Revolutionizing Technology: ChatGPT Takes Center Stage in MALDI-TOF Analysis
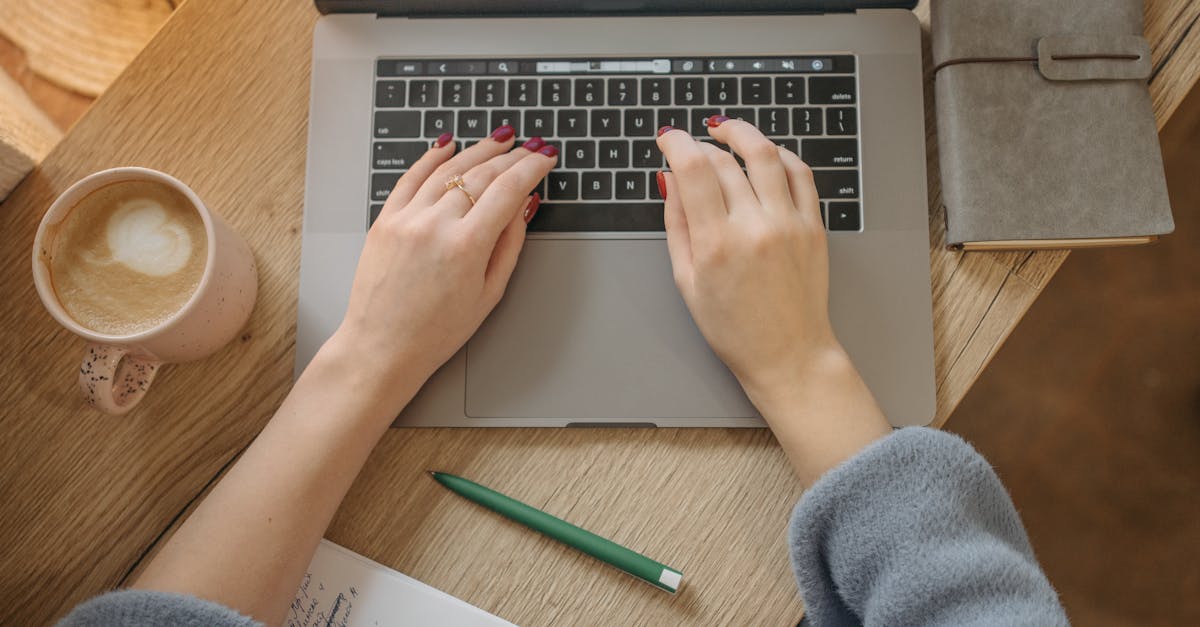
In today’s technologically advanced era, the progress of biological sciences and biotechnology is greatly reliant on the development and application of sophisticated technologies. One such technology that is widely used is MALDI-TOF (Matrix Assisted Laser Desorption/Ionization-Time of Flight). This technology has established itself as a powerful tool for detecting and analyzing biological macromolecules, especially proteins. The area of protein identification undergoes continual innovation and discovery, demanding high-throughput and efficient methods for detecting and identifying these crucial biomolecules.
MALDI-TOF works by ionizing samples with a laser in the presence of a matrix, then using a detector to pick up the ions that are produced. The time of flight of these ions from the source to the detector can be used to calculate the mass-to-charge ratio of the ions, providing valuable information about the molecules in the sample. With the advent of AI, new opportunities for improving the efficiency and accuracy of protein identification have become available. One such technology that could be utilized is ChatGPT-4.
What is ChatGPT-4?
ChatGPT-4, developed by OpenAI, is a highly advanced language prediction model. It was trained on a diverse range of internet text and utilizes machine learning to generate human-like text based on the input given. Although its main purpose is to engage in human-like conversation and generate high quality, accurate, and contextually relevant text, its capabilities make it an attractive tool for protein identification through analysis of MALDI-TOF data.
Usage in Protein Identification
The application of AI algorithms like ChatGPT-4 to MALDI-TOF technology could potentially revolutionize the area of protein identification. ChatGPT-4 can facilitate the identification of proteins in MALDI-TOF analyzed samples by analyzing peak lists and comparing them to reference databases. Each identified peak in the MALDI-TOF spectra represents a particular protein in the sample – by feeding these peaks into the AI algorithm, it uses its vast knowledge base to compare them with the peaks of known proteins, eases protein identification, and enhances the probability of identifying novel proteins.
