Revolutionizing the Rolling Stock: Enhancing Technology with ChatGPT
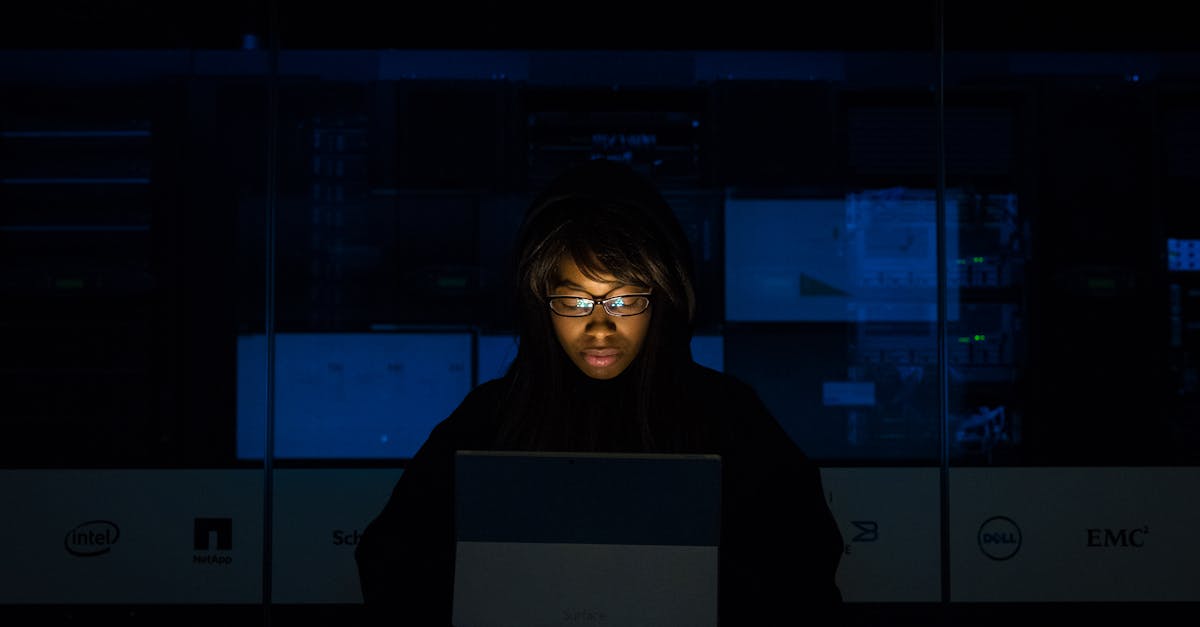
In the realm of transportation, rolling stock refers to the collection of vehicles used in a railway system. These vehicles, including trains and their components, play a crucial role in ensuring the smooth operation of railways.
One of the key challenges in maintaining rolling stock is the timely detection and prevention of failures or breakdowns. The traditional maintenance approach often follows a fixed schedule or relies on reactive measures, leading to potential service disruptions and increased costs.
However, with advancements in technology, predictive maintenance has emerged as a game-changer. By utilizing predictive analytics and machine learning algorithms, it becomes possible to predict when a train component may fail or require maintenance. This is where ChatGPT-4, an advanced language model, comes into play.
The Power of ChatGPT-4
ChatGPT-4 is an artificial intelligence model developed by OpenAI, designed to understand and generate human-like text based on given prompts. It can analyze vast amounts of data to identify patterns and correlations that humans may overlook. With its ability to comprehend and process complex information, ChatGPT-4 proves to be a valuable tool for predictive maintenance in the rolling stock industry.
By training ChatGPT-4 on historical data from rolling stock and maintenance logs, the model can learn to identify potential failure patterns and provide proactive recommendations. It can predict when specific components are likely to fail, giving railway companies the ability to plan for maintenance in advance.
Benefits of Predictive Maintenance
Predictive maintenance enabled by ChatGPT-4 offers several notable benefits:
- Cost Reduction: By predicting maintenance needs accurately, railway companies can avoid costly unplanned repairs and minimize the impact of failure-related disruptions.
- Improved Safety: Proactively identifying potential failures allows for timely intervention, reducing the risk of accidents or incidents caused by faulty components.
- Enhanced Efficiency: With scheduled maintenance based on predictive insights, trains can operate at peak performance levels, optimizing operational efficiency.
- Extended Component Lifespan: Identifying maintenance needs before failures occur can help extend the lifespan of train components, reducing the need for premature replacements.
