Revolutionizing Survey Analysis with Text Mining: Harnessing ChatGPT for Unparalleled Insights
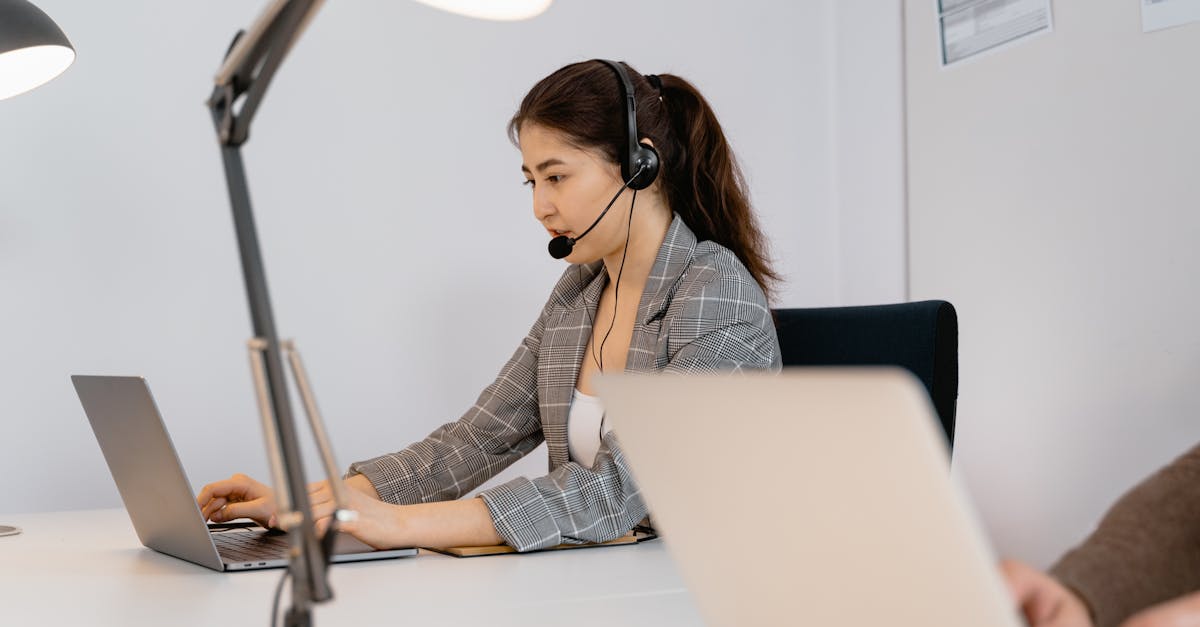
Every day, terabytes of data are generated from various sources, including articles, blogs, social media posts, customer reviews, and surveys. Traditional data analysis methods are insufficient when dealing with such large volumes of unstructured data. Here is where Text Mining comes into the picture. It is a technology designed to handle and analyse big data. More specifically, Text Mining can be applied to the area of survey analysis to extract meaningful information.
This article briefly discusses how Text Mining is employed in the analysis of survey data, particularly, the role of the promising AI model, ChatGPT-4, in analysing open-response survey data, to categorise responses and trace patterns.
What is Text Mining?
Text mining, also known as text analytics, is the process of deriving high-quality information from text by devising patterns and trends such as statistical pattern learning. Text mining involves complex processes including text categorization, text clustering, concept/entity extraction, production of granular taxonomies, sentiment analysis, document summarization, and entity relation modeling (i.e., learning relations between named entities).
Survey Analysis
Surveys are a common tool for gathering information and gauging public opinion. They can provide invaluable data and insights in various research areas. It is key to thoroughly analyse these surveys for a complete understanding of the feedback received. Text mining can be very effective in analysing this qualitative data and providing significant insights.
Usage of Text Mining in Survey Analysis with ChatGPT-4
The most recent version of OpenAI's Generative Pre-training Transformer, ChatGPT-4, represents a breakthrough in the application of Text Mining in survey analysis. The model can analyze open-response survey data, categorize responses, and identify patterns.