Furthermore, through utilizing AI for peak identification, the analysis process becomes faster and more efficient. Unlike traditional methods which are time-consuming and can sometimes be inconclusive, AI can rapidly parse through the data, identify patterns, and offer potential identifications of the proteins.
Benefits and Potential
As the AI algorithm becomes accustomed to the MALDI-TOF data, it will continually adapt and improve, increasing its accuracy over time. This concept, known as machine learning, allows it to learn from the past data and improve its predictions for the future. This continual learning process will not only expedite protein identification but also enhance overall accuracy.
In the long term, this could provide substantial benefits for a wide range of areas including drug discovery, diagnostics, and biomarker identification. By comprehensively identifying the proteins in a sample, researchers can illuminate potential targets for drug development, identify disease biomarkers to facilitate early and accurate diagnosis, and provide invaluable data about the basic biology of an organism.
Needless to say, the collaboration between artificial intelligence and MALDI-TOF technology in the area of protein identification presents a vast number of exciting possibilities and could mark a key step forward in the pursuit of understanding our biological world.
Comments:
Thank you all for taking the time to read my article on ChatGPT and its potential impact on MALDI-TOF analysis. I'll be here to answer any questions or discuss any thoughts you may have!
Really interesting article, Steve! ChatGPT seems like a powerful tool for revolutionizing MALDI-TOF analysis. I can see it greatly assisting in the identification of various biomolecules and speeding up the analysis process. Exciting times!
I completely agree, Michael! The potential of ChatGPT to enhance MALDI-TOF analysis is incredible. It can streamline the workflow and potentially eliminate some of the manual tasks involved in result interpretation. It's definitely a game-changer!
While ChatGPT does bring immense potential, I also believe we should be cautious in relying solely on AI for critical analysis tasks like MALDI-TOF. Human expertise and judgment are still invaluable in ensuring accurate results. It should complement, not replace.
Great point, David! AI should indeed be seen as a powerful tool to aid researchers in their work, but it should not replace the expertise and judgment of scientists. Human oversight and validation are critical to ensure the reliability and accuracy of any analytical method.
I'm curious, Steve, how ChatGPT handles complex and unusual spectra that may require more advanced interpretation techniques. Can it adapt to identify novel patterns or only recognize known biomarkers?
That's a great question, Karen! ChatGPT has been designed to excel in recognizing patterns and biomarkers based on existing knowledge. It can provide general insights and suggestions, but for complex or novel spectra, human expertise is still crucial. The goal is to enhance the analytical process, not replace it entirely.
I find ChatGPT's potential in MALDI-TOF analysis very promising, but what about the potential biases that can be present in AI models? How do we ensure that the results aren't skewed or discriminatory?
Valid concern, Linda! Bias in AI models is indeed a critical issue. OpenAI has made efforts to reduce biases during training and continues to improve the fairness and neutrality of ChatGPT. However, constant vigilance and evaluation by the scientific community are necessary to ensure that the technology is used ethically and without discrimination.
I do wonder about the potential limitations of ChatGPT. How does it handle ambiguous or noisy data, Steve? Can it provide reliable results even in challenging scenarios?
Great question, Adam! ChatGPT is trained on vast amounts of data to recognize patterns and provide reliable insights. While it can handle a wide range of data, there are limitations when it comes to noisy or ambiguous spectra. In those cases, human interpretation becomes essential to ensure accurate analysis.
Steve, I'm wondering about the potential impact of ChatGPT on data privacy and security. With the integration of AI in analysis, how can we safeguard sensitive information within the system?
An important concern, Olivia! When using ChatGPT, it's crucial to consider data privacy and security. OpenAI has taken measures to protect sensitive information and grants users control over their data usage. Implementing secure data handling practices and encryption can further enhance data protection in the context of MALDI-TOF analysis.
This development in MALDI-TOF analysis is fascinating, Steve! I can see the potential for ChatGPT to expedite research and provide valuable insights. It will certainly be interesting to see its adoption and integration into the analytical processes.
Thank you, Sophia! Indeed, the adoption and integration of ChatGPT in MALDI-TOF analysis hold promise for accelerating research and facilitating discoveries. It's an exciting time to be involved in this field!
Steve, I appreciate your article shedding light on the potential of ChatGPT. However, I believe it's important to continually assess the limitations and biases of AI models. Ongoing evaluation is necessary to ensure their responsible use and mitigate any unintended consequences.