Implementation and Challenges
Implementing predictive maintenance in the rolling stock industry involves integrating sensors and data collection devices in train components. These devices continuously monitor crucial parameters such as temperature, vibration, and pressure. The collected data is then fed into ChatGPT-4 for analysis and prediction.
However, there are several challenges that need to be addressed during the implementation:
- Data Quality: Reliable and accurate data is crucial for training the predictive model. Ensuring data completeness and consistency is essential.
- Data Integration: Integrating various data sources and systems across different train components and manufacturers requires standardized protocols and interfaces.
- Model Accuracy: Continuously improving the accuracy of ChatGPT-4's predictions is necessary to maintain its effectiveness in preventing failures.
- Privacy and Security: Protecting sensitive data while complying with privacy regulations is vital to maintain public trust in the system.
The Future of Predictive Maintenance
As technology continues to evolve, the capabilities of predictive maintenance are expected to grow further. ChatGPT-4 is just the beginning, and future iterations of the model may incorporate real-time data analysis and enhanced accuracy.
With proactive planning enabled by predictive maintenance, railway companies can reduce costs, improve operational efficiency, and ensure safe and reliable transportation services. By leveraging the power of AI, the rolling stock industry can stay ahead of maintenance needs and optimize the lifespan of train components.
Comments:
Thank you all for your comments and feedback on the article!
ChatGPT seems like an interesting innovation for enhancing technology in the rolling stock industry. It could potentially improve communication and various other aspects.
I agree, Michael. The ability to utilize ChatGPT within rolling stock systems can lead to more efficient and reliable operations.
Indeed, Laura. ChatGPT has the potential to revolutionize the way we approach rolling stock technology. What are your thoughts on its integration challenges?
One challenge I can think of is ensuring the language model of ChatGPT is well-trained and understands the specific vocabulary and jargon used in the rolling stock industry.
That's a valid point, Jack. To overcome this challenge, it would require a comprehensive training dataset that covers rolling stock terminology and context.
Jack, I agree with your point about training the language model adequately. The accuracy and relevance of ChatGPT's responses will heavily depend on the quality of its training data.
Michael, I completely agree. Adequate training data, including rolling stock-specific terminology, will help ChatGPT provide accurate and contextually appropriate responses.
Absolutely, Laura. The deep learning algorithms powering ChatGPT require extensive and relevant training to ensure the reliability and usefulness of its responses.
Laura, I share your excitement about the potential benefits ChatGPT can bring to the rolling stock industry. It can optimize maintenance schedules, improve operational efficiency, and much more.
I can see how incorporating ChatGPT into rolling stock systems can improve customer service. It could provide quick and accurate responses to queries or issues passengers may have.
Absolutely, Sophia. ChatGPT can enhance the overall passenger experience by offering personalized assistance and resolving problems in real-time.
Sophia, I can see how ChatGPT can not only improve customer service but also enable predictive maintenance and optimize rolling stock performance.
While ChatGPT can be useful, we also need to consider the privacy and security aspects of incorporating such technology in rolling stock systems.
Good point, Emily. We must ensure that any data processed by ChatGPT is handled securely and in compliance with privacy regulations.
One potential drawback of relying on ChatGPT for critical rolling stock operations is its dependency on stable internet connectivity. In case of network outages, it could pose challenges.
Indeed, John. The reliability of internet connectivity is crucial when relying on AI-based technologies like ChatGPT. Backup systems and redundancy measures would be necessary.
John, you're right about the dependency on internet connectivity. However, implementing failover systems and backup communication channels can mitigate this risk.
Nathan, you make a valid point. Implementing redundancy and backup systems can help ensure continuous and reliable operation of ChatGPT in rolling stock environments.
Indeed, John. Redundancy and failover mechanisms are essential to minimize the impact of any network failures or disruptions.
Privacy and security should be top priorities when implementing ChatGPT in rolling stock systems. Anonymized data and strong encryption should be considered.
Absolutely, Emily. Protecting passenger data and ensuring secure communication channels should be integral to the adoption of ChatGPT in the rolling stock industry.
Having backup communication channels and reliable failover systems is crucial indeed. We can't afford any disruptions in operations due to ChatGPT's dependency on internet connectivity.
Agreed, John. A robust and resilient infrastructure that accounts for potential network disruptions is necessary to ensure uninterrupted functioning of ChatGPT.
Exactly, John. Network resilience and redundancy measures are essential to minimize any potential disruptions caused by internet connectivity issues.
Predictive maintenance is an exciting application. With the right inputs and accurate predictions, it can help avoid costly breakdowns and improve rolling stock availability.
