Revolutionizing Structural Biology: Exploring the Potential of ChatGPT in Technology
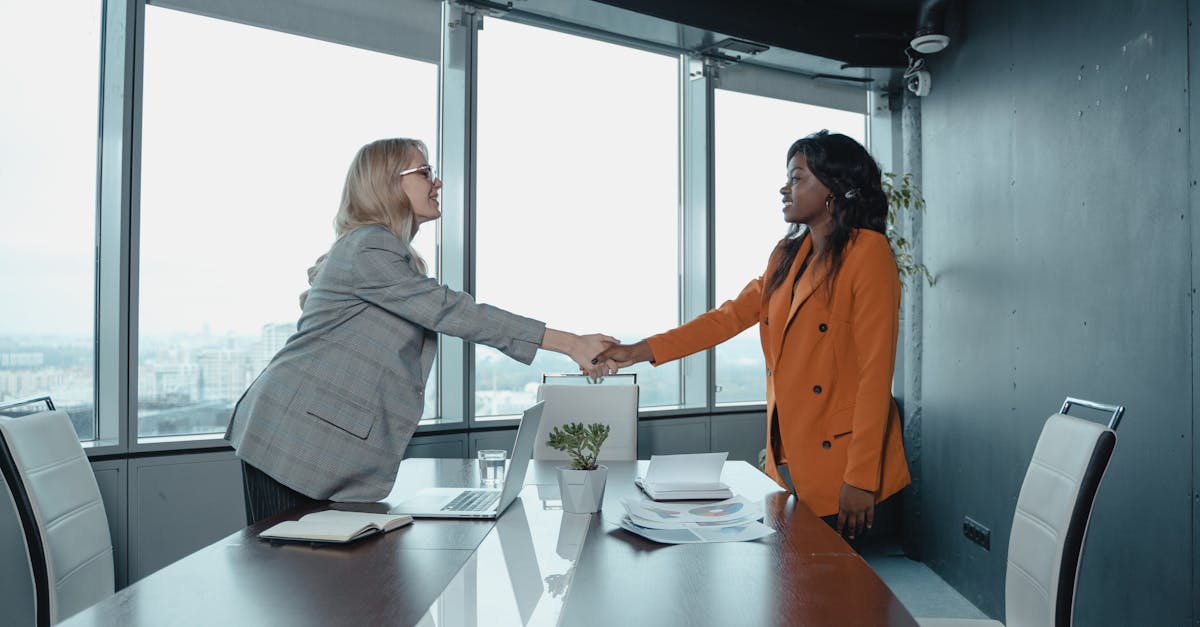
Introduction
In the field of Structural Biology, the study of proteins plays a crucial role in understanding their functions and interactions within living systems. Protein data analysis involves the interpretation of experimental data to obtain meaningful insights into the structure and function of proteins. With the advancements in Artificial Intelligence (AI) and Natural Language Processing (NLP), tools like ChatGPT have emerged as valuable resources to assist in the analysis of protein data.
Structural Biology and Protein Data Analysis
Structural Biology focuses on elucidating the three-dimensional structures of biological macromolecules, particularly proteins. Experimental techniques such as X-ray crystallography, NMR spectroscopy, and cryo-electron microscopy produce vast amounts of protein structural data. Analyzing this data allows researchers to understand the principles that govern protein folding, stability, dynamics, and interactions.
Protein data analysis involves several steps, including data preprocessing, structure determination, validation, and interpretation. These steps require expertise in computational biology, bioinformatics, and biophysics. ChatGPT can assist researchers and scientists by providing a user-friendly interface to interactively examine and explore their protein structure investigation results.
Usage of ChatGPT in Protein Data Analysis
ChatGPT can be utilized in various ways to aid protein data analysis:
- Data Interpretation: ChatGPT can assist in interpreting complex structural data by answering questions related to protein conformation, secondary structure elements, ligand interactions, and more. Researchers can interactively query ChatGPT to obtain valuable insights into their experimental results.
- Comparative Analysis: ChatGPT can compare protein structures and provide detailed comparisons of their similarities and differences. This allows researchers to identify conserved regions, structural motifs, and evolutionary relationships among related proteins.
- Structure Visualization: ChatGPT can generate detailed textual descriptions of protein structures, allowing researchers to visualize their findings in the absence of actual images or videos. These descriptions can help communicate structural features and observations effectively.