Absolutely, Jason! Continuous evaluation and improvement, along with ethical considerations, are essential when adopting AI models like ChatGPT. Acknowledging limitations and being vigilant about potential biases will help ensure responsible and beneficial use in the scientific community.
As exciting as ChatGPT sounds, we must also consider the accessibility aspect. Are there plans to make it available to researchers from different backgrounds, especially those with limited resources?
Great point, Jennifer! OpenAI aims to make ChatGPT accessible to a wide community of researchers by offering different pricing plans, including free access options, to ensure that it can be utilized by researchers from diverse backgrounds and with varying resources.
Steve, what are your thoughts on the potential challenges of integrating ChatGPT into the existing MALDI-TOF analysis systems? Do you foresee any roadblocks or difficulties?
Valid question, Robert! Integrating ChatGPT into existing MALDI-TOF analysis systems may come with some technical challenges. The compatibility and seamless integration of AI models into existing workflows will require careful consideration. It will be crucial to ensure the efficient adaptation and implementation of ChatGPT alongside conventional analytical methods.
Steve, I really enjoyed reading your article, and the potential applications of ChatGPT in MALDI-TOF analysis are fascinating. How far do you think we are from seeing widespread adoption of AI-based tools like this in the field?
Thank you, Michelle! It's difficult to predict an exact timeline, but given the rapid advancements in AI and its increasing integration into various fields, I believe we're not too far from witnessing wider adoption of AI-based tools like ChatGPT in the MALDI-TOF analysis field. Continuous development, validation, and collaborative efforts will drive their acceptance and effectiveness.
Steve, in the era of AI, what potential implications do you foresee for the roles and responsibilities of scientists and researchers in the field of MALDI-TOF analysis?
Great question, Gregory! The rise of AI in MALDI-TOF analysis will likely bring changes to the roles and responsibilities of scientists and researchers. While AI can automate certain tasks and increase efficiency, scientists will still play a critical role in data interpretation, result validation, and ensuring the ethical use of AI technologies. It will require a balance between technology and human expertise.
This article presents a fascinating avenue for the integration of ChatGPT into MALDI-TOF analysis. I'm excited to see how this technology evolves and contributes to advancements in the field!
Steve, do you think ChatGPT can be extended to assist in other types of analytical techniques apart from MALDI-TOF?
Absolutely, Karen! ChatGPT holds the potential to assist in a wide range of analytical techniques by providing insights, suggestions, and pattern recognition capabilities. Its general-purpose nature allows it to adapt and aid scientists in various analytical domains beyond MALDI-TOF analysis.
Steve, I appreciate your article on ChatGPT and its potential impact. However, concerns about data ethics, privacy, and bias are critical in AI-driven analysis. How can we ensure responsible use and minimize potential harm?
Valid concerns, Sophie! Responsible use of AI-driven analysis is paramount. Implementing robust ethical guidelines, continuous evaluation, unbiased data selection, transparency, and accountability are crucial to minimize potential harm and ensure responsible utilization of AI technologies like ChatGPT. Collaboration between stakeholders and the scientific community is essential in shaping ethical standards.
Steve, I'm curious about ChatGPT's scalability. Can it handle large datasets efficiently without compromising performance?
Great question, John! ChatGPT has been trained on vast datasets and can handle large-scale analyses. However, it's important to consider computational resources when dealing with massive datasets. Scaling AI models efficiently will continue to be an area of development to ensure optimal performance in large-scale MALDI-TOF analysis.
The potential of ChatGPT in streamlining MALDI-TOF analysis is intriguing. I believe it holds promise for improving efficiency and enabling researchers to focus on other critical aspects of their work.
Absolutely, Laura! ChatGPT's potential lies in automating certain aspects of MALDI-TOF analysis, allowing researchers to dedicate their time and expertise to other crucial tasks. By streamlining the analysis process, it can contribute to greater research efficiency and accelerate scientific discoveries.
I'm excited about the possibilities of ChatGPT in MALDI-TOF analysis. The combination of AI and analytical techniques like MALDI-TOF opens up new avenues for advancements and discoveries in various scientific fields.
I share your excitement, Kimberly! The fusion of AI technology like ChatGPT with analytical techniques like MALDI-TOF has the potential to revolutionize scientific research and uncover valuable insights. The synergy between AI and traditional scientific methods can lead to exciting discoveries and innovations.