I agree, Oliver. Predictive maintenance can result in increased reliability and better resource planning by identifying maintenance needs in advance.
Privacy concerns are valid, especially when it comes to handling passenger data. The collection, storage, and processing of information must comply with strict privacy regulations.
Absolutely, rolling stock companies can benefit greatly from better maintenance scheduling and resource allocation using ChatGPT's capabilities.
Certainly, Michael. ChatGPT can also be utilized for real-time performance monitoring, energy efficiency optimization, and even assisting with troubleshooting complex issues.
Right, Laura. With real-time performance monitoring facilitated by ChatGPT, maintenance issues or anomalies can be detected earlier, allowing for quicker response and resolution.
Absolutely, Michael. Timely detection of any performance deviations can contribute to improved rolling stock safety and reliability.
Indeed, Laura. A well-trained language model with a deep understanding of rolling stock terminology is vital for ChatGPT to be an effective tool in the industry.
Anonymization of passenger data can help address privacy concerns. It ensures personal information is not directly linked to ChatGPT's responses.
Customer service improvements and predictive maintenance are just a few examples of how ChatGPT can add value to the rolling stock industry. Can you think of any other applications?
Training ChatGPT specifically for the rolling stock industry could be a challenge. It requires a diverse and extensive dataset encompassing various scenarios encountered in the field.
You're correct, Jack. Building an inclusive training dataset is crucial for ChatGPT to understand the nuances and complexities of the rolling stock industry.
Real-time personalized assistance for passengers can greatly improve their experience. ChatGPT can provide relevant information, updates, and guidance throughout their journey.
Exactly, Sophia. ChatGPT can act as a helpful virtual assistant, catering to the individual needs of passengers and providing seamless support throughout their travel.
Encryption plays a crucial role in securing data transmitted between rolling stock systems and ChatGPT. It must be implemented with strong encryption protocols to prevent unauthorized access.
Efficient resource allocation and availability optimization can lead to cost savings for rolling stock companies and improved service reliability for passengers.
Agreed, anonymization helps protect passenger privacy while leveraging the capabilities of ChatGPT in the rolling stock industry.
Emily, you raised an important point about privacy and security. It's crucial to adhere to data protection regulations and ensure passengers' personal information remains safeguarded.
Privacy and security considerations are vital when implementing ChatGPT in rolling stock systems. Protecting passenger data and ensuring secure communication channels should be a priority.
Building a diverse and comprehensive dataset is a challenge, but it's crucial for ChatGPT to understand a wide range of rolling stock industry contexts and provide accurate responses.
Indeed, Jack. The success of ChatGPT's implementation in the rolling stock industry depends on its ability to comprehend and address various scenarios effectively.
Real-time assistance provided by ChatGPT can reduce passenger stress by promptly addressing queries or concerns they may have during their journey.
I agree, Sophia. ChatGPT's ability to provide personalized information and assistance can significantly enhance the overall travel experience for passengers.
Sophia, you're right. By leveraging ChatGPT, operators can address passenger queries and concerns promptly, leading to higher satisfaction levels.
Absolutely, building a diverse and representative training dataset covering rolling stock scenarios will be crucial for ChatGPT's competence in the industry.
Indeed, ChatGPT's real-time guidance and support can be invaluable, especially for passengers who are unfamiliar with the rolling stock system or need special assistance.
Resource optimization and efficient allocation can positively impact on-time performance, reducing delays and improving passenger satisfaction.
Exactly, ensuring network resilience and implementing redundancy measures will be vital for the reliable operation of ChatGPT in rolling stock environments.
John, you're right. Backup communication channels and failover systems would be necessary to prevent any major disruptions due to network outages or connectivity issues.
Anonymization can help build trust with passengers, as their privacy is protected while utilizing the services powered by ChatGPT.
Optimized resource allocation can lead to more efficient rolling stock operations, reduced costs, and improved overall system performance.
Strong encryption should be a priority to ensure the secure transmission and storage of data in the rolling stock systems utilizing ChatGPT.
Absolutely, Emily. Implementing robust encryption protocols and secure data handling practices will be crucial to protect passenger privacy and maintain trust.
Privacy regulations exist for a reason, so it's our responsibility to ensure passengers' personal information is handled with utmost security and privacy.
Michael, you raise an excellent point. The quality of ChatGPT's training data will directly influence its ability to provide accurate and relevant responses in the rolling stock domain.
