Revolutionizing Technology: Harnessing ChatGPT for Enhanced Exploratory Data Analysis (EDA)
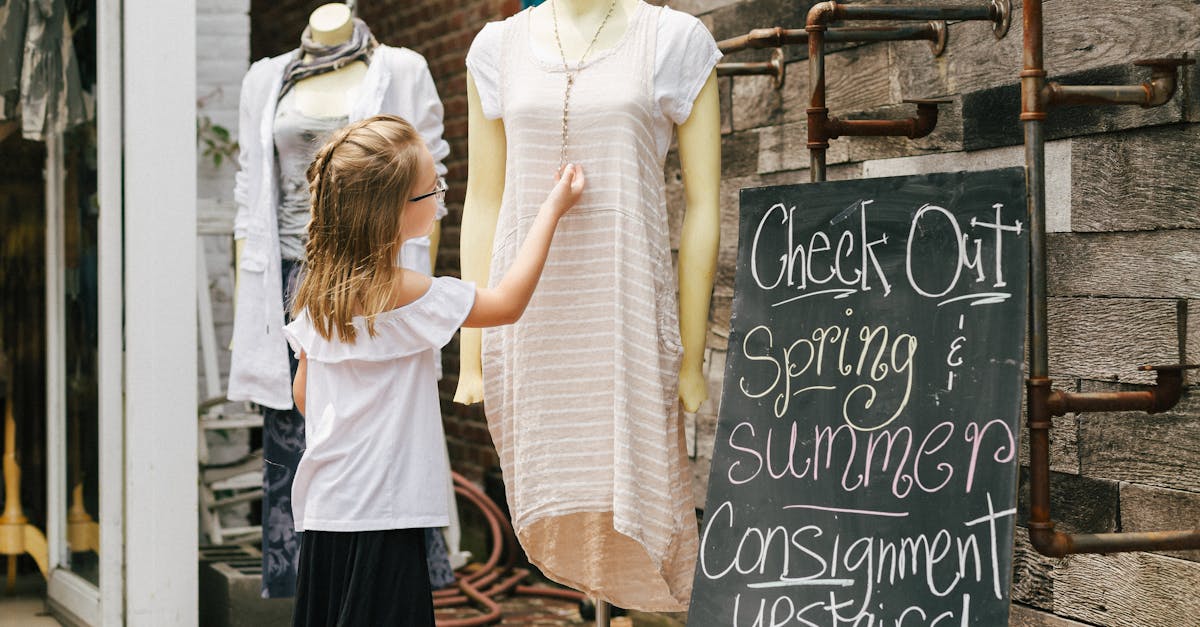
In the field of Exploratory Data Analysis (EDA), ensuring clean and error-free data is of utmost importance. One new technology that has emerged as a powerful tool in this area is ChatGPT-4. This advanced conversational AI model can assist data scientists and analysts in the process of making sure their data is correctly formatted and free of errors, ultimately leading to more accurate and reliable analysis.
Understanding EDA and Data Cleaning
EDA is a crucial step in the data analysis pipeline. It involves exploring and summarizing datasets to gain insights, identify patterns, and detect anomalies. However, before jumping into analysis, it is essential to ensure that the data is clean.
Data cleaning, also known as data cleansing or data scrubbing, refers to the process of identifying and correcting or removing errors, inconsistencies, and inaccuracies from a dataset. These errors can arise from various sources, such as data entry mistakes, missing values, duplicate entries, or formatting issues. Cleaning the data is necessary to eliminate potential biases or misleading conclusions that may arise from flawed information.
The Role of ChatGPT-4 in Data Cleaning
ChatGPT-4 is an AI model developed by OpenAI that excels in natural language processing and understanding. Its advanced capabilities make it a valuable tool for data scientists and analysts, especially in the domain of EDA.
By leveraging ChatGPT-4, data professionals can conduct interactive conversations with the model to assist in the data cleaning process. The model can comprehend complex instructions and perform tasks such as:
- Identifying and handling missing values: ChatGPT-4 can help identify missing values in a dataset and suggest appropriate methods for imputation or removal.
- Removing duplicate entries: The model can analyze the dataset and provide recommendations on identifying and removing duplicate records.
- Standardizing data formats: ChatGPT-4 can aid in standardizing data formats by suggesting changes to variables, ensuring consistency across the dataset.
- Validating data against predefined formats: The AI model can check if data adheres to predefined formats or regulations, helping maintain compliance and accuracy.
- Identifying and correcting data entry errors: ChatGPT-4 has the capability to identify potential data entry errors and propose corrections based on contextual understanding.
Benefits of Using ChatGPT-4 in EDA Data Cleaning
The integration of ChatGPT-4 in EDA data cleaning offers numerous benefits:
- Efficiency: ChatGPT-4 can quickly analyze large datasets and provide suggestions for cleaning, reducing the time and effort required from data professionals.
- Accuracy: By leveraging its deep learning capabilities, ChatGPT-4 can accurately identify errors and provide effective solutions.
- Interactivity: Data scientists and analysts can hold dynamic conversations with ChatGPT-4 to clarify instructions or seek further assistance.
- Adaptability: ChatGPT-4 can handle different data types and formats, making it suitable for a wide range of EDA tasks.
- Ease of Integration: The model can be seamlessly integrated into existing data cleaning workflows, enhancing the overall data analysis process.
Conclusion
Data cleaning plays a critical role in EDA, and with the emergence of advanced technologies like ChatGPT-4, the process has become more efficient and accurate. Data professionals can benefit greatly from this AI model, which can not only understand complex instructions but also provide valuable suggestions and insights throughout the data cleaning journey. As EDA technologies continue to evolve, leveraging such tools can significantly enhance the quality and reliability of data analysis results.
Comments:
Thank you all for your comments! I appreciate the engagement and different perspectives.
This article is fascinating! ChatGPT seems like a powerful tool for EDA. Have any limitations been identified?
Hi David! Great question. While ChatGPT is indeed a powerful tool, it does have limitations. It can sometimes generate responses that sound plausible but aren't accurate. It's important to critically evaluate the results and verify them with other methods.
I'm impressed with the potential of ChatGPT for EDA. It can help make data exploration more interactive and efficient.
Hi Rachel! Absolutely, ChatGPT can greatly enhance the exploratory data analysis process. It allows users to ask questions to uncover patterns or insights that traditional methods may overlook.
I wonder, can ChatGPT handle large datasets effectively?
Hi Samuel! ChatGPT may have difficulty with very large datasets due to computational limitations. It's recommended to use it on smaller subsets or to summarize the data before applying ChatGPT.
ChatGPT seems like a game-changer for data scientists. It can simplify the EDA process and make it more accessible.
Hi Jennifer! Absolutely, ChatGPT has the potential to revolutionize the EDA process by providing a more user-friendly interface for data scientists.
I'm concerned about ChatGPT's bias. How can we ensure it doesn't amplify existing biases in the data?
Hi Andrew! Bias is an important concern, and it's crucial to provide diverse and balanced training data to ChatGPT. Additionally, continuously evaluating and mitigating biases is essential to ensure fair and reliable results.
ChatGPT sounds like a promising tool, but I wonder about its learning curve. Is it easy for non-technical users to utilize?
Hi Eleanor! While ChatGPT is designed to be user-friendly, non-technical users may still face a learning curve. Familiarity with basic data analysis concepts would be helpful to effectively utilize and interpret the results.
I like the idea of using ChatGPT for EDA, but can it handle textual data efficiently?
Hi Oliver! ChatGPT is well-suited for analyzing textual data since it has been trained on a vast amount of text. It can help uncover patterns, sentiment, or other insights within textual datasets.
This article is quite enlightening! I'm excited to try out ChatGPT for my next EDA project.
