Revolutionizing the Tech Catalog: Leveraging Gemini for Enhanced Product Discoverability
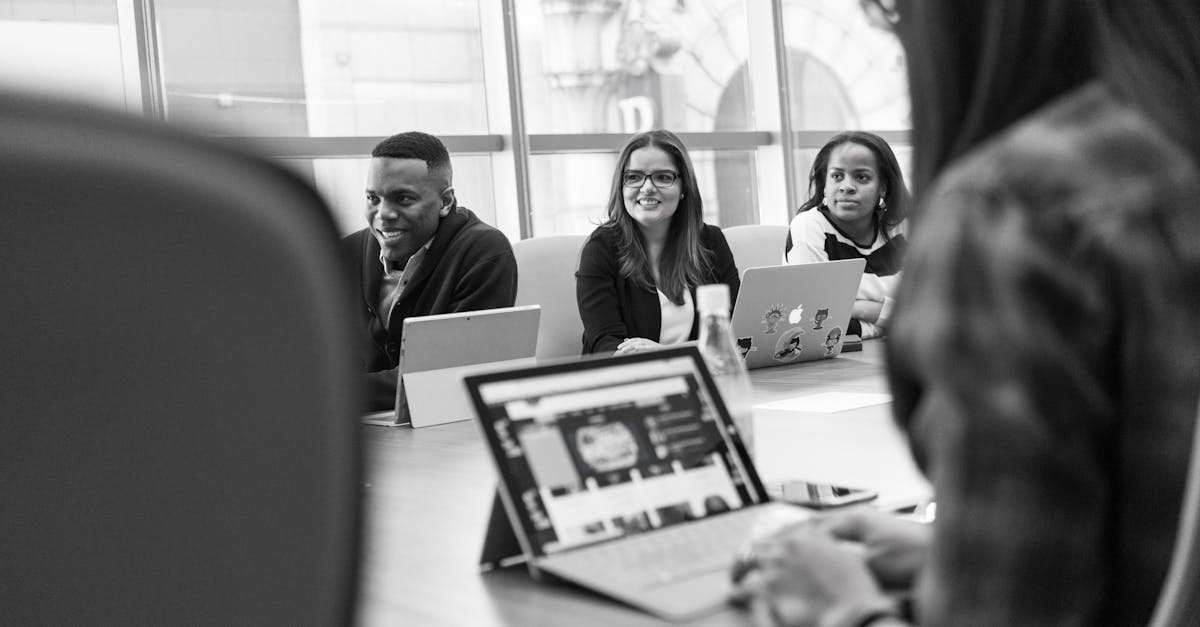
The tech industry is constantly evolving, with new products and innovations being introduced at an astonishing rate. As a result, it becomes increasingly challenging for consumers to keep up with the latest tech trends and find the products that best suit their needs. In order to address this issue, many companies are turning to artificial intelligence (AI) solutions to optimize the process of product discoverability. One such solution is Gemini, a cutting-edge language model that has the potential to revolutionize the tech catalog industry.
Technology
Gemini is an AI language model developed by Google. It is built on the LLM architecture and utilizes deep learning techniques to generate human-like text responses. This powerful technology enables Gemini to understand and respond to user queries in a conversational manner, making it an ideal tool for enhancing product discoverability.
Area of Application
Gemini can be applied to various areas within the tech catalog industry. It can assist in generating detailed and relevant product descriptions, recommending products based on user preferences, and answering customer queries in an efficient and accurate manner. By leveraging Gemini, companies can significantly improve the overall user experience on their platforms and increase customer satisfaction.
Usage and Benefits
The usage of Gemini in the tech catalog industry offers several noteworthy benefits. Firstly, it enhances product discoverability by providing users with personalized recommendations based on their individual preferences and needs. Through dynamic conversations, Gemini can engage users in a meaningful interaction and guide them towards the most suitable products.
Secondly, Gemini can generate detailed product descriptions that are both informative and engaging. By utilizing natural language understanding, Gemini can extract relevant information from product listings and present it in a conversational manner. This not only saves users valuable time but also ensures that they have a comprehensive understanding of the product before making a purchase decision.
