Revolutionizing Revenue Analysis: Unleashing the Power of ChatGPT in Demand Forecasting
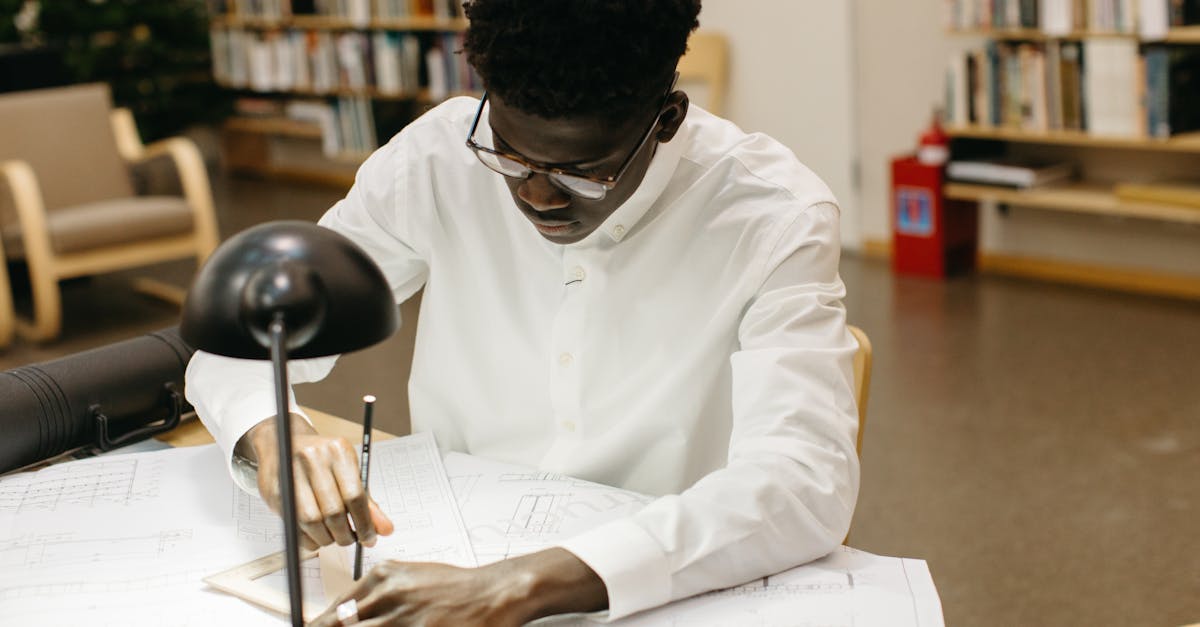
In the fast-paced business world, revenue analysis plays a crucial role in the success of companies. By accurately forecasting demand, businesses can optimize their inventory management, production planning, and ultimately maximize their revenue generation. To achieve such accurate forecasting, companies can leverage the power of technology like ChatGPT-4.
ChatGPT-4 is an advanced language model that utilizes artificial intelligence (AI) to analyze historical sales data, market trends, seasonality factors, and other relevant variables. By processing a vast amount of information, it can provide valuable insights and predict future demand levels for products or services. This technology helps businesses make informed decisions, allocate resources effectively, and stay ahead of the competition.
Benefits of ChatGPT-4 in Revenue Analysis
ChatGPT-4 offers several benefits when it comes to revenue analysis and demand forecasting. Some of the notable advantages include:
- Accurate Demand Forecasting: With its advanced AI capabilities, ChatGPT-4 can analyze complex patterns and correlations in data, allowing for accurate demand forecasting. This helps businesses avoid overstocking or understocking, resulting in optimized inventory management.
- Comprehensive Market Analysis: By processing market trends and other external factors, ChatGPT-4 can provide a comprehensive analysis of various market conditions. This information assists businesses in making strategic decisions related to pricing, promotions, and market positioning.
