Revolutionizing Soil Quality Analysis: Enhancing Ecological Restoration with ChatGPT Technology
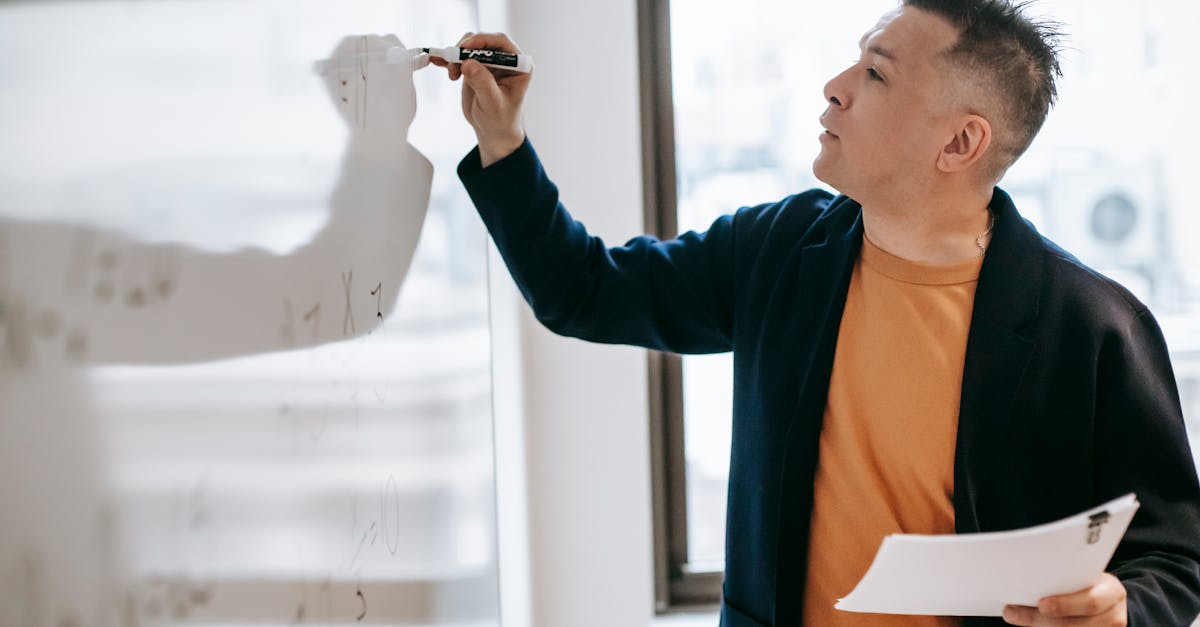
Ecological restoration involves the process of repairing, rehabilitating, and restoring degraded ecosystems for environmental sustainability and biodiversity conservation. One of the key factors determining the success of restoration projects is soil quality. Soil analysis provides valuable insights into soil health and fertility, enabling restoration practitioners to make informed decisions about suitable restoration strategies and interventions.
Advancements in technology, particularly in the field of algorithms, have greatly enhanced the accuracy and efficiency of soil quality analysis. Algorithms, defined as step-by-step procedures or instructions for solving problems, can be used to process large amounts of soil data and extract relevant information. This information can then be used to assess soil health, identify potential deficiencies, and recommend appropriate restoration measures.
Soil quality analysis algorithms leverage various data inputs to evaluate soil characteristics such as pH levels, nutrient content, organic matter composition, and physical properties. These algorithms employ statistical models, machine learning techniques, and data mining approaches to analyze the data and generate meaningful insights.
One common algorithm used in soil quality analysis is the Random Forest algorithm. This algorithm combines multiple decision tree models to generate predictions and assess the importance of different soil variables in determining soil health. By analyzing soil data collected from various locations within a restoration site, the Random Forest algorithm can identify patterns, correlations, and anomalies, enabling restoration practitioners to formulate targeted restoration strategies.
Another widely used algorithm is the Support Vector Machine (SVM), which is particularly effective in classifying soil samples based on different attributes. SVM utilizes a mathematical approach to create a hyperplane that separates different classes of soil types based on their properties. By analyzing soil data using SVM, restoration practitioners can gain insights into the spatial distribution of soil types within a restoration site, facilitating the selection of appropriate plant species and restoration techniques.
Furthermore, algorithms can assist in predicting the potential impact of restoration interventions and designing optimal restoration plans. By inputting soil data, environmental factors, and project objectives into suitable algorithms, restoration practitioners can simulate different scenarios and evaluate the potential outcomes. This allows for informed decision-making and improved cost-effectiveness in ecological restoration projects.
In conclusion, the application of algorithms in soil quality analysis plays a crucial role in ecological restoration projects. By leveraging technology and data analysis techniques, restoration practitioners can gain valuable insights into soil health and fertility, enabling them to make informed decisions regarding restoration strategies and interventions. As technology continues to advance, algorithms will continue to be an indispensable tool in the pursuit of effective and sustainable ecological restoration.
