Revolutionizing Technology OLAP with ChatGPT: Enhancing Analytics and Decision-Making
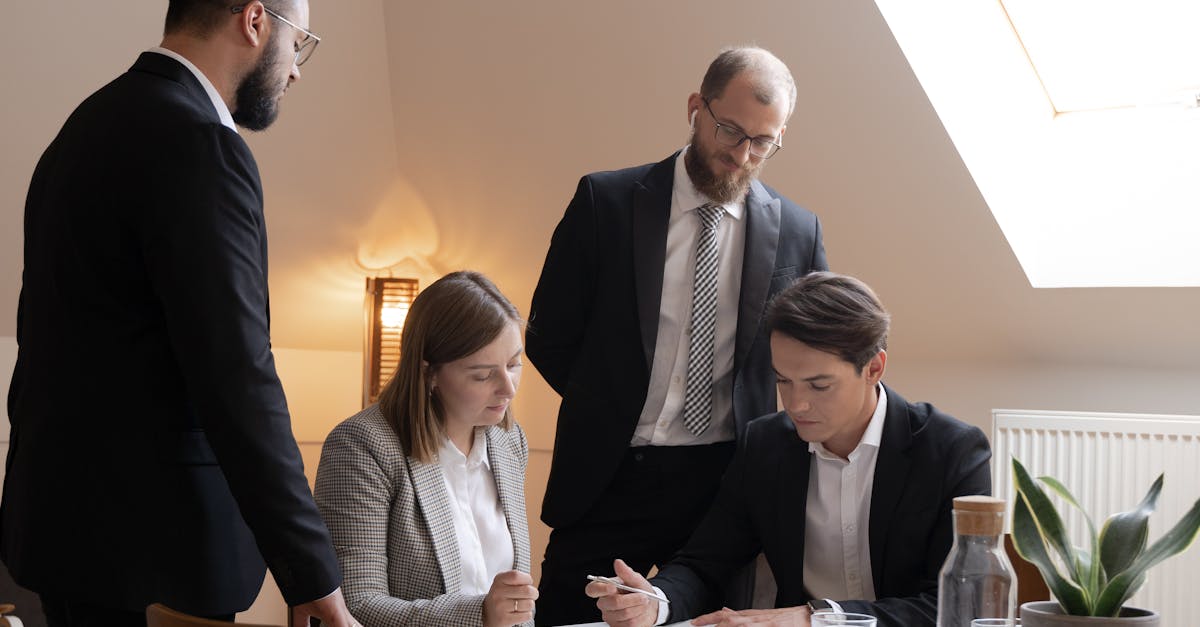
OLAP (Online Analytical Processing) is a technology used in the field of data analysis. It allows users to extract multidimensional data and perform complex analytical calculations on large datasets. While OLAP provides powerful capabilities for data analysis, it can often be quite complex and challenging to understand.
This is where ChatGPT-4 comes in. ChatGPT-4 is an advanced language model developed by OpenAI. It is designed to understand and generate human-like text responses. With its contextual understanding and ability to generate insightful responses, ChatGPT-4 can greatly simplify the complexity of data analysis in OLAP.
One of the key advantages of using ChatGPT-4 in OLAP is its ability to provide understandable insights. Traditional OLAP tools often present data in a raw format, making it difficult for users to grasp the meaning behind the numbers. ChatGPT-4, on the other hand, can interpret and explain the data in a way that humans can easily comprehend.
For example, if you're analyzing sales data in OLAP and want to understand the performance of different product categories, you can simply ask ChatGPT-4 specific questions like "Which product category had the highest sales last month?" or "What is the trend of sales for a particular product category over time?". The model will provide clear and concise responses, enabling you to quickly gain valuable insights without having to dive into complex data analysis workflows.
Additionally, ChatGPT-4 can assist in generating custom reports and visualizations based on OLAP data. You can ask the model to create charts, graphs, or even summaries of specific metrics. This not only saves time but also allows you to communicate your findings more effectively to stakeholders or team members.
