Revolutionizing the ISPF: Harnessing the Power of Gemini for Cutting-Edge Technology Transformation
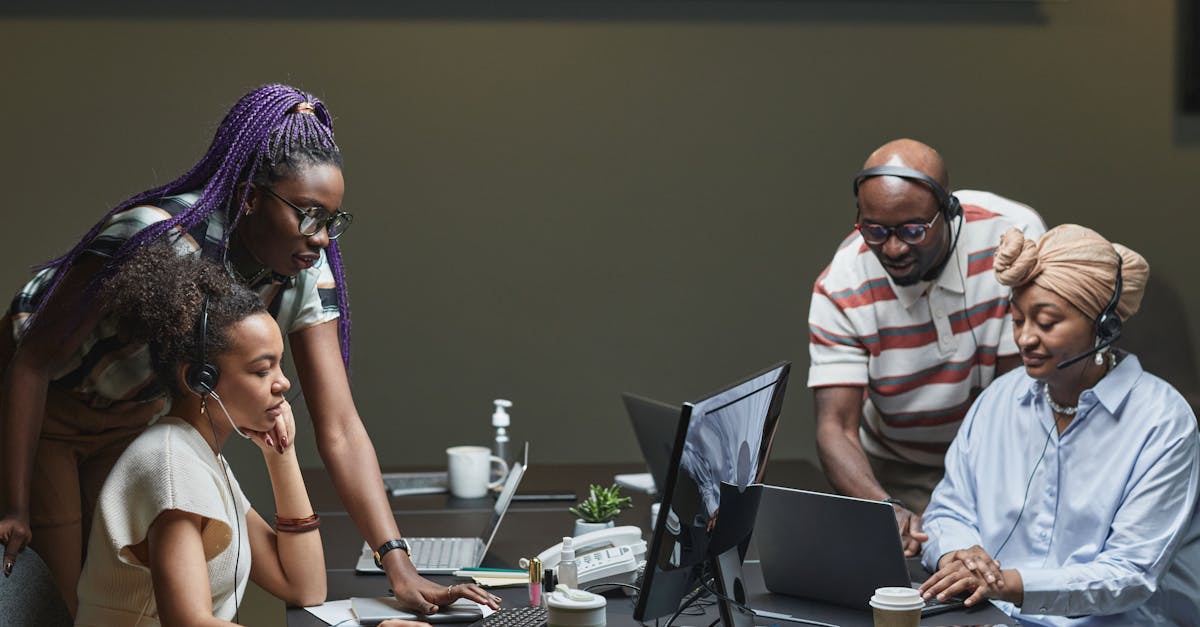
The landscape of technology is continually evolving, with new advancements and innovations shaping the way we interact with systems and applications. Within this dynamic environment, the Interactive System Productivity Facility (ISPF) has played a crucial role in enabling efficient and streamlined user interactions on IBM mainframe systems.
The Evolution of ISPF
ISPF, initially introduced in the 1970s, has been the primary interface for IBM mainframe systems. It provides a robust set of tools and utilities for navigating, editing, and executing commands on these systems. Over the years, ISPF has undergone significant enhancements to improve usability and productivity.
Introducing Gemini
One such transformative enhancement is the integration of Gemini into ISPF. Gemini is a language model developed by Google that utilizes deep learning to generate human-like responses based on provided prompts.
Benefits and Applications
By integrating Gemini into ISPF, users can benefit from enhanced user experiences and improved productivity. Here are a few key advantages and applications of this technology:
1. Interactive Guidance
Gemini can provide interactive guidance to users within the ISPF environment. It can understand prompts and provide helpful suggestions, reducing the learning curve for new users and enhancing the productivity of experienced users.
2. Automated Assistance
With the power of AI, Gemini can automate routine tasks and provide assistance with complex operations. This automation reduces human effort, increases accuracy, and promotes overall efficiency within the ISPF ecosystem.
3. Natural Language Conversations
Gemini enables natural language conversations with the ISPF system. Users can interact with the system using conversational prompts, allowing for a more intuitive and user-friendly experience. This helps bridge the gap between technical complexities and user interactions.
