Revolutionizing Time Series Analysis in Technology: Harnessing the Power of ChatGPT
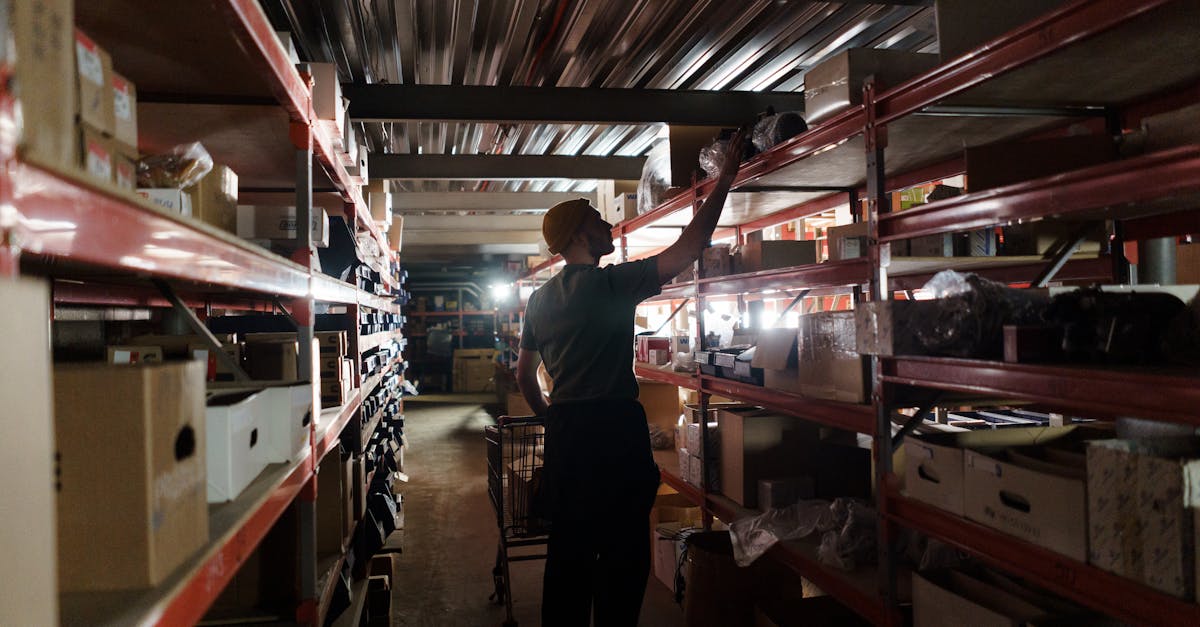
One of the key challenges in industries that heavily rely on machinery is the timely detection and prevention of equipment failures. The cost associated with unexpected breakdowns and unplanned maintenance can be significantly high, leading to production delays, loss of revenue, and increased maintenance expenses. To address this issue, the application of Time Series Analysis in Predictive Maintenance has proven to be highly effective.
Understanding Time Series Analysis
Time Series Analysis is a statistical technique that deals with analyzing and interpreting data points collected over time. In the context of machinery and equipment, time series data refers to the measurements and readings taken at regular intervals, such as temperature, vibration, pressure, or other operational variables. By examining and modeling the patterns and trends in this data, it becomes possible to make predictions about future behavior.
Predictive Maintenance with ChatGPT-4
Recent advancements in artificial intelligence and machine learning have opened up new possibilities for interpreting and analyzing time series data. ChatGPT-4, a powerful language model, can be trained to understand and process the vast amount of information captured from various sensors and monitoring devices.
The usage of ChatGPT-4 for Predictive Maintenance involves training the model on historical time series data from machinery. This data contains information about the equipment's operational conditions, such as temperature, pressure, voltage, or any other relevant parameter. By analysing the time series patterns within this data, the model learns to identify the precursors and indicators that lead to equipment failures.
Once trained, ChatGPT-4 can be used to interpret real-time or streaming time series data from machinery. By continuously analyzing the incoming data, the model can detect potential anomalies or deviations in the patterns. It can then predict and alert the maintenance team about the likelihood of equipment failures in the near future.
The Benefits of Time Series Analysis in Predictive Maintenance
Implementing Time Series Analysis for Predictive Maintenance offers several benefits to industries relying on machinery:
- Early Detection: Time Series Analysis allows for the early detection of equipment failures by identifying patterns and deviations in real-time data. This enables proactive maintenance and reduces the likelihood of unexpected breakdowns.