- Functional Annotations: ChatGPT can assist in assigning functional annotations to proteins by analyzing their structures, sequence motifs, and known databases. This automated approach can speed up the annotation process, enabling researchers to focus on other aspects of their investigation.
- Data Mining and Analysis: ChatGPT can integrate with other bioinformatics tools and databases to perform advanced mining and analysis of protein data. This allows researchers to extract valuable information from large datasets and discover patterns, trends, and correlations in protein structure and function.
By leveraging the capabilities of ChatGPT in protein data analysis, researchers can efficiently explore and interpret their experimental results, saving time and effort while uncovering valuable insights in the field of Structural Biology.
Conclusion
The application of AI and NLP in Structural Biology has opened up new opportunities for streamlining protein data analysis. ChatGPT, with its conversational interface and advanced language understanding capabilities, can assist in interpreting data generated from protein structure investigations. Its usage ranges from data interpretation and comparative analysis to structure visualization and functional annotations. By harnessing the power of ChatGPT, researchers can enhance the efficiency and effectiveness of their protein data analysis workflows, driving advancements in the field of Structural Biology.
Comments:
Thank you all for reading my article! I'm excited to discuss the potential of ChatGPT in revolutionizing structural biology.
This is such an interesting topic! I can definitely see the value of using AI like ChatGPT in structural biology research.
Absolutely! The ability of ChatGPT to generate hypotheses and explore protein structures is mind-blowing.
I wonder if ChatGPT can help identify new druggable targets within protein structures.
Great point, Karen! ChatGPT could potentially assist in drug discovery by predicting binding sites and analyzing protein-ligand interactions.
While ChatGPT is impressive, I wonder about the reliability of its predictions in such a complex field like structural biology.
You raise a valid concern, Benjamin. The reliability and accuracy of ChatGPT's predictions are areas that need further exploration and validation in structural biology applications.
The potential ethical implications of using AI like ChatGPT in structural biology also need to be addressed.
Absolutely, Samantha! Ensuring ethical considerations in AI-driven research is crucial, especially in fields where human lives can be impacted.
I'm curious about the computational power required to run ChatGPT for structural biology tasks.
Good question, Sophia! The computational requirements can be significant, but advancements in hardware and optimization techniques can help make it more feasible.
I'm amazed by how quickly AI is advancing in various fields. ChatGPT's potential in structural biology is just the tip of the iceberg.
You're absolutely right, Ryan! The rapid progress of AI is opening up new possibilities across many domains, including structural biology.
I hope the scientific community embraces AI as a powerful tool in structural biology research.
I share the same hope, Olivia! Collaborative efforts will be crucial in leveraging AI effectively for groundbreaking discoveries in structural biology.
I think it would be interesting to compare the performance of ChatGPT with other state-of-the-art tools in structural biology.
Agreed, Amy! Comparative studies can provide valuable insights into the strengths and limitations of various tools, including ChatGPT.
What's the current state of commercial tools that utilize AI for structural biology purposes?
Commercial tools in this space are emerging, David, but the field is still relatively new. ChatGPT represents a step towards democratizing AI in structural biology, making it more accessible to researchers.
I'm impressed with the potential of AI in structural biology, but we must establish guidelines and standards for its responsible use.
Absolutely, Michael! Ensuring responsible AI practices, such as transparency, bias mitigation, and reproducibility, is vital for the adoption and acceptance of AI in structural biology.
I wonder if ChatGPT can help in protein folding prediction, which is a challenging problem in structural biology. Any thoughts?
Great question, Emma! Protein folding prediction is indeed a challenging problem, and while ChatGPT may not directly solve it, it can aid in exploring potential conformations and guiding experimental design.
Are there any limitations or disadvantages to using AI models like ChatGPT for structural biology research?
Certainly, Sophie! One limitation is the need for large and diverse training datasets to ensure generalizability. Additionally, biases in the training data can impact the predictions. Ongoing research aims to mitigate these challenges.
Can ChatGPT be used to analyze the dynamics of protein structures?
That's an intriguing idea, Nathan! While ChatGPT may not directly analyze protein dynamics, it can generate hypotheses and assist in designing experiments to study dynamics.
What are some potential future applications of ChatGPT in the field of structural biology?
Great question, Oliver! ChatGPT's capabilities can be expanded to assist in de novo design of proteins, understanding protein-protein interactions, and even drug repurposing.
