Revolutionizing Tolerance Analysis in Product Lifecycle Management with ChatGPT
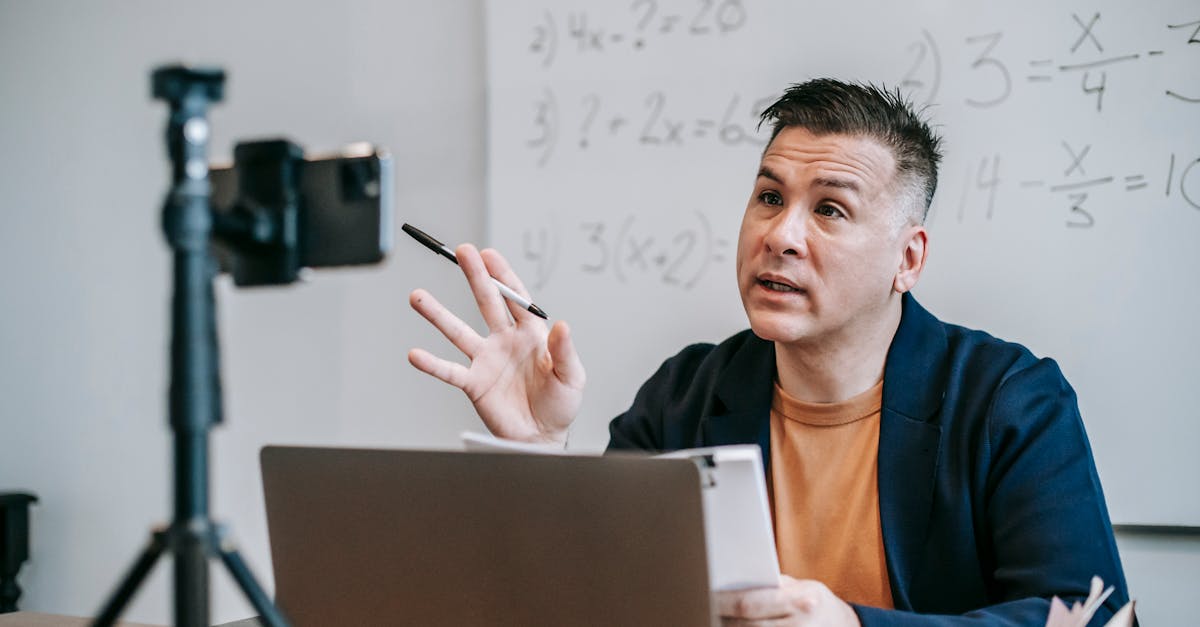
Tolerance analysis is an essential aspect of product lifecycle management (PLM). It involves evaluating and understanding how variations in dimensions and specifications can impact the overall performance and functionality of a product throughout its lifecycle. With the advancements in technology, tools like ChatGPT-4 have emerged, providing valuable insights into how tolerances may alter over a product's lifecycle, considering factors such as wear and tear.
What Is Tolerance Analysis?
Tolerance analysis is a systematic process that determines the acceptable range of variations in a product's design dimensions, ensuring that the product meets its intended function. It helps manufacturers identify potential issues early on, reducing the likelihood of design and production errors. By analyzing the tolerances in a product's design, engineers can optimize their designs, enhance product quality, and minimize the risk of performance failures.
Tolerance Analysis in Product Lifecycle Management
Product lifecycle management (PLM) is a comprehensive approach to managing a product's entire lifecycle, from conception to retirement. Tolerance analysis plays a critical role in PLM as it ensures that products perform as expected throughout their lifespan. By considering tolerances at each stage of the product's lifecycle, manufacturers can factor in wear and other environmental factors that may alter the tolerances over time.
ChatGPT-4, a state-of-the-art language model, can be utilized to gain insights into how tolerances may change over a product's lifecycle. With its natural language processing capabilities, ChatGPT-4 can analyze historical data, accumulated feedback, and real-world usage scenarios to provide valuable guidance on how tolerances may be affected by wear and other factors as a product ages.