Challenges and Considerations
While the integration of Gemini into ISPF brings undoubted benefits, it also poses some challenges and considerations:
1. Data Privacy and Security
As Gemini relies on machine learning models, sensitive and confidential data may be processed during user interactions. Ensuring robust data privacy and security measures are in place is crucial to prevent unauthorized access or breach.
2. Accuracy and Contextual Understanding
Gemini's responses are generated based on patterns and examples from training data. However, ensuring accurate and contextually appropriate responses may pose a challenge in certain situations. Continuous model training and validation are necessary to maintain high response quality.
The Future of ISPF with Gemini
The integration of Gemini into ISPF marks a significant step towards revolutionizing the user experience on IBM mainframe systems. As advancements in natural language processing and AI continue, the capabilities of Gemini will only improve, paving the way for further innovation within the ISPF ecosystem.
Conclusion
The integration of Gemini brings a new wave of interaction possibilities to the ISPF environment. Harnessing the power of advanced language models not only enhances user experiences but also streamlines complex operations, propelling technology transformation within IBM mainframe systems. As we move forward, it is essential to address challenges, ensuring privacy, security, and accuracy to fully leverage the potential of Gemini for the benefit of ISPF users.
Comments:
Thank you all for taking the time to read my article on revolutionizing the ISPF with Gemini! I am excited to hear your thoughts and engage in insightful discussions.
Great article, Hank! The potential of Gemini in transforming technology sounds incredibly promising. I'm curious to know how it can be implemented practically within ISPF environments.
I agree with Alice, Hank. The practical implementation details of Gemini within ISPF systems would be fascinating to explore. Can you explain the potential use cases where users can benefit from this technology?
Thank you, Alice and Frank! Integrating Gemini into ISPF can bring numerous benefits. For instance, it can assist users in navigating and understanding complex menus, provide real-time suggestions, and automate repetitive tasks. These are just a few potential use cases that can greatly enhance user experience within ISPF environments.
Well written, Hank! I've been following the advancements in AI for a while, and this integration of Gemini in ISPF seems like a significant step forward. Can you elaborate on the challenges that could arise during its implementation?
Bob, you raised an important point regarding implementation challenges. One potential challenge could be the need for continuous AI model training to keep up with the evolving ISPF environment and user needs. Additionally, minimizing false positives and negatives in AI-generated responses would be crucial. Hank, would you like to add anything?
Excellent insights, Grace! Continuous training of the AI model is essential, and striking the right balance between accurate responses and minimizing false results will indeed be a challenge. To address this, a feedback loop can be established to gather user feedback and improve the AI model's response accuracy as it learns from user interactions over time.
Impressive article, Hank! As an ISPF user, I can see the potential of leveraging Gemini for enhancing user experience. However, I'm also concerned about the security implications of integrating such powerful AI technology. Could you please address those concerns?
Eve, I share your concerns about security. The integration of powerful AI technology like Gemini should be accompanied by robust security measures. It would be interesting if Hank could elaborate on the security considerations and potential safeguards that could be implemented.
Absolutely, Dave! Ensuring the confidentiality, integrity, and availability of sensitive ISPF data is crucial. Implementing user authentication, data encryption, and access controls are some fundamental security measures to address these concerns. Regular security audits and updates to tackle emerging threats should also be implemented alongside the integration of Gemini.
Dave, thank you for bringing up security again. Along with encryption and access controls, it's important to ensure that AI biases or skewed behavior don't compromise the overall integrity of the system. Hank, how can we address those concerns when integrating Gemini?
Valid point, Eve! Addressing biases and skewed behavior is crucial for ethical and fair AI integration. Regularly auditing and monitoring the AI model's responses for any unwarranted bias, and providing mechanisms for users to report concerns can help in addressing these issues. Incorporating diverse datasets during training and involving multidisciplinary teams during development and deployment can also contribute to more responsible AI integration.
Hank, your answer addresses my concerns as well. It's important for Gemini to be adaptive and flexible in providing relevant suggestions, even in unexpected scenarios. The ability to handle such variations would be a testament to the AI's robustness.
Hank, I appreciate your insights on addressing biases and skewed behavior. It's essential to have multidisciplinary teams involved in the development and deployment of Gemini to ensure a holistic approach. Transparency about the AI's limitations and potential biases would also contribute to building user trust.