- Real-time Data Processing: With its ability to process and analyze data in real-time, ChatGPT-4 can provide up-to-date insights on demand fluctuations, allowing businesses to respond swiftly. This helps companies adjust their production planning and make necessary adjustments to meet changing customer demands effectively.
- Improved Revenue Generation: By leveraging the power of ChatGPT-4, businesses can optimize their revenue generation by aligning their inventory levels, production volumes, and sales strategy with predicted demand. This helps companies maximize their sales potential and increase their overall profitability.
- Enhanced Decision-making: ChatGPT-4 serves as a valuable decision-making tool, providing businesses with accurate and reliable information for forecasting, planning, and resource allocation. This enables companies to make informed decisions based on data-driven insights, leading to better overall business outcomes.
Conclusion
In the dynamic business landscape, revenue analysis is essential for companies aiming to stay competitive and achieve sustainable growth. ChatGPT-4, with its powerful AI capabilities, offers a valuable solution for demand forecasting and revenue optimization. By utilizing this technology, businesses can gain accurate insights into future demand levels, leading to improved inventory management, production planning, and overall revenue generation.
Incorporating ChatGPT-4 in revenue analysis empowers businesses to make informed decisions, respond effectively to market fluctuations, and ultimately drive success in their respective industries. With its unparalleled ability to process data and provide accurate predictions, ChatGPT-4 is a game-changer for revenue analysis and demand forecasting.
Comments:
Thank you all for taking the time to read my article on Revolutionizing Revenue Analysis with ChatGPT in Demand Forecasting. I hope you found it informative and thought-provoking. I'm here to answer any questions or hear your thoughts!
Great article, Hitesh! I love how ChatGPT can bring a conversational approach to demand forecasting. It seems like a promising tool for businesses to enhance their revenue analysis. Have you personally used it in any projects?
Thank you, Jennifer! Yes, I have had the opportunity to work with ChatGPT in a few projects. Its ability to capture complex patterns and provide interactive conversations have proven helpful in understanding demand fluctuations. It's definitely a game-changer in revenue analysis.
I have some concerns about the accuracy of using ChatGPT for demand forecasting. How reliable is the model in predicting future sales? Can it handle different types of industries?
Valid concerns, Michael. While ChatGPT is an impressive model, its predictions are based on the information it has been trained on. It performs well in many industries but might require additional fine-tuning for specific domains. Like any forecasting method, it's important to validate the generated insights through historical data analysis and domain knowledge.
Hitesh, I enjoyed your article! ChatGPT seems like an innovative approach to demand forecasting. How does it compare to traditional statistical models like ARIMA or regression analysis?
Thank you, Emily! ChatGPT offers a different perspective compared to traditional statistical models. While ARIMA and regression analysis are based on mathematical formulas, ChatGPT leverages the power of deep learning and natural language processing to have interactive conversations. It can capture long-term dependencies and is more adaptable to unstructured data.
Has ChatGPT been deployed in industries with highly volatile demand? I'm curious to know how well it handles unpredictable shifts in consumer behavior.
Good question, David! ChatGPT has shown promise in dealing with volatile demand scenarios. Its ability to understand contextual cues and capture nuanced patterns helps in adapting to shifts in consumer behavior. However, it's important to regularly update the model with new data to maintain its performance in rapidly changing environments.
Interesting read, Hitesh! Do you think ChatGPT can replace human experts in revenue analysis, or is it more effective as a complementary tool?
Thank you, Sophia! While ChatGPT is a powerful tool, it is best seen as a complementary tool rather than a replacement. It can augment human expertise by assisting in generating insights and exploring different demand scenarios. Ultimately, the combination of human domain knowledge and AI capabilities yields the best results.
How scalable is the implementation of ChatGPT in demand forecasting? Can it handle large datasets without sacrificing performance?
Good question, Daniel! ChatGPT implementation scales well with the availability of computational resources. By leveraging distributed systems and efficient algorithms, it can handle large datasets and still maintain its performance. However, it's important to ensure the infrastructure is appropriately set up to make the most out of its capabilities.