Hi Lily! I'm glad you found the article helpful. I wish you the best of luck with your EDA project using ChatGPT. Feel free to share your experience and any insights you discover.
What are the security and privacy implications of using ChatGPT for EDA?
Hi Daniel! Security and privacy are important concerns. It's recommended to be cautious and avoid sharing sensitive or confidential data when utilizing ChatGPT or any other cloud-based tool. Additionally, ensure the data is properly anonymized.
I'm wondering, can ChatGPT be combined with other automation tools to streamline the EDA workflow?
Hi Emily! Absolutely, ChatGPT can be combined with other automation tools to create a more streamlined EDA workflow. The seamless integration of different tools can enhance efficiency and improve the overall analysis process.
How easy is it to fine-tune ChatGPT for specific EDA tasks?
Hi Sophia! Fine-tuning ChatGPT for specific EDA tasks requires some technical expertise. It involves providing task-specific data and formulating the problem as a supervised learning task. However, OpenAI provides documentation and resources to guide users through the fine-tuning process.
ChatGPT seems like it would be great for hypothesis generation during EDA. It could help identify new avenues for investigation.
Hi Grace! Absolutely, ChatGPT can be a valuable tool for generating hypotheses during EDA. It can provide fresh insights and suggest new directions for further exploration.
I'm concerned about the cost of using ChatGPT for EDA. Is it affordable for small-scale projects?
Hi Matthew! ChatGPT does come with a cost, which can vary depending on usage. For small-scale projects, it may be more affordable, but users should consider their budget and usage requirements to determine if it aligns with their project's scale.
ChatGPT can empower data analysts by providing interactive and conversational data exploration. It's an exciting development!
Hi Amy! Indeed, the interactive and conversational nature of ChatGPT can empower data analysts and drive more engaging and efficient data exploration. It's an exciting step forward in the field of data analysis.
I wonder if ChatGPT can handle complex statistical analyses during EDA.
Hi Henry! While ChatGPT may not directly handle complex statistical analyses, it can be a valuable tool for identifying patterns and relationships in the data. For advanced statistical analysis, complementary approaches may be required.
The potential of ChatGPT for EDA is intriguing. It could democratize data analysis and make it more accessible to a wider audience.
Hi Victoria! Absolutely, the potential of ChatGPT to democratize data analysis is very intriguing. By providing a more user-friendly interface and interactive experience, it can make data analysis more accessible to a wider range of users.
ChatGPT sounds like a great tool for hypothesis testing. It can help in identifying relationships and dependencies within the data.
Hi Zoe! You're absolutely right. ChatGPT can assist in hypothesis testing by identifying relationships and dependencies within the data. It can help validate or challenge existing hypotheses, leading to more robust analysis.
I'm concerned about the interpretability of ChatGPT's results. How can we ensure they're meaningful and informative?
Hi Michael! Ensuring the interpretability of ChatGPT's results is important. It's advisable to compare the results with other analytical methods and critically evaluate the insights generated. Remember to use ChatGPT as a complementary tool and not solely rely on its outputs.
ChatGPT could be a game-changer for beginner data analysts. It provides an intuitive and conversational approach to exploring data.
Hi Sarah! You're absolutely right. ChatGPT's intuitive and conversational approach can be especially beneficial for beginner data analysts, making the data exploration process more engaging and accessible.
I wonder if ChatGPT can be used for time series analysis during EDA.
Hi Nathan! While ChatGPT is not specifically designed for time series analysis, it can still provide valuable insights by identifying patterns, trends, or potential anomalies within the time series data.
ChatGPT could be a valuable tool for data storytelling during EDA. It can help analysts explain their findings more effectively.
Hi Jessica! Indeed, ChatGPT's interactive and conversational nature can enhance data storytelling during EDA. It can assist data analysts in explaining their key findings and insights in a more compelling and understandable manner.
I'm curious if ChatGPT can handle unstructured data effectively during EDA.
Hi Benjamin! ChatGPT is quite effective with unstructured data, such as text or document-based datasets. It can help identify patterns, sentiment, or other interesting aspects within unstructured data, thereby facilitating deeper analysis.
ChatGPT seems like a promising tool, but I'm wondering about its availability. Can it be accessed by everyone?
Hi Caroline! OpenAI provides access to ChatGPT, making it available to users across the globe. However, it's important to check the specific availability and accessibility details on OpenAI's website.
I'm concerned about ChatGPT's performance with noisy or incomplete datasets. Can it handle such data effectively?
Hi Robert! ChatGPT may struggle with noisy or incomplete datasets as it heavily relies on patterns and relationships in the data. Preprocessing the data to reduce noise and ensure completeness can improve its effectiveness in such cases.
This article is eye-opening! ChatGPT has the potential to transform how we approach EDA and gain insights from data.
Hi Hannah! I'm glad you found the article eye-opening. ChatGPT does indeed hold the potential to transform the EDA process, enabling data analysts to gain new and valuable insights from their data.
Can ChatGPT handle image or audio data during EDA?
Hi Tyler! ChatGPT is primarily designed for text-based data. While it may not handle image or audio data directly, it can still assist in analyzing textual metadata associated with such files.
I'm impressed by the versatility of ChatGPT. It seems like a powerful tool for various stages of EDA.
Hi Chloe! You're absolutely right. ChatGPT's versatility makes it a powerful tool for different stages of EDA, from initial exploration to hypothesis generation and validation.
ChatGPT can make EDA more interactive. It enables data analysts to explore data in a more conversational and dynamic manner.
Hi Oscar! Indeed, ChatGPT's conversational and interactive nature can greatly enhance the EDA experience. It brings a new level of engagement and dynamism to the data exploration process.
I'm curious about the resources required to train and deploy ChatGPT for EDA.
Hi Scarlett! Training and deploying ChatGPT requires substantial computational resources. OpenAI provides guidance and resources to help users with the process, but it's important to be aware of the resource requirements.
This article has given me a fresh perspective on EDA. ChatGPT can help data analysts approach their work from new angles.
Hi Mason! I'm glad the article provided you with a fresh perspective. ChatGPT encourages data analysts to approach their work from new angles and explore the data in a more conversational and flexible way.
ChatGPT can be a valuable tool for collaborative data exploration. It allows analysts to discuss and refine their insights with the help of the model.
Hi Alice! You're absolutely right. ChatGPT's collaborative nature makes it a valuable tool for team-based data exploration. It promotes discussions and facilitates the refinement of insights among analysts.
I'm concerned about the ethical aspects of using ChatGPT for EDA. How can we ensure ethical data practices?
Hi Jason! Ensuring ethical data practices is essential. It's crucial to adhere to data protection laws, ensure proper consent and anonymization, and be aware of potential biases in the data. Engaging in responsible data usage is key.
ChatGPT opens up opportunities for more interactive and dynamic data storytelling. It can make complex analysis more accessible.
Hi Emma! Absolutely, ChatGPT's interactive and dynamic nature can elevate the data storytelling process. It allows analysts to present complex analysis in a more accessible and engaging way, promoting better understanding and decision-making.
I'm curious about the training data used for ChatGPT. How diverse is it?
Hi Leo! ChatGPT has been trained on a diverse range of internet text to make it more versatile. However, biases may still exist, highlighting the importance of critical evaluation and unbiased training data for the best outcomes.
ChatGPT can be a valuable tool for quality assurance during EDA. It can help identify anomalies or inconsistencies in the data.
Hi Grace! You're absolutely right. ChatGPT can aid in quality assurance during EDA by identifying anomalies, inconsistencies, or other potential issues within the data. It complements traditional QA methods.
I wonder how ChatGPT handles missing data during EDA.
