Revolutionizing Solvency Analysis: Unleashing the Power of ChatGPT for P&L Responsibility Technology
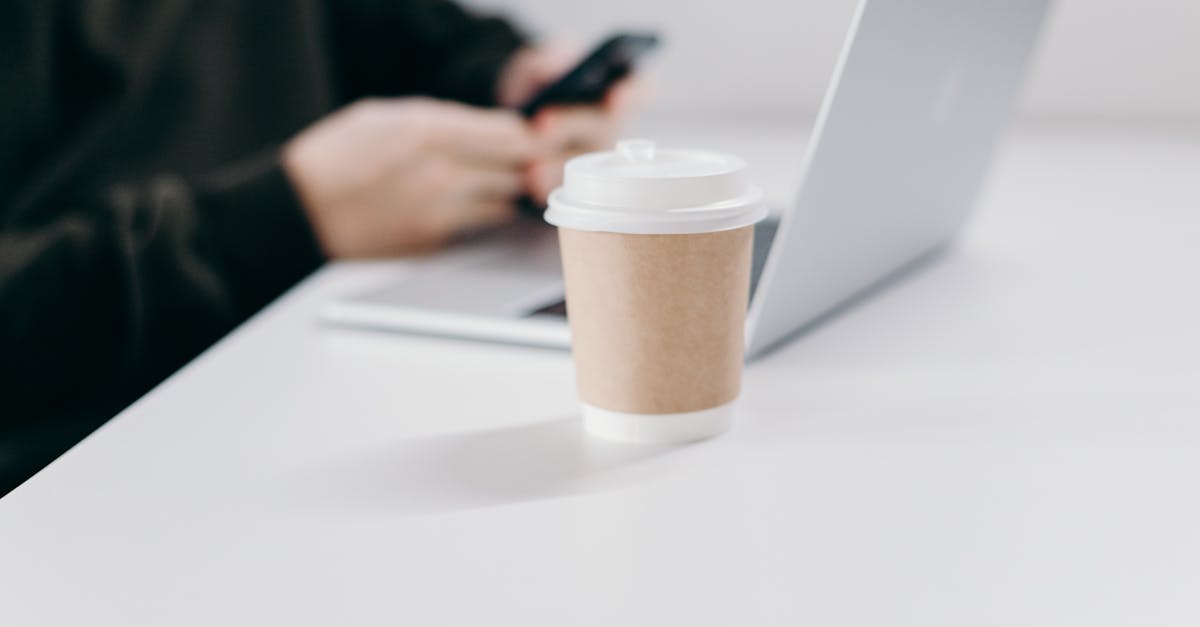
As companies strive to maintain financial stability and ensure their long-term viability, measuring solvency becomes a crucial task. Solvency analysis involves evaluating a company's ability to meet its long-term obligations and remain solvent.
Traditionally, solvency analysis has relied on financial ratio calculations and other traditional methods. However, with the advancements in technology, machine learning techniques have emerged as a powerful tool to predict and assess a company's solvency.
P&L Responsibility
P&L (Profit and Loss) responsibility refers to the accountability of an individual or department for managing and achieving the financial performance of a company. Solvency analysis falls under the P&L responsibility of financial analysts and risk managers.
Solvency Analysis
Solvency analysis is the process of assessing a company's long-term financial health and its ability to meet its financial obligations. It involves analyzing various financial indicators, ratios, and trends to estimate the solvency position of a company.
Machine learning techniques can be integrated into solvency analysis, enabling financial professionals to make more accurate predictions and improve decision-making processes.
The Role of Machine Learning
Machine learning algorithms can analyze large volumes of financial data and identify patterns and trends that may not be evident through traditional analysis methods. By training the models with historical data and relevant variables, machine learning algorithms can learn to predict the solvency of a company.
The key advantage of machine learning is its ability to process vast amounts of data from different sources, including financial statements, market data, and industry trends. This helps in obtaining a holistic view of a company's financial situation and identifying potential risks that may affect its solvency.
Benefits of Machine Learning in Solvency Analysis
By incorporating machine learning techniques into solvency analysis, analysts can benefit in several ways:
- Improved Accuracy: Machine learning algorithms can analyze a wide range of variables and generate more accurate predictions, reducing the likelihood of errors in solvency assessments.
- Real-time Monitoring: Machine learning models can be continuously updated with real-time data, allowing for proactive monitoring of a company's solvency position.
- Early Warning System: Machine learning algorithms can identify early signs of financial distress and alert analysts to take necessary actions.