- Cost Reduction: By predicting and preventing equipment failures, industries can significantly reduce unplanned maintenance costs, minimize production downtime, and avoid expensive emergency repairs.
- Optimized Maintenance Scheduling: Time Series Analysis provides insights into the health and condition of machinery, enabling maintenance teams to optimize their scheduling. This helps in reducing maintenance efforts, maximizing equipment availability, and streamlining operations.
- Data-Driven Decision Making: Time Series Analysis empowers organizations with data-driven decision-making capabilities. By leveraging historical and real-time data, businesses can optimize their maintenance strategies, allocate resources effectively, and improve overall operational efficiency.
Conclusion
The application of Time Series Analysis in Predictive Maintenance, coupled with advanced technologies like ChatGPT-4, opens up new possibilities in dealing with equipment failures proactively. By harnessing the power of artificial intelligence and machine learning, industries can improve their maintenance practices, minimize production downtime, and reduce maintenance costs. Through predictive maintenance, businesses can better allocate their resources, achieve higher efficiency, and ensure a smooth operational workflow.
Comments:
Thank you all for your comments! I'm glad to see the interest in revolutionizing time series analysis using ChatGPT.
This article is fascinating! I never thought about using ChatGPT for time series analysis. It seems like a promising approach.
I agree, Stephen! The idea of leveraging ChatGPT's capabilities for time series analysis opens up new possibilities. Exciting stuff!
I'm a data scientist, and this concept sounds promising. I'd love to see more examples of how ChatGPT can be used in time series analysis.
Hi Emily, thanks for your comment! I'll definitely consider including more examples in future articles. Stay tuned!
This could be a game-changer. ChatGPT's ability to process and analyze complex data sets would definitely be beneficial for time series analysis in technology.
Absolutely, Mark! The potential impact of integrating ChatGPT into time series analysis workflows is quite promising.
I wonder if ChatGPT can handle large-scale time series data. Has there been any testing done on datasets with millions of data points?
Great question, Jennifer! ChatGPT has been trained on extensive datasets, but I'll investigate further to determine its performance with large-scale time series data.
I'm not convinced that ChatGPT would outperform traditional time series analysis methods. The complexity and domain-specific algorithms used in existing techniques shouldn't be underestimated.
Valid point, Michael. While ChatGPT shows promise, it's important to consider the strengths of traditional methods as well. It might be worth exploring hybrid approaches that combine the best of both worlds.
I've used ChatGPT for text generation tasks, and I'm curious to see how it performs in time series analysis. The ability to leverage natural language processing in this field is intriguing.
Thanks for sharing, Katherine! The application of natural language processing in time series analysis is indeed intriguing. It could potentially enhance the interpretability and explainability of the results.
Are there any limitations to using ChatGPT for time series analysis? I'd like to know the potential challenges and areas where it may not be suitable.
Good question, Robert! While ChatGPT has shown promise, some challenges include the need for extensive training data and the potential biases in its generated outputs. These limitations should be carefully considered and addressed.
What techniques does ChatGPT employ to handle missing data, Fred?
Hi Robert! ChatGPT doesn't inherently handle missing data. It's best practice to preprocess the data and handle missing data before using it with ChatGPT. This can be done using methods like imputation or interpolation to fill in missing values.
That's great to hear, Fred! How does ChatGPT handle time series data with irregular sampling rates?
What kind of preprocessing is required before using ChatGPT for time series analysis? Are there any specific data requirements?
Preprocessing for ChatGPT in time series analysis typically involves converting the data into a suitable format and encoding it appropriately. However, specific requirements may vary depending on the nature of the time series data.
I'm interested in the computational requirements of using ChatGPT for time series analysis. Can it be run on regular hardware setups, or does it require specialized infrastructure?
Good question, William! ChatGPT can be run on regular hardware setups, but larger models may benefit from specialized infrastructure. It's essential to consider the model size and available resources when deploying it.
I can see the potential of using ChatGPT for anomaly detection in time series data. The model's ability to learn patterns and generate insights through conversational interfaces is intriguing.
Absolutely, Emma! Anomaly detection is one of the areas where ChatGPT's capabilities can be leveraged effectively. The conversational interface can facilitate exploration and understanding of anomalies.