ChatGPT-4 uses machine learning to analyze and categorize survey responses. This advanced AI model has been trained on a diverse range of internet text, but it can also be fine-tuned with human feedback for specific tasks, like analyzing survey data.
First, ChatGPT-4 reads the open-ended responses in the survey data. Each response is then encoded as an array of numbers, or a 'vector', which represents the response in the AI model's 'thought space'. These vectors are then used to classify the responses into different categories, based on patterns identified in the training data.
For instance, in a customer satisfaction survey regarding a product, responses could be categorized as comments on product quality, customer service, pricing, etc. These categorizations make it easier to identify general trends in the data, such as widespread dissatisfaction with customer service, or appreciation for product quality.
The ChatGPT-4 model can also identify patterns in the responses. For example, it may notice that many negative responses contain phrases like 'too expensive', 'poor customer service’, or 'low quality'. These patterns provide a more nuanced understanding of the response categories, and can inform strategies for improvement. Furthermore, sub-patterns within categories can also be identified, like specific issues within the broader category of 'Customer Service'.
Thus, using text mining and AI for survey analysis can make the process far more efficient and revealing. The use of the ChatGPT-4 model accelerates the otherwise time consuming manual process of sifting through each response individually, and offers more nuanced and intricate analysis and understanding of the response data.
This article has only scratched the surface of the potential applications of text mining and AI in survey analysis. With further research and development, these tools can undoubtedly provide even more valuable insights into public opinion and consumer habits.
Comments:
Great article, Samuel! Text mining is truly revolutionizing survey analysis. The insights gained from analyzing text data have the potential to uncover valuable information that traditional analysis methods may miss.
I agree, Mark. The use of chatbots powered by GPT has opened up new possibilities for survey analysis. It allows for a more natural and interactive experience for respondents.
Text mining is definitely a game-changer in survey analysis. It can help identify patterns, sentiment, and even detect emerging trends. It's exciting to see how far technology has come!
I'm curious, Samuel, how does chatGPT handle unstructured responses with grammatical errors or slang? Does it affect the accuracy of the analysis?
Good question, Sophia. ChatGPT is designed to understand and generate human-like text, including handling grammatical errors and slang to some extent. However, the accuracy of the analysis can be affected if the responses contain excessive errors or are incoherent.
Samuel, I see the potential of using chatGPT for survey analysis, but what about biases in the training data? Can it introduce biases into the analysis?
Valid concern, Michael. Bias in training data can impact the analysis. It's crucial to carefully curate and review the training data to minimize biases as much as possible. Continual monitoring and refinement of the model's performance is also necessary to address any biases that may arise.
Samuel, I love the idea of leveraging GPT for survey analysis, but what are the potential ethical considerations associated with it?
Great question, Natalie. Ethical considerations play a significant role in adopting AI for survey analysis. Privacy concerns, informed consent, and data security should be prioritized. Transparency in how the model works and its limitations is also important to ensure ethical usage.
This is a fascinating article, Samuel. I wonder, how does the accuracy of chatGPT compare to traditional survey analysis methods?
Thank you, Robert. ChatGPT is a powerful tool, but its accuracy may vary depending on the specific use case and data quality. Traditional survey analysis methods have their strengths too. It's always beneficial to consider a combination of approaches for comprehensive insights.
Samuel, what are the potential limitations of using chatGPT for survey analysis?
Good question, Jennifer. ChatGPT has some limitations. It may generate plausible-sounding but incorrect or nonsensical responses. It can also be sensitive to input phrasing, producing different results for slightly rephrased questions. These limitations highlight the need for careful evaluation and refinement to ensure accurate analysis.
I'm excited about the potential of text mining and chatGPT for survey analysis, but what are the computational requirements for implementing this approach?
Great enthusiasm, Angela! Implementing text mining and chatGPT for survey analysis does require computational resources. The exact requirements depend on factors such as the size of the dataset and complexity of analysis. Adequate computational power and efficient infrastructure are necessary for smooth implementation.
The limitations you mentioned, Samuel, highlight the importance of human supervision and careful evaluation when leveraging chatGPT for survey analysis.