Furthermore, ChatGPT-4 can offer suggestions on how to optimize OLAP queries and improve performance. It can analyze your query patterns and provide recommendations to enhance the efficiency of your data retrieval and analysis processes. This is particularly beneficial when dealing with large volumes of data in OLAP systems.
It's important to note that while ChatGPT-4 can simplify data analysis in OLAP, it does not replace the need for domain expertise and a solid understanding of data analysis principles. It should be used as a complementary tool for assisting in the exploration and interpretation of data.
In conclusion, ChatGPT-4 is a powerful resource for simplifying the complexity of data analysis in OLAP. With its ability to provide understandable insights, generate reports, offer query optimization suggestions, and more, it helps analysts and data scientists make sense of their data more efficiently. By leveraging this technology, you can enhance your OLAP experience and gain deeper insights from your data.
Comments:
This article on revolutionizing technology OLAP with ChatGPT is quite interesting. The use of chatbots in analytics and decision-making can definitely enhance the overall efficiency of the process.
I agree, Sarah. ChatGPT has the potential to transform the way we analyze and make decisions based on data. It can provide real-time insights and assist in complex data interpretations.
Thank you, Sarah and Eric, for your comments. It's great to see your enthusiasm for the topic. Indeed, ChatGPT has the capability to improve analytics and decision-making by providing a conversational interface and assisting with data exploration. Feel free to share any specific experiences or thoughts on the matter!
I've been using ChatGPT for a while now, and it has been a game-changer in terms of data analysis. The natural language processing capabilities make it so much easier to interact with and understand complex datasets.
That's interesting, Sophia. Could you provide some examples of how ChatGPT has helped you in your data analysis tasks?
Sure, Maria! One example is when I needed to analyze customer feedback. Instead of manually sorting through thousands of survey responses, I could simply have a conversation with ChatGPT, and it would help me extract key insights based on the given criteria. It saved me so much time!
ChatGPT seems promising. However, have you encountered any limitations or challenges while handling sensitive or confidential data?
That's a valid concern, David. While ChatGPT has been helpful in my analyses, I do exercise caution when dealing with sensitive information. It's crucial to ensure proper data anonymization and security measures to protect confidentiality.
Thank you, Sophia and David, for sharing your thoughts. It's essential to maintain data privacy and security, especially when using conversational AI in sensitive domains. Let's continue the discussion and explore more perspectives!
I think the application of ChatGPT in OLAP can be a game-changer in organizations. It can empower decision-makers with instant insights and facilitate data-driven decision-making at all levels.
Absolutely, Laura! With ChatGPT, decision-makers can swiftly navigate through complex data, ask follow-up questions, and receive contextual explanations. This can significantly enhance the decision-making process.
Well said, Laura and Christopher! The integration of conversational AI like ChatGPT with OLAP can unlock new possibilities in data-driven decision-making. Are there any specific challenges or use cases you'd like to discuss?
I'm curious to know more about the training process of ChatGPT for OLAP. How do you ensure it understands the context and domain-specific language?
Great question, Emily! The training process involves fine-tuning the model using domain-specific datasets and providing it with context-relevant prompts. Additionally, continuous feedback loops help improve the performance and language understanding.
Thank you for the clarification, Steve. It's fascinating to see the continuous advancements in AI, enabling us to leverage such powerful tools for analytics and decision-making.
You're welcome, Emily. Indeed, the progress in AI applications like ChatGPT opens up new avenues for innovation. Let's stay connected and explore more developments in this field!
I'm wondering if there are any specific industries or sectors that can benefit the most from incorporating ChatGPT in their analytics processes?
That's a great question, Michael. I believe sectors like finance, healthcare, and customer service, where quick insights and decision-making are paramount, can benefit the most from using ChatGPT in analytics.