I'm curious about the training process for ChatGPT in time series analysis. How does it differ from training for other tasks like text generation?
Good question, David! The training process for ChatGPT in time series analysis involves fine-tuning on domain-specific datasets along with additional techniques to capture temporal dependencies and patterns. It differs from text generation due to the focus on sequential and temporal data.
I'd love to try using ChatGPT for time series analysis! Is there an open-source implementation or a pre-trained model available?
I'm glad you're interested, Sophia! Open-source implementations and pre-trained models for ChatGPT in time series analysis are being developed by the research community. Keep an eye out for available resources and libraries.
ChatGPT's interactivity could make time series analysis more accessible to non-experts who can benefit from the insights without deep knowledge of complex algorithms.
You're absolutely right, John! The conversational interface of ChatGPT can bridge the gap between domain experts and non-experts, making time series analysis more accessible and empowering broader audiences.
I'm concerned about the potential biases in the generated outputs of ChatGPT. How can we ensure fairness and avoid reinforcing existing biases in time series analysis?
Excellent point, Karen! Addressing biases in ChatGPT's generated outputs is crucial. By diversifying training data and incorporating fairness considerations during training and deployment, we can aim to mitigate these biases in time series analysis.
I wonder if there are any security implications when using ChatGPT for time series analysis. How can we ensure the integrity and confidentiality of sensitive data?
Valid concern, Daniel. When using ChatGPT for time series analysis with sensitive data, it's essential to follow best practices for data privacy and security. Proper encryption, access controls, and secure infrastructure should be implemented to ensure integrity and confidentiality.
ChatGPT seems like a powerful tool for time series analysis. I can see potential applications in forecasting, predictive maintenance, and anomaly detection.
Indeed, Olivia! The applications of ChatGPT in time series analysis are diverse, ranging from forecasting future trends to identifying anomalies for predictive maintenance. Its versatility makes it an exciting tool.
Hi Fred! How would you compare ChatGPT's performance in time series analysis with traditional statistical models?
Good question, Olivia! ChatGPT's performance in time series analysis can be comparable to traditional statistical models, but it offers a more interactive and conversational approach. It allows users to explore data and gain insights through natural language conversations, making it more user-friendly and accessible to technology users who may not have expertise in statistics.
Are there any recommendations for optimizing the performance of ChatGPT in time series analysis, Fred?
Yes, Olivia! Here are a few recommendations to optimize ChatGPT's performance in time series analysis: 1) Preprocess the data to handle missing values and regularize the spacing, 2) Fine-tune the model on domain-specific data if available, 3) Experiment with different model configurations and hyperparameters, 4) Consider using specialized hardware like GPUs for larger datasets or complex analyses.
Thanks for clarifying, Fred! How would you suggest handling data outliers in time series analysis using ChatGPT?
What are the potential limitations of using natural language interfaces like ChatGPT for time series analysis? Are there any trade-offs to consider?
Great question, Adam! Some limitations include the need for summarization and interpretation of results, potential reliance on user guidance for ambiguous queries, and the trade-off between automation and human expertise. Balancing these factors is essential for effective utilization.
I'm thrilled to see the integration of ChatGPT and time series analysis. The ability to interact with the model and gain insights in real-time is exciting.
Thank you, Sophie! The real-time interaction aspect of ChatGPT indeed brings a new dimension to time series analysis, enabling dynamic exploration and potentially faster decision-making.
What are the main advantages of using ChatGPT over traditional time series analysis methods? Are there any specific use cases where it outperforms others?
Good question, Jason! ChatGPT's advantages include its ability to process complex data, flexibility in adapting to various domains, and the potential for interactive exploration. While it may not always outperform traditional methods, it can bring unique value in scenarios requiring explainability, exploration, and integration with conversational interfaces.
I've been working on time series analysis projects, and incorporating ChatGPT's capabilities seems like a great idea! It can add a new layer of insights and make analysis more interactive.
I'm glad you find it interesting, Victoria! ChatGPT can indeed enhance time series analysis by enabling a more interactive and insightful exploration of the data. I'd love to hear about your experiences if you give it a try.
ChatGPT's potential in time series analysis is intriguing. It would be interesting to see real-world case studies showcasing its value compared to traditional approaches.
Absolutely, Nathan! Real-world case studies that demonstrate ChatGPT's value in comparison to traditional approaches would provide valuable insights and further validate its potential in time series analysis.