Hi Daniel! ChatGPT doesn't specifically handle missing data. It's important to preprocess the data and handle missing values appropriately before applying ChatGPT for optimal results.
This article has sparked my curiosity about ChatGPT! It seems like an innovative approach to EDA.
Hi Julia! I'm glad the article sparked your curiosity about ChatGPT. It is indeed an innovative approach that can revolutionize the EDA process. Feel free to explore it further and see how it can augment your data analysis workflows.
I'm concerned about the potential for bias in ChatGPT's responses. How can we address this issue?
Hi Patrick! Bias is an important concern, and it's crucial to provide diverse and unbiased training data to ChatGPT. OpenAI is actively working on reducing biases, and user feedback is valuable in identifying and addressing any biases that may arise.
I'm curious if ChatGPT is suitable for real-time EDA, especially in scenarios where data is streaming continuously.
Hi Zachary! ChatGPT may not be suited for real-time EDA, particularly with continuously streaming data. It's more suitable for interactive exploration of pre-existing datasets or summary statistics obtained from streaming data.
Could ChatGPT assist with data preprocessing tasks during EDA?
Hi Leah! While ChatGPT is primarily geared towards analysis and exploration, it can provide insights that might aid in the data preprocessing stage. However, it's important to leverage other specialized tools and techniques for comprehensive data preprocessing.
ChatGPT has the potential to democratize data analysis. It can enable users without advanced technical skills to gain insights from their data.
Hi Mia! You're absolutely right. ChatGPT's user-friendly interface and conversational approach can democratize data analysis by making it more accessible to individuals without advanced technical skills.
I'm concerned about the computational resources required to run ChatGPT. Can it be resource-intensive?
Hi Jackson! ChatGPT can be resource-intensive, especially for large datasets or complex analyses. It's recommended to have access to significant computational resources, such as GPUs or cloud-based computing, to ensure optimal performance.
ChatGPT can be a valuable tool for data-driven decision-making during EDA. It helps analysts uncover insights to support informed choices.
Hi Ella! You're absolutely right. ChatGPT assists in data-driven decision-making during EDA by helping analysts uncover insights and gather evidence to support informed choices. It enhances the decision-making process.
I wonder if ChatGPT can handle structured data effectively during EDA.
Hi Joshua! ChatGPT's strength lies in analyzing unstructured or textual data. While it may not handle structured data as effectively, it can still provide insights by analyzing metadata or textual elements associated with structured data.
This article has inspired me to explore ChatGPT for my next EDA project! It seems like a valuable tool.
Hi Caleb! I'm glad the article has inspired you to explore ChatGPT for your next EDA project. It can indeed be a valuable tool, and I hope it enhances your data analysis process. Don't hesitate to share your experience and insights with the community.
I'm curious if ChatGPT can handle multivariate data analysis during EDA.
Hi Eva! While ChatGPT is not specifically designed for multivariate data analysis, it can still assist in exploring relationships and patterns between variables within the data.
ChatGPT can be a valuable tool for exploratory analysis of large datasets. It helps identify interesting patterns and trends.
Hi Samuel! Absolutely, ChatGPT's exploratory capabilities make it a valuable tool for analyzing large datasets. It aids in uncovering interesting patterns, trends, or potential outliers, enriching the exploratory analysis process.
I'm interested in the potential applications of ChatGPT beyond EDA. Can it be used for other data analysis tasks?
Hi Grace! While ChatGPT is particularly suited for EDA, it can also be applied to other data analysis tasks. Its conversational approach can be leveraged to gain insights and explore data in different domains, beyond EDA.
ChatGPT seems like a great tool for interactive data exploration. It can facilitate collaboration and knowledge sharing among data analysts.
Hi Harrison! You're absolutely right. ChatGPT's interactive and collaborative nature fosters knowledge sharing and collaboration among data analysts, making the data exploration process more effective and insightful.
ChatGPT can be a valuable tool for data validation during EDA. It helps ensure the accuracy and integrity of the data.
Hi Claire! Absolutely, ChatGPT can contribute to data validation during EDA by assisting in identifying potential anomalies, inconsistencies, or errors within the data. It complements traditional data validation techniques.
I'm curious if ChatGPT can handle high-dimensional data effectively during EDA.
Hi Isaac! ChatGPT's effectiveness with high-dimensional data may vary. While it can still provide insights, complex visualizations or dimensionality reduction techniques may be necessary to ensure a more comprehensive analysis of high-dimensional datasets.
ChatGPT could be a valuable tool for hypothesis generation during EDA. It can suggest potential relationships for further investigation.
Hi Paige! You're absolutely right. ChatGPT's ability to suggest potential relationships and patterns can greatly aid in hypothesis generation during EDA. It opens up new avenues for data analysts to explore.
ChatGPT seems like it would be helpful for data analysts working with unstructured datasets. It can provide guidance and insights in navigating such data.
Hi Connor! Absolutely, ChatGPT's guidance and insights can greatly assist data analysts in working with unstructured datasets. It can help uncover the hidden value within unstructured data.
I'm interested in how ChatGPT handles outliers during EDA. Can it effectively identify and analyze outliers?
Hi Audrey! While ChatGPT may not explicitly focus on outlier detection, it can still assist in identifying potential outliers through its analysis of patterns and relationships in the data. To comprehensively analyze outliers, complementary statistical techniques are recommended.
Can ChatGPT be used iteratively during EDA?
Hi Thomas! Absolutely, ChatGPT can be used iteratively during EDA. Its conversational and interactive nature allows for an iterative exploration process, where questions can be refined based on previous insights and new paths of analysis can be pursued.
ChatGPT can be a valuable tool for data analysts looking to uncover hidden insights in their datasets. It complements traditional analysis techniques.
Hi Alexis! You're absolutely right. ChatGPT's ability to uncover hidden insights and complement traditional analysis techniques makes it a valuable tool for data analysts. It augments the analysis process.
I'm concerned about potential biases in ChatGPT's responses. How can we address this issue and ensure more fairness in its outputs?
Hi Brandon! Addressing biases is crucial. OpenAI is actively working on reducing biases in ChatGPT's responses. They seek user feedback to identify and understand potential biases, which helps in making the model more fair, reliable, and useful.
ChatGPT seems like a promising tool for hypothesis generation and validation during EDA. It can spark new ideas and help verify them.
Hi Bella! Absolutely, ChatGPT can be a game-changer for hypothesis generation and validation during EDA. It stimulates new ideas and supports analysts in verifying their hypotheses more effectively.
I'm impressed with the potential of ChatGPT for supporting decision-making during EDA. It seems like a valuable ally for data analysts.
Hi Jordan! You're absolutely right. ChatGPT's ability to support decision-making during EDA makes it a valuable ally for data analysts. It promotes data-driven and informed choices.
ChatGPT could be a valuable tool for data analysts looking to communicate their findings effectively. It helps bridge the gap between data analysis and stakeholders.
Hi Hailey! Indeed, ChatGPT can facilitate effective communication between data analysts and stakeholders. It bridges the gap by presenting complex analysis in a conversational and understandable manner, making the findings more accessible.
I'm curious if ChatGPT can assist with exploratory visualization during EDA. Can it generate charts or plots based on data?
Hi Beatrix! While ChatGPT is primarily focused on textual interactions and insights, it can suggest visualization approaches or generate textual descriptions of potential visualizations, aiding in exploratory visualization during EDA.
This article has opened my eyes to the potential of ChatGPT for EDA. It seems like an exciting development in the field.
Hi Jake! I'm glad the article has piqued your interest in ChatGPT for EDA. It is indeed an exciting development, and I hope you find it valuable in your data exploration endeavors.
I wonder if ChatGPT can handle streaming data effectively during EDA.