Nathan, accurate and extensive training data is crucial to mitigate biases and ensure ChatGPT can handle a wide range of rolling stock-related queries effectively.
Absolutely, Michael. A diverse and comprehensive training dataset will enhance the versatility of ChatGPT in addressing different rolling stock scenarios.
Absolutely, Michael. Protecting passenger information and ensuring their privacy is maintained is of utmost importance when implementing ChatGPT in rolling stock systems.
Indeed, Michael. Timely detection of potential performance deviations will contribute to a safer and more reliable rolling stock system for passengers and operators alike.
Performance optimization through real-time monitoring can lead to improved rolling stock reliability, timeliness, and ultimately, passenger satisfaction.
Laura, you're right. Building a well-trained language model for ChatGPT, one that includes industry jargon, will be crucial to its successful adoption in the rolling stock domain.
Indeed, John. ChatGPT should possess a comprehensive understanding of the rolling stock industry's terminology to provide accurate and meaningful responses.
Predictive maintenance can greatly reduce unexpected breakdowns and improve rolling stock availability, resulting in better service reliability.
Encryption should be applied not just during data transmission but also when storing passenger data, minimizing the risk of unauthorized access.
Real-time performance monitoring can allow operators to detect anomalies early, enabling proactive maintenance and reducing the likelihood of major disruptions.
Building a comprehensive training dataset should incorporate diverse scenarios, covering maintenance, troubleshooting, safety measures, and passenger interactions.
Another application could be leveraging ChatGPT for demand forecasting, enabling operators to optimize rolling stock deployment based on anticipated passenger volumes.
Exactly, Oliver. Predictive maintenance, optimization of rolling stock availability, and energy efficiency are some of the key benefits of integrating ChatGPT in the industry.
Ensuring network resilience and implementing robust backup systems will be crucial to mitigate any potential disruptions in ChatGPT's operation within rolling stock environments.
Backup systems and redundancy measures will provide the necessary support in case of network outages or connectivity issues impacting ChatGPT's operation.
Optimizing rolling stock performance can result in better energy efficiency, reducing operational costs and making the system more environmentally friendly.
Having backup communication channels and network redundancy will be vital to ensure uninterrupted operation of ChatGPT during network disruptions.
Privacy and security measures should be implemented with meticulous attention to detail, ensuring that rolling stock systems utilizing ChatGPT adhere to the highest standards.
Reducing passenger stress by providing real-time assistance can contribute to a more pleasant and comfortable travel experience for everyone.
Sophia, the real-time assistance offered by ChatGPT can significantly enhance passenger experience, minimize disruptions, and improve overall satisfaction.
Real-time performance monitoring helps operators identify potential issues early, enabling them to take proactive measures to maintain rolling stock reliability.
Precisely, training ChatGPT with diverse and complex rolling stock industry scenarios will allow it to handle a wide range of real-world situations effectively.
Encryption is crucial in protecting data privacy during communication between rolling stock systems and ensuring the integrity of the information shared.
A comprehensive training dataset encompassing various rolling stock industry scenarios will be instrumental in ChatGPT's reliable and effective functioning.
Indeed, building a diverse dataset covering various rolling stock situations will enhance ChatGPT's contextual understanding and improve the accuracy of its responses.
Absolutely, Jack. The more scenarios and contexts we include in ChatGPT's training data, the more equipped it will be to handle a wide range of rolling stock challenges.
You're absolutely right, Jack. Accurate training data reflecting various aspects of the rolling stock industry will significantly improve ChatGPT's understanding and response quality.
Data anonymization is a crucial step in ensuring the privacy and security of passenger information. Implementation should follow best practices to minimize any risks.
Efficient resource allocation can help optimize the utilization of rolling stock, reducing waste and unnecessary operational costs.
Demand forecasting powered by ChatGPT can lead to better resource planning and utilization, improving the overall efficiency of rolling stock operations.
Indeed, Oliver. Sharing real-time information and updates through ChatGPT can minimize passenger confusion and ensure smoother travel experiences.
Real-time performance monitoring facilitated by ChatGPT can enable operators to identify and address emerging maintenance issues proactively.
The diversity and comprehensiveness of training data will determine ChatGPT's competence in simulating human-like responses in rolling stock contexts.
Precisely, predictive maintenance can help rolling stock operators avoid unexpected breakdowns, reducing passenger inconveniences, and improving system reliability.
Encryption acts as a protective shield, securing data from unauthorized access or tampering. It should be an integral part of any rolling stock system incorporating ChatGPT.