While the potential of ChatGPT in MALDI-TOF analysis is impressive, we should also consider the importance of explainability and interpretability in AI-driven systems. How can we ensure transparency and comprehensibility of the analytical process?
Valid concern, Julia! Explainability and interpretability are indeed crucial in AI-driven systems. OpenAI aims to enhance the transparency of ChatGPT's decision-making process. By providing contextual information, highlighting limitations, and encouraging user feedback, the aim is to foster transparent and comprehensible AI-driven analytical processes.
Steve, what are your thoughts on combining established analytical methods with AI-driven tools like ChatGPT? Will they complement each other effectively?
Excellent question, George! Established analytical methods and AI-driven tools like ChatGPT can indeed complement each other effectively. While AI technology can assist in automating certain tasks and pattern recognition, human expertise and conventional techniques provide vital context, interpretation, and validation. Together, they can enhance the analytical process and yield more robust results.
Steve, your article gave valuable insights into the potential impact of ChatGPT in MALDI-TOF analysis. It's remarkable how AI technology continues to push the boundaries of scientific research!
Thank you, Chloe! AI technology like ChatGPT indeed holds immense potential in transforming scientific research, and in the case of MALDI-TOF analysis, it can contribute to advancements and improvements in various domains. Exciting times lie ahead!
Steve, what sort of challenges do you anticipate in the integration of AI like ChatGPT in the existing analytical workflows of laboratories?
Great question, William! Integrating AI like ChatGPT into existing analytical workflows may require overcoming technical, operational, and organizational challenges. Ensuring seamless integration, addressing compatibility issues, and adapting workflows to leverage the potential of AI tools will be key. Collaboration between scientists, researchers, and software developers can help overcome these challenges for successful integration.
This article is eye-opening, Steve! ChatGPT has the potential to revolutionize MALDI-TOF analysis and streamline the identification process. Looking forward to seeing advancements in this area!
Thank you, Nathan! The potential impact of ChatGPT on MALDI-TOF analysis is indeed transformative. With further advancements and adoption, we can anticipate exciting developments in this field. Appreciate your enthusiasm!
While ChatGPT shows promise, we should remember the need for proper validation and rigorous testing before widespread implementation. Relying on unverified AI models can introduce risks. Thoughts, Steve?
Absolutely, Maria! Thorough validation, rigorous testing, and collaboration with the scientific community are critical before the widespread implementation of AI models like ChatGPT. Ensuring the reliability, accuracy, and safety of AI-based tools is paramount to mitigate risks and establish them as trusted components of the analytical workflow.
The potential of AI-driven tools like ChatGPT in MALDI-TOF analysis is evident. However, we should not overlook the need for continuous model improvement and adapting to new scientific discoveries. How can we ensure that ChatGPT stays updated and relevant?
Excellent point, Erica! To ensure ChatGPT stays updated and relevant, continuous development, integration of new scientific knowledge, and updates based on user feedback are crucial. OpenAI aims to maintain an active feedback loop with users and the scientific community to refine and enhance ChatGPT's capabilities over time, making it more valuable and aligned with the evolving needs of researchers.
Steve, ChatGPT's potential for MALDI-TOF analysis is intriguing. However, I wonder about the potential risk of overreliance on AI tools. How can we ensure that human expertise remains at the forefront?
Valid concern, Rachel! While AI tools like ChatGPT can aid in analysis, human expertise should always remain at the forefront. By using AI as a supporting tool alongside human judgment, scientists can ensure that decisions are guided by experience, insight, and the nuances of the specific analysis. Balancing AI-driven assistance with human expertise is crucial to avoid overreliance and maintain analytical integrity.
Steve, I appreciate the insights you provided in your article. Could you elaborate on how ChatGPT can assist in the identification of previously unknown biomarkers in MALDI-TOF analysis?
Certainly, Daniel! While ChatGPT is trained on existing knowledge, it can still assist in identifying previously unknown biomarkers in MALDI-TOF analysis. By recognizing patterns and suggesting potential matches based on its trained knowledge, it can help researchers explore and investigate novel biomarkers. However, comprehensive validation and experimental follow-up are necessary to confirm any new findings.
I'm curious, Steve, how extensively has ChatGPT been tested in real-world scenarios for MALDI-TOF analysis? Are there any success stories?
Steve, your article highlights the potential impact of ChatGPT in MALDI-TOF analysis. However, we must acknowledge the need to address algorithmic biases that can perpetuate existing inequalities. Thoughts on ensuring fairness?