That's a valid point, Rachel. Human involvement is essential for quality control and ensuring accurate analysis.
Samuel, how does chatGPT handle different languages in survey responses? Is multilingual analysis possible?
Good question, William. ChatGPT can handle multiple languages, but its performance depends on the quality and amount of training data available for each language. Multilingual analysis is possible but may require additional customization and careful evaluation.
That's interesting, Samuel. With multilingual capabilities, chatGPT can extend its analysis to a broader range of surveys and offer more diverse insights.
I can see how chatGPT can complement traditional survey analysis methods. It can provide additional insights and help discover hidden patterns. Exciting times!
Absolutely, Lisa! Embracing new techniques like text mining and chatGPT can enhance the entire survey analysis process. It's a step towards more comprehensive understanding.
Indeed, Benjamin. By embracing innovative techniques, we can unlock new levels of understanding and obtain more valuable insights from surveys.
I've used chatGPT for survey analysis, and I must say it's been impressive. It handles unstructured responses quite well, even with grammatical errors or slang.
Addressing biases in training data is crucial to ensure fair analysis. Additionally, actively seeking diverse perspectives during model development can help reduce biases.
Ethical considerations should also include responsible data usage and preventing the misuse of AI-generated insights.
When it comes to computational requirements, cloud platforms can provide scalable resources for implementing text mining and chatGPT.
That's true, Daniel. Cloud-based solutions offer flexibility and enable organizations to utilize the necessary resources without significant infrastructure investments.
While chatGPT opens up possibilities, it's essential to consider potential biases in the language model itself. Ongoing evaluation and improvement are crucial.
Continual monitoring and refinement of the model's performance is vital not only for addressing biases but also for optimizing the analysis accuracy.
Combining approaches seems like the way to go. Traditional analysis methods and chatGPT can complement each other and provide more comprehensive insights.
Careful evaluation of chatGPT-generated responses is necessary to ensure they align with the actual meaning intended by the respondents.
The combination of human expertise and chatGPT's capabilities can lead to more accurate and reliable survey analysis outcomes.
Ethical considerations go hand in hand with AI adoption. Organizations should prioritize user privacy, consent, and responsible data handling.
Human supervision also enables monitoring and mitigating potential biases that may arise during chatGPT-powered survey analysis.
Adequate computational resources and infrastructure are essential to avoid processing bottlenecks and ensure a smooth survey analysis workflow.
Cloud-based solutions provide scalability, enabling organizations to scale up or down based on their analysis requirements without investing heavily in infrastructure.
Text mining has the potential to uncover patterns and trends that may not be apparent through traditional survey analysis. It adds a valuable dimension.
Combining traditional and innovative survey analysis methods can help organizations gain a more comprehensive understanding of their respondents.
The interactive nature of chatbots powered by GPT can help gather more accurate and detailed responses from survey participants. It's a win-win!
Transparency is crucial in gaining user trust and ensuring ethical AI usage. Users should have a clear understanding of how their data is being processed and analyzed.
Addressing biases in AI models and training data is an ongoing process. Regular audits and updates are necessary to maintain fairness in the analysis.
Human involvement adds a layer of quality control to ensure that the analysis outcomes are reliable and accurately represent the survey responses.
Evaluating the generated responses against ground truth or involving experts for validation can help identify potential limitations and refine the model's accuracy.
Cloud-based solutions also offer scalability, allowing organizations to adapt their computational resources based on the survey analysis workload.
Considering the strengths and weaknesses of both chatGPT and traditional methods, a combined approach can provide more robust and reliable insights.
I completely agree, Matthew. Incorporating diverse analysis methods empowers organizations to make more confident data-driven decisions.
Efficient computational resources also contribute to faster analysis, allowing organizations to derive insights more quickly and drive timely actions.
Continual evaluation and improvement are fundamental in keeping up with evolving biases and ensuring fair and accurate analysis outcomes.
Organizations should take a proactive stance in developing and enforcing ethical guidelines for AI-powered survey analysis to maintain user trust.