Revolutionizing Quantitative Research: Harnessing the Power of ChatGPT in Text Mining
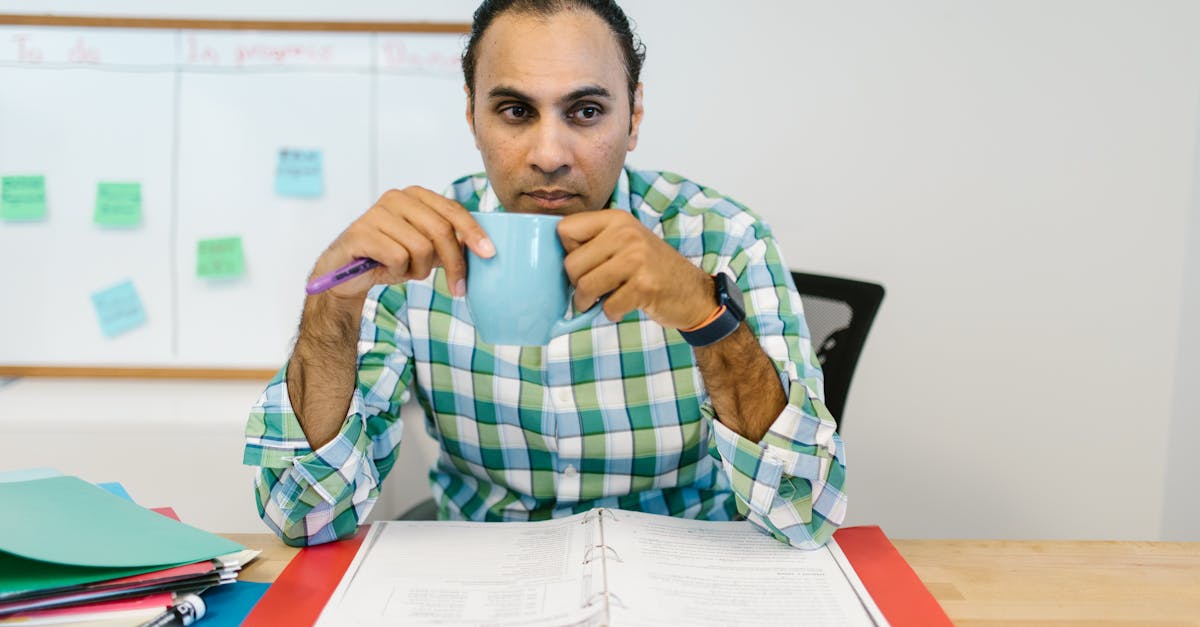
In today's data-driven world, quantitative research plays a crucial role in understanding and making informed decisions. One of the key methods used in quantitative research is text mining, which focuses on extracting meaningful insights and patterns from large volumes of textual data. With the advent of advanced AI models like ChatGPT-4, text mining has become even more powerful and efficient.
Technology
ChatGPT-4 is an advanced language generation model that leverages state-of-the-art techniques in natural language processing and deep learning. It has been trained on a massive amount of internet text, enabling it to understand and generate high-quality human-like text. This technology breakthrough has opened up new possibilities in various domains, including quantitative research.
Area: Text Mining
Text mining is a subfield of data mining that focuses on extracting useful information from unstructured text data. It involves various techniques such as document clustering, topic modeling, sentiment analysis, named entity recognition, and entity relation extraction. These techniques help researchers gain valuable insights from large collections of textual data, leading to better decision-making and understanding of complex phenomena.
Usage in Text Mining Tasks
ChatGPT-4 can be utilized effectively in multiple text mining tasks due to its language generation capabilities and contextual understanding of text. Some of the tasks where ChatGPT-4 can be employed are:
- Document Clustering: ChatGPT-4 can analyze text documents and group them into clusters based on their similarity. This helps in organizing large document collections and identifying similar themes or topics.
- Topic Modeling: By applying unsupervised learning techniques, ChatGPT-4 can identify the main topics present in a collection of documents. This is particularly useful in understanding the content of a large corpus and extracting relevant information.
- Sentiment Analysis: ChatGPT-4 can determine the sentiment expressed in a piece of text, whether it is positive, negative, or neutral. This analysis aids in understanding public opinion, customer feedback, and other forms of sentiment-based data.
- Named Entity Recognition: With its contextual understanding, ChatGPT-4 can identify and extract named entities such as persons, organizations, locations, and other important entities. This is valuable for tasks that require entity identification, like information extraction or knowledge graph creation.
- Entity Relation Extraction: ChatGPT-4 can also discover relationships between different named entities in a text, providing insights into how entities interact or co-occur. This can be valuable for building knowledge bases or understanding complex networks.