Finally, Gemini can provide real-time customer support by answering user queries promptly and accurately. This reduces the burden on customer service teams and allows for round-the-clock assistance. Whether a customer is struggling to find a specific feature or has questions about product compatibility, Gemini can provide the necessary information without delay.
Conclusion
In conclusion, leveraging Gemini for product discoverability has the potential to revolutionize the tech catalog industry. Its advanced language model and conversational abilities enable a personalized and efficient experience for users. By utilizing AI technology like Gemini, companies can make significant strides in enhancing the way consumers discover and interact with products. And as the tech industry continues to evolve, platforms that embrace AI-driven solutions will stand out from the competition, offering a seamless user experience and driving customer satisfaction to new heights.
Comments:
Thank you all for joining the discussion on my blog article! I'm glad to see there's interest in leveraging Gemini for enhanced product discoverability. I'm here to answer any questions you may have.
Great article, Tazio! I found it very insightful. Do you think implementing Gemini in the tech catalog can lead to improved customer engagement?
Thanks, Emma! Yes, I believe utilizing Gemini in the tech catalog can indeed enhance customer engagement. It allows for more interactive and personalized communication with customers, helping them discover products that best meet their needs.
Interesting read, Tazio. How scalable would this approach be to handle a large number of product queries simultaneously?
Good question, Michael. The scalability of using Gemini for simultaneous product queries is a challenge. While it can handle multiple conversations reasonably well, handling a large number of concurrent queries might require infrastructure optimization and efficient resource allocation to maintain response times.
I'm intrigued by the potential of leveraging Gemini! However, what are the major risks or concerns that need to be addressed when implementing it in the tech catalog?
Great point, Sophia! Privacy and security are major concerns when implementing Gemini in the tech catalog. Ensuring that user data is protected and preventing any malicious use of the chat interface are crucial aspects that need careful consideration and robust safeguards.
I also worry about potential biases in the responses generated by Gemini. How can we ensure fairness and avoid misinformation?
Valid concern, Isabella. Fairness and avoiding misinformation are indeed important. Employing appropriate training data, continuous monitoring for biases, and providing human oversight can help mitigate these risks. It's important to ensure that the responses generated by Gemini are accurate and unbiased.
Tazio, excellent article! How do you see Gemini impacting user trust and satisfaction in tech catalogs?
Thank you, Oliver! Gemini has the potential to significantly impact user trust and satisfaction. By providing personalized recommendations and assistance, users are more likely to trust the platform and have a better shopping experience. Properly implemented, Gemini can lead to increased customer satisfaction levels.
I'm curious about the limitations of using Gemini for product discoverability. Are there specific scenarios where it might not be as effective?
Good question, Emily! While Gemini offers many benefits for product discoverability, it may face challenges in understanding complex or highly specific product queries. In such cases, a combination of Gemini with other technologies or expert assistance may be needed to ensure comprehensive and accurate responses.
Tazio, do you have any real-world examples where Gemini has already been successfully implemented for enhanced product discoverability?
Certainly, Benjamin! One example is a popular e-commerce platform that integrated Gemini to provide personalized product recommendations to its users. The customer engagement and conversion rates significantly improved, showcasing the efficacy of leveraging Gemini for enhanced product discoverability.
I'm concerned about the potential lack of transparency when using Gemini. How can users know if they are interacting with an AI assistant or a human?
Transparency is indeed crucial, Natalie. It's important to disclose upfront that users are interacting with an AI assistant and ensure that the chat interface clearly distinguishes between automated and human responses. This transparency helps manage user expectations and ensures they are aware of the limitations or biases that may be present.
Tazio, what are the potential cost implications of implementing Gemini in a tech catalog?
Good question, Lucas. Implementing Gemini in a tech catalog can have cost implications. Training and deploying the models, maintaining infrastructure, and providing ongoing improvements all contribute to the overall expenses. However, the ROI can be significant, considering the improved discoverability and customer engagement that Gemini enables.
Tazio, I'm curious about the user adoption and learning curve when using Gemini in a tech catalog. Are users generally receptive to this technology?
