Revolutionizing Technology's AFM with Gemini: Enhancing Automation and Efficiency
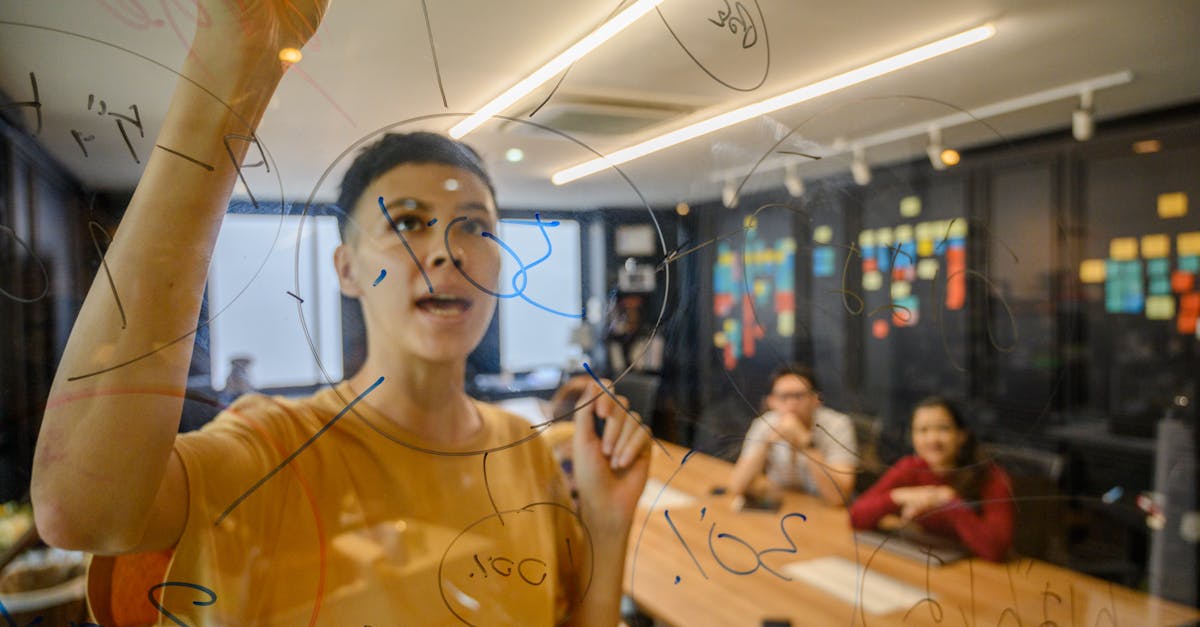
Technology has always been at the forefront of innovation, pushing boundaries, and transforming various industries. In recent years, the advent of artificial intelligence (AI) has further accelerated this transformative process by introducing automation and efficiency-enhancing solutions. One such revolutionary technology that is changing the game for the technology industry's atomic force microscopy (AFM) is Gemini.
AFM, an essential tool in nanotechnology, enables scientists and researchers to study and manipulate materials at the atomic and molecular level. Traditionally, operating AFM required significant expertise, manual intervention, and meticulous measurements. However, with the integration of Gemini, AFM's capabilities have been revolutionized.
The Power of Gemini in AFM Automation
Gemini, an AI language model developed by Google, utilizes deep learning algorithms to understand and generate human-like text responses. This powerful language model has been trained on a massive dataset, allowing it to comprehend complex scientific concepts and offer insightful guidance in real-time.
Gemini's integration with AFM technology enables automation at a level never seen before. Previously, scientists would manually set up AFM experiments, adjust parameters, and interpret results. However, with Gemini's assistance, these processes can be streamlined, saving valuable time and effort.
Enhancing Efficiency with Gemini
With Gemini, researchers can interact with the AI model using natural language queries and instructions. This interaction facilitates streamlined data analysis, instrument calibration, and experiment optimization. By leveraging the power of AI, researchers can now achieve results faster and make more informed decisions based on the AI model's intelligent suggestions.
The integration of Gemini with AFM technology also enhances efficiency by reducing the learning curve for new users. Traditionally, becoming proficient in operating AFM required extensive training and hands-on experience. However, with Gemini's intuitive guidance, new users can quickly grasp the fundamentals and start conducting AFM experiments with confidence.