By utilizing ChatGPT-4 in these text mining tasks, researchers and data analysts can save time and resources while obtaining valuable insights from large volumes of textual data. The ability of ChatGPT-4 to generate human-like text responses also opens up possibilities for interactive exploration and experimentation in the field of quantitative research.
Conclusion
Text mining is a powerful tool in quantitative research, offering researchers a way to extract meaning from vast amounts of unstructured text data. With the advancement of AI models like ChatGPT-4, the capabilities of text mining have expanded significantly. ChatGPT-4's language generation abilities and contextual understanding make it well-suited for a range of text mining tasks such as document clustering, topic modeling, sentiment analysis, named entity recognition, and entity relation extraction. Incorporating ChatGPT-4 into the text mining workflow can enhance the efficiency and accuracy of quantitative research, paving the way for deeper insights and more informed decision-making.
Comments:
This article on harnessing the power of ChatGPT in text mining is fascinating. The potential for revolutionizing quantitative research is enormous!
Thank you, Maria! I appreciate your enthusiasm. Indeed, ChatGPT has shown great promise in various applications, including text mining.
I have been using traditional text mining techniques for years. It's exciting to see how AI-powered technologies like ChatGPT can take it to the next level.
Absolutely, Vincent! ChatGPT has the potential to uncover new insights and patterns that might have been missed using traditional approaches.
I'm curious about the potential limitations of ChatGPT in text mining. Are there any specific challenges researchers should be aware of?
Valid question, Emily. ChatGPT still has its limitations, such as generating plausible but incorrect responses. Researchers need to ensure rigorous validation and fine-tuning to mitigate such risks.
I'm concerned about potential biases in the data and how they might impact the outcomes of quantitative research using ChatGPT. Has this been addressed?
Great point, Daniel. Biases in training data can indeed affect the results. Research efforts are ongoing to reduce biases in AI models like ChatGPT, but it's crucial for researchers to be mindful of such challenges and take appropriate steps to address them.
I've used ChatGPT in my research, and I'm amazed by its capabilities. It has significantly accelerated my text mining tasks and provided valuable insights.
That's wonderful to hear, Olivia! ChatGPT has indeed shown promising results in many use cases. It's exciting to see researchers benefiting from its capabilities.
In a field like quantitative research, reproducibility is crucial. How can researchers ensure the reproducibility of findings when relying on ChatGPT?
Excellent question, Benjamin. Researchers should document the parameters used for fine-tuning, provide access to the dataset (if allowed), and share code whenever possible. Open collaboration and transparent reporting can aid in reproducibility efforts.
Can ChatGPT be used to mine and analyze social media data? It seems like it could be a powerful tool for understanding public sentiment.
Definitely, Sophia! ChatGPT can be employed for text mining and sentiment analysis on social media data. Its ability to understand natural language makes it a valuable tool for extracting insights from user-generated content.
Do you anticipate any ethical concerns when using ChatGPT for text mining, particularly in sensitive domains like healthcare?
Ethical considerations are crucial, Michael. In sensitive domains like healthcare, researchers need to carefully handle data privacy, security, and ensure appropriate anonymization. Ethical frameworks must guide the use of AI technologies like ChatGPT to mitigate potential risks.
I'm thrilled by the possibilities ChatGPT offers for text mining! It can aid researchers in analyzing vast amounts of text data and extract valuable insights efficiently.
Indeed, Isabella! ChatGPT's ability to handle massive amounts of text data and generate relevant responses can significantly enhance the efficiency of researchers working in the field of text mining.
Are there any pre-trained models available for ChatGPT specifically designed for text mining, or do researchers need to train their own models from scratch?
Good question, Connor. Currently, OpenAI provides pre-trained models like gpt-3.5-turbo that can be fine-tuned for specific tasks, including text mining. Fine-tuning can be a valuable approach, especially for researchers without the resources to train models from scratch.
As an AI enthusiast, I'm excited about ChatGPT's potential. But are there any drawbacks or challenges in practical implementation that we should be aware of?
Great question, Grace. Practical challenges include the cost of using ChatGPT and potential limitations of the API rate limits. Balancing the benefits with associated costs and limitations is crucial during implementation.
How can researchers validate the accuracy of ChatGPT's responses in text mining scenarios? Is manual evaluation necessary?
Validating ChatGPT's responses is essential, Alexandra. Researchers often employ manual evaluation, comparing the model's outputs to ground truth or using human annotators for evaluating the quality of the responses. It helps ensure accuracy and improve the reliability of text mining tasks.
ChatGPT seems like a great tool for automated summarization of research papers. Is there ongoing work to improve this aspect for text mining?
