Revolutionizing Result Prediction in Transfection Technology with ChatGPT
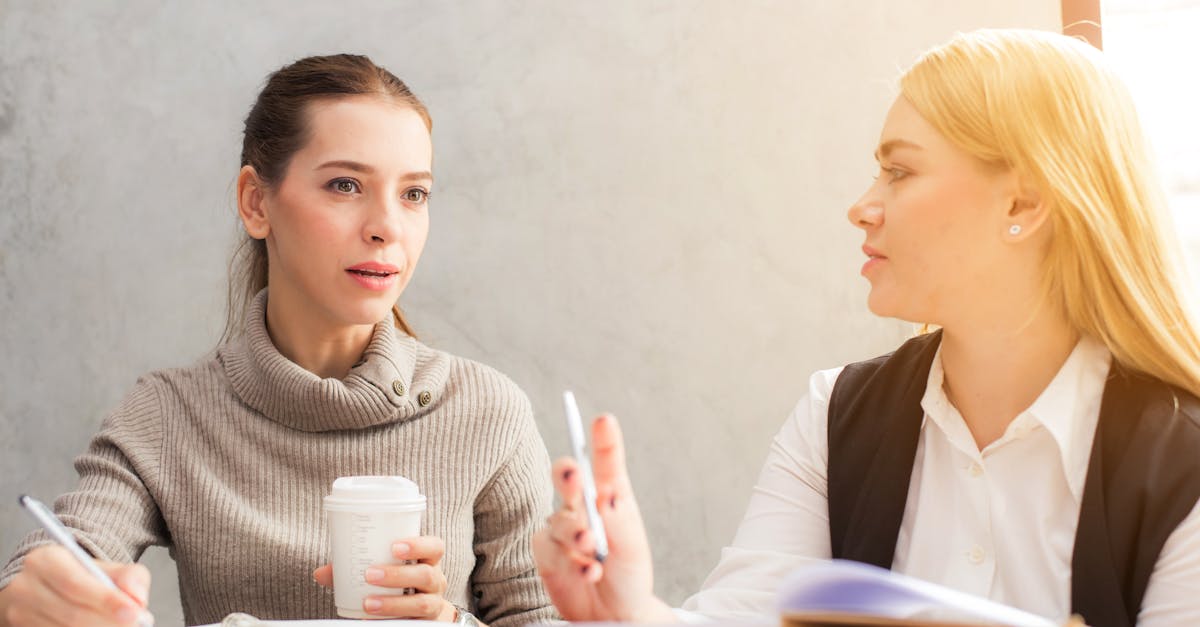
Transfection is a technology used to introduce foreign genetic material into cells. It plays a crucial role in molecular biology and genetic engineering experiments. One of the key challenges in transfection experiments is predicting the outcome or efficiency of the process. To address this challenge, researchers have developed models that can be trained to predict the results of different transfection experiments.
Technology
Transfection is the process of introducing exogenous nucleic acids, such as plasmid DNA or small interfering RNA (siRNA), into cells. This technology enables researchers to study gene expression, protein production, and cellular function by manipulating the genetic material of the cells. There are several methods of transfection, including chemical-based, electroporation, and viral-based techniques.
Area: Result Prediction
The area of result prediction in transfection experiments aims to develop models or algorithms that can estimate the outcome of a particular transfection experiment. The models are trained using a variety of input data, such as cell type, transfection method, concentration of genetic material, and other experimental variables. By analyzing and learning from a large dataset of previously conducted experiments, these models can generate predictions for new experiments.
Usage
The predictive models developed for transfection result prediction have various applications in research and industry. Some of the common usage scenarios include:
- Optimizing Transfection Conditions: By predicting the outcome of different transfection experiments, researchers can fine-tune the experimental conditions to maximize efficiency and desired outcomes. This can save time, resources, and increase the success rate of experiments.
- Gene Therapy Development: Transfection is a critical process in gene therapy, where therapeutic genes are delivered to cells to treat genetic disorders. Result prediction models can aid in identifying the most effective transfection methods and optimizing the delivery of therapeutic genes.
- Drug Development: Prediction of transfection outcomes can be valuable in drug development. Researchers can use these models to determine the effectiveness of drug candidates at targeting specific genes or cellular pathways. This information can aid in screening and selecting potential drug candidates for further investigation.
- Biological Research: Prediction models can also support basic biological research by providing insights into the behavior of different cell types and genetic materials. This information can contribute to a deeper understanding of cellular processes and help unravel complex biological mechanisms.
- Quality Control: In production environments, transfection result prediction models can be used to assess the efficiency and consistency of transfection processes. This ensures the reliability and reproducibility of experimental outcomes, especially in settings where large-scale transfection is employed.
In conclusion, transfection result prediction is an area of technology that enables researchers to estimate the outcome of various transfection experiments. By developing and training models using historical data, researchers can optimize experimental conditions, aid in gene therapy and drug development, contribute to biological research, and ensure quality control in production environments.