What are the key challenges in deploying ChatGPT for structural biology in real-world settings?
Good question, Rachel! Some challenges include optimizing computational resources, addressing interpretability concerns, and integrating ChatGPT with existing experimental techniques.
Is there a possibility of integrating ChatGPT with other computational tools commonly used in structural biology?
Absolutely, Jessica! Integrating ChatGPT with existing tools, such as molecular docking and molecular dynamics simulations, can enhance the capabilities and utility in structural biology research.
That would be fantastic! A seamless integration of ChatGPT and other tools could revolutionize how we approach structural biology problems.
I appreciate all your questions and insights! It's inspiring to see the enthusiasm and curiosity around the potential of ChatGPT in advancing structural biology research.
Unfortunately, I won't be able to respond to new comments at the moment. Feel free to continue the discussion among yourselves. Thank you again!
Thank you all for reading this article on the potential of ChatGPT in structural biology. I'm excited to hear your thoughts and engage in insightful discussions!
This is a fascinating article! The advancements in structural biology are remarkable, and it's intriguing to explore how ChatGPT can contribute to this field. Can you provide more details about how ChatGPT can revolutionize structural biology?
Absolutely, Sarah! ChatGPT has the potential to transform structural biology by aiding in protein folding predictions, modeling complex molecular interactions, and accelerating drug discovery processes. It can analyze vast amounts of data and provide valuable insights, allowing researchers to efficiently narrow down potential solutions and make breakthrough discoveries.
I'm skeptical about the accuracy of AI-generated models in biology. How reliable is ChatGPT in predicting protein structures compared to traditional methods?
That's a valid concern, Mark. While ChatGPT alone may not yet match the accuracy of traditional methods, it has the ability to complement and enhance existing techniques. By leveraging its vast knowledge and pattern recognition capabilities, it can offer valuable insights and predictions that can assist researchers in accelerating their work. It can act as a powerful tool for hypothesis generation and aiding in the analysis of complex biological systems.
I'm excited about the potential of ChatGPT in structural biology! However, what measures are in place to ensure ethical use and prevent biases in its analysis and predictions?
Great question, Emily! OpenAI is actively working on addressing these concerns. They are committed to transparency and are putting efforts into reducing biases and allowing external audits. With community involvement, they aim to ensure the responsible and ethical use of AI technology in all fields, including structural biology.
I can see how ChatGPT can greatly aid researchers, but won't it also reduce the need for human experts in the long run?
An excellent point, Alexandra! While ChatGPT can automate certain tasks and provide valuable insights, human expertise remains vital in the field of structural biology. ChatGPT should be seen as a collaborative tool that empowers researchers and allows them to focus on more complex challenges. It can assist in data analysis and hypothesis generation, ultimately augmenting human capabilities.
I'm curious about the limitations of ChatGPT. What are the challenges it faces when applied to structural biology research?
Great question, Thomas! ChatGPT still faces challenges in accurately predicting complex protein structures due to the vast conformational space and intricacies involved. It heavily relies on the data it has been trained on and can struggle to generalize beyond that. However, ongoing research and fine-tuning of the model can help address these limitations and improve its performance in the specific domain of structural biology.
Could ChatGPT be applied to other areas of biological research or is it specifically tailored to structural biology?
Good question, Matt! While ChatGPT shows promise in structural biology, its application can extend to various areas of biological research. From gene expression analysis to drug discovery and personalized medicine, ChatGPT's ability to analyze complex data and provide valuable insights can potentially revolutionize multiple domains of biology.
It's impressive to see how AI technology can contribute to the advancement of scientific research. I'm excited to witness the potential impact of ChatGPT in structural biology!
Indeed, Olivia! The integration of AI technology like ChatGPT has the potential to unlock new discoveries and accelerate scientific progress in the field of structural biology. It's an exciting time for research and innovation!
I have a concern regarding potential biases in the data used to train ChatGPT. Could that impact the reliability and accuracy of its predictions?
Valid point, Michael. Biases in training data can impact AI systems. OpenAI is actively working on addressing this issue, encouraging transparency, and reducing biases. They are also taking steps to allow external audits and soliciting public input to ensure fair and ethical deployment of AI in all fields, including structural biology.
Great article, Daniel! I am fascinated by the potential of ChatGPT in structural biology. It can truly revolutionize the field.