Hi Sophie! ChatGPT's effectiveness with streaming data may be limited, as it's primarily designed for interactive exploration of pre-existing datasets. For analyzing streaming data, real-time data analysis frameworks and specialized tools may be more suitable.
ChatGPT seems like it would be great for data analysts seeking a more conversational and interactive approach. It can enhance engagement.
Hi Toby! Absolutely, ChatGPT's conversational and interactive approach can greatly enhance engagement among data analysts. It adds a new level of dynamism to the data exploration process.
ChatGPT could be a useful tool for data analysts seeking insights from their data without complex programming or modeling.
Hi Victoria! You're absolutely right. ChatGPT's user-friendly interface and conversational approach can empower data analysts who may not have extensive programming or modeling skills. It facilitates insights without the need for complex techniques.
I'm curious about the model's ability to explain its reasoning. Can ChatGPT provide explanations for its insights during EDA?
Hi Thomas! Explaining its reasoning is an area where ChatGPT has limitations. While it can provide insights and suggestions based on patterns it has learned, providing detailed explanations for those insights might not be within its capabilities.
ChatGPT can be a valuable tool for data analysts looking to uncover interesting patterns and relationships within their datasets.
Hi Chloe! Absolutely, ChatGPT's ability to identify interesting patterns and relationships within datasets makes it a valuable tool for data analysts. It aids in uncovering hidden insights.
This article has broadened my horizons about EDA techniques. ChatGPT can bring an interactive and engaging dimension to the process.
Hi Jason! I'm glad the article broadened your horizons about EDA techniques. Indeed, ChatGPT's interactive and engaging dimension can be transformative in the data exploration process, enabling analysts to approach their work from new perspectives.
I wonder if ChatGPT can help with exploratory clustering during EDA.
Hi Caroline! While ChatGPT may not specifically focus on exploratory clustering, it can still generate insights and suggest potential groupings based on patterns or relationships it identifies in the data.
ChatGPT seems like it would be an excellent tool for educational purposes. It can help students explore and analyze a variety of datasets.
Hi Benjamin! You're absolutely right. ChatGPT's user-friendly and conversational nature can make it an excellent educational tool. It helps students explore, analyze, and gain insights from various datasets, promoting hands-on learning.
I'm impressed with the potential of ChatGPT for interactive data exploration. It seems like a step towards a more intuitive analysis process.
Hi Luna! Absolutely, ChatGPT's interactive and intuitive nature significantly enhances the data exploration process. It's a step forward in making data analysis more accessible and engaging.
I'm curious if ChatGPT can handle geospatial data effectively during EDA.
Hi Owen! While ChatGPT is not specifically designed for geospatial analysis, it can still provide insights by analyzing textual metadata associated with geospatial data points. For in-depth geospatial analysis, specialized tools may be more suitable.
This article has sparked my interest in exploring ChatGPT for data analysis and visualization. It seems like a powerful resource.
Hi Isla! I'm glad the article sparked your interest in exploring ChatGPT for data analysis and visualization. It is indeed a powerful resource that can elevate your analysis and visualization efforts.
I wonder if ChatGPT can assist with data wrangling tasks during EDA. Can it suggest data transformations or cleansing steps?
Hi Elliot! While ChatGPT is not specifically designed for data wrangling, it can provide suggestions and insights regarding potential data transformations or cleansing steps through its analysis of patterns within the data.
This article has given me a fresh perspective on EDA techniques. ChatGPT can facilitate a more iterative and dynamic exploration approach.
Hi William! I'm glad the article provided you with a fresh perspective on EDA techniques. Indeed, ChatGPT's iterative and dynamic nature allows for a more engaging and exploratory analysis approach.
I wonder if ChatGPT can be fine-tuned on domain-specific datasets to enhance its effectiveness in that particular area.
Hi Maya! You're absolutely right. ChatGPT can be fine-tuned on domain-specific datasets to make it more effective and relevant in that particular area of analysis. Fine-tuning enables the model to specialize for specific use cases.
ChatGPT seems like a tool that can bridge the gap between data and insights. It facilitates a more interactive exploration process.
Hi Kai! You're absolutely right. ChatGPT's ability to bridge the gap between data and insights makes it a valuable tool. It facilitates a more interactive and dynamic exploration process, enhancing the data analysis experience.
I wonder if ChatGPT can handle high-dimensional data effectively during EDA.
Hi Julian! ChatGPT can analyze high-dimensional data to some extent by identifying patterns and relationships within the data. However, for a comprehensive analysis of high-dimensional datasets, specialized techniques like dimensionality reduction may be required.
This article has highlighted the potential of ChatGPT for enhancing the EDA process. It can streamline and simplify complex data analysis tasks.
Hi Holly! I'm glad the article highlighted the potential of ChatGPT for enhancing the EDA process. It can indeed streamline and simplify complex data analysis tasks, making them more accessible and informative.
I'm impressed by ChatGPT's ability to generate insights and suggestions during EDA. It seems like a valuable aid for data analysts.
Hi Joseph! You're absolutely right. ChatGPT's ability to generate insights and suggestions makes it a valuable aid for data analysts during the EDA process. It augments their analysis and facilitates data-driven decision-making.
ChatGPT seems like a flexible and versatile tool for data analysts. It can adapt to various domains and analysis requirements.
Hi Sophia! You're absolutely right. ChatGPT's flexibility and versatility enable its adaptation to various domains and analysis requirements. It can cater to a wide range of data analysis needs.
I'm curious if ChatGPT can handle real-time data during EDA. Can it process and analyze data as it arrives?
Hi Connor! ChatGPT's primary strength lies in interactive analysis of pre-existing datasets, rather than real-time data analysis. It's more suitable for analyzing data that has already been collected and is available for exploration.
This article has sparked my interest in exploring ChatGPT for interactive data analysis. It seems like a valuable aid for data scientists and researchers.
Hi Alex! I'm glad the article sparked your interest in exploring ChatGPT for interactive data analysis. It can indeed be a valuable aid for data scientists and researchers, enhancing their analysis capabilities.
I wonder if ChatGPT can assist in exploratory feature engineering. Can it suggest potentially useful features to explore during EDA?
Hi Owen! While ChatGPT may not directly suggest particular features for exploration, it can provide insights and potential relationships within the data, which can guide feature engineering efforts during EDA.
ChatGPT could be a useful tool for data analysts looking to uncover hidden patterns or insights in their datasets.
Hi Lily! Absolutely, ChatGPT's ability to uncover hidden patterns and insights makes it a useful tool for data analysts. It aids in exploring data from new angles and gathering valuable insights.
I'm impressed with ChatGPT's interactive and conversational nature. It makes data analysis a more engaging and collaborative process.
Hi Ethan! You're absolutely right. ChatGPT's interactive and conversational nature adds a new dynamic to the data analysis process. It fosters collaboration and engagement among analysts, making the process more rewarding.
ChatGPT can be a valuable tool for analysts looking to explore data in a more conversational and engaging way. It adds a human touch to the analysis process.
Hi Hayden! Absolutely, ChatGPT's conversational and engaging approach brings a human touch to the analysis process. It encourages analysts to interact with the data in a more intuitive and insightful manner.
This article has opened my eyes to the potential of ChatGPT for EDA. It can make the analysis process more dynamic and exploratory.
Hi Archie! I'm glad the article opened your eyes to the potential of ChatGPT for EDA. It indeed brings a new level of dynamism and exploration to the data analysis process, making it more engaging and insightful.
I'm curious if ChatGPT can generate reports summarizing the key findings during EDA.
Hi Kylie! While ChatGPT may not explicitly generate reports, it can assist analysts in summarizing their key findings and insights. Analysts can utilize ChatGPT's conversational nature to uncover and distill the most essential aspects for reporting purposes.