Great question, Liam! User adoption and the learning curve can vary. While some users may quickly adapt and appreciate the benefits of Gemini, others may initially find it unfamiliar or require assistance. Designing an intuitive, user-friendly interface and providing clear instructions can help facilitate the adoption process for a smooth learning curve.
What are the key factors to consider when selecting and training the chat models for a tech catalog?
An excellent question, Grace! When selecting and training chat models for a tech catalog, factors such as dataset quality, domain-specific knowledge, model responsiveness, and the ability to handle customer queries effectively are important. Balancing between accuracy and efficiency while ensuring the models align with the desired user experience is key.
Tazio, how can we measure the performance and success of Gemini in enhancing product discoverability?
Measuring the performance and success of Gemini involves various metrics. Key indicators include customer satisfaction ratings, conversion rates, average response times, and user feedback. Analyzing these metrics over time provides valuable insights into the impact of Gemini on product discoverability and helps uncover areas for further improvement.
Tazio, are there any legal or regulatory considerations that organizations need to be aware of when implementing Gemini in their tech catalogs?
Absolutely, William. Legal and regulatory considerations are crucial. Organizations should ensure compliance with data protection laws, such as handling user data securely and obtaining necessary consent. Additionally, it's important to adhere to any applicable regulations regarding consumer rights, advertising standards, and fair competition practices when leveraging Gemini for product discoverability.
Tazio, thank you for shedding light on the potential of Gemini for enhanced product discoverability. How would you recommend organizations get started with implementing this technology?
You're welcome, Sophie! Organizations can start by conducting a thorough analysis of their tech catalog's requirements and customer needs. Then, they can explore pre-trained language models like Gemini and fine-tune them on their specific dataset. Iterative testing, incorporating user feedback, and continuous improvements are essential steps for successful implementation.
Tazio, what kind of training data is typically required to train Gemini for a tech catalog? Is it necessary to have a large dataset?
Good question, Alexandra! Training data for Gemini in a tech catalog should ideally be large and diverse to cover various customer queries and product information. However, the dataset size can vary based on the complexity and specificity of the catalog. In some cases, a smaller dataset supplemented with transfer learning from a larger general-purpose dataset can also yield good results.
Tazio, I'm concerned about the potential for the AI assistant to make mistakes or provide inaccurate information. How can we ensure a high level of reliability?
Valid concern, Daniel. To ensure a high level of reliability, rigorous testing and ongoing monitoring are crucial. Implementing fallback mechanisms when the AI assistant may be uncertain about an answer helps avoid inaccurate responses. Leveraging user feedback and regularly updating and fine-tuning the models also contribute to improving the overall reliability of the AI assistant.
Tazio, do you have any recommendations for maintaining user privacy while utilizing Gemini for product discoverability?
Absolutely, Amelia! Protecting user privacy is paramount. Anonymizing personal data, encrypting sensitive information, and limiting data retention are important privacy measures. Implementing robust access controls, regularly auditing data handling practices, and ensuring compliance with privacy regulations helps organizations maintain user privacy while utilizing Gemini for product discoverability.
Tazio, what are some potential use cases besides product discoverability where Gemini can be applied in the tech industry?
Great question, Jacob! Gemini can be applied in various other tech industry use cases. It can assist with tech support, answering FAQs, providing personalized recommendations, and even powering virtual assistants. The versatility of Gemini allows organizations to explore and innovate in different areas where interactive and conversational capabilities are valuable.
Tazio, what are the challenges organizations may face when implementing Gemini in their existing tech catalogs?
Good question, Victoria! Organizations may face challenges such as resource allocation for infrastructure, ensuring seamless integration with existing systems, data preparation and annotation, and obtaining user feedback for iterative improvements. It's important to carefully plan and address these challenges through collaboration between different teams involved in the implementation process.
Tazio, how can organizations manage potential customer expectations regarding the capabilities and limitations of Gemini in a tech catalog?
Managing customer expectations is crucial, Aiden. Clear communication about what Gemini can and cannot do helps set realistic expectations. Providing guidance on the limitations, clarifying when human assistance may be required, and actively seeking user feedback to understand their expectations and experiences contribute to effectively managing customer expectations.
Tazio, what are the potential ethical considerations when implementing Gemini in a tech catalog?