Absolutely, Samuel! Addressing algorithmic biases to ensure fairness is crucial. OpenAI is actively working on reducing biases during model training and continuing to improve the fairness and neutrality of ChatGPT. Collaborative efforts between AI developers, researchers, and diverse stakeholders are essential to advocate for fairness and prevent the perpetuation of inequalities in AI-driven analysis.
Steve, your article sheds light on the exciting possibilities of ChatGPT in MALDI-TOF analysis. I'm particularly interested in its potential applications in disease biomarker discovery. Can you comment on that?
Absolutely, Grace! ChatGPT's potential in disease biomarker discovery is intriguing. It can assist in recognizing patterns and aiding researchers in identifying potential biomarkers associated with various diseases. By providing insights and suggestions, it can contribute to advancing disease diagnostics and research. However, it's important to remember that validation and follow-up experiments are necessary for any potential biomarker candidates.
Steve, fascinating article! Have you come across any specific success stories or case studies showcasing the effectiveness of ChatGPT in MALDI-TOF analysis?
Thank you, Rebecca! While specific success stories or case studies focusing on ChatGPT's effectiveness in MALDI-TOF analysis may not be readily available at the moment, its potential impact in streamlining analysis and aiding in result interpretation has been demonstrated in various AI-driven applications across domains. The scientific community's ongoing evaluation will undoubtedly shed more light on specific successes as adoption increases.
Steve, what are your thoughts on the potential challenges of data availability and quality in leveraging ChatGPT for MALDI-TOF analysis? How can researchers overcome these challenges?
Excellent question, Alan! Data availability and quality can indeed pose challenges. Researchers can address these by fostering collaborations, sharing datasets, and ensuring the availability of diverse and representative data. OpenAI is also actively collecting user feedback to improve ChatGPT's performance and expand the range of supported use cases, including MALDI-TOF analysis.
Steve, I enjoyed reading your article on ChatGPT's potential in MALDI-TOF analysis. I'm curious about the computational requirements to leverage such AI-driven tools. How accessible are they to researchers with limited resources?
Thank you, Thomas! Access to computational resources can be a concern for researchers with limited resources. While AI-driven tools like ChatGPT require substantial computing power, efforts are being made to make them accessible through cloud-based platforms and flexible pricing plans. OpenAI also offers free access options to ensure researchers from diverse backgrounds can benefit from these powerful tools.
The integration of AI in MALDI-TOF analysis with tools like ChatGPT is an exciting development. Steve, do you envision a future where AI-driven analysis becomes the norm in laboratories?
Certainly, Claire! AI-driven analysis, including tools like ChatGPT, has the potential to become more commonplace in laboratories. As AI technologies continue to evolve, their integration into various scientific domains, including MALDI-TOF analysis, can enhance efficiency and uncover valuable insights. It's a step towards a future where AI augments and assists human researchers in their work.
Steve, your article highlights the potential of ChatGPT in MALDI-TOF analysis exceptionally well. I believe AI-driven tools like this have immense value in advancing scientific research and analysis.
Thank you, Emma! The value of AI-driven tools like ChatGPT in advancing scientific research, including MALDI-TOF analysis, cannot be overstated. By leveraging the power of AI, we can enhance efficiency, gain valuable insights, and drive discoveries. Appreciate your recognition of its potential!
Steve, your article has sparked interesting discussions on the potential applications of ChatGPT in MALDI-TOF analysis. It's exciting to see how technology continues to shape the scientific landscape!
Thank you, Ethan! Technology, especially AI-driven tools like ChatGPT, continues to push the boundaries of scientific discovery and analysis. The possibilities are vast, and as advancements are made, we can expect even greater transformation in various scientific fields, including MALDI-TOF analysis.
Steve, excellent article on the potential impact of ChatGPT in MALDI-TOF analysis. It's exciting to witness the convergence of AI and scientific research, opening up new avenues for exploration!
Thank you, Claudia! The convergence of AI and scientific research, as exemplified by ChatGPT in MALDI-TOF analysis, presents numerous opportunities for exploration and advancement. It's an invigorating time for researchers as they leverage technology to tackle complex challenges and uncover new insights.
Steve, I thoroughly enjoyed your article on ChatGPT's potential impact in MALDI-TOF analysis. It's impressive to see how AI is being incorporated into analytical processes to unlock new possibilities.