ChatGPT seems like a promising tool for data analysts looking to explore and analyze their datasets in an interactive manner.
Hi Poppy! You're absolutely right. ChatGPT's interactive approach makes it a promising tool for data analysts seeking to explore and analyze their datasets more dynamically. It encourages experimentation and deeper analysis.
I wonder if ChatGPT can handle complex data structures during EDA.
Hi Camila! While ChatGPT may not directly handle complex data structures, it can provide insights and identify patterns within the data. For in-depth analysis of complex structures, specialized techniques or complementary tools may be necessary.
This article has given me a new perspective on how to approach EDA. ChatGPT's interactive nature can help uncover fresh insights.
Hi Harry! I'm glad the article provided you with a fresh perspective on approaching EDA. ChatGPT's interactive and insightful nature aids in uncovering new and valuable insights from data. Happy exploring!
ChatGPT seems like a valuable tool for exploratory analysis of complex datasets. It assists analysts in uncovering noteworthy patterns and trends.
Hi Lucas! Absolutely, ChatGPT's role in exploratory analysis of complex datasets is invaluable. It helps analysts identify noteworthy patterns, trends, anomalies, or relationships within the data, enriching the analysis process.
I'm curious about the instances where ChatGPT is most effective during EDA. Can you provide some examples?
Hi Ava! ChatGPT is particularly effective in scenarios where analysts want to explore data interactively and have open-ended conversations to uncover patterns, insights, or potential relationships. It shines when applied to textual or unstructured data analyses but can also provide valuable guidance with structured data.
This article has broadened my horizons about the possibilities of EDA. ChatGPT's interactivity can inspire new analysis paths.
Hi George! I'm glad the article broadened your horizons about the possibilities of EDA. ChatGPT's interactivity and flexibility indeed inspire data analysts to approach their analysis from different angles, leading to new and valuable insights.
I wonder if ChatGPT can be biased in its responses, especially when analyzing sensitive or controversial topics.
Hi Stella! Bias is an important concern, particularly when analyzing sensitive or controversial topics. Careful training and evaluation, along with user feedback, are essential in identifying and addressing biases in ChatGPT's responses.
ChatGPT seems like a powerful tool for data analysis, but I wonder about its resource requirements. Can it run on modest hardware?
Hi Jasmine! ChatGPT may have significant resource requirements, particularly for large-scale analyses or complex computations. While it may run on modest hardware, the model's performance might be slower or limited. Having access to stronger computing resources can ensure better performance.
This article has inspired me to experiment with ChatGPT for my next EDA project. It seems like a game-changer.
Hi Elijah! I'm glad the article inspired you to experiment with ChatGPT for your next EDA project. It can indeed be a game-changer, and I wish you success and valuable insights in your analysis.
ChatGPT seems like a valuable tool for data analysts looking to uncover insights from their datasets. It facilitates an exploratory mindset.
Hi Maya! You're absolutely right. ChatGPT's ability to uncover insights and promote an exploratory mindset makes it a valuable tool for data analysts. It adds a new dimension to the analysis process.
I'm curious if ChatGPT can assist in identifying outliers during EDA.
Hi James! While ChatGPT may not explicitly identify outliers, it can assist in identifying potential outliers through its analysis of patterns and relationships in the data. For a comprehensive outlier analysis, complementary statistical techniques would be more appropriate.
ChatGPT can be a valuable tool for EDA, as it brings a conversational and interactive approach to exploring data.
Hi Zara! Absolutely, ChatGPT's conversational and interactive approach greatly enhances the EDA process. It encourages a more engaging and intuitive exploration of data, unveiling hidden insights.
I'm curious if ChatGPT can assist with data imputation during EDA.
Hi Sophie! While data imputation falls outside the primary scope of ChatGPT, it can still provide insights and suggestions based on patterns it identifies in the data. Specific tools or algorithms may be more suitable for comprehensive data imputation tasks.
ChatGPT can make the data exploration process more interactive and engaging. It helps analysts delve deeper into their datasets.
Hi Maddison! Absolutely, ChatGPT's interactive and engaging nature makes the data exploration process more rewarding and insightful. It assists analysts in delving deeper into their datasets and discovering hidden gems of knowledge.
I wonder if ChatGPT can handle imbalanced datasets effectively during EDA.
Hi Angelina! While ChatGPT is not specifically designed to address imbalanced datasets during EDA, it can provide insights and suggestions regarding patterns or relationships in the data. Imbalanced data analysis may require the use of specialized techniques or approaches.
This article has given me a fresh perspective on EDA. ChatGPT's conversational approach can inspire new analysis directions.
Hi Bentley! I'm glad the article provided you with a fresh perspective on EDA. ChatGPT's conversational approach indeed inspires new analysis directions and encourages analysts to explore their data from different angles, uncovering novel insights.
ChatGPT can be a valuable tool for analysts looking to gain quick insights from their datasets in a conversational manner. It adds a collaborative aspect to the analysis process.
Hi Mya! You're absolutely right. ChatGPT's conversational nature and quick insights make it a valuable tool for analysts. It fosters collaboration and helps uncover insights more efficiently.
I'm curious if ChatGPT can assist with feature selection during EDA.
Hi Eli! While ChatGPT may not directly assist with feature selection, it can help identify patterns, insights, or potential interdependencies within the data, which can guide feature selection efforts during EDA.
ChatGPT seems like a powerful tool for exploratory analysis. It can help uncover interesting patterns and relationships within datasets.
Hi Joseph! Absolutely, ChatGPT's ability to uncover interesting patterns and relationships makes it a powerful tool for exploratory analysis. It enriches the overall analysis process and leads to valuable insights.
This article has sparked my curiosity about using ChatGPT for data-driven storytelling during EDA. It adds a narrative element to the analysis.
Hi Katherine! I'm glad the article sparked your curiosity about data-driven storytelling using ChatGPT. It indeed adds a narrative element to the analysis process, making it more engaging and informative for diverse audiences.
I'm curious if ChatGPT can assist with dimensionality reduction during EDA.
Hi Matthew! While ChatGPT is not specifically designed for dimensionality reduction, it can provide insights and suggestions based on patterns it identifies in the data. For comprehensive dimensionality reduction, specialized techniques like principal component analysis or t-SNE may be more suitable.
ChatGPT seems like a valuable tool for analysts looking to dive deeper into their datasets and gain insights through conversation.
Hi Brianna! Absolutely, ChatGPT's conversational approach is valuable for analysts seeking to dive deeper into their datasets. It aids in uncovering insights by fostering interactive and intuitive exploration.
I wonder if ChatGPT can assist with data integration and fusion during EDA.
Hi Megan! While data integration and fusion falls beyond ChatGPT's primary focus, it can provide insights and suggestions, guiding analysts towards potential relationships or patterns within the integrated data.
ChatGPT seems like a promising tool for analysts looking to explore and gain insights from their datasets in a more conversational manner.
Hi Hazel! You're absolutely right. ChatGPT's conversational approach holds great promise for analysts seeking to explore and gain insights from their datasets in a more interactive and conversational manner. It enriches the analysis process.
This article has inspired me to explore ChatGPT's potential for hypothesis generation during EDA. It seems like a valuable tool.
Hi Danielle! I'm glad the article inspired you to explore ChatGPT's potential for hypothesis generation during EDA. It can indeed be a valuable tool, assisting analysts in generating fresh hypotheses and direction for their analyses.
I'm curious about the training duration required for ChatGPT. Can you provide an idea of the time investment involved?
Hi Carter! Training ChatGPT requires significant computational resources and time investment. The exact duration depends on factors like the dataset size, hardware resources, and the desired performance level. It can range from several hours to days.