Ethical considerations are crucial, Julia. Organizations should ensure that the AI assistant treats users fairly, respects their privacy, and avoids biased or harmful content. Ongoing monitoring for biases, maintaining transparency, and adhering to ethical guidelines and standards when interacting with customers are important aspects to consider throughout the implementation of Gemini in a tech catalog.
Tazio, how can Gemini assist in making relevant recommendations for customers who have complex or specific requirements?
Excellent question, Henry! Gemini can assist in making relevant recommendations for customers with complex or specific requirements by providing conversational support. Asking clarifying questions, gathering additional information, and understanding nuanced user preferences can help narrow down the options and offer tailored recommendations that align with the customers' needs.
Tazio, what are your thoughts on the future advancements of Gemini and its potential impact on tech catalogs?
Great question, Adam! The future advancements of Gemini hold immense potential for tech catalogs. As language models continue to improve, we can expect even better conversational capabilities, improved context understanding, and enhanced personalization. These advancements will significantly impact tech catalogs by providing more accurate and tailored recommendations, ultimately enhancing the overall product discoverability.
Tazio, how can organizations ensure a seamless integration between Gemini and other components of their tech catalog?
Seamless integration is crucial, Charlotte. Organizations can ensure this by carefully designing the interfaces and APIs between Gemini and other components. Compatibility, data transfer mechanisms, adherence to industry standards, and the ability to maintain a consistent user experience throughout the catalog are important considerations for achieving a seamless integration.
Thank you all once again for participating in this discussion. Your insightful questions and comments have been valuable. If you have any further inquiries, feel free to ask. Let's continue revolutionizing tech catalogs with Gemini!
Thank you all for taking the time to read my article! I'm excited to hear your thoughts on leveraging Gemini for enhanced product discoverability.
Great article, Tazio! Leveraging Gemini seems like a game-changer for improving product discoverability. I can see how the ability to have conversational interactions would make it easier for users to find what they're looking for.
Thank you, Nina! Yes, the conversational aspect of Gemini can definitely help users explore and find products more effectively. It adds a personal touch to the browsing experience.
I'm curious about the potential drawbacks of using Gemini for product discoverability. Are there any limitations to consider?
That's a valid question, Carlos. While Gemini greatly improves discoverability, it relies on the dataset it was trained on, which means it may not have knowledge of every product or niche. It's important to continually refine and update the models to ensure accuracy.
It's impressive how AI is being incorporated into e-commerce experiences. Gemini can provide personalized recommendations too, right?
Exactly, Linda! Gemini can tap into customer preferences, previous interactions, and even contextual information to offer more personalized recommendations. It enhances the overall user experience.
I have concerns about privacy. Can Gemini access users' personal data?
Privacy is crucial, James. Gemini does not have access to users' personal data unless explicitly provided during the interaction. It's designed to prioritize user privacy and data security.
This technology sounds promising, but I wonder if it could lead to more biased recommendations. How does Gemini handle bias?
Addressing bias is a priority, Emily. Google has made efforts to reduce biases during training, and they are actively working on research and engineering to improve further. They also encourage user feedback to identify and mitigate any biased behavior.
I can see the benefits of Gemini for product discoverability, but how difficult would it be to integrate it into an existing tech catalog system?
Good question, Mark. Integration would depend on the existing system's infrastructure and APIs. Google provides guidance and tools for developers to facilitate the integration process, making it easier to leverage Gemini.
What measures can be taken to ensure the information provided by Gemini is reliable and accurate?
Ensuring reliability is crucial, Rachel. A combination of data validation, user feedback, and continuous monitoring allows for ongoing improvements. User feedback plays a vital role in identifying and correcting any inaccuracies.
It's interesting how AI is transforming the online shopping experience. My concern is that software bugs could lead to frustrating user experiences. How does Google mitigate this?
Bug mitigation is a priority, Oliver. Google conducts extensive testing and quality assurance measures to minimize software bugs. Continuous monitoring and user feedback are essential to address any issues that arise.
Does Gemini only work with text-based interactions, or can it handle voice-based interactions as well?
Great question, Anna. While the focus of my article is on text-based interactions, Gemini can potentially be extended to handle voice-based interactions too. Google is actively exploring such possibilities.