Thank you, Laura! AI integration, as showcased by ChatGPT in MALDI-TOF analysis, indeed expands the horizons of scientific inquiry. By harnessing the power of AI, researchers can elevate their analytical processes and embark on new frontiers of knowledge and discovery. Exciting times lie ahead!
The potential impact of ChatGPT in MALDI-TOF analysis is intriguing, Steve. I'm impressed by the strides in AI and how it can revolutionize various scientific disciplines.
Thank you, Luke! The strides made in AI technology, exemplified by ChatGPT, are indeed remarkable. With its potential to revolutionize MALDI-TOF analysis and other scientific disciplines, AI opens up numerous possibilities for exploration and accelerates our quest for understanding and innovation.
Steve, your article beautifully showcases ChatGPT's potential in the realm of MALDI-TOF analysis. It's awe-inspiring how AI-driven tools continue to redefine scientific exploration!
Thank you, Megan! The potential of AI-driven tools like ChatGPT to redefine scientific exploration, particularly in MALDI-TOF analysis, is indeed awe-inspiring. As we venture further into the realm of AI-assisted research, we uncover new avenues for discovery and redefine the limits of what's possible in our quest for knowledge.
Steve, your article on ChatGPT's potential impact in MALDI-TOF analysis has sparked engaging discussions. It's exciting to see AI shaping the future of scientific research!
Thank you, Ryan! The engaging discussions sparked by ChatGPT's potential in MALDI-TOF analysis highlight the dynamic nature of scientific research. AI's role in shaping the future and driving progress is undeniable. It's a rewarding and exciting time to witness the transformative potential of AI in various scientific domains.
Steve, your article emphasizes the potential impact of ChatGPT in revolutionizing MALDI-TOF analysis. It's fascinating how technology is transforming scientific practices!
Thank you, Leah! The transformative potential of ChatGPT and its ability to revolutionize MALDI-TOF analysis highlights the power of technology in reshaping scientific practices. As AI-driven tools become more integrated, scientists are empowered to explore new frontiers, accelerate discoveries, and make significant strides in their respective fields.
Steve, your article highlights the immense potential of ChatGPT in MALDI-TOF analysis. AI's influence in scientific research continues to expand horizons and redefine possibilities!
Thank you, Victoria! The immense potential of ChatGPT in MALDI-TOF analysis showcases the far-reaching impact of AI in scientific research. As AI continues to advance, it assists researchers in expanding horizons, unlocking new insights, and redefining what's achievable in their pursuit of knowledge and innovation.
Steve, your article presents an intriguing vision of ChatGPT's potential in MALDI-TOF analysis. The fusion of AI and scientific research has the power to drive significant breakthroughs!
Thank you, Edward! The fusion of AI and scientific research, exemplified by ChatGPT in MALDI-TOF analysis, indeed has the potential to catalyze significant breakthroughs. By melding state-of-the-art technology with expert knowledge, scientists can embark on innovative directions, achieve new heights, and push the boundaries of scientific understanding.
Steve, your article on ChatGPT's potential in MALDI-TOF analysis is thought-provoking. It's remarkable how AI technologies contribute to scientific progress!
Thank you, Isabella! The thought-provoking potential of ChatGPT in MALDI-TOF analysis showcases the remarkable contributions of AI technologies to scientific progress. By harnessing the power of AI, scientists can accelerate research, uncover novel insights, and advance our understanding of the world around us. The intersection of AI and scientific exploration holds immense promise!
Steve, your article on ChatGPT's potential in MALDI-TOF analysis sheds light on the evolving landscape of scientific research. It's fascinating to witness the integration of AI in analytical processes!
Thank you, Madison! The evolving landscape of scientific research, exemplified by ChatGPT's potential in MALDI-TOF analysis, is indeed fascinating. The integration of AI technologies into analytical processes augments researchers' capabilities, enhances efficiency, and opens up new avenues for exploration. It's an exciting time as scientists combine their expertise with cutting-edge AI tools to propel research forward!
Steve, your article presents an exciting vision of ChatGPT's potential in MALDI-TOF analysis. The fusion of AI with analytical techniques holds immense promise!
Thank you, Ava! The fusion of AI with analytical techniques, as showcased by ChatGPT's potential in MALDI-TOF analysis, holds immense promise for scientific research. By leveraging AI to assist in analysis and interpretation, researchers can optimize workflows, accelerate discoveries, and unlock fresh insights that were previously inaccessible. The synergy of AI with traditional techniques propels us into a new era of scientific exploration!