Promoting Research Collaboration
Another significant advantage of Gemini's integration with AFM is the promotion of research collaboration. By utilizing a shared AI model, scientists worldwide can interact and share their knowledge, experiences, and experimental setups. This promotes collaboration, accelerates innovation, and fosters a community-driven approach to scientific discovery.
The Future of AFM: Towards Unprecedented Discoveries
The integration of Gemini with AFM technology marks an exciting milestone in the field of nanotechnology. As AI continues to evolve and improve, the possibilities for AFM are limitless. The enhanced automation and efficiency offered by AI-powered AFM will lead to unprecedented discoveries, enabling researchers to unlock new insights into the world of atoms and molecules.
With the assistance of Gemini, scientists can focus more on analysis, interpretation, and expanding the frontiers of their field, rather than getting lost in the intricacies of instrument operation. By harnessing the combined power of technology and AI, AFM is set to revolutionize industries ranging from materials science to semiconductor research and beyond.
Comments:
Thank you all for taking the time to read my article! I'm excited to hear your thoughts on how Gemini can enhance automation and efficiency in technology's AFM. Let's start the discussion!
Great article, Julie! Gemini seems like a promising tool to improve automation. I'm curious about its accuracy compared to traditional methods. Any insights?
I agree, Michael! Julie, could you elaborate on how Gemini ensures accuracy when used in AFM automation? Are there any specific validation techniques?
Michael and Emily, Gemini's accuracy is achieved through extensive fine-tuning using large datasets that include AFM measurements. Additionally, we validate Gemini's performance against established AFM techniques to ensure high accuracy.
Interesting read, Julie! It's fascinating how AI-powered tools like Gemini can revolutionize technology. How does it handle complex tasks that require precise measurements?
That's a great question, Mark! Accuracy is crucial, especially in AFM. Julie, could you share any examples where Gemini excels in tackling complex tasks and maintaining precision?
Mark and Jennifer, Gemini excels in complex tasks by adapting its learning from a wide range of input data. It can understand patterns and make precise measurements based on the available information, similar to how expert scientists interpret AFM data. We've seen promising results in various AFM applications.
Hi, Julie! I enjoyed your article. One concern I have is the potential biases in AI models. How does Gemini address bias issues, particularly in AFM applications?
Lisa, ensuring fairness and addressing biases are essential. During the training process, we carefully curate datasets and apply debiasing techniques. Continuous monitoring helps us improve and identify any potential biases that may arise.
Thank you all for your engaging questions! Let me address them one by one.
Impressive use case, Julie! I'm curious about Gemini's limitations. Are there any challenges or areas where it may struggle to provide accurate results?
Great question, Samuel! Gemini is a powerful tool, but it has limitations. It may struggle when faced with uncommon or unexpected scenarios where it lacks exposure to relevant training data. In such cases, human intervention or additional training is required.
Julie, it's fascinating to see how Gemini can maintain precision in complex tasks. Are there any ongoing developments to further improve its capabilities?
Absolutely, Timothy! We are constantly working on improving Gemini's capabilities. Ongoing research and feedback from users like you help us refine the model and explore new ways to enhance precision and efficiency in AFM automation.
Hey, Julie! I've really enjoyed your article. Could you also explain how Gemini handles the diversity of AFM data and provides accurate analysis?
Hi, Daniel! Gemini handles the diversity of AFM data by training on a wide range of examples, ensuring it understands the nuances and variations in AFM measurements. It leverages this knowledge to provide accurate analysis across different types of AFM data.
Thanks for the clarification, Julie!
Julie, as AFM data often involves nanoscale measurements, I'm curious about the precision of Gemini. Does it handle measurements at such a small scale effectively?
Hi, Sophia! Gemini has shown promising results in handling nanoscale measurements. Its ability to understand patterns and relationships enables accurate analysis even at such a small scale. We've observed its effectiveness in various AFM applications involving nanoscale measurements.
That's great to hear, Julie! Thanks for your response.
Julie, I'm curious about the computational resources required to implement Gemini for AFM automation. Could you shed some light on the system requirements?
Sure, Alex! Gemini requires substantial computational resources during training. However, for practical implementation, we have optimized its architecture to be computationally efficient, enabling it to run on standard hardware systems commonly used in AFM setups.