Comments:
This article is fascinating! The use of ChatGPT technology to enhance ecological restoration is truly innovative. I'm curious to know more about how it works and its potential impact.
Thank you, Emily! ChatGPT technology is a language model developed by OpenAI. It can generate human-like text based on the prompts it receives. By using ChatGPT for soil quality analysis, we can obtain insights and recommendations to enhance ecological restoration practices.
I agree, Emily! This technology could revolutionize the way we approach soil quality analysis and potentially lead to more efficient ecological restoration projects. Kyle, have there been any practical applications or case studies with ChatGPT in this context?
Absolutely, Oliver! There have been some successful pilot studies where ChatGPT was used to analyze soil quality data and provide actionable recommendations. It showed promising results, but further research and testing are still necessary to fully validate its potential impact.
That's great to hear, Kyle! I can see the potential of ChatGPT to assist land managers, conservationists, and researchers in making informed decisions. It would be interesting to see how it compares to traditional soil analysis methods in terms of accuracy and cost-effectiveness.
Indeed, Oliver! Comparing ChatGPT to traditional methods is an important part of the evaluation process. While ChatGPT can provide quick and automated analysis, ensuring its accuracy and cost-effectiveness in different scenarios is crucial before wider adoption.
This sounds like a game-changer! The ability to obtain real-time insights and recommendations for ecological restoration based on soil quality analysis can greatly improve decision-making and resource allocation. How accessible is ChatGPT technology for practitioners in the field?
Good question, Sophia! OpenAI aims to make their models accessible to as many people as possible. While deployment details are still being worked on, the intention is to provide easy-to-use interfaces that allow practitioners to leverage the power of ChatGPT for their ecological restoration projects.
I appreciate the focus on accuracy. Soil health is a critical factor in ecological restoration, and the information provided by ChatGPT needs to be reliable. How does the model handle uncertainties in soil data or potential errors in analysis?
Valid concern, Sophia! ChatGPT acknowledges uncertainties and potential errors. It aims to highlight these aspects to users and provides confidence estimates for its generated responses. It's imperative for practitioners to interpret and validate the information provided by ChatGPT, considering the inherent uncertainties.
That makes sense, Kyle. Having transparent and interpretable results is crucial to trust the technology. I'm glad to see the consideration given to uncertainties associated with soil analysis. It would be interesting to see real-world case studies showcasing the model's performance.
I completely agree, Sophia. Case studies can provide valuable insights into ChatGPT's practical performance and help establish its credibility in the field. Kyle, are there any ongoing studies or plans for such case studies?
Absolutely, Oliver! OpenAI is actively collaborating with ecological restoration organizations to conduct extensive field tests and case studies. These studies will help assess the model's performance across different ecosystems and allow practitioners to understand its strengths and limitations.
That's fantastic news! Real-world application and validation are crucial for the adoption of new technologies. It's great to hear that OpenAI is actively engaged in conducting studies to ensure the reliability and usefulness of ChatGPT.
I'm curious about the scalability of ChatGPT. Soil quality analysis often involves large datasets and complex analyses. Can the model handle the volume and complexity of data typically encountered in ecological restoration?
Great question, Emily! ChatGPT can handle large volumes of data, but there are limitations to consider. Processing and analyzing complex datasets might require specialized adaptations and enhancements. OpenAI is actively exploring ways to improve the model's scalability for different applications, including ecological restoration.
Thank you for the clarification, Kyle. It's encouraging to know that scalability is being addressed. This technology certainly has the potential to transform soil quality analysis and make ecological restoration processes more data-driven and precise.
Absolutely, Emily. The combination of advanced AI technology like ChatGPT with comprehensive soil analysis can unlock new possibilities in ecological restoration. I'm excited to see how this field evolves with the integration of such innovative tools.
This article is a game-changer! The potential of ChatGPT technology in ecological restoration is immense. It's amazing to envision a future where we can leverage AI models like ChatGPT to make data-driven decisions for restoring degraded ecosystems.
I agree, Henry! The ability to analyze soil quality efficiently and accurately is crucial for successful ecological restoration. ChatGPT technology seems like a promising tool that can greatly simplify and enhance the process.
This technology could bring about a paradigm shift in ecological restoration practices. The insights and recommendations provided by ChatGPT can empower practitioners and researchers, enabling them to make informed choices and achieve better outcomes.
Absolutely, Jack! The integration of AI in soil quality analysis could revolutionize the field. It has the potential to save time, resources, and improve restoration strategies, leading to more efficient and sustainable outcomes.
As a soil scientist, I'm thrilled to see the advancements in technology that can enhance soil quality analysis. ChatGPT's potential for ecological restoration is intriguing, and I'm eager to see its practical application in the field.