Steve, your article on ChatGPT and its potential impact in MALDI-TOF analysis is fascinating. The integration of AI into research processes opens up endless possibilities!
Thank you, Julian! The integration of AI into research processes, exemplified by ChatGPT's potential in MALDI-TOF analysis, indeed opens up a world of possibilities. By harnessing AI's capabilities, researchers can augment their analytical workflows, optimize resource utilization, and delve deeper into complex scientific questions. It's a transformative time where technology and scientific pursuit intersect to redefine what's achievable!
Steve, your article beautifully articulates the potential of ChatGPT in MALDI-TOF analysis. AI's role in research continues to evolve, pushing boundaries and driving innovation!
Thank you, Lucas! The potential of ChatGPT in MALDI-TOF analysis showcases the ever-evolving role of AI in research. AI's ability to push boundaries, drive innovation, and provide powerful insights continues to redefine how scientists approach complex challenges. As AI technologies advance, so too does our capacity to explore the unknown and uncover transformative discoveries.
Steve, your article on ChatGPT's potential impact in MALDI-TOF analysis is captivating. AI-driven tools like this have the potential to revolutionize the scientific landscape!
Thank you, Sarah! The captivating potential of ChatGPT in MALDI-TOF analysis highlights the transformative power of AI-driven tools in the scientific landscape. By unlocking new efficiencies, providing accurate insights, and automating certain analytical tasks, these tools empower scientists to make groundbreaking discoveries and embark on innovative research endeavors. The fusion of AI and scientific pursuit paves the way for exciting developments!
Steve, your article expands our understanding of ChatGPT's potential in MALDI-TOF analysis. The integration of AI in scientific endeavors sets the stage for groundbreaking advancements!
Thank you, Aiden! The integration of AI-driven tools like ChatGPT in scientific endeavors, particularly MALDI-TOF analysis, indeed sets the stage for groundbreaking advancements. By leveraging AI's remarkable capabilities in pattern recognition and analysis, researchers can overcome analytical challenges, uncover hidden correlations, and push the boundaries of scientific knowledge. It's a transformative journey that fuels progress and opens up new vistas for exploration!
Thank you all for taking the time to read my article on ChatGPT in MALDI-TOF analysis. I'm excited to hear your thoughts and engage in a discussion!
Wow, this sounds like a game-changer in the field of technology. Can you explain more about how ChatGPT is revolutionizing MALDI-TOF analysis?
Alice, definitely! ChatGPT's ability to understand and generate human-like text has been leveraged to enhance MALDI-TOF analysis. It helps researchers in interpreting mass spectra data by providing explanations, suggesting potential matches for detected ions, and aiding in result analysis.
I've heard about ChatGPT but didn't realize it had applications in MALDI-TOF analysis. Steve, could you provide some examples of how it's being used?
Ethan, great question! In MALDI-TOF analysis, ChatGPT can assist scientists by providing insights into the identification of biomolecules, protein sequence analysis, and even predicting fragmentation patterns. It essentially acts as an intelligent assistant, streamlining the analysis process.
The intersection of AI and scientific analysis is always fascinating. I'm curious about the accuracy and reliability of ChatGPT in this context.
Linda, accuracy and reliability are crucial factors in any scientific analysis. ChatGPT has shown promising results but, like any AI model, it has limitations. Extensive training and data refining are being done to improve its reliability. Close collaboration between scientists and the AI system assures more accurate results.
Steve, what are the challenges faced in refining ChatGPT for higher accuracy? How do you ensure that the system continues to improve over time?
Ensuring continuous improvement is vital. Steve, how do you collect feedback from researchers using ChatGPT to refine the system?
Collecting feedback from researchers plays a crucial role in refining AI systems. Steve, could you share how researchers' input has influenced the development of ChatGPT?
Steve, what kind of data is used to refine the training of ChatGPT specifically for MALDI-TOF analysis? Is it publicly available or mainly sourced from research collaborations?
User feedback can help identify system biases and areas for improvement. Steve, have there been any instances where user feedback led to significant system enhancements?
Steve, considering data availability is key too. Can researchers with limited resources and restricted access to data leverage ChatGPT effectively for MALDI-TOF analysis?
Linda, you bring up an important point. While larger and more diverse datasets yield better results, efforts are being made to develop models that generalize well with limited data. Empowering researchers with limited resources is a priority for the community.