Comments:
Thank you all for reading my article on revolutionizing result prediction in transfection technology with ChatGPT! I'm eager to hear your thoughts and discuss any questions you may have.
Great article, Chirag! The potential of ChatGPT in optimizing transfection technology is truly exciting. Can you share some specific use cases where you think it can make a significant impact?
Thank you, Emily! ChatGPT can have a significant impact on several use cases, such as optimizing transfection protocols based on cell type, predicting protein expression levels, and identifying potential transfection inhibitors.
Hi Chirag, I enjoyed your article. Do you think using ChatGPT can enhance the reproducibility of transfection experiments?
Hi David, I'm glad you enjoyed the article! Yes, by using ChatGPT, researchers can share and discuss their experimental setups, troubleshoot potential issues, and get suggestions to improve the reproducibility of transfection experiments.
Moreover, ChatGPT can analyze experimental data and suggest improvements, generate personalized recommendations for transfection parameters, and even predict potential challenges or limitations in scaling up transfection processes.
This is fascinating! The idea of leveraging AI to optimize transfection technology is mind-blowing. Can ChatGPT be customized for specific cell lines or does it have limitations?
Hi Jennifer! Thanks for your comment. ChatGPT can indeed be customized for specific cell lines. By training the model with specific data from different cell types, it can provide more accurate predictions and suggestions tailored to a particular cell line.
However, it's important to note that ChatGPT has a few limitations. It relies on the data it was trained on and may not be aware of recent scientific developments or experimental techniques that are not part of its training data.
I'm curious about the ethical implications of using AI in transfection technology. What are your thoughts, Chirag?
Hi Amy, that's an important aspect to consider. While AI like ChatGPT can greatly assist in research, it's crucial to ensure that its use aligns with ethical guidelines. Transparency, accountability, and fairness should be at the forefront when applying AI in any field, including transfection technology.
Chirag, could ChatGPT potentially replace the need for human expertise in transfection research?
Hi Michael, while ChatGPT can provide valuable insights and predictions, it cannot completely replace human expertise in transfection research. Human researchers bring domain knowledge, intuition, and adaptability, which are essential to navigate the complexities of experimental design, interpret results, and make informed decisions.
ChatGPT is a tool to augment and support researchers, but human expertise remains irreplaceable!
Can ChatGPT be used for other applications in the field of biotechnology?
Absolutely, Rachel! ChatGPT's capabilities extend beyond transfection technology. It can be employed in various biotechnology applications such as drug discovery, genetic engineering, protein design, and optimizing cell culture conditions.
The versatility of ChatGPT enables it to assist researchers and scientists in finding innovative solutions and generating insights in different areas of biotechnology.
How accessible is ChatGPT for researchers? Are there any cost implications?
Hi Robert, accessibility is a key focus. ChatGPT is designed to be accessible to researchers in the field of transfection technology. While there may be certain costs associated with deploying and training a model specific to a lab's needs, efforts are being made to democratize AI technologies and make them more affordable and widely available.
Do you see any potential challenges in implementing ChatGPT for industrial-scale transfection processes?
Hi Daniel, scaling up the application of AI like ChatGPT for industrial-scale transfection processes can indeed pose challenges. It's crucial to ensure the model's generalizability, adaptability to different manufacturing setups, and the availability of large-scale training data. Addressing these challenges is essential for successful implementation in the industry.
What are the potential future developments you envision for ChatGPT in the field of transfection technology?
Hi Sophia, the future possibilities are immense! I envision ChatGPT becoming more refined and effective in providing personalized recommendations and insights based on specific experimental setups. Collaborative platforms integrating ChatGPT could be developed, facilitating real-time discussions among researchers to accelerate advancements in transfection technology.
Are there any privacy concerns associated with using ChatGPT in transfection research?
Hi Karen, privacy concerns are certainly critical when dealing with AI technologies. The use of ChatGPT in transfection research should ensure that sensitive data, such as proprietary experimental protocols or patient-related information, is handled securely and in compliance with applicable privacy regulations and ethical standards.
Do you think ChatGPT can contribute to reducing the time and resources required for transfection research?
Hi William, absolutely! By leveraging ChatGPT, researchers can potentially optimize their experimental designs, thereby reducing the need for trial and error. This can save both time and resources in the transfection research process, enabling scientists to focus on other aspects and accelerate advancements in the field.
Chirag, really enjoyed reading your article! How do you see the adoption of AI like ChatGPT in the field of transfection technology evolving in the coming years?
Hi Emma, I'm glad you found the article interesting! In the coming years, I believe the adoption of AI, including ChatGPT, in the field of transfection technology will continue to grow. As researchers witness the benefits of AI-assisted experimental design, result prediction, and troubleshooting, it's likely to become an integral part of their workflow, facilitating faster and more efficient research practices.
Chirag, what are the main challenges that need to be addressed to fully leverage the potential of ChatGPT in transfection technology?