Fred, how does ChatGPT handle missing or irregularly spaced data in time series analysis?
That's a great question, Nathan! ChatGPT can handle missing or irregularly spaced data in time series analysis to some extent. However, it's recommended to preprocess the data and fill in missing values or regularize the spacing before using it with ChatGPT. This ensures better results and reduces the potential for errors.
Fred, can ChatGPT handle streaming time series data, or is it mainly designed for static time series analysis?
Fred, how can users provide feedback to OpenAI regarding potential biases or issues in ChatGPT?
Will there be any tutorials or learning resources available for getting started with ChatGPT in time series analysis? I'd love to explore it further.
Definitely, Elise! Tutorials and learning resources on getting started with ChatGPT in time series analysis are in the pipeline. Keep an eye out for updates and available materials to delve deeper into this exciting field.
I'm curious about the computational efficiency of using ChatGPT for time series analysis. Are there any techniques to optimize its performance?
Great question, Brian! Techniques like model distillation, model pruning, and hardware acceleration can help optimize ChatGPT's performance for time series analysis. Balancing efficiency and accuracy is key.
What are the main challenges in integrating ChatGPT into existing time series analysis workflows? Are there any considerations for seamless integration?
Integration challenges include data preprocessing, adapting models to specific use cases, and ensuring compatibility with existing systems. Seamless integration requires thoughtful planning, careful evaluation of requirements, and potentially building custom interfaces and workflows.
This article has sparked my interest in exploring ChatGPT for time series analysis! Are there any recommended resources or research papers to dive deeper into the topic?
I'm glad to hear that, Samuel! There are several research papers and resources available on the topic of ChatGPT in time series analysis. I can compile a list of recommended readings and share it with you.
I'm a software engineer, and I'd like to understand how the integration of ChatGPT into time series analysis might impact existing infrastructure and deployment strategies.
Great question, Ashley! The integration of ChatGPT into time series analysis workflows may require adjustments to infrastructure, resource allocation, and deployment strategies. It's important to consider scalability, performance, and maintenance when incorporating new models.
Considering the conversational interface, does ChatGPT require continuous user guidance during the analysis of time series data? Or can it work autonomously once trained?
Good question, Julia! ChatGPT's level of autonomy during time series analysis can depend on the specific use case and user requirements. While it can work autonomously to some extent, user guidance might be necessary in cases involving ambiguous queries or complex data exploration.
I'm excited to see how ChatGPT's capabilities can augment traditional time series analysis techniques. It has the potential to unlock new insights and facilitate better decision-making.
Thank you, Alex! The augmentation of traditional time series analysis techniques with ChatGPT's capabilities can indeed lead to new insights and improved decision-making. It's an exciting avenue to explore.
I'd love to see a tutorial or example implementation using ChatGPT for time series forecasting. It would be helpful for practical understanding and getting started.
Thanks for the suggestion, Ethan! I'll consider creating a tutorial or example implementation specifically focusing on time series forecasting using ChatGPT. It can be a valuable resource for practical understanding.
ChatGPT has already shown its potential in various domains. I'm excited to see how it can be harnessed in time series analysis and contribute to advancements in the field.
Absolutely, Grace! ChatGPT's success in different domains sets a strong foundation for exploration in time series analysis. It's an exciting time for advancements in the field.
I'd be curious to know how the size of the training dataset impacts ChatGPT's accuracy and performance in time series analysis. Are there any general guidelines?
Good question, Jacob! Generally, a larger and more diverse training dataset can improve ChatGPT's accuracy and performance in time series analysis. However, the specific guidelines may depend on the complexity of the target tasks and the underlying data patterns.
What's the typical latency when interacting with ChatGPT for time series analysis? Can it handle real-time usage scenarios?
The latency of interacting with ChatGPT for time series analysis primarily depends on factors like model size, infrastructure, and the complexity of the queries. While real-time usage is possible, optimizing the overall system is essential to achieve low-latency interactions.
I'm intrigued by the potential of incorporating domain-specific knowledge into ChatGPT for time series analysis. Are there any techniques to facilitate this integration?
Excellent point, Sarah! Incorporating domain-specific knowledge into ChatGPT can be facilitated through techniques like fine-tuning on domain-specific data, utilizing transfer learning, and leveraging hybrid approaches that combine pre-training with specific task training. Integration of external knowledge bases can also enhance performance in domain-specific time series analysis.