I'm amazed by the potential of ChatGPT in revolutionizing MALDI-TOF analysis. The ability to provide explanations and suggest matches could save scientists a lot of time in data interpretation.
The accuracy and reliability of AI systems are always important to consider in scientific applications. How does ChatGPT compare to traditional analysis methods in terms of accuracy?
Are there any limitations to ChatGPT in the context of MALDI-TOF analysis? How adaptable is it to different sample types and experimental conditions?
How does ChatGPT assist in protein sequence analysis? Can it handle the complexity and variability in protein structures?
Traditional analysis methods often require extensive manual work and expertise. It would be interesting to see how ChatGPT's accuracy compares to human experts in MALDI-TOF analysis.
I wonder if ChatGPT can handle noisy data or unexpected experimental conditions. It would be crucial to assess its robustness in real-world scenarios and different sample types.
It would be great to hear about specific cases where ChatGPT helped researchers achieve breakthroughs in MALDI-TOF analysis.
Protein sequence analysis requires resolving complex patterns and structures. I'm curious to know how ChatGPT handles this intricacy.
Comparing ChatGPT's accuracy to human experts would be intriguing. It could validate the feasibility and reliability of incorporating AI into this field.
Validating the robustness of ChatGPT under different experimental conditions would be crucial. It needs to handle various uncertainties that can occur in real-world settings.
Knowing specific success stories would demonstrate the practical impact of ChatGPT in MALDI-TOF analysis. It can inspire other researchers to adopt similar approaches.
Indeed, protein structure variations pose challenges. I'm curious if ChatGPT leverages existing databases or learns it during training.
ChatGPT's potential to save time in data analysis is impressive. It could free up scientists to focus on other critical aspects of their research.
Considering the vast variability in protein structures, ChatGPT must have a robust framework to handle diverse scenarios effectively.
ChatGPT streamlining the analysis process sounds promising. It might lead to increased efficiency and speed in MALDI-TOF analysis routines.
Understanding how ChatGPT handles complex patterns in protein sequences would be insightful. It could open doors for automation and accelerate research.
Exactly, Olivia! If ChatGPT can effectively interpret and analyze diverse protein structures, it could greatly aid in areas like drug discovery and treatment development.
Validating ChatGPT's accuracy against human experts in MALDI-TOF analysis could potentially establish it as a reliable tool for researchers worldwide.
Sophia, I agree. Data-driven AI systems can provide insights beyond human analysis capabilities, but validation against established methods is crucial to build trust.
Indeed, Sophia. Handling diverse protein structures effectively is crucial for ChatGPT's applicability in various biotechnological applications as well.
Emily, understanding ChatGPT's capabilities in analyzing complex protein patterns could be a game-changer, especially in understanding diseases and genetic disorders better.
Automation can save time and reduce human errors. ChatGPT's versatility in different scientific domains makes it an exciting tool for researchers in various fields.
Absolutely, Jacob. Streamlining analysis processes could accelerate research progress and enable scientists to delve deeper into their specific areas of focus.
Jacob, indeed. By automating certain aspects, ChatGPT allows scientists to focus on more sophisticated analysis methods that could lead to groundbreaking discoveries.
Comparing ChatGPT's accuracy against human experts could be a stepping stone for researchers in the field of MALDI-TOF analysis to adopt AI-assisted workflows.
Time saved through automation can be utilized for exploring new research directions. ChatGPT can bring about a positive shift in the pace of scientific breakthroughs.
Validating ChatGPT's robustness against unexpected experimental conditions would be interesting. Real-world applicability is a significant factor to consider.
Sophia, I completely agree. Robustness is key since experimental conditions can vary significantly, and ChatGPT should adapt accordingly for optimal performance.
Agreed, Sophia. The ability to handle varying protein structures equips ChatGPT to tackle a vast range of biological and medical analyses beyond MALDI-TOF.
Absolutely, Emily. The potential impact of ChatGPT expands beyond the field of MALDI-TOF analysis. Its versatility makes it a powerful tool in various biotechnological and medical settings.
Emily, the ability to handle various protein structures would be highly beneficial in understanding the complex interactions involved in disease mechanisms.
User feedback plays a crucial role. By actively engaging the research community, it ensures that ChatGPT meets the evolving needs of scientists and continues to evolve itself.
Amy, you're absolutely correct. User feedback has been invaluable in uncovering edge cases, biases, and areas for improvement. It has resulted in significant enhancements to ChatGPT's performance and usability.