ChatGPT can be a valuable tool for data analysts looking to explore their datasets interactively. It provides a fresh perspective.
Hi Daisy! You're absolutely right. ChatGPT's interactive nature makes it a valuable tool for data analysts seeking to explore their datasets from new angles. It adds a fresh perspective to the analysis process.
I wonder if ChatGPT can assist with data profiling and summary generation during EDA.
Hi Lucas! While ChatGPT may not specifically handle data profiling or summary generation, it can provide insights and suggestions based on patterns and relationships it identifies in the data. Analysts can utilize these insights to facilitate data profiling and summarization processes.
ChatGPT seems like a powerful tool for analysts looking to gain new insights from their datasets. It promotes a more interactive exploration approach.
Hi Samuel! Absolutely, ChatGPT's ability to generate new insights and promote an interactive exploration approach makes it a powerful tool for analysts. It adds depth and versatility to the analysis process.
I wonder if ChatGPT can assist with feature importance analysis during EDA.
Hi Alice! While ChatGPT may not specifically focus on feature importance analysis, it can provide insights and potential relationships within the data, which can guide feature importance determination efforts during EDA.
This article has given me a fresh perspective on EDA techniques. ChatGPT can augment the analysis process by providing a conversational approach.
Hi Cole! I'm glad the article provided you with a fresh perspective on EDA techniques. ChatGPT's conversational and intuitive approach indeed augments the analysis process, promoting more engaging and insightful exploration.
ChatGPT seems like a promising tool for data analysts looking for a more conversational and engaging approach to EDA. It opens up new possibilities.
Hi Emilia! Absolutely, ChatGPT's conversational and engaging approach opens up new possibilities for data analysts, making the EDA process more interactive and rewarding. Happy exploring!
I'm curious about ChatGPT's limitations regarding large datasets. Can it handle datasets with millions of records?
Hi Henry! ChatGPT's effectiveness with very large datasets is limited due to computational constraints. It's recommended to use it on smaller subsets or to summarize the data before applying ChatGPT. This helps ensure optimal performance.
ChatGPT can be a valuable tool for analysts looking to extract insights from their data in a more interactive and intuitive manner.
Hi Amelia! You're absolutely right. ChatGPT's interactive and intuitive nature makes it a valuable tool for analysts. It enriches the data exploration process, enabling them to extract deeper insights from their data.
I wonder if ChatGPT can assist with exploratory time series analysis during EDA.
Hi Tyler! While ChatGPT is not specifically designed for exploratory time series analysis, it can still provide valuable insights by detecting patterns, trends, or potential anomalies within the time series data, aiding in the exploration process.
ChatGPT seems like a powerful tool for data analysts. It can facilitate a more interactive and insightful analysis process.
Hi Madison! You're absolutely right. ChatGPT's interactive and insightful capabilities make it a powerful tool for data analysts seeking a more engaging and exploratory analysis process. It uncovers hidden gems of knowledge within the data.
I wonder if ChatGPT can assist with exploratory analysis of time-varying data during EDA.
Hi Joshua! While ChatGPT is not explicitly designed for exploratory analysis of time-varying data, it can still provide insights by detecting patterns, trends, or potential relationships within the time-varying data points, enabling more comprehensive analysis.
This article has inspired me to explore ChatGPT for my next EDA project. It seems like a powerful and innovative approach.
Hi Emily! I'm glad the article inspired you to explore ChatGPT for your next EDA project. It is indeed a powerful and innovative approach that can enhance your data analysis endeavors. Happy exploring!
I'm curious if ChatGPT can handle imbalanced datasets effectively during EDA.
Hi Daniel! While ChatGPT may not directly handle imbalanced datasets during EDA, it can assist in identifying potential patterns or relationships within the data, which can guide analysis efforts for imbalanced datasets.
ChatGPT seems like a useful tool for data analysts seeking a more interactive approach to exploring their datasets. It can help uncover new insights.
Hi Olivia! You're absolutely right. ChatGPT's interactive approach makes it a useful tool for data analysts seeking to uncover new and valuable insights from their datasets. It fosters a more engaging and exploratory analysis process.
I wonder if ChatGPT can assist with exploratory analysis of geospatial data during EDA.
Hi Sebastian! While ChatGPT is not specifically designed for exploratory analysis of geospatial data, it can provide insights by analyzing textual metadata associated with geospatial data. Specialized tools or algorithms may be more suitable for detailed geospatial analysis.
ChatGPT can be a valuable tool for analysts seeking a more conversational and interactive approach to their data explorations. It adds a human touch.
Hi Isabella! Absolutely, ChatGPT's conversational and interactive approach adds a human touch to the data exploration process. It makes it more engaging and bridges the gap between analysts and their data.
This article has broadened my horizons about EDA techniques. ChatGPT opens up new possibilities for exploration.
Hi Maxwell! I'm glad the article broadened your horizons about EDA techniques. ChatGPT's interactive approach indeed opens up new possibilities and encourages analysts to uncover unique patterns and insights within their data.
I'm curious about ChatGPT's performance on small-scale datasets. Can it be equally effective regardless of dataset size?
Hi Christian! ChatGPT's effectiveness can vary depending on the dataset size. While it can provide valuable insights for small-scale datasets, its performance might be limited or less effective on very large datasets. It's advisable to consider the dataset size and resource requirements when using ChatGPT.
ChatGPT seems like a valuable tool for data analysts looking to extract and communicate insights from their datasets more effectively.
Hi Faith! You're absolutely right. ChatGPT's ability to extract and communicate insights from datasets makes it a valuable tool for data analysts seeking to enhance their analysis process. It promotes effective communication of findings.
I'm impressed with the potential of ChatGPT for facilitating conversational data exploration. It adds a more human element to the analysis process.
Hi Jade! Absolutely, ChatGPT's conversational nature enhances the data exploration process, adding a more human element. It promotes a deeper understanding and interaction with the data, leading to meaningful insights.
ChatGPT could be a valuable tool for data analysts looking to uncover hidden insights in their datasets. It promotes an exploratory mindset.
Hi Yara! You're absolutely right. ChatGPT's ability to uncover hidden insights promotes a more exploratory mindset among data analysts. It enhances the overall analysis process and drives deeper understanding.
I wonder if ChatGPT can analyze streaming data during EDA.
Hi Willow! While ChatGPT may not be designed for analyzing streaming data in real-time, it can still provide insights and suggestions based on summary statistics or metadata extracted from the streaming data. For real-time analysis, specialized tools or complementary approaches would be more appropriate.
This article has given me a fresh perspective on EDA. ChatGPT's interactive and conversational approach can significantly impact the analysis process.
Hi Hayden! I'm glad the article provided you with a fresh perspective on EDA. ChatGPT's interactive and conversational approach indeed has a significant impact on the analysis process, enriching it with new dynamics and insights.
I'm curious if ChatGPT can assist in exploratory analysis of multi-modal data during EDA.
Hi Lexi! While ChatGPT is not specifically designed for exploratory analysis of multi-modal data, it can still provide insights by analyzing textual or metadata aspects associated with the multi-modal data points. For in-depth analysis, complementary techniques or tools may be necessary.
ChatGPT seems like a valuable tool for exploratory analysis. It can assist analysts in uncovering interesting findings and insights.
Hi Louis! Absolutely, ChatGPT's ability to uncover interesting findings and insights makes it a valuable tool for exploratory analysis. It enriches the analysis process and drives deeper understanding of the data.
I'm curious about the potential use cases of ChatGPT beyond EDA. Can it be applied to other analysis tasks?
