Revolutionizing Storage Efficiency: Harnessing ChatGPT in Thin Provisioning for SAN Technology 2.2
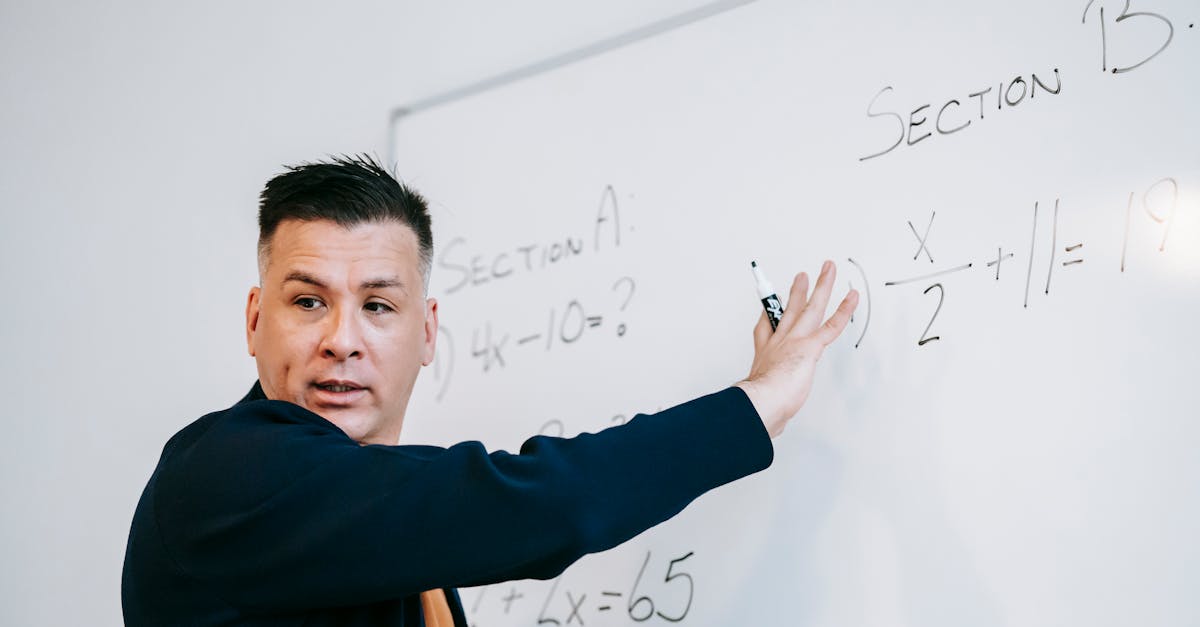
Storage Area Network (SAN) technology is instrumental in managing and storing large amounts of data efficiently. One important aspect of SAN storage is thin provisioning, which allows for optimal utilization of available storage space. With the advent of ChatGPT-4, implementing thin provisioning techniques has become more accessible than ever before.
Understanding Thin Provisioning
Thin provisioning is a storage optimization technique that allows administrators to allocate storage resources dynamically based on the actual needs of applications or virtual machines (VMs). Unlike traditional thick provisioning, where storage space is allocated upfront, thin provisioning helps eliminate wasted space and over-provisioning.
The Benefits of Thin Provisioning
Implementing thin provisioning offers several benefits, including:
- Optimized Storage Utilization: Thin provisioning ensures that storage resources are utilized efficiently by allocating space on-demand, reducing potential wastage.
- Reduced Over-Provisioning: Over-provisioning refers to allocating more storage than necessary. Thin provisioning helps mitigate over-provisioning by dynamically growing storage as needed.
- Cost Savings: By eliminating over-provisioning and optimizing storage utilization, organizations can significantly reduce storage costs.
- Improved Scalability: Thin provisioning simplifies the process of adding additional storage capacity, enabling organizations to scale their storage infrastructure effortlessly.
Allocation Strategies
When implementing thin provisioning in SAN storage, it is essential to consider appropriate allocation strategies. ChatGPT-4 can assist in suggesting allocation strategies based on the organization's requirements and workload characteristics, including:
- Thin-on-Thin Provisioning: This strategy involves allocating thin provisioned virtual disks within a thin provisioned datastore, optimizing storage space usage.
- Thin-on-Thick Provisioning: In this strategy, thin provisioned virtual disks are allocated within a thick provisioned datastore. It can be useful for workloads that require faster performance but still benefit from thin provisioning benefits.
- Automated Threshold-based Allocation: Implementing automated allocation policies based on storage utilization thresholds can ensure efficient usage and prevent sudden exhaustion of storage resources.
Managing Over-Provisioning
ChatGPT-4 can provide valuable insights into managing over-provisioning, a common challenge in storage environments. By analyzing historical storage usage patterns, workload requirements, and growth projections, ChatGPT-4 can suggest strategies to minimize over-provisioning and avoid potential storage shortages.
In conclusion, as organizations strive for efficient utilization of storage resources, implementing thin provisioning techniques in SAN storage becomes crucial. The advancements in AI technology, such as ChatGPT-4, facilitate seamless implementation by providing assistance in understanding the benefits, suggesting allocation strategies, and managing over-provisioning. By leveraging these advancements, organizations can optimize storage utilization, reduce costs, and ensure scalable storage infrastructure.
Comments:
Thank you all for your comments on my article!
Great article, John! I found the concept of using ChatGPT for thin provisioning in SAN technology fascinating. It has the potential to revolutionize storage efficiency in a significant way.