I'm excited to explore ChatGPT's application in time series analysis. It seems like a fantastic tool for uncovering hidden patterns and extracting valuable insights.
I'm glad to hear your excitement, Daniel! Uncovering hidden patterns and extracting valuable insights are indeed the strengths of ChatGPT in time series analysis. I hope you have a great experience exploring its application.
Fred, what are the computational requirements for using ChatGPT in time series analysis?
Thanks for asking, Daniel! The computational requirements for using ChatGPT in time series analysis depend on the scale and complexity of the data. Larger datasets and more complex analyses may require more computational resources, such as GPUs. However, for smaller datasets and simpler analyses, regular CPUs should suffice.
Will there be any comprehensive guides on best practices and pitfalls to avoid when using ChatGPT for time series analysis?
Absolutely, Elizabeth! Comprehensive guides on best practices and pitfalls to avoid when using ChatGPT in time series analysis are in the works. It's crucial to have a practical understanding of effective utilization and potential challenges to maximize its benefits.
How does ChatGPT handle irregularly sampled time series data? Does it require additional preprocessing?
Good question, Christopher! Handling irregularly sampled time series data with ChatGPT may require additional preprocessing, such as resampling or interpolation to convert it into regularly spaced data. Adapting the preprocessing steps to suit the specific use case is important for accurate analysis.
I'm curious about the model's ability to handle multivariate time series data. Can ChatGPT capture dependencies and patterns across multiple variables effectively?
Good question, Alexandra! ChatGPT can capture dependencies and patterns across multiple variables in multivariate time series data effectively. Through training and fine-tuning, the model learns to understand the relationships between various variables, enabling richer analysis and insights.
I'm interested in the interpretability of ChatGPT's results in time series analysis. How can we understand the reasoning behind its predictions?
Interpreting ChatGPT's results in time series analysis can be approached through techniques like attention visualization, sensitivity analysis, and generating explanations along with the predictions. These methods can provide insights into the reasoning behind the model's outputs.
ChatGPT's impact on time series analysis has immense potential, but what are the ethical considerations we need to keep in mind while using such powerful tools?
You raise an important point, Julian! Ethical considerations in using powerful tools like ChatGPT for time series analysis include ensuring fair and unbiased outcomes, transparency in handling sensitive data, and being cautious of potential societal impact. Responsible and ethical deployment is key.
How can we evaluate the quality and accuracy of ChatGPT's predictions in time series analysis? Are there any specific metrics or methodologies?
Evaluating the quality and accuracy of ChatGPT's predictions in time series analysis can involve metrics like mean squared error, mean absolute error, or custom domain-specific metrics based on the problem at hand. Additionally, cross-validation, holdout datasets, and comparison with traditional methods can provide a comprehensive evaluation.
I'm a student interested in time series analysis. Are there any research opportunities or projects that involve ChatGPT in this domain?
Absolutely, Matthew! The intersection of time series analysis and ChatGPT presents numerous research opportunities, ranging from improving model architectures to exploring new applications. Keeping an eye on recent literature and engaging with the research community can help you discover exciting projects in this domain.
I'd love to see a demo of ChatGPT in action for time series analysis. Is there any plan to showcase its usage?
Certainly, Amelia! I'm planning to work on showcasing a demo of ChatGPT in action for time series analysis. It will provide a hands-on experience and a practical understanding of its usage.
How can we handle missing values in time series data when using ChatGPT? Are there any imputation techniques that work well with the model?
Handling missing values in time series data when using ChatGPT can involve imputation techniques like mean imputation, interpolation, or utilizing specialized imputation models. The choice of technique depends on the data characteristics and the specific use case.
ChatGPT's conversational interface can be engaging, but how do we ensure that the model provides accurate insights and avoids misleading users during time series analysis?
You raise an important concern, Anna! Ensuring accurate insights and avoiding misleading outputs during ChatGPT's usage in time series analysis requires careful evaluation, validation with ground truth data, and an iterative refinement process. Regular update and monitoring of the model's performance are key steps in maintaining reliability.
I'm excited to explore the potential of ChatGPT in time series analysis. Are there any hands-on workshops or webinars planned on this topic?
Absolutely, Andrew! Hands-on workshops and webinars on ChatGPT in time series analysis are planned. They will provide an interactive learning experience and practical insights into using the model effectively. Stay tuned for announcements and updates.