The Benefits of Tolerance Analysis in PLM
Tolerance analysis offers various benefits in the field of product lifecycle management:
- Improved Design Optimization: By determining the critical dimensions and identifying potential variations, engineers can optimize the design to meet performance requirements and minimize manufacturing costs.
- Enhanced Quality Control: Tolerance analysis helps ensure that products consistently meet the desired specifications, reducing the risk of recalls or customer dissatisfaction due to performance issues.
- Cost Reduction: By minimizing dimensional variations, manufacturers can reduce waste, rework, and production costs, resulting in improved profitability.
- Accurate Predictive Maintenance: With insights on how tolerances may alter over a product's lifecycle, maintenance activities can be planned and executed more effectively, minimizing downtime and optimizing maintenance schedules.
Conclusion
Tolerance analysis is a critical aspect of product lifecycle management. By considering variations in dimensions and specifications throughout a product's lifespan, manufacturers can optimize designs, enhance product quality, and reduce costs. The utilization of advanced language models like ChatGPT-4 further amplifies the power of tolerance analysis by providing valuable insights into how tolerances may change over a product's lifecycle, considering wear and other environmental factors. Incorporating tolerance analysis into the product development process can lead to better-performing products, improved customer satisfaction, and increased profitability.
Comments:
This article on revolutionizing tolerance analysis in product lifecycle management with ChatGPT is fascinating! It's amazing how advances in AI can greatly improve efficiency and accuracy in various industries.
I agree, Alice. AI has the potential to revolutionize multiple aspects of product lifecycle management. ChatGPT seems like a promising tool for enhancing tolerance analysis. Can't wait to see how it develops further!
As an engineer, I'm excited to explore the possibilities of using ChatGPT for tolerance analysis. It has the potential to streamline the design process and minimize errors. Looking forward to its implementation.
Charlie, as an engineer, have you used any other AI tools for tolerance analysis? How does ChatGPT compare?
Frank, I've used a few AI-based tools before, but ChatGPT seems more intuitive and flexible. It allows for more interactive analysis and dynamic adjustments. However, further testing and validation are necessary to truly assess its capabilities.
Frank, ChatGPT's ability to understand and respond to natural language makes it more user-friendly compared to some other AI tools. It enhances the ease of communication between engineers and non-technical stakeholders.
Charlie, I appreciate your insights. If ChatGPT proves to be versatile and user-friendly, it could indeed revolutionize the way we approach tolerance analysis. Looking forward to further developments and validations.
Charlie, that's great to hear. Clear communication and understanding are fundamental to successful engineering projects. ChatGPT's natural language processing capabilities could bridge the gap between technical and non-technical teams.
Charlie, let's hope that ChatGPT's user-friendly nature makes it more accessible to a broader range of teams and stakeholders, facilitating better collaboration and understanding throughout the product lifecycle.
Frank, absolutely! Improved communication and understanding across teams can lead to better collaboration, fewer errors, and ultimately, more successful engineering projects.
While AI is undoubtedly powerful, I hope there are robust quality control measures in place when implementing ChatGPT in product lifecycle management. Accurate tolerance analysis is crucial, and any AI tool should undergo rigorous testing.
Thank you all for your comments! It's great to see your enthusiasm about ChatGPT in tolerance analysis. David, you raise an important point. Quality control is indeed vital, and thorough testing and validation should be conducted before widespread adoption.
Erik Girsen, as the author, how do you envision the integration of ChatGPT with existing product lifecycle management systems?
Bob, the integration would involve developing APIs or plugins that allow seamless communication between ChatGPT and existing systems. This would ensure compatibility and ease of adoption for companies already using product lifecycle management solutions.
Thank you for the clarification, Erik. It's crucial to have smooth integration for widespread adoption. Exciting times ahead!
Erik, can you share any successful case studies where ChatGPT has already been implemented for tolerance analysis?
Frank, there are ongoing pilot projects where ChatGPT is being tested for tolerance analysis. While it's still early to share extensive case studies, initial results are promising. More data and real-world deployments will provide a clearer picture of its success.