Absolutely, Eve! Transparency plays a vital role in building trust and ensuring responsible AI integration. Clearly communicating to users about the limitations, potential biases, and guidelines for ethical usage helps foster a reliable and trustworthy environment. Collaboration between technical, ethical, and legal experts can contribute to a more comprehensive understanding of AI's impact and facilitate responsible decision-making throughout the integration process.
Thanks for sharing your insights, Hank! The possibilities of combining ISPF with Gemini are indeed exciting. I'm particularly interested in understanding how this integration can streamline complex workflows and enhance productivity.
Charlie, I'm also interested in how the integration of Gemini in ISPF can enhance productivity. Are there specific tasks or workflows that you think can be significantly improved?
Certainly, Frank! Complex tasks like generating complex code snippets, troubleshooting issues, or even assisting in debugging can be streamlined with the integration of Gemini. Users can receive real-time contextual assistance, reducing the time spent searching for answers and enabling them to focus more on their core tasks.
Charlie, I completely agree with you. As someone who regularly works in ISPF, having a powerful assistant like Gemini would greatly improve productivity and help resolve issues more efficiently. Hank, what are your thoughts on this?
Thank you, Eva! Indeed, streamlining complex tasks and troubleshooting within ISPF environments is a primary objective of integrating Gemini. By assisting users with code generation, debugging, and providing contextual guidance, it can enable more efficient workflow execution and improve productivity. The potential impact on users' experience is tremendous.
Hank, can you please clarify how the real-time suggestions from Gemini in ISPF would work? Would it be based on the user's previous actions or some other form of contextual awareness?
Certainly, Alice! Real-time suggestions can harness the contextual awareness of the AI model. By considering the user's history, the current state of the workflow, and utilizing the power of language processing, Gemini can recommend relevant actions, functions, or even anticipate user needs based on the ongoing task. This contextual awareness helps tailor suggestions to individual users and improves their overall experience within ISPF.
Hank, regarding the feedback loop you mentioned, would it be an iterative process where user feedback helps refine the AI model's responses in near real-time? Also, how can users provide feedback?
Great questions, Bob! Yes, the feedback loop can indeed be an iterative process. Implementing mechanisms for users to rate the helpfulness or relevance of AI-generated responses can aid in refining the model's accuracy over time. Users can easily provide feedback through the ISPF interface, enabling them to report any false positives, false negatives, or suggest improvements. Analyzing this user feedback can help enhance the AI model and its responses.
Hank, gathering user feedback for improving the AI model's responses seems like an excellent approach. Would the feedback loop also involve human reviewers to ensure quality control and prevent biased or inappropriate responses?
Great point, Bob! Implementing a system with human reviewers can indeed help ensure quality control and address biases or inappropriate responses. Human reviewers can play a crucial role in refining the AI model's behavior, providing oversight, and ensuring ethical considerations are met. Their expertise can complement the advantages of AI technology and contribute to the continuous improvement of the AI-generated responses within ISPF.
Hank, having human reviewers involved in the feedback loop is crucial for maintaining the quality and appropriateness of AI-generated responses. Their expertise ensures that the system doesn't generate inaccurate or biased suggestions that may have unintended consequences. It helps in building user trust as well.
You're absolutely right, Bob! Human reviewers provide valuable oversight and ensure that the AI-generated responses meet quality standards. The fusion of human expertise with AI technology helps establish user trust by minimizing the risks of flawed or inappropriate suggestions. Furthermore, the feedback from human reviewers allows for continuous improvement, making Gemini's assistance within ISPF environments more reliable and effective.
Hank, the idea of contextual awareness in real-time suggestions sounds promising! Just one concern: how would the system handle cases where the user deviates from their typical workflow or tries something new? Could the AI handle such unpredictable scenarios?
Excellent question, Alice! While Gemini leverages historical user data and workflow context, it should also be capable of adaptability. The system can handle unpredictable scenarios by maintaining a dynamic understanding of the user's intent, grasping contextual cues, and adapting its suggestions accordingly. The AI model should be trained on diverse scenarios to ensure its ability to handle various situations, including instances where users deviate from their typical workflows.
Hank, adaptability is a critical aspect! Considering users' typical workflows while allowing for flexibility in handling new scenarios ensures that Gemini can cater to a wide range of user needs. It adds versatility and usefulness to the AI integration within ISPF.