Thank you, Julie!
While Gemini seems promising, human intervention for accurate results might slow down the automation process. How do you balance the need for human intervention and automation?
Sarah, that's a valid concern. We aim to strike a balance between automation and human intervention. Gemini can handle many routine tasks, but for critical cases or uncertain scenarios, it's crucial to involve human experts for final decisions. This ensures accurate and reliable results while maximizing automation efficiency.
Thank you for addressing my concern, Julie!
Julie, I'm fascinated by the potential of Gemini in AFM. Are there any limitations or challenges when deploying Gemini in real-world AFM setups?
Hi, Robert! Deploying Gemini in real-world AFM setups does come with challenges. Some limitations include the need for careful integration, data compatibility, and addressing potential hardware constraints. However, with proper setup and consideration of these factors, Gemini can be seamlessly integrated into AFM workflows.
Understood! Thanks for the insight, Julie.
Julie, I'm curious about the training process of Gemini. How do you ensure comprehensive training and avoid biased training data?
Olivia, training Gemini involves using a diverse dataset that spans various types of AFM measurements. We take special care to ensure comprehensive training by including a wide range of real-world scenarios. To address bias, we meticulously curate and review the training data and apply debiasing techniques to improve fairness and minimize biases.
Thank you, Julie! It's good to hear that steps are taken to ensure comprehensive and fair training.
Julie, how does Gemini handle noisy AFM data? Sometimes measurements can contain inaccuracies due to noise or external factors.
Hi, Sophie! Gemini has robustness built into its training process to handle noisy AFM data. By learning from diverse examples, it becomes resilient to noise and can differentiate between relevant measurements and potential inaccuracies arising from noise or external factors. This enables accurate analysis even in the presence of noise.
That's great! Thanks for clarifying, Julie.
Julie, you mentioned Gemini's ability to make precise measurements in complex tasks. Can it adapt to new measurement techniques or emerging technologies in AFM?
Jacob, Gemini's adaptability is one of its strengths. While it may require additional training or exposure to new measurement techniques, it can adapt to emerging technologies in AFM. Its flexible learning framework enables it to learn from new data and adapt its analysis capabilities.
That's impressive! Thank you for your response, Julie.
Julie, how does Gemini handle uncertainties or ambiguous situations where AFM data might not provide clear guidance?
Karen, when faced with uncertainties or ambiguous situations, Gemini can provide insights based on its training and available data. However, it's essential to involve human experts in such cases to interpret and make final decisions based on their domain knowledge. Human expertise combined with Gemini's analysis ensures accurate guidance even in complex scenarios.
Thank you, Julie! It's great to understand how Gemini and human experts can complement each other.
Julie, what kind of support or assistance is available when implementing Gemini for AFM automation? Does your team provide guidance or resources?
Jonathan, our team provides comprehensive support during the implementation process. We offer guidance, documentation, and resources to assist users in integrating Gemini into their AFM automation workflows. We also welcome feedback and address any issues that may arise.
Thank you for the information, Julie!
Julie, how does Gemini handle different AFM measurement modes? Are there any limitations in terms of compatibility with specific modes?
Adam, Gemini is designed to understand and provide analysis across different AFM measurement modes. While it adapts well to various modes, compatibility may depend on the specific implementation, hardware, and associated data. We work closely with users to ensure seamless integration and compatibility with their preferred AFM measurement modes.
I appreciate your response, Julie!
Julie, have you encountered any ethical challenges or considerations while developing and deploying Gemini in AFM technology?
Emma, ethics and responsible AI deployment are at the core of our development process. We ensure transparency, fairness, and accountability throughout. We actively address potential biases, prioritize user safety and privacy, and aim for transparency in implementing and deploying Gemini. Regular ethical evaluations and ongoing improvement processes contribute to socially responsible technology.
Thank you for your dedication to ethical development, Julie!
Thank you all for reading my blog article on revolutionizing technology's AFM with Gemini! I'm excited to discuss this topic with you.
Great article, Julie! The potential of Gemini to enhance automation and efficiency in AFM is truly fascinating. It could really streamline research processes.
I agree, Michael! The ability of Gemini to provide real-time analysis and suggestions during AFM experiments could greatly benefit researchers by saving time and improving accuracy.