Hi Valentina! While ChatGPT is primarily designed for EDA, its conversational approach and ability to uncover insights can be applied to various analysis tasks. It can be used to explore and understand datasets across different domains, making it a versatile tool for data analysis.
ChatGPT seems like it would be a valuable tool for data analysts struggling to explore and understand their datasets. It provides a fresh perspective.
Hi Callum! You're absolutely right. ChatGPT's fresh perspective and interactive nature make it a valuable tool for data analysts seeking assistance in exploring and understanding their datasets. It encourages deeper insights and promotes a more intuitive analysis process.
This article has sparked my interest in exploring ChatGPT for my next EDA project. It seems like a powerful resource.
Hi Skye! I'm glad the article sparked your interest in exploring ChatGPT for your next EDA project. It is indeed a powerful resource that can significantly enhance your analysis and exploration efforts. Happy analyzing!
I wonder if ChatGPT can assist in visual data exploration during EDA. Can it generate visualizations based on data?
Hi Talia! While ChatGPT focuses on textual interactions and insights, it can suggest visualization approaches or provide textual descriptions of potential visualizations based on the data, aiding in visual data exploration during EDA.
ChatGPT could be a valuable tool for analysts looking to extract insights from their datasets in a more interactive and conversational manner.
Hi Thomas! You're absolutely right. ChatGPT's interactive and conversational approach makes it a valuable tool for analysts seeking to extract insights from their datasets in a more intuitive and engaging manner. It enriches the analysis process.
I'm curious if ChatGPT can assist with exploratory analysis of multi-dimensional data during EDA.
Hi Molly! While ChatGPT may not specifically focus on exploratory analysis of multi-dimensional data, it can still provide insights and potential relationships within the data. However, specialized techniques like dimensionality reduction or advanced visualization methods may be more appropriate for comprehensive analysis.
This article has given me a fresh perspective on EDA techniques. ChatGPT's interactive and versatile approach can significantly impact the analysis process.
Hi Henry! I'm glad the article provided you with a fresh perspective on EDA techniques. ChatGPT's interactive and versatile approach indeed has a significant impact on the analysis process, promoting a more engaging and insightful exploration.
ChatGPT can be a valuable tool for analysts looking to unlock the potential of their datasets. It adds a conversational and intuitive layer.
Hi Nicole! Absolutely, ChatGPT unlocks the potential of datasets for analysts by adding a conversational and intuitive layer to the analysis process. It inspires new insights and facilitates communication with the data.
I'm concerned about the computational requirements of ChatGPT for EDA. Can it be resource-intensive?
Hi David! ChatGPT can indeed be resource-intensive, particularly for large-scale EDA or complex analyses. It's recommended to have access to significant computational resources, such as GPUs for optimal performance.
ChatGPT seems like a powerful tool for interactive data exploration. It enables analysts to uncover insights through conversation.
Hi Gabriella! You're absolutely right. ChatGPT's interactive and conversational approach makes it a powerful tool for interactive data exploration. It encourages analysts to engage in conversation with the data, unveiling deeper insights.
I wonder if ChatGPT can assist with exploratory analysis of multi-source data during EDA.
Hi Matthew! While ChatGPT may not specifically focus on exploratory analysis of multi-source data, it can provide insights and potential relationships within the different data sources. Analysts can leverage these insights to explore and understand the interconnectedness of the multi-source data.
This article has given me a new perspective on how to approach EDA. ChatGPT's interactive and conversational nature can help analysts uncover new insights.
Hi Claudia! I'm glad the article provided you with a new perspective on approaching EDA. ChatGPT's interactive and conversational nature significantly enhances the analysis process and empowers analysts to uncover fresh insights.
I'm concerned about the model's responsiveness with long text sequences during EDA. Can ChatGPT handle lengthy inputs effectively?
Hi John! ChatGPT's responsiveness with long text sequences can be limited due to its maximum token limit. If working with excessively long inputs, it's advisable to truncate or summarize the text to ensure optimal performance and effectiveness.
ChatGPT seems like it would be a valuable tool for data analysts looking to explore and understand their datasets more interactively.
Hi Rachel! Absolutely, ChatGPT's interactive nature makes it a valuable tool for data analysts seeking to explore and understand their datasets more interactively. It adds a conversational layer to the analysis process, fostering engagement and deeper insights.
I'm curious about the potential downsides of using ChatGPT for EDA. Can you elaborate on any limitations or risks?
Hi Ellie! ChatGPT's limitations include the potential for generating plausible but inaccurate responses, biases in its outputs, and a dependency on coherent context. It's important to critically evaluate the results, mitigate biases, and be mindful of potential limitations when using ChatGPT for EDA.
ChatGPT seems like a valuable tool for analysts looking to explore their datasets interactively. It helps in discovering subtle patterns and insights.
Hi Tyler! You're absolutely right. ChatGPT's interactive approach aids analysts in exploring their datasets interactively, uncovering subtle patterns and insights that may not be immediately apparent. It enhances the analysis process.
This article has opened my eyes to the potential of ChatGPT for enhancing the EDA process. It seems like a powerful tool for data exploration.
Hi Alexa! I'm glad the article opened your eyes to the potential of ChatGPT for enhancing the EDA process. It is indeed a powerful tool that can augment data exploration and lead to valuable insights. Happy exploring!
Thank you all for joining this discussion on my article, 'Revolutionizing Technology: Harnessing ChatGPT for Enhanced Exploratory Data Analysis (EDA)'. I'm excited to hear your thoughts!
Great article, Dorothy! ChatGPT seems like a game-changer for EDA. Can you share any specific examples of how it has enhanced the analysis process?
Thanks, Maxwell! Absolutely, ChatGPT has proven to be highly useful in EDA. For instance, it can quickly generate summaries of large datasets, provide insights into data patterns and outliers, and even recommend valuable visualizations or specific data transformations based on the given context.
I'm curious about the limitations of ChatGPT in the context of EDA. Are there any scenarios where it struggles to provide accurate or useful analysis recommendations?
That's a great question, Samantha. While ChatGPT is powerful, it does have limitations. It may struggle with datasets that have complex or ambiguous relationships between variables. Additionally, if the dataset is too large or if the analysis requires domain-specific knowledge, ChatGPT may not be as accurate or useful in its recommendations.
I've used ChatGPT for EDA recently, and it has been a huge time-saver. It can quickly analyze multiple dimensions simultaneously and offer valuable suggestions. Highly recommended!
Interesting article, Dorothy! How does ChatGPT handle missing or incomplete data during the EDA process?
Thank you, Lauren! ChatGPT can handle missing or incomplete data to some extent. It can provide imputation suggestions based on the available information and even suggest the most appropriate imputation method when given the specific context.
I'm concerned about the potential biases in the analysis provided by ChatGPT. How is it trained to ensure unbiased recommendations in EDA?
Valid point, Nathan. ChatGPT is trained using diverse datasets to minimize biases. Additionally, pre-training and fine-tuning techniques are employed to make the model more reliable and unbiased. However, continuous improvements in training data and techniques are ongoing to address any potential biases further.
This sounds like an exciting development! Could you share any real-world EDA use cases where ChatGPT has significantly improved the analysis outcomes?
Certainly, Olivia! ChatGPT has been successfully applied in various industries. For example, in finance, it has helped identify hidden correlations and potential risks. In healthcare, it has assisted in analyzing patient data and identifying patterns for early disease detection. These are just a few examples where ChatGPT has enhanced exploratory data analysis significantly!
I'm wondering about the computational resources required to use ChatGPT effectively for EDA. Are there any hardware or software requirements to consider?
Great question, Emma. ChatGPT can run on standard hardware, but for large-scale analysis, more powerful systems with GPUs are recommended. As for software, OpenAI provides user-friendly libraries and APIs to facilitate the integration of ChatGPT into various data analysis workflows.