I agree, Michael. The ability of ChatGPT to generate accurate models and predictions based on complex data is quite remarkable.
Absolutely, Sophia! The advancements in artificial intelligence and natural language processing have opened up new possibilities for research in structural biology.
I'm impressed with the potential of ChatGPT in structural biology. However, could you explain any limitations or risks associated with its implementation?
Certainly, Emma! While ChatGPT can provide valuable insights, it's essential to be mindful of its limitations. It may encounter challenges in complex scenarios with limited data, where its predictions may be less accurate. Additionally, ethical considerations should be taken into account to ensure responsible use and prevent potential biases. Collaborative research and careful validation of its findings remain crucial.
Agreed, Daniel. Collaborations foster innovation and can lead to groundbreaking discoveries at the intersection of artificial intelligence and structural biology.
I find it interesting how AI technology continues to advance in various fields. Do you believe ChatGPT can eventually contribute to personalized medicine?
Absolutely, Sophia! Personalized medicine heavily relies on understanding individual variations in genes and proteins. ChatGPT's ability to analyze vast amounts of genomics and healthcare data can contribute to tailoring treatment plans and predicting patient outcomes. It can facilitate more precise and effective personalized medicine approaches, benefiting patients in the long run.
What are the key factors that make ChatGPT a powerful tool for structural biology, and how will the model continue to improve?
Great question, David! ChatGPT's power lies in its ability to process and analyze vast amounts of data, aiding researchers in hypothesis generation and analysis. Through continued research, model fine-tuning, and the integration of additional domain-specific data, ChatGPT will only get better at assisting in structural biology. It's an exciting time as we witness the evolution of AI technology intersecting with scientific research.
It's fascinating to think about the potential impact of AI in advancing structural biology. Is there any ongoing research or collaboration between researchers and AI developers to further enhance this technology?
Absolutely, Claire! There is active collaboration between researchers, scientists, and AI developers to explore the full potential and address the challenges of integrating AI in structural biology research. This collaborative approach ensures that AI technology, such as ChatGPT, is aligned with the needs and requirements of researchers, making it a valuable asset in advancing the field.
Thank you, Daniel, for the detailed response! The potential applications of ChatGPT in structural biology are indeed impressive, and I'm excited to see how it develops in the future.
Thank you for addressing my concerns, Daniel. It's clear that ChatGPT can complement existing methods and provide valuable insights in structural biology research.
Thank you for the explanation, Daniel! It's reassuring to know that OpenAI is actively working towards responsible and unbiased use of AI technology in structural biology.
I appreciate your response, Daniel. ChatGPT's collaborative nature makes it more of a valuable tool than a replacement for human experts in structural biology research.
Thank you for clarifying, Daniel. It's important to be aware of the limitations while utilizing ChatGPT as a supportive tool in structural biology research.
That's great to know, Daniel! ChatGPT's potential in various biological research areas opens up exciting possibilities for future advancements.
Thank you for addressing my concern, Daniel. It's reassuring to see efforts being made to ensure the reliability and fairness of the AI system in structural biology.
I appreciate your response, Daniel. It's crucial to consider the limitations and risks associated with implementing ChatGPT in structural biology, ensuring responsible utilization.
Thank you for clarifying, Daniel. The potential impact of ChatGPT in personalized medicine is exciting, considering the ability to tailor treatments based on individual variations.
Thank you for the insightful response, Daniel. It's interesting to envision the advancements and improvements that lie ahead for ChatGPT in structural biology research.
Thank you for the comprehensive answer, Daniel! The ongoing collaboration between researchers and AI developers showcases the potential for further enhancements in this technology.
This article has given me a different perspective on the potential of AI in structural biology research. I'm excited to see how ChatGPT can contribute further!
The advancements in AI are truly remarkable. It's amazing to witness how ChatGPT can bring new insights to the field of structural biology.
ChatGPT has the potential to revolutionize research processes, and it's fascinating to explore its applications in structural biology.
As someone working in structural biology, I can see the profound impact that ChatGPT could have on our research methodologies. Exciting times ahead!
AI technology, when responsibly integrated into scientific fields like structural biology, can lead to groundbreaking discoveries. ChatGPT shows great promise!
The intersection of AI and biology is a captivating field to observe. ChatGPT's potential in structural biology is just the beginning!