Erik Girsen, as the author, do you think ChatGPT's tolerance analysis can outperform traditional methods in terms of accuracy?
Bob, ChatGPT's tolerance analysis shows promise in terms of accuracy, thanks to its ability to process large amounts of data and identify patterns. However, it shouldn't be viewed as a complete replacement for traditional methods yet. Collaborative use with human experts can yield optimal results.
Erik Girsen, as the author, what are the potential challenges in implementing ChatGPT for tolerance analysis?
Bob, some challenges include addressing biases in training data, minimizing false positives/negatives, and ensuring the tool is user-friendly. Additionally, managing the volume and complexity of data can be a significant hurdle. These challenges need careful consideration for successful implementation.
Erik Girsen, it's good to consider ChatGPT as a tool to enhance accuracy rather than replace traditional methods completely. Human expertise combined with AI's capabilities can lead to optimal tolerance analysis.
Indeed, Bob. The collaborative use of AI and human expertise can create a synergy that enhances accuracy and efficiency in tolerance analysis. It's about leveraging the strengths of both.
Erik Girsen, thank you for highlighting the potential challenges. Overcoming these hurdles will be crucial for the successful implementation of ChatGPT in tolerance analysis. Exciting times lie ahead!
The challenges certainly require careful consideration, Bob. By addressing them proactively, we can ensure a more seamless integration of ChatGPT into tolerance analysis practices, opening up new possibilities and efficiencies.
David, I agree that quality control is crucial. The accuracy of tolerance analysis directly impacts product performance and reliability. Ongoing monitoring and periodic assessments would be essential.
Alice, you summed it up well. Continuous monitoring, validation, and audits can help maintain the robustness of ChatGPT's tolerance analysis. Human oversight should also be incorporated to catch any potential AI biases or errors.
David, incorporating feedback loops and continuous improvement processes can help refine ChatGPT's accuracy over time. Regular updates and learning from real-world scenarios can drive improvements in tolerance analysis.
Alice, you're right. Continuous improvement is a crucial aspect of implementing AI tools like ChatGPT. Regular assessments and necessary adaptations ensure that the tool keeps up with evolving requirements.
David, feedback loops and continuous improvement are essential not only for enhancing accuracy but also for adapting ChatGPT to evolving industry standards and requirements. The flexibility to incorporate new knowledge and insights is vital.
David, adopting an agile approach to AI implementation can help continuously refine ChatGPT's accuracy and optimize the tool for evolving industry needs. Ongoing improvement efforts are essential.
Alice, transparency and explainability should go hand in hand. Users should have a clear understanding of how ChatGPT works and the reasoning behind its outputs. This ensures trust and helps identify and rectify any potential biases or errors.
Grace, fully agree. Explainability and interpretability are crucial in building trust and acceptance of AI tools like ChatGPT. It also enables users to identify any shortcomings and potential improvements.
That's a valid concern, Alice. Transparency regarding data usage and user consent should be prioritized. Ethical considerations must always accompany AI implementation to ensure responsible and accountable use.
As a project manager, I'm excited about the potential of ChatGPT in improving communication between engineers and other stakeholders. Clearer understanding and collaboration can significantly enhance product development processes.
Eva, I completely agree with you. Improved communication and collaboration are vital for successful product lifecycle management. If ChatGPT can enhance these aspects, it will have a significant positive impact.
This article highlights the potential benefits of integrating AI into the product lifecycle management process. However, we should also be cautious about potential ethical and privacy concerns that arise when using AI technology extensively.
This article highlights the potential of AI in product lifecycle management. However, it's essential to emphasize the importance of human expertise and judgement, especially in critical decision-making processes.
Helen, you make an essential point. AI tools like ChatGPT should complement human expertise rather than replacing it. Human judgement is invaluable in ensuring quality standards and making critical decisions.
Helen, I couldn't agree more. AI tools should always be seen as augmenting human skills rather than replacing them entirely. The combination brings about the best results.