One potential challenge with ChatGPT could be handling ambiguous queries or requests. How well does it handle those situations?
Good point, John. In my experience, ChatGPT typically handles ambiguous queries quite well. However, context plays a crucial role, and refining the prompts or introducing additional constraints can help improve the accuracy of responses.
Thank you, Michael, Sarah, John, and Eric, for your valuable contributions to the discussion. It's exciting to see the diverse perspectives and thoughtful questions. Keep them coming!
The potential of ChatGPT in enhancing analytics and decision-making is immense. It can democratize data access and empower non-technical users to derive insights independently.
Absolutely, Olivia! By providing a user-friendly conversational interface, ChatGPT can bridge the gap between technical and non-technical users and facilitate a more inclusive data-driven decision-making culture.
Well said, Olivia and Sarah! The democratization of data-driven insights is an important aspect, and ChatGPT can play a significant role in enabling a broader audience to make informed decisions based on data.
As much as I see the potential, I also worry about over-reliance on AI-driven analytics. It's crucial to strike a balance between human expertise and AI assistance.
I agree with you, Andrew. While AI can significantly enhance our capabilities, it should always be seen as a tool to augment decision-making, not replace human expertise. Humans bring critical thinking and domain knowledge to the table.
You bring up an important point, Andrew. Human expertise should indeed guide the decisions, with AI acting as a support system. It's essential to find the right balance and not solely rely on AI-driven analytics.
ChatGPT seems like a promising tool for data analysis. Are there any known limitations or areas where it may struggle?
Indeed, Natalie. While ChatGPT has shown impressive capabilities, it may still struggle with nuanced or highly complex queries. It's essential to frame questions appropriately and manage expectations regarding its limitations.
Thank you, Natalie and Eric, for highlighting this aspect. Managing expectations and understanding the boundaries of ChatGPT's capabilities is crucial for an effective integration with analytics workflows.
I'm curious about the scalability of using ChatGPT in large-scale analytics processes. Can it handle heavy workloads without performance deterioration?
Scalability is a valid concern, Jennifer. While ChatGPT is powerful, it's essential to consider resource allocation, infrastructure, and optimization to ensure optimal performance during heavy workloads.
Great question, Jennifer. David makes an important point. Ensuring scalability and performance under heavy workloads requires careful system architecture and resource management in combination with ChatGPT's capabilities.
I can see the potential of using ChatGPT in immersive analytics experiences. Combining real-time conversation with data visualizations can provide a highly interactive and engaging analytics environment.
Absolutely, Oliver! Immersive analytics can greatly benefit from the integration of conversational AI like ChatGPT. It can make the exploration and interpretation of complex data more intuitive and engaging.
Well said, Oliver and Sarah. Immersive analytics offers a unique approach to data exploration, and the real-time conversational integration of ChatGPT can further enhance the interactive and engaging nature of the experience.
I'd love to hear more about the practical implementation of ChatGPT in OLAP systems. Are there any considerations or prerequisites for successfully incorporating it?
Practical implementation requires a careful understanding of the existing OLAP system architecture, APIs, and data integration. Additionally, user training, feedback loops, and continuous improvement are crucial to maximize the gains from ChatGPT in OLAP.
Thank you, Ryan and Sophia, for bringing up this aspect. Successful implementation of ChatGPT in OLAP systems indeed requires consideration of various technical and organizational factors to maximize its potential benefits.
What are the potential risks in using ChatGPT for analytics? Are there any cases where it might provide misleading or incorrect interpretations?
Valid concern, Liam. While ChatGPT strives to provide accurate insights, there might be scenarios where it can provide misleading interpretations due to inherent bias in training data or limitations in comprehending context accurately.
You raise an important point, Liam. While ChatGPT aims to be reliable, it's crucial to critically evaluate and verify the interpretations it provides to ensure accuracy and avoid potential misleading conclusions.