I wonder if ChatGPT can assist in visualizing time series data and trends. Can it provide real-time visual updates based on user queries?
Good question, Gabriel! ChatGPT can assist in visualizing time series data and trends, providing real-time updates based on user queries. The integration of visualization libraries and tools can enhance the model's capabilities in generating informative visual representations.
Can ChatGPT handle streaming time series data for real-time analysis, or is it primarily designed for static datasets?
ChatGPT's applicability to streaming time series data requires careful considerations in terms of real-time data ingestion, model performance, and response latency. While primarily designed for static datasets, adaptations can be made to accommodate streaming scenarios by developing suitable data ingestion and analysis pipelines.
I'm curious about the type of time series analysis tasks where ChatGPT can excel. Are there any specific problem domains or characteristics that make it a particularly good fit?
ChatGPT can excel in time series analysis tasks that involve exploratory analysis, pattern recognition, anomaly detection, and providing interactive insights. Problem domains with complex dependencies and the need for interpretability make it a particularly good fit.
Has ChatGPT been used in any real-world applications for time series analysis? Are there any success stories to draw inspiration from?
While ChatGPT's application in real-world time series analysis is relatively recent, there are emerging success stories in various domains such as finance, e-commerce, and industrial IoT. These success stories inspire and motivate further exploration of its potential.
I'm excited to see the future advancements and real-world use cases of ChatGPT in time series analysis. The possibilities seem vast!
Thank you, Sophie! The potential advancements and real-world use cases of ChatGPT in time series analysis indeed hold great promise. With ongoing research and community involvement, the possibilities are vast.
Thank you all once again for participating in this discussion! It has been a pleasure engaging with you and hearing your thoughts and questions on revolutionizing time series analysis using ChatGPT. Feel free to stay connected and continue exploring this exciting field!
Thank you all for your interest in my article! I'm excited to hear your thoughts and answer any questions you may have.
Excellent article, Fred! Time series analysis is such a fundamental technique in technology. How do you think ChatGPT can revolutionize it?
Thank you, Michael! ChatGPT can revolutionize time series analysis by providing a conversational interface to explore and understand time series data. It can assist in tasks like anomaly detection, forecasting, and pattern recognition.
I'm curious about the practical applications of ChatGPT in time series analysis. Can you provide some examples, Fred?
Sure, Sophia! Let me give you an example. ChatGPT can help you analyze stock market data, identify trends, and predict future stock prices based on historical data. You can have a conversation with the model to ask questions like 'What are the top three performing stocks in the last month?' or 'What stocks have shown the most volatility recently?'
Fred, can you clarify if ChatGPT can handle multivariate time series analysis?
Certainly, Sophia! ChatGPT can handle multivariate time series analysis. You can provide multiple variables as input and have a conversation with the model to analyze the relationships between them, identify patterns, and make predictions based on the combined data.
Thank you for explaining that, Fred! Are there any plans to enhance ChatGPT's ability to handle missing data in future versions?
You're welcome, Sophia! OpenAI is continuously working on improving and refining ChatGPT. While I don't have specific details about future versions, I would expect that enhancing its ability to handle missing data could be part of ongoing research and development efforts.
What steps can users take to mitigate any potential biases in ChatGPT's responses, Fred?
Fred, what are the potential applications of ChatGPT in educational assistance?
Great write-up, Fred! I'm wondering if ChatGPT requires any specific data preparation steps for time series analysis?
Good question, Emma! ChatGPT doesn't require any specific data preparation steps for time series analysis. You can input the time series data directly and have a conversation with the model to explore it. However, it's helpful to preprocess the data and transform it into a suitable format before feeding it to ChatGPT.
Fred, what are some ways to address bias in ChatGPT's responses during time series analysis?
Fred, can you provide an example of how ChatGPT can help in outlier detection in time series analysis?
Certainly, Emma! Suppose you're analyzing a time series dataset representing temperature readings. You can have a conversation with ChatGPT to explore the patterns in the data. By asking questions like 'Are there any unusually high temperature readings?' or 'Have there been any sudden temperature drops?', you can gather insights and potentially detect outliers that deviate significantly from the expected temperature measurements.
I find the interactive and user-friendly aspect of ChatGPT very appealing. Are there any limitations to be aware of?