- Efficiency: The automation of solvency analysis through machine learning can save time and resources for financial professionals, enabling them to focus on more strategic tasks.
Limitations and Challenges
While machine learning offers several advantages, it is important to understand its limitations and challenges:
- Data Quality: Machine learning models heavily rely on the quality and accuracy of the input data. Inaccurate or incomplete data can lead to misleading predictions.
- Model Interpretability: Some machine learning algorithms, such as deep learning models, can be black boxes, making it difficult for analysts to interpret the underlying factors influencing solvency predictions.
- Data Privacy and Security: The use of sensitive financial data in machine learning models raises concerns about data privacy and security.
- Human Expertise: While machine learning can enhance solvency analysis, human expertise is still essential to interpret the results and make informed decisions.
Conclusion
The application of machine learning in solvency analysis has the potential to revolutionize the way financial professionals predict and evaluate the solvency of companies. By harnessing the power of machine learning algorithms, analysts can obtain more accurate and timely insights, enabling them to make informed decisions and mitigate financial risks effectively.
However, it is crucial to recognize the limitations and challenges associated with machine learning to ensure the integration of this technology aligns with ethical and regulatory standards.
Overall, machine learning in solvency analysis opens up new opportunities for financial professionals to enhance their risk management capabilities and contribute to the long-term success of organizations.
Comments:
Thank you all for joining the discussion on my blog article! I'm excited to hear your thoughts on how ChatGPT can revolutionize solvency analysis.
Great article, Agha Morano! ChatGPT definitely has the potential to transform P&L responsibility technology. The natural language capabilities can make risk assessment and decision-making processes more efficient.
I appreciate your insights, Agha Morano. However, I have concerns about the reliability of AI-based tools like ChatGPT. How can we ensure unbiased and accurate analysis?
Connor Gallagher, you bring up an important point. AI biases can be a concern. Transparency in the training and deployment of these models is crucial. Implementing a robust and diverse training dataset can help mitigate biases.
Thank you for addressing my concern, Agha Morano. Transparency and diverse training data indeed play a crucial role in tackling biases. User education on the limitations of AI tools is also vital.
I completely agree, Agha Morano. Educating users about the limitations of AI models is as important as implementing transparency and diverse training data to ensure responsible use.
I agree that ChatGPT could be a game-changer for solvency analysis. It could assist analysts in extracting key insights from vast amounts of complex financial data, improving decision-making.
Agha Morano, how do you see ChatGPT integrating with existing solvency analysis tools? What are the potential challenges in implementing this technology in real-world applications?
While the idea seems promising, I wonder about the legal and regulatory aspects of relying on AI models for P&L responsibility. Are there any compliance issues to consider?
That's an interesting angle, Samuel Fischer. Compliance is important in solvency analysis, and ensuring AI models meet regulatory requirements is vital. Agha Morano, have you come across any frameworks to address this?
As an actuary, I see immense potential in using ChatGPT for solvency analysis. It can help automate repetitive tasks and free up time for more complex analyses. Exciting times ahead!
Jacob Thompson and Samuel Fischer, you raise valid concerns. Regulatory compliance is crucial. Frameworks like Model Audit Rule (MAR) can provide guidelines for assessing and validating AI models in the financial sector.
Agha Morano, I'm curious about the scalability of ChatGPT. Can it handle large volumes of financial data in real-time without compromising speed or accuracy?
Adam Clarke, scalability is a key aspect. While ChatGPT has limitations, advancements are being made to improve its performance on large datasets. Techniques like model parallelism and distributed training can enhance scalability.
The potential benefits of leveraging ChatGPT for P&L responsibility technology are exciting, but we must also address cybersecurity risks. How can we ensure the protection of sensitive financial data in AI-powered systems?
Olivia Roberts, cybersecurity is indeed vital. Implementing robust encryption, data anonymization, and access controls can help safeguard sensitive financial data.
Agha Morano, thank you for addressing the cybersecurity aspect. Regular security audits, strong encryption, and strict access controls should be part of the AI system implementation to mitigate risks.
Agha Morano, what are the potential ethical concerns that come with relying on AI models like ChatGPT for financial analyses, especially when it comes to decision-making and accountability?
Rebecca Nguyen, you raise a significant concern. Ethical considerations are paramount. AI models must be transparent, auditable, and used as decision aids rather than black boxes. Human accountability should always be involved.
Agha Morano, I'm pleased to hear your emphasis on transparency and human accountability. It's essential to ensure AI supports human judgment without replacing it.