The article mentions 'revolutionizing' OLAP. To what extent can ChatGPT truly revolutionize the domain?
Great question, Emma. ChatGPT has the potential to revolutionize OLAP by making the exploration and analysis of data more accessible, intuitive, and interactive. It can enhance the decision-making process and enable quicker insights generation.
I agree, Emma and Sarah. The integration of ChatGPT can bring significant advancements to OLAP, transforming it into a more dynamic, conversational, and user-centered experience. It has the potential to revolutionize the way we interact with and derive insights from data.
Could the integration of ChatGPT in OLAP potentially disrupt traditional roles in analytics teams? How can we ensure a smooth transition and collaboration?
Valid concern, Michael. Rather than disrupting traditional roles, integrating ChatGPT in OLAP can augment the capabilities of analytics teams. Proper change management, training, and fostering collaborative environments can help ensure a smooth transition and effective collaboration.
You make an excellent point, Michael. The focus should be on leveraging ChatGPT to enhance collaboration and complement the expertise of analytics teams, leading to more efficient and impactful outcomes.
As with any AI-driven technology, ethical considerations are crucial. How can we address potential biases or ethical challenges in using ChatGPT for analytics?
Ethical considerations are indeed paramount, Amelia. Ensuring diverse and unbiased training data, implementing transparency mechanisms, and regular audits can help address potential biases and ethical challenges associated with AI-driven analytics.
Well said, Amelia and Jennifer. Ethical frameworks and responsible practices, coupled with robust monitoring mechanisms, should be established to minimize biases and ensure the ethical deployment of ChatGPT in analytics workflows.
I'd like to know more about the potential impact of ChatGPT in data storytelling. Can it assist in creating compelling narratives based on complex datasets?
Absolutely, Oliver. ChatGPT can play a significant role in data storytelling by providing interactive conversations and contextual explanations. It can assist in creating compelling narratives that make complex data more approachable and impactful.
You bring up an interesting aspect, Oliver. ChatGPT's conversational nature and ability to explain complex data provide a powerful tool to enhance data storytelling, making it more engaging and easier to convey insights effectively.
While ChatGPT seems promising, what are some potential security risks that organizations should consider when implementing it in their analytics processes?
Security risks should always be a priority, Laura. Organizations must ensure secure data access, prevent data leakage, and implement robust access controls to protect sensitive information when using ChatGPT in their analytics workflows.
Excellent point, Laura. Prioritizing security measures, including encryption, user authentication, and secure infrastructure, is essential to mitigate potential risks when integrating ChatGPT into analytics processes.
I have a question regarding data privacy. How can organizations ensure the privacy of user data when leveraging ChatGPT for analytics?
Data privacy is a critical aspect, Hannah. Organizations should establish robust data anonymization procedures, obtain user consent, and comply with relevant privacy regulations to protect user data while utilizing ChatGPT for analytics.
Thank you for the question, Hannah. Data privacy is paramount, and organizations must adhere to stringent privacy policies, implement anonymization practices, and ensure compliance to build trust and maintain user data privacy when incorporating ChatGPT in analytics processes.
I'm curious about the potential future developments of ChatGPT in OLAP. What can we expect in terms of advancements or new features?
Great question, Anna! While it's difficult to predict specific future developments, we can expect continuous advancements in language models like ChatGPT, fine-tuning for better domain-specific understanding, and potential integration with other emerging technologies to further enhance OLAP capabilities.
You bring up an interesting point, Anna. The future of ChatGPT in OLAP holds exciting possibilities, including improved language understanding, expanded integration with visualization tools, and more sophisticated conversational capabilities.
In terms of implementation challenges, what are some key considerations when integrating ChatGPT with existing OLAP systems?
You've pointed out important considerations, Michael. The successful integration of ChatGPT with existing OLAP systems requires technical coordination, comprehensive testing, and iterative improvements to achieve seamless compatibility and optimal performance.