ChatGPT's ability to analyze complex data in structural biology research opens up new possibilities and accelerates our understanding of biological systems.
ChatGPT's assistance in structural biology research may unlock novel insights into complex molecular interactions, ultimately transforming our understanding of biological processes.
I'm excited about the role ChatGPT can play in accelerating drug discovery processes in structural biology. It has the potential to revolutionize the field!
ChatGPT's ability to process vast amounts of data can assist researchers in efficiently navigating the complex landscape of structural biology, leading to more efficient discoveries.
The combination of AI and structural biology is a fascinating development. ChatGPT's insights could propel our understanding of complex molecular structures to new heights.
The potential of ChatGPT in predicting protein structures and modeling molecular interactions is incredible. It's an exciting time for structural biology research!
The advancements in AI continue to amaze me. ChatGPT's potential in structural biology brings hope for groundbreaking discoveries in the future.
As a researcher in the field, I'm excited about the opportunities that ChatGPT can bring to structural biology. Collaboration between AI and scientists is key!
ChatGPT's data analysis capabilities can greatly assist researchers in structural biology, facilitating the understanding of complex biological systems at a faster pace.
The integration of AI technology like ChatGPT can help overcome the challenges faced in structural biology. Exciting times lie ahead for the field.
ChatGPT's potential in structural biology research showcases the powerful amalgamation of AI and scientific fields, paving the way for new discoveries.
AI technology's ability to complement human expertise in structural biology research highlights its potential as a collaborative tool rather than a replacement.
The advancements in AI are revolutionizing numerous fields, and ChatGPT's potential in structural biology is incredibly exciting. Collaboration is key to its success.
ChatGPT's potential in personalized medicine is remarkable. It can contribute immensely to tailoring treatment plans and improving patient outcomes.
As an AI enthusiast, I'm thrilled to see AI technology making strides in fields like structural biology. ChatGPT's capabilities are awe-inspiring!
ChatGPT's integration into structural biology research has the potential to unlock new discoveries, accelerate breakthroughs, and improve our understanding of biological systems.
The collaboration between researchers and AI developers is key to maximize ChatGPT's potential in structural biology. It's exciting to envision the possibilities!
The insights provided by ChatGPT can enhance the efficiency and effectiveness of structural biology research, fostering advancements earlier deemed improbable.
I'm amazed at the possibilities ChatGPT presents for researchers in structural biology. It's a great example of how AI can augment human abilities.
The integration of AI technology like ChatGPT in structural biology research opens up new dimensions in understanding complex biological systems. Exciting times!
ChatGPT's ability to process vast amounts of data can aid researchers in structural biology, empowering them to navigate complex challenges more efficiently.
The potential impact of ChatGPT in structural biology research is substantial. It has the capacity to transform the way we approach discovery and analysis.
Collaboration between researchers and AI developers will be instrumental in harnessing ChatGPT's potential to revolutionize structural biology research.
ChatGPT's ability to assist in hypothesis generation and analysis can be a game-changer for researchers in the field of structural biology. Exciting times ahead!
The integration of AI technologies like ChatGPT has the potential to address the complex challenges faced in structural biology research, leading to accelerated discoveries.
The research community's collaboration with AI developers is necessary to shape the future of ChatGPT in structural biology. It's an opportunity for groundbreaking advancements!
ChatGPT's ability to handle large datasets and provide valuable insights makes it a powerful tool for structural biology research. Collaboration will undoubtedly drive progress.
The potential of ChatGPT in predicting protein folding and understanding molecular interactions is immense. It's exciting to witness the intersection of AI and structural biology.
The collaboration between researchers and AI developers will be crucial in refining ChatGPT to overcome limitations and maximize its impact in structural biology.
ChatGPT's potential in structural biology is astounding. The integration of AI technology has the capability to revolutionize the field and unlock new discoveries.
As AI technology continues to evolve, the partnership between researchers and AI developers can further enhance ChatGPT's applicability and benefits in structural biology.
ChatGPT's potential to accelerate drug discovery processes is particularly exciting. It can help researchers identify potential solutions more efficiently, leading to new treatments.
AI technology's collaborative role in structural biology research showcases how it can amplify human efforts, leading to new horizons of scientific acceptance and discoveries.
The potential of ChatGPT in structural biology research lies not in replacing human experts but in empowering them with new tools and insights. Collaboration leads to progress!