Hi Jason, a few key challenges need to be addressed. Firstly, the need for robust data collection and labeling to train ChatGPT, ensuring a wide range of transfection experiments are covered. Secondly, further research is required to enhance model interpretability, providing researchers with insights into how predictions are generated by ChatGPT. Lastly, collaborative efforts between AI researchers and domain experts are vital to solve specific challenges faced in transfection technology.
How can the scientific community ensure that the use of AI in transfection technology remains trustworthy and reliable?
Hi Olivia, ensuring the trustworthiness and reliability of AI in transfection technology requires a multi-faceted approach. Apart from rigorous validation studies and continued improvements to the underlying AI models, the scientific community should encourage open sharing of data, methodologies, and code. Collaboration, transparency, and peer review play crucial roles in fostering trust and ensuring the reliability of AI-driven research practices.
What are the advantages of using AI technologies like ChatGPT over traditional methods in transfection research?
Hi Ava, AI technologies like ChatGPT offer several advantages over traditional methods in transfection research. They can process large volumes of experimental data more efficiently, identify patterns and correlations that may not be obvious to humans, and provide personalized recommendations based on specific experimental setups. AI can potentially augment human capabilities and accelerate the pace of advancements by assisting in experimental design, result prediction, and troubleshooting.
Can ChatGPT assist in identifying potential challenges or risks associated with transfection experiments?
Hi Ian, absolutely! ChatGPT can analyze experimental setups, historical data, and scientific literature to identify potential challenges or risks in transfection experiments. It can suggest alternative protocols, highlight possible limitations, and even provide insights into mitigating strategies based on existing knowledge. ChatGPT's predictive capabilities can be instrumental in maximizing the chances of success in transfection research.
Chirag, do you see any limitations in using ChatGPT for result prediction in transfection technology?
Hi Liam, while ChatGPT is a powerful tool, it has a few limitations. Firstly, it heavily relies on the data it was trained on, which means it might not be aware of recent scientific discoveries or experimental techniques not present in its training data. Secondly, like all AI models, it may generate predictions that are outliers or incorrect in specific scenarios. The scientific community should use ChatGPT as a supportive tool and exercise critical thinking while interpreting its predictions.
Chirag, can researchers leverage ChatGPT to optimize the transfection efficiency of non-standard cell types?
Hi Peter, definitely! ChatGPT can be leveraged to optimize transfection efficiency for non-standard cell types. By training the model using relevant experimental data from non-standard cell lines, ChatGPT can generate tailored recommendations and predictions specific to those cell types. It enables researchers to adapt and optimize transfection protocols for a wide range of cell lines, including those that don't fall under well-studied or commonly used categories.
Chirag, how do you envision the integration of ChatGPT with existing laboratory platforms or software?
Hi Anthony, integrating ChatGPT with existing laboratory platforms or software can enhance the user experience and accessibility. For example, researchers can have an interactive chat interface within a laboratory software where they can input experimental details and receive real-time predictive insights or recommendations from ChatGPT. APIs or plugins can facilitate such integrations, enabling seamless interactions and improving the efficiency of researchers working with transfection technology.
Chirag, what impact do you think ChatGPT can have on the democratization of scientific research in the field of transfection technology?
Hi Grace, ChatGPT can play a significant role in democratizing scientific research in transfection technology. By providing accessible and personalized recommendations, it empowers researchers at all levels, regardless of their expertise or access to resources. It has the potential to bridge the knowledge gap, facilitate collaboration, and accelerate scientific progress by enabling a wider community to leverage AI-assisted methodologies in their transfection research.
Are there any potential pitfalls or challenges in implementing ChatGPT for transfection technology?
Hi Oliver, there are a few potential pitfalls and challenges to consider in implementing ChatGPT for transfection technology. Firstly, the reliability of its predictions depends on the quality and diversity of training data. Inadequate or biased training data may lead to unreliable outcomes. Secondly, the challenge of interpretability and explainability arises, as understanding how ChatGPT generates predictions can be complex. Addressing these challenges requires continuous research and development in the field of AI.
Chirag, how can ChatGPT aid in optimizing the dosage and timing of transfection experiments?
Hi Lucy, ChatGPT can assist in optimizing the dosage and timing of transfection experiments by analyzing historical data and suggesting appropriate ranges of dosages and timings based on specific experimental objectives. It can also factor in cell type characteristics or experimental conditions to generate personalized recommendations. By engendering an iterative process, researchers can refine their dosage and timing strategies while considering the limitations and sensitivities of their transfection experiments.
Thank you, Chirag, for the insightful article! How do you think ChatGPT can impact the future of transfection technology?
Hi Sarah, you're welcome! ChatGPT has the potential to positively impact the future of transfection technology. It can enhance experimental design, result prediction, troubleshooting, and optimization processes. By augmenting human expertise and facilitating collaboration among researchers, ChatGPT can accelerate the pace of advancements, improve reproducibility, and enable scientists to address more complex challenges in transfection research. Its integration with laboratory platforms could transform the way researchers approach and conduct experiments.