Integration challenges can include aligning data schema, ensuring API compatibility, training the model on relevant data, and establishing a feedback loop for continuous improvement. Proper coordination between data engineering and analytics teams is crucial for a successful integration.
Have there been any instances where ChatGPT provided unconventional or unexpected insights during data analysis?
Indeed, Oliver. ChatGPT can occasionally surprise us with unconventional insights or prompt us to consider alternative perspectives. It can challenge conventional thinking and contribute to more creative and out-of-the-box analytics.
You make an interesting point, Oliver. ChatGPT's ability to sometimes offer unconventional insights can be a valuable asset, encouraging fresh perspectives and uncovering hidden patterns in data during analysis.
What are some potential risks associated with the integration of ChatGPT in critical decision-making processes?
Critical decision-making requires careful evaluation, Liam. Risks can include over-reliance on AI-driven insights, potential biases in model training, and the need to ensure human oversight and domain expertise to prevent incorrect or misleading conclusions.
You raise a crucial concern, Liam. The integration of ChatGPT must involve a balanced approach, combining AI-driven insights with human expertise to mitigate risks and ensure critical decision-making processes are evaluated thoroughly and responsibly.
I'm impressed with the potential of ChatGPT in data analysis. Are there any specific industry use cases or success stories we can learn from?
Definitely, Andrew! In healthcare, ChatGPT has been used to extract insights from medical records for diagnosis support. In finance, it has assisted with fraud detection and anomaly analysis. These are just a few examples of successful industry use cases.
Thank you, Andrew and Sarah, for bringing up the practical implications. Industry use cases show the versatility of ChatGPT in various domains, and studying successful deployments can provide valuable insights for further adoption and optimization.
Can ChatGPT be used to automate common analytical tasks, such as report generation or data visualization?
Indeed, Natalie. ChatGPT has the potential to automate certain analytical tasks by generating reports based on requested insights or assisting in data visualization interpretation. It can save time and streamline routine analytics processes.
You've highlighted a significant aspect, Natalie. Automation of common analytical tasks through ChatGPT can enhance efficiency and enable analysts to focus on more complex and strategic aspects of their work.
Considering the constantly evolving nature of analytics, how can ChatGPT adapt to stay up to date with the latest data analysis techniques and methodologies?
Staying up to date is essential, Emily. ChatGPT can adapt through continuous model improvements, fine-tuning on relevant datasets, regular updates to incorporate new methodologies, and collaboration with data scientists and domain experts to ensure it remains effective and relevant.
You make an excellent point, Emily. Adapting and evolving ChatGPT to incorporate the latest analytical techniques and methodologies is a dynamic process that requires continuous development, iterative feedback, and collaboration with the data analysis community to ensure its ongoing effectiveness.
I'm curious if ChatGPT can be trained to understand specific industry jargon or terminology when used in sector-specific analytics?
Absolutely, Amelia. ChatGPT's training can be fine-tuned with sector-specific datasets, including industry jargon and terminologies, to enhance its understanding and contextual relevance in sector-specific analytics.
You bring up a valuable point, Amelia. Fine-tuning ChatGPT with sector-specific terminology enables a more precise understanding and better contextual relevance in different industries, enhancing its overall effectiveness in sector-specific analytics.
Are there any ongoing research initiatives to further advance ChatGPT's capabilities in the realm of analytics and decision-making?
Ongoing research is actively exploring ways to improve ChatGPT's capabilities, Olivia. Efforts include enhancing the model's explainability, reducing biases, scaling to larger datasets, and addressing specific challenges in analytics domains.
You raise an important aspect, Olivia. Continuous research is essential to further advance ChatGPT's capabilities, making it more robust, reliable, and adaptable in addressing the challenges and requirements of analytics and decision-making domains.
Is ChatGPT suitable for small businesses or startups? Are there any limitations or considerations for organizations with limited resources?