The collaboration between AI and structural biology researchers sets the stage for innovative discoveries and unprecedented insights. ChatGPT plays an invaluable role!
AI's potential in structural biology is inspiring. By enabling data analysis and hypothesis generation at an unprecedented scale, ChatGPT accelerates scientific progress.
As an AI enthusiast, I'm excited about ChatGPT's potential in structural biology research. It offers a promising path towards scientific advancements and breakthroughs.
Thank you all for reading my article on revolutionizing structural biology using ChatGPT in technology! I'm excited to hear your thoughts and engage in a discussion.
I'm curious, Daniel, what are some specific applications where ChatGPT can be used in structural biology?
Great question, Lily! ChatGPT can be utilized in protein folding simulations, ligand discovery, and analyzing complex molecular structures.
While the potential is exciting, we should also be cautious about relying solely on AI models. They are not perfect and may have limitations.
You make a valid point, Oliver. Human expertise and validation will always remain crucial in structural biology research.
The potential of ChatGPT in drug discovery seems promising. It can significantly speed up the process of identifying potential candidates.
I completely agree, Ryan. ChatGPT could help in identifying novel drug targets and designing more effective treatment strategies.
What are the challenges in implementing ChatGPT in structural biology research? Are there any limitations we should be aware of?
Good question, David. One challenge is the availability of diverse and high-quality data to train ChatGPT models specific to structural biology.
Another challenge is interpretability. Complex AI models can be difficult to interpret, so it's important to ensure the reliability of the generated results.
We should also consider potential biases in the data used to train ChatGPT models to avoid any misleading or inaccurate outcomes.
Do you think ChatGPT can be used in collaborative research projects, where multiple researchers contribute their expertise?
Absolutely, Sophie! ChatGPT can enhance collaboration by assisting researchers in exchanging ideas, sharing insights, and accelerating the research process.
That's an interesting point, Sophie. ChatGPT can act as a virtual collaborator, providing valuable suggestions and aiding in decision-making.
However, it's important to maintain the human touch in collaborative research. Face-to-face interactions and diverse perspectives are essential.
What are the ethical considerations surrounding the use of ChatGPT in structural biology?
Good question, Emily. We need to ensure responsible use, protect data privacy, and mitigate potential biases in AI-generated insights.
Also, the transparency of the models and the algorithms used should be addressed to maintain trust and credibility in the research community.
Are there any real-world examples where ChatGPT has already shown promising results in structural biology?
Yes, James! ChatGPT has been used to predict protein structures, analyze protein-ligand binding affinities, and aid in drug discovery processes.
That's exciting! It would be interesting to see how ChatGPT can contribute to personalized medicine and targeted therapies.
Definitely, John! By utilizing patient-specific data, ChatGPT can assist in tailoring treatments and optimizing drug delivery systems.
I wonder if there are any ongoing trials or projects that are utilizing ChatGPT in structural biology research?
Good question, Natalie. There are several research groups and organizations exploring the application of ChatGPT in structural biology, but I'm not aware of any specific ongoing trials.
Thank you for the response, Daniel. I'll keep an eye out for any updates regarding the use of ChatGPT in trials.
Can ChatGPT be utilized in fields beyond structural biology, such as materials science or nanotechnology?
Absolutely, Sophie! ChatGPT's capability to handle complex data and generate insights can be applied in various scientific disciplines to aid in research and development.
How can researchers without an AI background benefit from utilizing ChatGPT in their work?
Good question, David. ChatGPT's user-friendly interface and natural language processing abilities make it accessible to researchers without extensive AI knowledge.
Indeed, Sophia. Researchers can leverage ChatGPT to gain insights and generate hypotheses, leading to new avenues of exploration in their respective fields.
It can act as a valuable tool for researchers to seek guidance and recommendations, even if they don't possess advanced AI skills.
What are your thoughts on potential collaborations between AI experts and structural biologists to further utilize ChatGPT's potential?
Collaboration is key, Lily. By combining AI expertise and domain knowledge in structural biology, we can unlock the full potential of ChatGPT.
Collaborative efforts can also help in addressing the challenges and limitations associated with implementing AI models in structural biology research.
Absolutely, James. It's important to combine the strengths of different fields to tackle complex problems effectively.
Overall, I'm excited about the potential of ChatGPT in structural biology. It will be interesting to witness its adoption and contribution in the field.