Correct, network resilience and redundancy will be vital in maintaining the continuous operation of ChatGPT within the rolling stock environment, even during challenging connectivity situations.
Network resilience and backup communication channels are key components to ensure uninterrupted operation of ChatGPT in rolling stock systems.
Timely detection of potential performance deviations and anomalies through real-time monitoring can contribute to maintaining high levels of safety and efficiency in the rolling stock industry.
Maintaining strong encryption protocols and secure data handling practices is vital in protecting passenger privacy and building trust in ChatGPT-enabled rolling stock systems.
Predictive maintenance enables operators to take proactive measures, enhancing rolling stock performance and preventing unexpected failures or accidents.
Thank you all for your comments on my article! I'm excited to hear your thoughts.
The idea of using ChatGPT to enhance technology in rolling stock sounds intriguing.
I agree, Jeff! It could lead to improved efficiency and better customer experiences.
Exactly, Lisa! I can imagine ChatGPT assisting passengers with real-time information and personalized recommendations.
Mark, that's an interesting thought! ChatGPT could offer a more personalized travel experience for passengers.
Definitely, Lisa! It could even provide language translation and recommendations for local attractions.
While the concept is exciting, I have concerns about relying too heavily on AI for critical operations.
I understand your concern, Sarah. It's crucial to maintain a balance between AI and human decision-making.
I think integrating ChatGPT with rolling stock technology can be a game-changer, but we need to prioritize safety measures.
Absolutely, Alex! Safety must always be the top priority in these technological advancements.
I'm curious about the potential impact on maintenance and troubleshooting procedures.
Good point, Rachel! ChatGPT could assist technicians in diagnosing issues more efficiently.
Having AI-powered support during maintenance could reduce downtime and increase overall productivity.
I wonder how ChatGPT would handle unexpected situations or emergencies on the train.
Good question, Tom! Handling emergency situations would require careful AI training and human intervention.
I assume privacy concerns would arise when it comes to implementing ChatGPT in public transportation.
You're right, Jeff! It's crucial to ensure passenger data is protected and not misused.
The article didn't cover potential challenges in maintaining and updating the AI models used.
Great point, Rachel! Adapting and improving AI models over time is indeed a significant challenge.
I wonder if ChatGPT could also be utilized for ticketing or fare information.
Interesting idea, Sarah! ChatGPT could provide quick and accurate responses to ticketing queries.
It could even enable contactless ticketing, especially in the current COVID-19 situation.
Absolutely, Mark! AI-powered contactless ticketing would be convenient and hygienic.
One concern could be the reliability of ChatGPT's responses in scenarios with poor internet connectivity.
Good point, Emily! A reliable internet connection would be crucial for the seamless operation of ChatGPT.
I think involving passengers in the development process would be important to address their needs.
Absolutely, Tom! Passenger feedback can provide valuable insights for enhancing the technology.
Involving passengers can also help identify potential flaws or limitations of ChatGPT.
That's true, Alex! User input is critical for ensuring the system meets the expectations and requirements.
While ChatGPT has immense potential, it should complement the human element, not replace it.
Well said, Lisa! Human expertise and decision-making are still indispensable.
I can see ChatGPT being a helpful tool for providing information to people with disabilities.
Definitely, Sarah! It could offer personalized support and accessibility-related information.
I'm excited about the potential of ChatGPT, but it should undergo rigorous testing and evaluation.
Agreed, Mark! Thorough testing ensures reliability and identifies any limitations or biases.
The integration of ChatGPT could also involve significant infrastructure upgrades.
You're right, Rachel! Implementing ChatGPT would require compatible hardware and software.
Considering potential technical glitches, human oversight is crucial for overriding incorrect AI decisions.
Absolutely, Tom! Humans should have ultimate authority in critical decision-making processes.
The article made me consider how ChatGPT can facilitate better communication between staff and passengers.
That's a great point, Alex! It could improve the overall customer service experience.
Indeed, Sarah! Real-time communication and assistance can lead to increased passenger satisfaction.
Plus, ChatGPT could ensure consistent information delivery across different staff members.
True, Rachel! It would reduce confusion or inconsistencies caused by varying staff knowledge.
We've had some great discussions here! It's fascinating to explore the potential of AI in rolling stock.
I agree, Lisa! This discussion showcased the potential and challenges associated with AI in rolling stock technology.
Absolutely, Alex! It's been great exploring different aspects of this innovative approach.
Agreed, Lisa! These conversations highlight the benefits and considerations surrounding its implementation.