Indeed, Alice! Gemini's adaptability enables it to be more versatile and useful for users in various situations. By striking a balance between leveraging historical user data and flexibility in accommodating new scenarios, it can provide valuable assistance across different workflows, ultimately enhancing the user experience and driving innovation within ISPF environments.
Charlie, it's fascinating to think about the potential improvements in troubleshooting with Gemini. Imagine the time saved by having an AI assistant suggest possible solutions based on error messages or stack traces. How cool would that be?
Absolutely, Frank! Having an AI assistant understand the context of error messages and stack traces, and providing accurate suggestions would be a massive time-saver. It can assist users in quickly identifying the cause of an error or narrowing down potential solutions, significantly improving their efficiency and reducing frustration.
Charlie, I couldn't agree more. Troubleshooting is often a pain point, and having a reliable AI assistant like Gemini could transform the debugging experience. Hank, do you have any insights on how Gemini can assist with complex code generation?
Indeed, Eva! Gemini's contextual understanding and language processing capabilities can play a significant role in complex code generation. It can assist users by suggesting code snippets, recommending best practices, and even automatically generating basic code structures based on the user's requirements. This assistance can help users streamline and accelerate their coding process within an ISPF environment.
Frank, just envision the productivity boost for programmers when Gemini can analyze error messages, stack traces, and suggest possible solutions! It would be a game-changer in accelerating problem resolution and reducing development time.
Charlie, I couldn't agree more! Troubleshooting and debugging are often time-consuming processes, and having an AI assistant that can quickly analyze the problem and propose solutions would undoubtedly enhance productivity in ISPF environments.
Charlie and Hank, the idea of Gemini suggesting code snippets and automating basic structures is exciting! It could greatly benefit developers who might be just starting or are looking for optimized solutions. Being able to speed up coding processes and encouraging best practices would be invaluable.
Absolutely, Eva! Gemini's ability to suggest code snippets and encourage best practices can be highly advantageous, particularly for novice or less experienced developers. It can also act as a valuable resource for experienced developers by offering alternative perspectives or new efficient approaches. By automating repetitive coding tasks, it enables faster development cycles and facilitates the adoption of best practices throughout ISPF workflows.
Thank you all for taking the time to read my article on Revolutionizing the ISPF with Gemini! I'm excited to hear your thoughts and have a fruitful discussion.
Great article, Hank! Gemini indeed has the potential to revolutionize ISPF by enhancing user experiences and increasing productivity.
I totally agree, Lisa! The power of conversational AI can truly transform how we interact with technology.
Absolutely, Danielle! The ability to have natural language conversations with ISPF opens up a world of possibilities for simplifying complex workflows.
I'm a bit skeptical about the reliability of Gemini. Has anyone encountered limitations or drawbacks in its application?
Carlos, while Gemini has shown remarkable progress, it can still generate responses that are plausible but incorrect. So, it's crucial to validate its output in critical scenarios.
Thanks for the insight, Megan! That's an important consideration when adopting such technologies.
I find this development fascinating! Hank, do you have any specific examples of how Gemini can enhance ISPF capabilities?
Certainly, David! Gemini can facilitate intuitive navigation through menus, provide contextual help, auto-generate JCL from human-readable descriptions, and assist in debugging complex problems.
While Gemini has its limitations, it's improving rapidly. Continuous training and fine-tuning of the model can help address its drawbacks.
It's great to see how AI advancements can transform traditional mainframe systems like ISPF.
Indeed, Patricia! The integration of AI technologies into such systems will pave the way for more efficient and user-friendly interactions.
I'm curious about the implementation process. Are there any specific requirements or challenges in incorporating Gemini into ISPF?
Hannah, integrating Gemini into ISPF may require data preprocessing, adapting the model to work with mainframe-specific use cases, and ensuring robustness against user input deviations.
Hank, have you witnessed any performance improvements or user feedback on early implementations of Gemini in ISPF?
Ryan, early implementations have shown promising results. Users appreciate the conversational aspect and find it helpful in reducing manual effort and improving their overall efficiency.
Did you face any challenges in training Gemini to handle the intricacies of ISPF and mainframe environments?