I completely agree, Daniel! Soil analysis is a critical component of ecological restoration, and technologies like ChatGPT can provide valuable insights to aid in the decision-making process. It's an exciting time for the field.
The ability to obtain real-time insights and recommendations through ChatGPT technology can accelerate the restoration process. It can empower land managers and practitioners to make informed decisions that contribute to more successful restoration outcomes.
I couldn't agree more, Thomas! With the potential of ChatGPT, restoration efforts can become more efficient and targeted. Improved decision-making based on reliable data can lead to significant positive impacts on ecosystems.
I'm curious about the level of accuracy achieved by ChatGPT in soil quality analysis. Can it match or surpass the accuracy of traditional laboratory methods?
That's a valid concern, Liam. While ChatGPT shows promising results, it's crucial to validate its accuracy against traditional laboratory methods. Comprehensive comparisons and validations will help determine its reliability and establish its credibility in the field.
Indeed, Sophie. Validating ChatGPT's accuracy against traditional methods is an ongoing process. By conducting rigorous comparisons and continuous improvements, we aim to ensure that ChatGPT provides reliable results that align with or surpass conventional approaches.
I appreciate the focus on rigorous validation, Kyle. Accurate soil analysis is crucial for successful ecological restoration. It's exciting to see technology like ChatGPT being developed and refined for such critical applications.
The potential of AI in ecological restoration is immense! I'm eager to see how tools like ChatGPT can empower and support restoration practitioners, taking the field to new heights.
I'm also excited, Emma! AI can augment human expertise and enhance decision-making in ecological restoration. The integration of ChatGPT technology seems like a step in the right direction for a more sustainable future.
The article highlights the exciting potential of leveraging AI for ecological restoration. It's great to see the advancements being made in soil quality analysis and how technologies like ChatGPT can contribute to more effective restoration practices.
I agree, Nathan! Innovation in soil quality analysis is key to driving progress in ecological restoration. ChatGPT shows promise in providing insights that can optimize restoration efforts and assist practitioners in achieving their goals.
It's incredible to see how AI technology is being applied in diverse fields, including ecological restoration. ChatGPT's potential to enhance soil quality analysis is certainly a development worth noting and exploring further.
Absolutely, Ethan! The integration of AI in ecological restoration brings new possibilities and approaches. The advancements in ChatGPT technology have the potential to empower practitioners and improve the outcomes of restoration projects.
The discussions around ChatGPT's potential applications in ecological restoration are fascinating. It's exciting to envision a future where AI technology can assist in making our planet healthier and more resilient.
I couldn't agree more, Max! By leveraging the capabilities of ChatGPT and other AI models, we can create more sustainable solutions for ecological restoration and contribute positively to our environment.
The integration of AI technology in ecological restoration has the potential to optimize resource allocation and improve restoration outcomes. ChatGPT's role in enhancing soil quality analysis is an exciting development for the field.
Well said, Audrey! The use of AI models like ChatGPT can enable restoration practitioners to make data-driven decisions, leading to more efficient and successful restoration efforts.
AI models like ChatGPT have the potential to revolutionize not just ecological restoration, but also various other fields. It's impressive to see how technology can pave the way for more sustainable and data-informed practices.
Absolutely, Adam! The applications of AI in ecological restoration are vast, and ChatGPT is just one example of how technology can be harnessed for positive environmental impact.
As an ecologist, I'm excited about the potential of ChatGPT technology in ecological restoration. The ability to leverage AI models for soil quality analysis can undoubtedly accelerate our understanding and decision-making in restoring ecosystems.
I share your excitement, Grace! The integration of AI and ecological restoration holds so much promise. ChatGPT's ability to analyze soil quality data can unlock new insights and strategies for successful restoration projects.
This article highlights the power of combining technology with ecological restoration goals. ChatGPT's potential in soil quality analysis can be a game-changer, ultimately leading to more effective and sustainable restoration practices.
I'm amazed by the exciting possibilities that AI technology brings to ecological restoration. ChatGPT's role in enhancing soil quality analysis is just the beginning of what AI can contribute to the field.
The advancements in AI technology like ChatGPT have the potential to revolutionize ecological restoration. By harnessing the power of data analysis, practitioners can make informed decisions that lead to more successful restoration outcomes.
I completely agree, Sophia! The integration of AI technology enables us to leverage data for more efficient and targeted restoration efforts. ChatGPT's potential in analyzing soil quality data aligns perfectly with the needs of ecological restoration.
The adoption of AI technology like ChatGPT in ecological restoration is a significant step forward. It has the potential to streamline soil quality analysis processes and guide more informed decision-making for restoration practitioners.
Well said, Lily! The application of advanced AI models opens up new possibilities for optimizing restoration practices. ChatGPT's potential in enhancing soil quality analysis can contribute to more successful and impactful ecological restoration projects.