gather insights from social media platforms.
While the idea is intriguing, I wonder how ChatGPT will handle biases and inaccuracies in data analysis. How can we ensure reliable results?
Emily, that's an excellent point. AI models like ChatGPT are trained on large datasets that can contain biases. It's crucial to carefully curate and validate training data to minimize bias and ensure accuracy in the results.
I'm concerned about data privacy. How can we ensure user data is protected when using ChatGPT for social network analysis?
Michelle, data privacy is indeed a valid concern. Jeff, could you please shed some light on how data privacy is addressed in the implementation of this technology?
Tom, data privacy is of utmost importance. When using ChatGPT, it's crucial to handle user data ethically and adhere to data protection regulations. Anonymization and secure storage practices should be implemented to safeguard user privacy.
I'm curious about the scalability of using ChatGPT for social network analysis. Can it handle analyzing vast amounts of data from various social media platforms?
Daniel, ChatGPT does have the potential for scalability. By leveraging powerful computing resources, it can process and analyze vast amounts of data efficiently. However, further research and optimizations may still be needed for optimal scalability.
This technology sounds promising, but what about the accuracy of sentiment analysis? It can be challenging to accurately determine emotions and context from text alone.
You make a valid point, Christina. Sentiment analysis is a challenging task, especially with the nuances of language. It's essential to continually improve models and fine-tune them to ensure accurate sentiment analysis, allowing for more reliable public opinion insights.
I'm curious to know how ChatGPT can handle different languages and cultural contexts. Public opinion varies across regions, and language can impact sentiment analysis.
Robert, language and cultural context indeed play a significant role in sentiment analysis. Ideally, ChatGPT should be trained on diverse datasets that cover different languages and cultures to better understand sentiments across regions. However, this remains an ongoing research challenge.
I can see how ChatGPT can enhance social network analysis, but what about the biases of the trained model itself? Can it reflect the diversity of opinions accurately?
Laura, addressing biases is crucial for accurate analysis. Training models with diverse datasets and ensuring inclusivity in data collection can help reduce biases. It's an ongoing effort, and the AI community is actively working towards developing fair and unbiased models.
Jeff, do you foresee any ethical challenges or potential misuse of this technology, particularly regarding manipulating public opinion?
Mark, ethical challenges and misuse are important considerations. It's crucial to use this technology responsibly, adhering to ethical guidelines and regulations. Transparency, accountability, and responsible usage policies can help mitigate potential risks.
Jeff, how does the accuracy of ChatGPT compare to existing methods used for social network analysis?
Lisa, ChatGPT has shown promising results in generating human-like responses, but its accuracy for social network analysis depends on the specific application and training data. Comparative studies with existing methods would be beneficial for assessing accuracy comprehensively.
This technology can definitely streamline public opinion polling processes, but I'm concerned about the potential impersonation or manipulation by malicious actors. How can we ensure authenticity?
Authenticity is crucial, Albert. Implementing methods to verify user identities and detect potential impersonation is essential. Combining AI-driven analysis with human moderation can help ensure authentic conversations and minimize manipulation.
I find it fascinating how AI can contribute to social network analysis. Jeff, any thoughts on the potential limitations or challenges we might face while deploying this technology?
Karen, deploying this technology comes with challenges. Technical limitations, biases, ethical concerns, and data privacy are some hurdles to overcome. We need multi-disciplinary efforts to address these issues and ensure responsible usage.
Jeff, could you share some real-world applications where ChatGPT for social network analysis has been successfully used?
Alex, there are various potential applications. ChatGPT has been used for sentiment analysis in social media data, identifying emerging trends, understanding public reactions to events, and even promoting safer online environments by detecting and addressing toxic conversations.
Those applications sound promising, Jeff. The ability to decipher sentiment and identify emerging trends in real-time can provide valuable insights for businesses, organizations, and policymakers alike.
I think the automation of social network analysis using chatbots can help streamline processes, but it might lack the human element of interpretation and contextual understanding.
Michael, you raise a valid concern. While automation can provide efficiency, human interpretation and contextual understanding are still crucial for more nuanced analysis. Combining AI-driven analysis with human expertise can lead to more accurate insights.
Since ChatGPT is trained on existing conversations, will it be able to handle new slang, abbreviations, or evolving language on social media platforms?
Emma, evolving language presents challenges. While ChatGPT can adapt well to known slang and abbreviations, it might struggle with entirely new language or specific dialects. Regular model updates and continuous training can help address these challenges.
Jeff, what are the key factors to consider when implementing ChatGPT for social network analysis in terms of computational resources and infrastructure?
Olivia, computational resources play a vital role. Depending on the scale of analysis, high-performance computing resources may be required to handle large amounts of data efficiently. Adequate infrastructure, including storage and processing power, is essential for optimal performance.
This technology seems promising, but what about the potential biases in training data and algorithmic decision-making? How can we ensure fairness?
Ensuring fairness is crucial, David. It starts with diverse and representative training data, careful handling of biases, and ongoing evaluations with respect to fairness metrics. Openness in model development, public scrutiny, and user feedback also contribute to identifying and mitigating biases.
This technology has immense potential, but what about the potential for misuse in amplifying misinformation or fake news?
Sophia, preventing the misuse of technology is crucial. Combining AI algorithms with effective moderation, fact-checking, and education can mitigate the potential spread of misinformation or fake news. Responsible usage and user awareness are key to countering such challenges.
I'm excited about the possibilities of chatbots for social network analysis, but what about the computational resources required? Will it be affordable for smaller organizations?
Edward, computational resources can be a limiting factor. Cloud computing services and infrastructure-as-a-service models can provide affordable scalability options, making it accessible to smaller organizations. Cost optimization strategies and resource planning would be essential for efficient utilization.
What are the possible risks associated with analyzing public opinion through a technology like ChatGPT?
Julia, risks include potential biases, data privacy concerns, ethical challenges, and ensuring authenticity. Overreliance on automated analysis without human oversight can also be problematic. Careful implementation, regulations, and responsible usage can help mitigate these risks.
Jeff, how can we address the potential challenges of scalability and response time while handling real-time analysis?
Adam, addressing scalability and real-time analysis involves optimizing computing resources, implementing efficient algorithms, and leveraging parallel processing capabilities. Continual advancements in hardware, software, and optimization techniques can help overcome these challenges.
I'm concerned about biased data collection from social media platforms, where specific demographics might be over- or under-represented. How can we ensure data collection is comprehensive and inclusive?
Grace, biased data collection can negatively impact analysis. Ensuring comprehensive and inclusive data collection involves mindful selection of data sources, actively including underrepresented groups, and addressing sampling biases. Careful consideration of data sources and diversity can help mitigate these concerns.
Jeff, how can organizations effectively communicate the use and benefits of chatbot-driven social network analysis without causing public concerns about data privacy?
William, transparency and clear communication are essential. Organizations should emphasize their commitment to data privacy, explain the anonymization and aggregation practices, and highlight the ways in which analysis benefits the public. Engaging in dialogue, addressing concerns, and seeking public input can help build trust.
Jeff, what are some potential future developments in this field that we can anticipate?
Elizabeth, the field of chatbot-driven social network analysis holds exciting possibilities. We can anticipate advancements in more accurate sentiment analysis models, improved language adaptability, enhanced privacy-preserving methods, and increased integration into decision-making processes. Continuous research and development will shape the future.
Thank you all for your insightful comments and questions. It was a pleasure discussing the potential of ChatGPT for social network analysis with you. Your feedback and engagement are invaluable in advancing this field. Stay curious and keep exploring!