ChatGPT can be beneficial for small businesses and startups, Anna. However, resource limitations should be considered, including infrastructure, training data availability, and costs associated with implementation. It's essential to evaluate the trade-offs based on specific business needs.
That's a great question, Anna. While ChatGPT can offer valuable insights for small businesses and startups, it's crucial to assess resource constraints and evaluate the cost-benefit ratio to ensure it aligns with the organization's goals and limitations.
I have concerns regarding privacy while using ChatGPT for analytics. How can we be sure that sensitive data is handled securely?
Privacy concerns should always be of utmost importance, Noah. Organizations must ensure robust security measures, including data encryption, stringent access controls, and secure infrastructure when leveraging ChatGPT for analytics to protect sensitive information properly.
You bring up a crucial concern, Noah. Organizations must prioritize data privacy and adopt stringent security measures to ensure sensitive data is handled securely throughout the analytics process when using ChatGPT.
How can organizations effectively measure the impact and success of integrating ChatGPT in their analytics workflows?
Effective measurement requires defining appropriate success metrics, Oliver. Key performance indicators such as time saved, insights generated, user feedback, and impact on decision-making quality can help assess the impact and success of ChatGPT integration in analytics workflows.
You've highlighted an important aspect, Oliver. By establishing relevant success metrics and continuously assessing user feedback, organizations can effectively measure the impact of ChatGPT integration in analytics workflows and gauge its success in achieving the desired outcomes.
Are there any concerns or limitations related to the explainability of insights derived from ChatGPT that organizations should be cautious about?
Explainability is indeed a concern, Michael. ChatGPT's insights should be critically examined and validated, especially in high-stakes scenarios. Organizations should establish procedures for explaining and validating the reasoning behind insights to ensure transparency and accuracy.
You raise an important point, Michael. Ensuring explainability and interpretability of insights derived from ChatGPT is crucial to avoid potential risks. Organizations should establish validation processes and transparency mechanisms to address this concern when leveraging the technology in analytics workflows.
Thank you all for your valuable contributions to this discussion on revolutionizing technology OLAP with ChatGPT! It has been enlightening to hear your perspectives, experiences, and questions. Feel free to reach out if you have any further thoughts or insights to share in the future!
Thank you all for your interest in my article! I'm glad to see the discussion starting.
Great article, Steve! ChatGPT seems like a game-changer for OLAP.
I agree with Samantha, ChatGPT has the potential to revolutionize analytics and decision-making.
The concept is interesting, but how do you ensure data accuracy with a language model like ChatGPT?
Emily, that's a valid concern. While ChatGPT is a powerful tool, data validation and verification are crucial steps in ensuring accuracy.
I wonder if ChatGPT can handle complex OLAP queries and provide meaningful insights.
Alex, ChatGPT can indeed handle complex queries and provide valuable insights. Its adaptability and natural language understanding make it an excellent tool for analytics.
What about data privacy and security? How can we trust ChatGPT with sensitive information?
Sarah, data privacy and security are of utmost importance. ChatGPT adheres to strict privacy protocols, and all data is encrypted and handled securely.
I'm curious to know how ChatGPT compares to traditional OLAP tools in terms of performance and efficiency.
Mark, while ChatGPT provides a unique approach to analytics, it may not match the performance and efficiency of specialized OLAP tools. However, its versatility and interactivity make it a valuable addition in certain use cases.
Can ChatGPT be integrated with existing OLAP systems, or is it a standalone solution?
Rachel, ChatGPT can be integrated with existing OLAP systems, enhancing their capabilities. It can act as a complementary tool for analysis and decision-making.
Is ChatGPT accessible to non-technical users, or does it require advanced programming skills?
Daniel, ChatGPT is designed to be user-friendly and accessible to non-technical users. Its natural language interface eliminates the need for advanced programming skills.
I'm concerned about bias in AI models. How does ChatGPT tackle this issue?