I must say, I'm excited about the possibilities ChatGPT brings for P&L responsibility. Its ability to analyze unstructured data and provide insightful analysis could greatly benefit financial institutions.
The advancements in AI and ChatGPT are fascinating, but we should be cautious in relying solely on technology for complex financial analysis. Human judgment and oversight are still crucial.
ChatGPT has the potential to reduce manual effort in solvency analysis, but we can't overlook potential biases ingrained in AI models. Continual monitoring and auditing are essential to prevent unintended consequences.
Agha Morano, how do you see the implementation of ChatGPT impacting the role of financial analysts? Will it replace certain tasks or simply enhance their capabilities?
Great article, Agha Morano! I'm particularly intrigued by the use of ChatGPT in solvency analysis. It has the potential to streamline financial risk assessment while considering a variety of factors.
Thank you, Mason Lewis! Indeed, ChatGPT can streamline risk assessment by automating certain tasks, allowing analysts to focus on more complex analysis and decision-making.
I couldn't agree more, Agha Morano. Regular audits and evaluations of AI models can help uncover biases and ensure they don't impact decision-making.
Agha Morano, could you shed some light on the training process of ChatGPT for solvency analysis? How can we ensure the model learns from accurate and reliable financial data?
Grace Mitchell, training ChatGPT requires a diverse dataset of accurate financial information. Emphasizing robust data collection methods and domain expertise while curating the dataset can help ensure reliable training.
Thank you for your response, Agha Morano. Ensuring accurate and reliable training data is indeed critical to prevent biases and improve model effectiveness.
Agha Morano, what are the potential limitations in using ChatGPT for solvency analysis? Are there certain scenarios where it may not be as effective or accurate?
ChatGPT seems like an exciting tool to enhance solvency analysis, but it's important to remember that AI models are only as good as the data they are trained on. Data quality and representativeness are of utmost importance.
Agha Morano, I'm impressed by the potential of ChatGPT in solvency analysis. Incorporating interpretable AI models can help financial institutions gain better insights and improve risk management practices.
The article shows great promise, Agha Morano. However, we need to ensure that AI models like ChatGPT are explainable and don't hinder regulatory compliance by being too opaque.
Absolutely, Aiden Thompson. Explainability is crucial in financial institutions where transparency and compliance with regulations are key requirements.
Great article, Agha Morano! I wonder how ChatGPT's performance compares to traditional solvency analysis methods. Are there any benchmarks or studies we can refer to?
Ella Myers, comparing ChatGPT's performance with traditional methods is a valuable question. Several studies on benchmark datasets have demonstrated promising results, but more research and real-world testing are necessary.
Agha Morano, what are your thoughts on the role of human judgment in conjunction with AI-powered solvency analysis? How do we strike the right balance?
Alex Cooper, human judgment plays a critical role in conjunction with AI-powered solvency analysis. The aim is to use AI as a decision aid, considering its insights alongside human expertise, intuition, and regulatory requirements.
Agha Morano, how can financial institutions ensure the transparency of AI models like ChatGPT to build trust with their stakeholders and regulators?
Victoria Foster, transparency in AI models can be fostered through clear documentation, disclosure of model limitations, and external audits. Engaging stakeholders and regulators in the process can help build trust.
ChatGPT's potential within solvency analysis is extraordinary. The ability to analyze complex financial data and generate insights can greatly benefit risk assessment processes.
Agha Morano, how do you see ChatGPT evolving in the future? Are there any exciting potential applications beyond solvency analysis that you can envision?
Ryan Reynolds, the evolution of ChatGPT is an exciting prospect. It can be further trained on domain-specific data, enabling applications like automated underwriting, risk assessment for insurance, and more.
As a risk analyst, I find this article intriguing. ChatGPT could potentially make solvency analysis more accessible, allowing non-technical stakeholders to engage in the process.
Jennifer Scott, I'm glad you find it intriguing! Making solvency analysis more accessible is indeed one of the potential advantages of AI-based tools like ChatGPT.
Agha Morano, given the dynamic nature of financial markets, how can we ensure that ChatGPT remains up-to-date and relevant? Is continuous model training necessary?
Emma Baker, financial markets' dynamics require regular updates to keep ChatGPT relevant. Continuous model training, incorporating new data, and monitoring its effectiveness are vital for accurate and up-to-date analysis.
Thank you for the clarification, Agha Morano. Regular model updates and data incorporation seem crucial to ensure reliable outputs.
Agha Morano, I'm curious about the potential limitations of ChatGPT's language understanding capabilities. Are there any challenges in accurately analyzing financial jargon or complex terminology?