Absolutely, Nathan! Automated summarization is an active area of research, and AI models like ChatGPT can play a significant role. Continued efforts are being made to improve summarization capabilities, making them even more valuable for researchers analyzing large volumes of research papers.
Are there any specific programming languages or frameworks that are recommended for researchers to utilize with ChatGPT for text mining purposes?
Researchers can interact with ChatGPT using OpenAI's API, which supports various programming languages. Python is commonly used, and OpenAI provides libraries and examples to facilitate integration. However, researchers can choose the programming language that best aligns with their existing infrastructure and preferences.
I'm concerned about potential biases in the training data used for ChatGPT. How can researchers ensure fairness in their text mining applications?
Addressing biases in AI models is crucial, Lucas. Researchers should carefully curate training data and conduct bias analysis to identify and mitigate potential biases. OpenAI is actively working on reducing biases in ChatGPT models, but ensuring fairness requires continuous effort and attention from the researchers too.
I'm interested in the computational resources required to run ChatGPT for text mining tasks. Can it be efficiently implemented on standard hardware?
ChatGPT can be resource-intensive, Ava. While it can run on standard hardware, large-scale text mining tasks might benefit from more powerful hardware or cloud-based solutions. It depends on the specific requirements and the scale of the text mining being performed.
I'm curious about the potential applications of ChatGPT in fields beyond quantitative research. Can it be used for qualitative analysis as well?
Certainly, Aiden! ChatGPT's natural language understanding capabilities make it suitable for qualitative analysis too. It can aid researchers in extracting insights, understanding sentiments, and analyzing various forms of textual data, expanding its applications beyond quantitative research.
Do you anticipate any challenges in deploying ChatGPT for production-level text mining applications? What factors should be considered?
Production deployment of ChatGPT in text mining applications comes with challenges. Factors such as ensuring data privacy, training the model on domain-specific data, monitoring for biased or inaccurate outputs, and developing a robust feedback loop for continuous improvement are critical considerations. It requires proper planning, testing, and implementation strategies.
ChatGPT's capabilities seem incredible for analyzing unstructured text data. Are there any limitations when it comes to structured data or databases?
Good question, Gabriel. ChatGPT's strength lies in processing and understanding unstructured text data. While it can provide some insights into structured data, tasks specifically related to structured databases might require other specialized tools or techniques to leverage the full potential of structured data.
I'm concerned about potential security risks associated with using ChatGPT in text mining, particularly if sensitive data is involved. What measures should researchers take to ensure data security?
Data security is vital, Ethan. Researchers should follow standard security practices, including data anonymization, restricted access to sensitive information, and secure storage and transmission protocols. Ensuring encryption and compliance with relevant data protection regulations is crucial when dealing with sensitive or personal data during text mining tasks.
Building upon Daniel's concern about biases, how can we address biases that arise from users providing biased input during fine-tuning for text mining?
Excellent question, Sophie. Addressing user-generated biases during fine-tuning is crucial. Researchers need to curate well-balanced training datasets and ensure diverse input during fine-tuning. Careful selection and preprocessing of training data can help minimize biases and improve the fairness of text mining applications powered by ChatGPT.
Beyond documenting parameters and sharing code, should researchers also consider sharing access to pre-trained models for reproducibility in ChatGPT-based text mining?
That's a valid point, Oliver. Sharing access to pre-trained models can enhance reproducibility efforts and facilitate collaboration. By sharing pre-trained models, researchers can allow others to build upon their work and reproduce findings more easily, fostering transparency and advancing the field of text mining based on ChatGPT.
In social media text mining, how do we handle the dynamic nature of language, slang, and evolving trends? Can ChatGPT adapt to these changes?
Adapting to dynamic language and evolving trends can be challenging, Liam. While ChatGPT can leverage its training data to understand and respond to current language patterns, continuous model updates and fine-tuning with new data can help ensure it remains relevant and adaptable to the ever-changing landscape of social media language in text mining tasks.
Apart from healthcare, what other sensitive domains can benefit from ChatGPT in text mining? Are there any ongoing research projects in those areas?
Sensitive domains like legal, finance, and political analysis can also benefit from ChatGPT in text mining tasks. Ongoing research projects explore the potential of AI models for legal document analysis, sentiment analysis in financial markets, and political discourse analysis. The versatility of ChatGPT enables applications across various domains with sensitive information.
For researchers who would like to train their own models, what are the possible challenges in terms of time and resources?
Training your own models can indeed require significant time and resources, Chloe. It involves collecting and preprocessing large amounts of relevant data, setting up infrastructure and hardware for training, and allocating computational resources. Additionally, fine-tuning and iterative improvement processes may further demand time and expertise. Considering these factors is important before opting to train models from scratch.