As much as I appreciate the potential of ChatGPT, what are the limitations or potential risks associated with using it in demand forecasting? Are there any ethical concerns to consider?
Great point, Adam! There are indeed limitations and potential risks to be aware of. ChatGPT's predictions heavily rely on the training data, which could introduce biases or reflect existing inaccuracies. It's crucial to ensure diverse and representative datasets are used. Ethical concerns include privacy, transparency, and accountability since AI models should be used responsibly to avoid unintended consequences.
Hitesh, do you have any recommendations for businesses interested in adopting ChatGPT for demand forecasting? How should they approach implementation and mitigate potential challenges?
Thanks for asking, Oliver! To adopt ChatGPT effectively, businesses should initially start with pilot projects to understand the tool's capabilities and limitations. It's important to involve domain experts to validate and interpret the outputs. Additionally, businesses should focus on continual model improvements, keep the data up-to-date, and establish clear guidelines for ethical and responsible AI usage.
Hitesh, what other areas of revenue analysis can benefit from ChatGPT? Are there any specific applications you foresee in the near future?
Great question, Sophia! ChatGPT has potential applications in various revenue analysis areas such as pricing optimization, market trend analysis, and customer behavior understanding. In the near future, I expect more advancements in merging ChatGPT with other analytical techniques to provide well-rounded revenue insights and proactive decision-making capabilities.
Your article has sparked my curiosity, Hitesh. Are there any open-source implementations or pre-trained models of ChatGPT that businesses can experiment with?
Glad to hear that, Emma! OpenAI has released GPT-3, the model behind ChatGPT, and several pre-trained models are available. Businesses can explore OpenAI's documentation and resources to experiment with the capabilities of ChatGPT in demand forecasting and other domains. It's exciting to see the opportunities it brings!
Hitesh, do you have any success stories or specific use cases where ChatGPT significantly improved revenue analysis for businesses?
Certainly, Sarah! In an e-commerce setting, ChatGPT helped a company understand the impact of personalized recommendations on sales, leading to a boost in revenue. In the hospitality industry, it assisted in predicting room demand based on customer preferences and external factors, optimizing pricing strategies. These are just a couple of examples where ChatGPT has shown its value.
Hitesh, what potential challenges might arise when integrating ChatGPT with existing demand forecasting systems?
Good question, Alice! One common challenge is ensuring a smooth integration between ChatGPT and existing systems. This may involve adapting data formats, establishing connectivity, and training domain-specific models if required. Organizations should also consider the computational resources needed to deploy and serve ChatGPT effectively.
Hitesh, I'm curious about the training data requirements for ChatGPT. How much historical data is typically needed for accurate demand forecasting?
Great question, Jessica! The amount of historical data required depends on various factors such as the complexity of the demand patterns, industry dynamics, and the specific problem at hand. As a rule of thumb, having at least a few years of quality data allows ChatGPT to learn and generalize well. However, more data is often useful to capture fine-grained trends and seasonality effectively.
Hitesh, how well does ChatGPT handle external factors like economic indicators or marketing campaigns? Can it analyze their impact on demand accurately?
Good question, Oliver! ChatGPT can indeed analyze the impact of external factors on demand, provided it has been trained with relevant data incorporating those factors. By incorporating economic indicators or marketing campaigns as contextual information, ChatGPT can capture their influence on demand patterns and help in forecasting accurately within the boundaries of the training data.
Hitesh, what are your thoughts on the interpretability of ChatGPT's predictions? How can businesses gain insights into the factors influencing the model's output?
Excellent question, Emma! ChatGPT's interpretability can be challenging due to its complex nature. However, using techniques like attention maps or surrogate models, businesses can gain insights into which parts of the input contribute to the model's output. Additionally, soliciting domain expert opinions alongside the predictions allows for a clearer understanding of the relevant factors influencing the generated forecasts.
Hitesh, how long does it typically take to deploy ChatGPT for demand forecasting in a real-world scenario?