Sara, training Gemini on mainframe-specific data and fine-tuning it to handle mainframe terminology and workflows was indeed challenging, but essential for its effectiveness.
I'm concerned about the potential impact of Gemini on traditional command-line interfaces that many developers are accustomed to.
Philip, while Gemini brings conversational benefits, it doesn't necessarily replace command-line interfaces. It can work alongside them, augmenting traditional workflows.
Absolutely, Brian! The goal is to enhance user experiences and provide more options for interacting with ISPF, not to eliminate existing interfaces.
Thanks for clarifying, Brian and Linda! It's good to know that it complements rather than replaces the existing interfaces.
This article is eye-opening! It made me realize the untapped potential of AI in mainframe systems like ISPF.
Hank, do you think Gemini will be widely adopted soon, or might there be hesitancy in integrating it into production systems?
Greg, while organizations might take some time to assess the reliability and security aspects, I believe Gemini holds immense promise and will eventually be adopted on a larger scale.
Integration into production systems should be gradual, with proper testing and validations. But the potential benefits make it worth exploring.
Thank you, Hank and Nicole, for sharing your thoughts on the practical aspects of integrating Gemini into production environments.
Hank, what are your recommendations for organizations planning to leverage Gemini in their ISPF systems?
Tom, it's crucial to evaluate potential use cases, start with pilot projects, involve domain experts during training, and iterate based on user feedback for successful adoption of Gemini.
Thank you, Hank! That's valuable guidance for organizations embarking on this transformative journey.
I've been wondering about the computational requirements to run Gemini alongside ISPF. Are there any specific hardware or performance considerations?
Adam, deploying Gemini alongside ISPF might require additional computational resources, but it can be optimized by using efficient hardware configurations and balancing system workloads.
Hank, does Gemini have built-in mechanisms for handling sensitive or confidential information in ISPF?
Julia, handling sensitive or confidential information is a critical aspect. Access controls, data anonymization, and sanitization techniques should be employed to protect such data.
Thank you, Hank! Ensuring data privacy and security is of utmost importance when leveraging AI technologies.
I'm thrilled by the potential of Gemini in simplifying complex workflows. It could significantly improve the learning curve for novice users.
Indeed, Gabriel! The conversational interface can provide real-time guidance and explanations, making it easier for new users to navigate through ISPF.
That's exactly what I had in mind, Rachel! Reduced learning curves will foster faster adoption and increased productivity.
Great article, Hank! This breakthrough can fundamentally change how we interact with mainframe systems.
Thank you, Oliver! I appreciate your kind words. The potential of Gemini to reshape mainframe interactions is indeed remarkable.
Hank, I'm curious to know if Gemini can handle multi-step tasks or more complex queries in ISPF systems.
Emily, Gemini can be trained to handle multi-step tasks by breaking them down into individual sub-tasks and defining appropriate conversational flows. Complex queries can also be addressed by leveraging its contextual understanding.
Hank, what training methods or techniques would you recommend to improve the accuracy and reliability of Gemini in ISPF contexts?
Marie, training Gemini with a diverse range of real-world ISPF examples, incorporating user feedback for continuous improvement, and validating against known workflows can significantly enhance its accuracy and reliability.
It's interesting to see how AI continues to transform various domains. Hank, do you foresee any challenges in the widespread adoption of Gemini in ISPF?
Patrick, besides the technical challenges of training and integrating Gemini, addressing user concerns about data privacy, reliability, and providing suitable documentation will be crucial for its widespread acceptance.
Hank, what factors should organizations consider before implementing Gemini in their ISPF environments?
Laura, organizations should evaluate the potential benefits, assess infrastructure readiness, consider existing user workflows, ensure proper testing and validation procedures, and plan for user training and onboarding.
The integration of Gemini with ISPF sounds promising, but are there any use cases where it may not be suitable?
Chris, in highly regulated or strictly governed environments, where human intervention is necessary, or where offline usage is critical, the suitability of Gemini should be carefully evaluated.
Thank you all for your valuable insights and questions! I truly enjoyed discussing the potential of Gemini in revolutionizing ISPF.
If you have any further questions or thoughts, please feel free to continue the discussion.
Signing off for now. Have a great day everyone!