But what about the limitations of Gemini? Is it able to handle the complexities and nuances involved in AFM experiments? It's one thing to provide suggestions, but another to truly understand the context.
That's a valid concern, Alan. While Gemini has shown promising capabilities, it's important to note that it's an AI model and may not fully grasp the intricacies of AFM experiments. However, continuous training and feedback could help improve its contextual understanding.
I think it's important to understand that Gemini should complement human expertise in AFM, rather than replace it. It could provide valuable insights and suggestions, but researchers should still rely on their own knowledge and judgment.
Absolutely, Sophia! Gemini is designed to assist researchers and enhance their capabilities, not replace them. It can help identify patterns, analyze data, and offer suggestions, while researchers remain in control of the experimental process.
One concern I have is the potential for biased or inaccurate suggestions from Gemini. How can we ensure the system is providing reliable information without any false positives or errors?
Valid point, Ethan. To minimize bias and errors, it's crucial to train Gemini with diverse and representative data. Regular updates and feedback loops can help identify and rectify any inaccuracies. Additionally, researchers can always verify suggestions independently.
The ability of Gemini to automate certain tasks in AFM experiments could free up researchers' time to focus on more complex and creative aspects of their work. Exciting possibilities ahead!
Indeed, Natalie! Automation through Gemini allows researchers to spend less time on repetitive tasks and more time on innovative research ideas. It could lead to significant advancements in AFM technology.
I'm curious about the computational requirements of utilizing Gemini in AFM experiments. Would it require significant computational resources or could it be feasible for small research labs too?
Good question, Benjamin! While Gemini does require some computational resources, there are different deployment options available. It can be used on local machines, cloud platforms, or even integrated into AFM software with varying levels of scalability and resource requirements.
Privacy is a concern when utilizing AI models like Gemini. How can we ensure that sensitive data from AFM experiments is protected and not accessible to unauthorized parties?
An important consideration, Lily. Data security and privacy are paramount. Organizations implementing Gemini should ensure proper encryption, access controls, and adherence to data protection regulations. By implementing robust security measures, sensitive data can be safeguarded from unauthorized access.
I wonder if Gemini could be adapted to work with other scientific instruments and research areas as well. Its capabilities seem promising beyond just AFM experiments.
Absolutely, Daniel! The potential applications of Gemini extend beyond AFM. It could be adapted to work with various scientific instruments and research domains, providing valuable insights and assisting researchers in different fields.
Will there be any limitations on the availability and access to Gemini for researchers? Should it be an open-source tool or a proprietary system?
Good point, Olivia. The availability and access to Gemini can vary depending on the organization developing and deploying it. Some models may be open-source, while others might be developed as proprietary systems. Balancing openness and commercial viability will be key in ensuring the widespread adoption of such tools.
I appreciate the potential benefits of Gemini in AFM experiments, but I'm also concerned about its impact on job roles. Could it potentially replace some positions in research labs?
A valid concern, Sophie. While Gemini can automate certain tasks, it's important to view it as a tool to enhance research capabilities, not as a replacement for researchers. It can free up time and improve efficiency, allowing researchers to focus on more challenging aspects of their work.
The article discussed the improvements Gemini brings to AFM, but are there any downsides we should consider? Let's not only focus on the positive aspects.
You're right, Ryan. While Gemini offers notable benefits, there are potential downsides to consider as well. For instance, overreliance on the system without proper verification or independent analysis could lead to errors. Balancing human judgment with AI assistance is crucial.
I can see how Gemini would be useful for researchers, but I'm concerned about the initial learning curve. Will researchers need extensive training to effectively use Gemini in AFM experiments?
Good question, Laura. While familiarity with AI models can be beneficial, the goal is to design Gemini with user-friendly interfaces and intuitive integration to minimize the learning curve. The system should provide clear instructions and be easy to use, even for researchers with limited AI experience.
I would love to hear some real-world examples or case studies where Gemini has already been applied in AFM experiments. Do you have any to share, Julie?
Certainly, Lucas! While Gemini is a relatively new technology, there are ongoing research projects and collaborations where it's being tested in AFM experiments. I encourage you to follow relevant publications and conferences for the latest updates on practical applications.
I'm curious about the potential impact of Gemini on collaborative research. Could it assist in sharing knowledge and insights among researchers from different institutions?