Olivia, addressing bias is a critical aspect. OpenAI is actively working to reduce both glaring and subtle biases in ChatGPT through research and continued development.
How does ChatGPT handle data from various sources? Can it integrate different data types effectively?
Gregory, ChatGPT can handle data from various sources, including structured and unstructured data. Its versatility allows it to integrate different data types effectively.
Are there any limitations to ChatGPT that we should be aware of before considering it for OLAP?
Natalie, while ChatGPT is a powerful tool, it's important to note that it may not be suitable for all OLAP scenarios. Assessing its fit with specific use cases and requirements is crucial.
ChatGPT sounds intriguing! Can you provide any real-world examples of how it has been successfully implemented in OLAP projects?
James, certainly! ChatGPT has been successfully implemented in finance companies for ad hoc analysis, enabling business users to gain insights more quickly and efficiently.
Do you have any recommendations on how to get started with ChatGPT for OLAP projects?
Grace, to get started, it's helpful to familiarize yourself with the ChatGPT documentation and API. Exploring sample use cases and experimenting with the tool can provide valuable insights.
What kind of computational resources are required to run ChatGPT effectively?
Lucas, running ChatGPT effectively requires substantial computational resources, including GPUs or TPUs. It's important to consider these requirements when planning to use the tool.
ChatGPT sounds like a promising technology. I'm excited to see how it evolves in the field of OLAP.
Melissa, I'm thrilled about its potential too. The development of ChatGPT and its application in OLAP is an exciting area to watch.
Are there any known limitations or risks associated with using ChatGPT as an analytics tool?
Tom, some limitations and risks include potential bias in responses and the need for careful data validation. It's essential to continuously monitor and evaluate the tool's performance.
How does ChatGPT handle scalability when dealing with large datasets and concurrent users?
Michelle, handling large datasets and concurrent users can be challenging for ChatGPT. Ensuring proper resource allocation and parallel processing is necessary to maintain scalability.
What is the typical response time of ChatGPT when handling complex OLAP queries?
Peter, response time may vary based on query complexity and system resources. While ChatGPT is designed for interactive use, it might not match the instant responsiveness of traditional OLAP tools.
Can you share insights on the cost implications of using ChatGPT for OLAP?
Ashley, the cost implications depend on factors like usage frequency and required computational resources. It's recommended to evaluate the cost-benefit based on specific project needs.
Are there any industry-specific use cases where ChatGPT has shown exceptional value in OLAP?
Brandon, ChatGPT has shown exceptional value in the finance industry, enabling dynamic exploration and analysis of financial data for better decision-making.
Can ChatGPT handle real-time data for analytics, or does it work only on static datasets?
Maria, ChatGPT has the capability to handle real-time data for analytics, making it suitable for scenarios where dynamic insights are required.
Do you anticipate any ethical challenges in using ChatGPT for decision-making in OLAP?
Jason, ethical challenges may arise when relying solely on AI models like ChatGPT for decision-making. It's important to strike a balance and incorporate human judgment and validation into the process.
How customizable is ChatGPT? Can it be tailored to specific business needs?
Laura, ChatGPT offers customization options, allowing it to be adapted to specific business needs. Fine-tuning and domain-specific training can enhance its performance and relevance.
Can ChatGPT facilitate collaborative decision-making by enabling multiple users to interact simultaneously?
Adam, enabling simultaneous user interaction and facilitating collaborative decision-making are indeed areas where ChatGPT can make a positive impact.
What kind of user interface does ChatGPT have? Is it intuitive and user-friendly?
Linda, ChatGPT interfaces can be designed to be intuitive and user-friendly. However, the implementation may vary depending on the specific platform or application.
Overall, it seems like ChatGPT has the potential to enhance analytics and decision-making. Exciting times ahead in the OLAP domain!
Indeed, Eric! The potential of ChatGPT in OLAP is promising, and I look forward to seeing how it unfolds.