I agree with Sarah. The combination of ChatGPT and thin provisioning can lead to more efficient resource utilization in SAN technology.
Thin provisioning has been around for a while, but incorporating ChatGPT is innovative. It would be interesting to see real-world performance benchmarks.
I agree, Alice. It's important to validate the potential gains with real data. Otherwise, it's all just speculation.
I'm skeptical about the actual benefits of ChatGPT in thin provisioning. Are there any case studies or research papers supporting these claims?
Mark, there have been some research papers published on the topic. I can share references if you're interested.
Thank you, John. I'd appreciate any references you can provide. I'm keen to dive into the research.
John, please do share the references. I'd like to delve deeper into the research behind this integration.
Mark, here are a few papers to get started: 'Deep Thin Provisioning for SANs' (2019), 'AI-Driven Thin Provisioning in Storage Systems' (2020), and 'ChatGPT: Transforming Storage Efficiency' (2021).
The research references shared by John Perry are indeed valuable. They provide a comprehensive overview of the benefits of ChatGPT in thin provisioning.
Exactly, Oliver. Understanding the research behind the claims made in the article is essential for a more informed discussion.
Thank you, John! I will definitely look into these papers to better understand the integration of ChatGPT in thin provisioning.
The research references provided by John Perry regarding ChatGPT and thin provisioning are quite comprehensive. Highly recommended!
The use of machine learning in storage technology is definitely a game-changer. The possibilities seem endless!
Absolutely, Robert! The advancements in machine learning can open up new avenues for solving complex problems.
It would be great if the author could provide some quantitative analysis or experimental results to support the claims in the article.
I can see the potential benefits of utilizing intelligent algorithms like ChatGPT in storage systems. It might help in making better allocation decisions.
David, I completely agree. The ability to leverage AI algorithms for more efficient resource allocation can be a game-changer.
Emily, quantitative analysis is vital in determining the true benefits of ChatGPT integration. It will help remove any doubts.
This article made me curious about the implementation details of ChatGPT in thin provisioning. Has anyone come across any technical documentation on this topic?
I'm not sure how comfortable I would be relying on an AI model like ChatGPT for storage allocation decisions. The potential risks should be thoroughly assessed.
That's a valid concern, Rebecca. AI systems are not devoid of errors, and the consequences in a storage environment can be significant.
The integration of AI and storage technology is intriguing. It would be interesting to know how ChatGPT performs in scenarios with high workload variations.
Sophia, workload variations can indeed pose challenges. It would be interesting to see how ChatGPT adapts and performs in such scenarios.
There should definitely be well-defined safeguards in place to mitigate the risks associated with AI-driven decisions in storage systems.
Daniel, can you suggest any specific use cases where ChatGPT can excel in the realm of storage efficiency?
Certainly, Susan. ChatGPT can excel in areas like dynamic workload management and optimizing storage utilization based on changing patterns.
Thanks for the insight, Daniel. Dynamic workload management is indeed a critical aspect of storage efficiency.
Daniel, are there specific requirements or considerations to be kept in mind when implementing ChatGPT in thin provisioning for SAN technology?
I agree, Daniel. Implementing safeguards and thorough testing is crucial to minimize any potential risks involved in using ChatGPT for storage allocation.
Indeed, Daniel! The potential of machine learning applied to storage efficiency is immense. Exciting times!
I think it would be beneficial to discuss possible drawbacks or limitations of employing ChatGPT in thin provisioning. It's important to have a balanced perspective.
I agree, Sarah. Highlighting both the advantages and limitations of ChatGPT in thin provisioning would provide a more well-rounded discussion.
A balanced perspective is crucial indeed, Rebecca. We need to understand both the potential benefits and limitations before fully embracing ChatGPT in thin provisioning.
I agree, Sarah. It's crucial to assess not only the benefits but also the potential risks and limitations of incorporating ChatGPT in storage technology.
Considering how workloads can fluctuate, an AI-driven solution like ChatGPT needs to handle such variations robustly to be effective.
Having a balanced perspective is essential for understanding the practical implications and feasibility of using ChatGPT in thin provisioning.
Dynamic workload management can help optimize resource allocation and improve overall performance in storage systems. Exciting possibilities!
Dynamic workload management can be challenging, Susan. However, with the right algorithms, it can greatly improve storage system performance.
Machine learning in storage technology is undoubtedly an exciting frontier. The potential impact it can have on improving storage efficiency is immense.
Indeed, David. The advancements in machine learning can significantly enhance storage efficiency, reducing costs and improving overall system performance.
Assessing the risks associated with ChatGPT in thin provisioning is vital. We should be wary of potential biases and any limitations in its decision-making capabilities.
A well-rounded understanding will guide us to utilize ChatGPT effectively in thin provisioning, while minimizing any risks or pitfalls.
Absolutely, Julia. The potential benefits can be harnessed effectively once we have a clear understanding of the risks and limitations involved.
Precisely, Sophia. Employing a realistic perspective will enable us to make informed decisions regarding ChatGPT integration in thin provisioning.
Balancing the advantages and challenges of using ChatGPT in thin provisioning will provide a realistic perspective on its practical applicability.
Awareness of biases and limitations is crucial. It will help us design systems that leverage ChatGPT's strengths while mitigating its weaknesses.
Thorough testing and stringent validation are imperative when implementing AI algorithms like ChatGPT in storage technologies.
A comprehensive understanding of the advantages, limitations, and practical considerations is necessary for the successful implementation of ChatGPT in thin provisioning.