Absolutely, Emma! Gemini has the potential to facilitate collaboration by enabling researchers to share expertise and insights across institutions. It could act as a bridge, connecting scientists and promoting knowledge exchange for mutual benefit.
Given that Gemini relies on language understanding, could language barriers be a potential limitation? How well does it handle non-English scientific terminology?
Language barriers can indeed be a limitation, David. While Gemini is designed to understand and generate text, its effectiveness with non-English scientific terminology may vary. Domain-specific training and improvements are necessary to ensure accurate comprehension and generation of content in different languages.
It's crucial to consider the ethical implications of using AI models like Gemini. Proper guidelines and governance should be established to ensure responsible and ethical deployment.
Well said, Sophia. Ethical considerations are paramount, and organizations must prioritize transparency, accountability, and fairness when implementing AI models like Gemini. Collaboration between researchers, developers, and policymakers is essential to shape guidelines and ensure responsible use.
I'm curious, Julie, what sparked your interest in revolutionizing AFM with Gemini? Were there any specific challenges or experiences that led to this idea?
Great question, Julian! Throughout my research career, I've encountered challenges in AFM experiments that could benefit from AI assistance. The need to streamline processes, improve efficiency, and enhance the overall AFM experience encouraged me to explore the potential of Gemini in revolutionizing technology's AFM.
Gemini sounds promising, but as with any AI technology, it's important to ensure that biases are identified and mitigated. How can we address potential biases in suggestions or analysis provided by Gemini in AFM experiments?
You're absolutely right, Claire. To address potential biases, it's crucial to continuously evaluate Gemini's performance, monitor its suggestions, and collect feedback from diverse user groups. By actively identifying and mitigating biases, we can improve the reliability and fairness of the system's outputs.
How does Gemini handle uncertainties or conflicting research findings? Can it recognize and provide insights when there's a lack of consensus in the scientific community?
An excellent question, Sophie. Gemini can process and provide insights based on the available data and scientific literature. However, in cases of conflicting research findings or lack of consensus, the system's output should be considered as a reference and not a definitive answer. Researchers must rely on critical analysis and consider multiple perspectives before drawing conclusions.
Gemini seems like a powerful tool, but I wonder if it's accessible to researchers without significant AI expertise. How user-friendly is it for those who aren't AI experts?
Valid concern, Amanda. The user-friendliness of Gemini should be a priority to ensure accessibility for researchers without extensive AI expertise. The system should have clear interfaces, detailed documentation, and possibly even tutorials to assist users in effectively utilizing its capabilities.
The potential impact of Gemini in AFM experiments is exciting! When do you think we'll start seeing widespread adoption of AI technologies like this in the scientific community?
It's hard to predict an exact timeline, Robert, but the scientific community is showing increasing interest in AI technologies. As AI models like Gemini continue to improve and demonstrate their value, we can expect a gradual but significant adoption in the coming years.
The ethical use of AI in research is crucial. What steps can be taken to ensure that researchers are properly trained and aware of the implications and limitations of using Gemini or similar tools?
Training and awareness programs play a vital role, Brian. Educational initiatives should be established to keep researchers informed about the implications, limitations, and responsible use of AI tools like Gemini. Universities, institutions, and professional organizations can develop workshops, courses, or resources dedicated to ensuring ethical and informed use of AI technologies.
Julie, do you think Gemini could also assist in teaching and training new researchers in the field of AFM? It could potentially act as a virtual mentor or guide.
That's an interesting idea, Caroline! Gemini's capabilities could be leveraged for educational purposes, serving as a virtual mentor or guide for new researchers in AFM. It would facilitate knowledge transfer and provide insights to help them navigate the field more effectively.
Considering the evolving nature of AFM technology, how frequently should Gemini be updated to keep up with the latest advancements and ensure its relevance?
A critical aspect, Sophia! Continuous updates are necessary to incorporate the latest advancements in AFM technology and research. Regular retraining of Gemini with new data and methodologies will help ensure its relevance and effectiveness in assisting researchers.
Thank you all for the engaging discussion and insightful comments! I appreciate your thoughts, concerns, and suggestions regarding the application of Gemini in revolutionizing technology's AFM. Let's continue exploring the potential of AI in scientific research together.