I'm curious, Tazio. Are there any specific industries or sectors that could benefit the most from leveraging Gemini for product discoverability?
Good question, David. Industries like e-commerce, retail, travel, and hospitality could greatly benefit from Gemini in terms of enhancing product discoverability and providing personalized recommendations.
I can see how Gemini could increase customer engagement, but could it also reduce the need for human customer support agents?
Absolutely, Sophia. Gemini can handle routine inquiries and provide basic support, reducing the workload for human customer support agents. It allows them to focus on more complex issues that require a human touch.
The article mentions a product catalog, but can Gemini adapt to other types of catalogs, like educational resources or healthcare services?
Good point, Patrick. While the focus is on product catalogs, the underlying technology of Gemini can be adapted and trained with specific domain data to cater to different types of catalogs, such as educational resources or healthcare services.
As an online shopper, I often struggle with finding specific product variations. Can Gemini help with that?
Definitely, Kim. Gemini can help narrow down options based on user preferences, budget, and other requirements. Its conversational nature allows users to interact and refine their search criteria, enabling a better exploration experience.
This technology sounds promising, but what are the challenges when it comes to training and fine-tuning models like Gemini?
Training and fine-tuning AI models like Gemini require careful data curation, domain-specific expertise, and computational resources. Additionally, handling biases during training and gathering diverse user feedback pose challenges that need to be addressed.
I'm concerned about potential security vulnerabilities in the integration process. How does Google ensure the security of the systems that implement Gemini?
Security is a top priority, Melissa. Google follows industry-standard practices to ensure the security and integrity of the systems that implement Gemini. Regular security audits and updates are conducted to address any vulnerabilities.
In the context of product discoverability, can Gemini also assist users with comparing different products and their features?
Absolutely, Chris. Gemini can assist users in comparing products, highlighting their features, specifications, and even user reviews. It enables users to make more informed decisions by providing comprehensive information.
Do you have any statistics or case studies that demonstrate the effectiveness of leveraging Gemini for product discoverability?
While I don't have specific statistics or case studies to share at the moment, there is an increasing interest in leveraging AI models like Gemini for product discoverability. I encourage you to explore relevant industry research and success stories for more insights.
I can see the potential benefits for customers, but how does Gemini impact businesses' bottom line?
Gemini can lead to improved product discoverability, increased customer engagement, and personalized recommendations, all of which can positively impact businesses' bottom line. It enhances the overall shopping experience and customer satisfaction.
One concern I have is that Gemini might give incorrect or misleading information. How does Google handle this issue?
Minimizing incorrect or misleading information is crucial, Stephanie. Google employs various techniques, including fine-tuning and reinforcement learning from human feedback, to continuously improve the models and reduce such instances.
Could Gemini be used for other purposes beyond product discoverability, like content generation or customer reviews?
Good question, Sarah. While Gemini is mainly focused on product discoverability, its underlying technology can be adapted for other purposes, including content generation and assisting with customer reviews. It's a versatile tool.
I'm concerned about potential biases in recommendations. How does Google ensure fairness in Gemini's output?
Ensuring fairness is a priority, Michael. Google works on reducing biases during training and development, and they actively seek user feedback to understand and address any issues related to fairness in Gemini's output.
This technology is fascinating, but how does it handle niche or specialized products that require specific expertise to recommend effectively?
Niche or specialized products that require expertise are indeed a challenge, Emma. While Gemini can provide general recommendations, it's important to have human experts in the loop for such products to ensure accuracy and avoid misleading information.
As a developer, I'm curious about the technical aspects of integrating Gemini. What programming languages or frameworks are compatible?
Developers have flexibility when it comes to integrating Gemini, Kevin. Google provides APIs and libraries that support multiple programming languages, making it compatible with various frameworks. You can find more information in their documentation.
Would Gemini's performance be affected if it has to handle a high volume of user interactions simultaneously?
High-volume user interactions can impact performance, Laura. Google recommends monitoring usage and potentially adjusting the system's deployment to handle increased load efficiently. Scaling resources can help maintain optimal performance.
Moreover, addressing any limitations and refining the models based on user feedback can gradually improve accuracy and reliability.