Absolutely, Michael! While ChatGPT is a powerful tool, it's important to be aware of its limitations. It may generate responses that sound plausible but are incorrect or biased. Careful analysis and critical thinking are still necessary when interpreting the results. Also, ChatGPT's performance can vary depending on the quality and relevance of the training data it has been exposed to.
Fred, what are the potential limitations of using ChatGPT in customer support?
ChatGPT's handling of time series data with irregular sampling rates is limited. While it can provide insights based on the available data, irregular spacing may impact its ability to capture fine-grained patterns accurately. It's generally recommended to preprocess the data and regularize the spacing before using it with ChatGPT for more reliable results.
Addressing bias in ChatGPT's responses is an ongoing area of research and improvement. OpenAI is actively working on reducing both glaring and subtle biases in how ChatGPT responds to various inputs. User feedback is invaluable in identifying and addressing issues related to bias in the system's outputs.
ChatGPT is primarily designed for static time series analysis rather than streaming data. It operates based on the available data at the time of the analysis. For streaming time series data, real-time analysis techniques and specialized tools may be more suitable.
Besides time series analysis, what other domains can benefit from the power of ChatGPT?
Great question, Daniel! ChatGPT can be beneficial in various domains such as natural language processing, customer support, educational assistance, and even creative writing. Its ability to generate coherent and contextually relevant responses makes it versatile for many applications where human-like interaction is desired.
Handling data outliers in time series analysis using ChatGPT is similar to traditional approaches. Outliers can be identified using statistical methods or visual inspection, and then appropriate actions can be taken, such as removing outliers or applying outlier detection algorithms. ChatGPT can assist in this process by helping to analyze and understand the impact of outliers on the overall time series patterns.
Users can take several steps to mitigate potential biases in ChatGPT's responses. Firstly, they can provide diverse and unbiased training data to the model. Secondly, they can carefully analyze and validate the outputs of ChatGPT through additional checks and evaluations. Finally, user feedback is crucial in identifying any biases and working towards improving the system's responses.
Are there any plans to develop a version of ChatGPT specifically tailored for streaming time series data analysis?
While I don't have insights into specific future plans, developing a version of ChatGPT tailored for streaming time series data analysis could be a promising direction for OpenAI. Streaming data analysis often requires real-time processing and online learning capabilities, which may differ significantly from static analysis. It's an exciting area to explore.
When using ChatGPT in customer support, one limitation is that it may generate responses that sound plausible but are incorrect or unrelated to the customer's query. Additionally, it may not have access to real-time customer-specific data, leading to less personalized responses. To mitigate these limitations, human review and oversight are advised to ensure the quality and accuracy of customer support interactions.
Users can provide feedback to OpenAI regarding potential biases or issues in ChatGPT by following the guidelines provided by OpenAI on their website or through specific channels they may have established for feedback. OpenAI highly values user feedback in order to improve the system and address any concerns related to bias or other issues.
How does the conversational nature of ChatGPT affect its performance in streaming time series data analysis, if adapted?
The conversational nature of ChatGPT could potentially provide benefits in streaming time series data analysis. It could allow users to have interactive discussions with the model about the ongoing streaming data, enabling real-time insights and rapid decision-making based on the current data. However, adapting ChatGPT for streaming analysis would require addressing the challenges of real-time processing and keeping up with the constantly evolving input stream.
ChatGPT can have various applications in educational assistance. It can help students by providing explanations, answering questions, and guiding them through problems in different subjects. It can simulate discussions and interactions with a tutor, offering personalized assistance and facilitating a better understanding of complex topics. ChatGPT's conversational nature makes it an engaging tool for interactive learning.
Can ChatGPT also detect contextual outliers in time series analysis?
ChatGPT can assist in detecting contextual outliers in time series analysis. By having a conversation with the model and asking questions about unusual patterns or unexpected behaviors, it can provide insights into potential contextual outliers. However, it's important to note that the ability to accurately detect contextual outliers will depend on the available data and the model's training.
Are there any plans to incorporate user feedback into future versions of ChatGPT?
Absolutely, Robert! User feedback plays a critical role in shaping and improving ChatGPT. OpenAI actively encourages user feedback and considers it for future iterations and enhancements of the system. Incorporating user feedback helps in identifying and addressing issues, expanding the capabilities, and ensuring that ChatGPT better aligns with user needs and expectations.