I'm intrigued by this approach! Are there any potential privacy concerns when using ChatGPT for EDA, especially with sensitive or confidential datasets?
Absolutely, Sophia. Privacy and security are essential considerations. ChatGPT could be used on-premises or with strictly controlled cloud environments to minimize data exposure. It's crucial to adhere to best practices and ensure the proper anonymization of sensitive or confidential datasets when performing EDA with ChatGPT.
It appears that ChatGPT can be a valuable addition to the analyst's toolkit. In what ways do you see future advancements in language models further enhancing EDA?
You're absolutely right, Victoria. As language models evolve, we expect them to gain a deeper understanding of complex data relationships and offer more sophisticated insights. Additionally, integrating domain-specific knowledge and refining the training process can further enhance the contextual relevance and accuracy of language models like ChatGPT in the realm of EDA.
What are the key differences between using ChatGPT and traditional analytics tools for EDA? Are there any specific scenarios where one outperforms the other?
Great question, Isaac. Traditional analytics tools provide structured interfaces and predefined functionalities, making them well-suited for standard analysis tasks. However, ChatGPT offers a more conversational and flexible approach, allowing for ad hoc analysis and exploring complex data scenarios. Therefore, traditional tools may be preferable for routine tasks, while ChatGPT shines when facing unique or exploratory challenges in EDA.
I'm wondering if ChatGPT also supports visualization suggestions during the EDA process. Can it recommend appropriate charts or graphs based on the data being explored?
Absolutely, Grace! ChatGPT can suggest suitable visualizations based on the data characteristics and patterns it identifies. Whether it's recommending scatter plots for exploring relationships or bar charts for categorical data, ChatGPT can provide valuable guidance on the most appropriate visual representations during the EDA process.
This sounds like an exciting tool for EDA! Can ChatGPT also assist in anomaly detection, and if so, how does it go about it?
Indeed, Emily! ChatGPT can help with anomaly detection. By analyzing the data patterns across different variables, it can identify unusual or unexpected observations in the dataset. It may suggest statistical techniques or recommend exploring specific features further to detect and understand anomalies during the EDA process.
I'm concerned about the interpretability of ChatGPT's recommendations. How can we trust the suggestions it provides in EDA?
Valid point, William. The transparency and interpretability of ChatGPT's recommendations are indeed important. While the model's internal decision-making process is complex, it's crucial to use it as an assistant rather than fully relying on it. Combining the model's insights with domain expertise and critical thinking helps ensure more reliable and trusted analysis outcomes.
How does ChatGPT handle time-series data during exploratory data analysis?
Great question, Ethan. ChatGPT can handle time-series data effectively. It can detect trends, seasonality, and other time-based patterns, enabling it to provide appropriate analysis recommendations specific to time-series datasets. When exploring time-dependent data, ChatGPT can assist in identifying important temporal relationships and patterns.
How can one get started with using ChatGPT for EDA? Are there any learning resources or tutorials available?
Absolutely, Aiden. OpenAI provides detailed documentation and tutorials on how to integrate ChatGPT into your exploratory data analysis workflows. They offer code examples, best practices, and guides to help users get started effectively. Additionally, the OpenAI community forum is a great place to seek guidance and connect with other users who have experience in using ChatGPT for EDA.
Do you foresee any challenges in adopting ChatGPT for EDA within organizations, particularly when it comes to data governance and security?
Definitely, Hannah. Organizations need to ensure proper data governance and security practices when implementing ChatGPT for EDA. This includes defining appropriate access controls, data anonymization protocols, and regularly auditing the system's usage to mitigate potential risks. Collaborating with stakeholders, IT teams, and data governance experts is crucial to address any challenges and ensure a secure and compliant implementation.
I'm impressed by the potential of ChatGPT in EDA! Are there any plans to make it even more customizable for specific analysis needs?
Absolutely, Liam! OpenAI recognizes the importance of customization in specific analysis scenarios. They are actively working on providing more flexibility and customization options for ChatGPT, enabling users to adapt it to their unique EDA requirements effectively. As the technology progresses, we can expect more tailored capabilities to enhance the model's usability and relevance.
Do you recommend using ChatGPT as the sole tool for EDA, or should it be used in conjunction with other established techniques and tools?
Good question, Evelyn. ChatGPT should be seen as a complementary tool rather than a standalone solution for EDA. While it brings valuable insights and recommendations, it's important to combine it with other established techniques and tools to ensure a comprehensive analysis approach. By leveraging ChatGPT alongside traditional methods, analysts can gain a deeper understanding and make more informed decisions during exploratory data analysis.
What are the computational costs associated with using ChatGPT for EDA? Are there any limitations when it comes to processing large datasets?
Good question, James. While ChatGPT is powerful, processing large datasets can be computationally expensive, especially when running on less powerful hardware. It's recommended to sample or preprocess the data to manageable sizes, focusing on the most relevant subsets. As technology progresses, computational costs may decrease, making it easier to handle larger datasets with ChatGPT.
I'm curious about the training process for ChatGPT in the context of EDA. How does it learn to provide accurate analysis recommendations?
Good question, Madison. ChatGPT's training process involves exposure to a large corpus of text data, including examples of EDA-related tasks. By learning patterns and relationships from this diverse dataset, the model gains the capability to generate accurate analysis recommendations based on the given context. Fine-tuning the model on specific EDA tasks further refines its suggestions and enhances its accuracy.
I'm excited about the possibilities of ChatGPT in EDA! Are there plans to integrate it with popular data analysis platforms like Python notebooks or SQL-based tools?
Absolutely, Aaron! OpenAI recognizes the importance of integration with existing data analysis platforms. While there are no specific details at the moment, they are actively exploring possibilities to make ChatGPT seamlessly accessible within popular tools like Python notebooks and SQL-based environments. This integration would further enhance the adoption and usability of ChatGPT in EDA workflows.
How does ChatGPT handle data preprocessing requirements for EDA? Does it provide any guidance on handling missing values or outlier detection, for example?
Great question, Sophie. ChatGPT can indeed provide guidance on data preprocessing requirements for EDA. It can suggest methods for handling missing values, recommend outlier detection techniques, and even propose data transformations to improve the analysis outcomes. By incorporating feedback on data quality and preprocessing steps, ChatGPT becomes a valuable assistant in the data preparation stage of EDA.
Has ChatGPT been tested on a wide range of datasets, including different types and sizes, to ensure its applicability in various EDA scenarios?
Absolutely, Gabriel. ChatGPT has undergone extensive testing on diverse datasets to validate its applicability across various EDA scenarios. The model has been trained on a wide range of data types and sizes, enabling it to offer relevant insights and recommendations for different analysis needs. Continuous testing and evaluation ensure its reliability and effectiveness in diverse real-world datasets.
I'm concerned about potential biases in the analysis recommendations provided by ChatGPT. How does the model handle various sources of bias to ensure fairness in EDA results?
That's an important concern, Ella. Efforts are made during training and development to minimize biases in ChatGPT's analysis recommendations. However, it's crucial to review and validate the suggestions critically. Monitoring and addressing biases in AI models like ChatGPT are continuous processes, and improvements are made over time to enhance the fairness and reliability of its recommendations in EDA.
Are there any case studies or success stories demonstrating the benefits of using ChatGPT for EDA?
Certainly, Daniel! OpenAI has published case studies and success stories highlighting the benefits of using ChatGPT for EDA across various industries. These real-world examples showcase how ChatGPT has expedited analysis processes, identified critical insights, and improved decision-making. Exploring these case studies can provide valuable insights into the capabilities and advantages of using ChatGPT for exploratory data analysis.