The deployment timeline for ChatGPT varies depending on several factors, Samuel. It includes activities like data preparation, model training, fine-tuning, integration with existing systems, and testing. A realistic estimate would be a few weeks to a couple of months depending on the organization's readiness and complexity of the deployment.
Hitesh, thanks for shedding light on ChatGPT. How costly is it to implement and maintain such a system for demand forecasting?
You're welcome, Ava! The cost of implementing and maintaining ChatGPT depends on various factors like the scale of the deployment, computational resources, data storage, and ongoing model updates. It involves a trade-off between infrastructure investment and the anticipated value provided by improved revenue analysis. Organizations should evaluate the costs against the potential benefits and prioritize accordingly.
Hitesh, what are the potential privacy concerns that need to be addressed when using ChatGPT for demand forecasting? How can sensitive business data be protected?
Privacy is indeed a crucial aspect, Jacob. When using ChatGPT, businesses should ensure sensitive data is appropriately handled and protected. This can involve techniques like data anonymization, access controls, and secure data storage. Organizations should also consider legal and compliance requirements while adopting and deploying ChatGPT to safeguard their customers' and proprietary information.
Hitesh, are there any constraints on the data formats or types that can be used with ChatGPT for demand forecasting?
Good question, Sophia! ChatGPT can handle a variety of data formats, including textual and numerical inputs. It can process time-series data, categorical variables, and contextual information effectively. However, it's important to ensure the data is properly formatted and prepared to match the inputs the model has been trained on.
Hitesh, do you foresee any limitations in scaling ChatGPT for massive enterprises with vast amounts of data and complex revenue streams?
Good question, Andrew! Scaling ChatGPT for massive enterprises with complex revenue streams can introduce challenges related to computational resources, data management, and fine-tuning for specific business contexts. While it's feasible, bespoke solutions and architecture enhancements may be necessary to ensure optimal performance and scalability at that scale.
Hitesh, how can businesses measure the success or effectiveness of using ChatGPT in their demand forecasting processes?
Measuring the success of using ChatGPT in demand forecasting involves multiple factors, Ethan. Key metrics can include forecasting accuracy, reduction in forecasting errors, improvements in revenue performance, and the ability to generate valuable insights. Establishing a baseline with existing forecasting methods allows for a comparison to understand the tangible benefits the integration of ChatGPT brings.
Hitesh, could you provide an overview of the specific steps involved in implementing ChatGPT for demand forecasting from scratch?
Certainly, Sophie! Implementing ChatGPT for demand forecasting involves steps like data collection, preprocessing, training, fine-tuning, evaluation, and integration. Data labeling or annotation might be necessary for specific applications. Organizations need to identify or develop infrastructure for model training and deployment, ensuring compatibility with existing systems. It's an iterative process that requires domain expertise and collaboration across teams.
Hitesh, what kind of computational resources are typically needed to train and deploy ChatGPT for demand forecasting?
Good question, Julia! The computational resources required for training and deploying ChatGPT vary depending on factors like the size of the model, complexity of the data, and the desired performance. Training large models on extensive datasets might require high-performance GPUs or TPUs. Deployment can be facilitated using cloud-based services, distributed computing, or dedicated server setups.
Hitesh, can ChatGPT handle demand forecasting for short-term periods effectively, like hourly or daily predictions?
Absolutely, Lucas! ChatGPT can handle short-term demand forecasting effectively, including hourly or daily predictions. By incorporating granular time-series data and contextual information, it can capture patterns and fluctuations at finer temporal resolutions. It offers the flexibility to generate forecasts at different time scales while preserving interpretability and conversational capabilities.
Hitesh, what are some potential drawbacks of using ChatGPT in demand forecasting, and how can they be mitigated?
Good question, Sophia! One drawback is that ChatGPT might generate plausible but incorrect-sounding responses. To mitigate this, organizations can employ ensemble models, cross-validation techniques, or employ dedicated feedback loops to improve accuracy. Additionally, continuously fine-tuning the model with new domain-specific data helps in addressing limitations and improving overall performance.