Thank you all for engaging in this insightful discussion! Your perspectives have been valuable.
Likewise, Jeff! It's been a pleasure sharing and learning from everyone.
I thoroughly enjoyed this conversation! Thanks to each of you for contributing your thoughts.
Thank you, Rachel! It's been an enlightening discussion indeed.
Thorough testing can ensure that ChatGPT meets the necessary safety and reliability standards.
Indeed, Sarah! Safety and reliability are of utmost importance when integrating AI technologies.
Sarah, your concerns about relying too much on AI are valid. We must prioritize human oversight.
Absolutely, Lisa! We shouldn't solely rely on AI and should always maintain human control and supervision.
Lisa, I completely agree. The human element in rolling stock should always be a vital part of decision-making.
Well said, Tom! AI should assist and augment human capabilities, but not replace them entirely.
It was great discussing the potential impact of ChatGPT in rolling stock. Let's stay curious about future developments!
Involving passengers in the development process would lead to user-friendly features and address diverse needs.
Definitely, Alex! Incorporating passengers' perspectives is crucial to designing an inclusive and reliable system.
Thank you, Jeff! It's been an engaging discussion with diverse perspectives and insights.
Thank you, Jeff! It was a pleasure participating in this insightful discussion with everyone.
ChatGPT could potentially enhance ticketing and fare information, improving the overall experience for passengers.
AI-powered contactless ticketing would indeed be a convenient and hygienic alternative.
Human control and supervision are essential factors to ensure AI systems are reliable and trustworthy.
Absolutely, Rachel! Continuous curiosity and exploration will drive the future advancements in rolling stock.
Minimizing physical contact and increasing efficiency are crucial considerations for modernizing public transportation.
Sarah, you raise a great point! AI has the potential to enhance accessibility for people with disabilities.
Thank you all for sharing your valuable insights and making this discussion so enriching.
Infrastructure upgrades will indeed play a significant role in harnessing the full potential of ChatGPT.
Agreed, Mark! A robust infrastructure will ensure seamless integration and operation of ChatGPT.
Finding the right balance between AI and human involvement will be crucial in implementing rolling stock solutions.
Absolutely, Alex! Human judgment and expertise should always complement AI technologies.
Indeed, accessibility should be a key consideration in implementing new technology in public transportation.
Safety should be the topmost priority in rolling stock technology advancements.
Absolutely, Rachel! We should prioritize passenger and staff safety above all else.
Human oversight is vital to ensure AI systems in rolling stock operate reliably and responsibly.
I completely agree, Mark! Human judgment is necessary to avoid misinterpretations or errors.
Correct, Sarah! Human intervention is crucial when AI systems encounter complex or ambiguous situations.
Real-time communication facilitated by ChatGPT can significantly enhance the overall travel experience.
ChatGPT's ability to provide accurate and quick responses would greatly benefit ticketing and fare information services.
Definitely, Emily! It can streamline the ticketing process and improve operational efficiency.
A robust and reliable infrastructure is essential for the successful implementation of ChatGPT in rolling stock.
I agree, Tom! Infrastructure upgrades will be necessary to support the seamless integration of AI technologies.
AI and human collaboration is the way forward in revolutionizing real-time passenger support.
Absolutely, Mark! Combining the strengths of AI and human expertise can lead to exceptional passenger experiences.
Providing personalized support and accessibility information for people with disabilities is a promising idea.
Indeed, Sarah! Implementing AI with a focus on enhancing accessibility can significantly improve inclusivity.
The successful implementation of ChatGPT in rolling stock requires careful planning and consideration of infrastructure needs.
You're absolutely right! Infrastructure readiness is a crucial aspect to ensure seamless AI integration.
Human judgment and intervention are necessary to address ethical concerns related to AI in rolling stock.
Ethical guidelines and frameworks need to be established to govern the use of AI in transportation.
AI can significantly improve real-time passenger support, but human oversight is essential to handle exceptional cases.
Better communication between staff and passengers leads to improved customer satisfaction and operational efficiency.
Considering maintenance and updates is crucial for keeping the AI models running smoothly and efficiently.
Absolutely, Sarah! Regular updates and maintenance will ensure optimal performance of the AI models.
AI-powered contactless ticketing not only improves hygiene but also offers a faster and more convenient experience.
Indeed, Rachel! Contactless ticketing aligns with the overall focus on improving efficiency and user experience.
Improved communication can lead to reduced misunderstandings and better service quality overall.
Absolutely, Sarah! Clear and timely communication minimizes inconvenience and improves the passenger experience.