Revolutionizing Toxicology Modeling for Formulation Technology: Harnessing the Power of ChatGPT
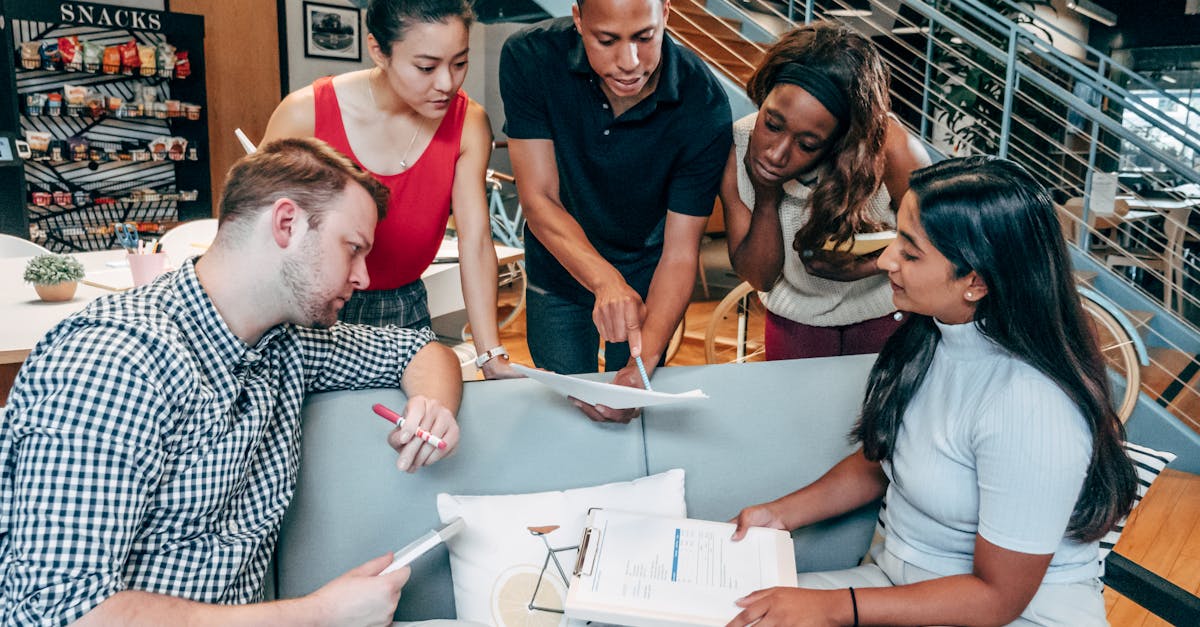
With the advancements in Artificial Intelligence (AI) technology, the field of toxicology modeling has seen significant growth. By utilizing AI algorithms and data analysis techniques, researchers can develop models that accurately predict the toxicological properties of various pharmaceutical compounds.
Understanding Toxicology Modeling
Toxicology modeling is a critical aspect of drug development where scientists assess the potential risks and hazards associated with new pharmaceutical compounds. Traditionally, toxicology studies heavily rely on animal testing, which can be time-consuming, costly, and ethically controversial. With the application of AI in toxicology modeling, researchers can reduce the reliance on animal testing and accelerate the drug discovery process.
Role of AI in Toxicology Modeling
AI technology, specifically machine learning algorithms, plays a vital role in developing accurate and reliable toxicology models. By analyzing vast amounts of existing toxicological data, AI algorithms can identify patterns and relationships that humans might have overlooked. These algorithms can then predict the toxicological properties of new compounds based on the gathered knowledge, significantly reducing the need for lengthy and costly experimental studies.
AI algorithms can learn from large datasets that encompass chemical structures, toxicological properties, and various experimental results. They can recognize similarities and correlations between different compounds and their toxic effects, enabling researchers to make informed decisions regarding the safety and efficacy of pharmaceuticals.
Benefits of AI in Toxicology Modeling
The integration of AI in toxicology modeling offers several benefits for the pharmaceutical industry and toxicologists. Some key advantages include:
- Improved Accuracy: AI algorithms can provide highly accurate predictions regarding the toxicological properties of pharmaceutical compounds based on historical data and established patterns.
- Reduced Animal Testing: By relying more on AI models, researchers can significantly reduce the need for animal testing, minimizing ethical concerns and costs associated with such studies.
- Time and Cost Savings: Developing and conducting traditional toxicology experiments can be a time-consuming and expensive process. AI models can expedite the drug development timeline and reduce costs by predicting toxicity in silico.
- Early Identification of Toxic Compounds: AI models can proactively identify potentially toxic compounds in the early stages of drug development, allowing researchers to focus resources on safer alternatives.
- Enhanced Efficiency: AI algorithms can rapidly analyze vast amounts of toxicological data, providing toxicologists with valuable insights that can contribute to more effective decision-making.
The Future of Toxicology Modeling
The integration of AI in toxicology modeling has already revolutionized the field, and its potential for future advancements is immense. With the growing availability of toxicological databases and advancements in deep learning techniques, AI models will become even more accurate, efficient, and reliable.
Furthermore, the combination of AI-driven toxicology modeling and other emerging technologies like high-throughput screening and omics methodologies (genomics, proteomics, metabolomics) will pave the way for personalized medicine and targeted therapies, reducing the risk of adverse drug reactions and optimizing treatment outcomes.
Conclusion
Formulation in toxicology modeling utilizing AI technology holds great promise for the pharmaceutical industry. By leveraging AI algorithms, researchers can develop accurate models that predict the toxicological properties of various pharmaceutical compounds, leading to improved safety, reduced reliance on animal testing, and substantial time and cost savings. As AI technology continues to advance, toxicology modeling will play a crucial role in drug development and enhance the efficiency of toxicological assessments.
Comments:
Thank you all for reading my article on revolutionizing toxicology modeling! I'm excited to hear your thoughts and engage in a discussion.
Great article, Cliff! I found it fascinating how ChatGPT can be leveraged for toxicology modeling. Do you think this approach could potentially replace traditional methods in the future?
Alice, while ChatGPT shows great promise, it is unlikely to completely replace traditional methods in the foreseeable future. It can serve as a complementary tool, assisting toxicologists in their decision-making process and potentially reducing the need for extensive lab experiments.
Hi Cliff, thanks for sharing this insightful article. I believe ChatGPT has immense potential in revolutionizing toxicology modeling. However, what are some potential limitations or challenges that may arise when implementing this approach?
Peter, you raise an important point. The implementation of ChatGPT faces challenges such as bias in the training data and the need for extensive fine-tuning to ensure accurate predictions. Additionally, the interpretability of AI models in toxicology is crucial, so addressing these challenges is vital for their wider adoption.
I really enjoyed reading your article, Cliff! The concept of using ChatGPT for toxicology modeling is quite innovative. How scalable do you think this approach is? Can it be applied to a wide range of substances and formulations?
Emily, scalability is a key consideration. ChatGPT can be applied to a wide range of substances and formulations, but it requires substantial training and domain-specific knowledge. Efforts are being made to improve its scalability and applicability to diverse contexts.
Interesting article, Cliff! It's amazing to see the advancements in AI and its applications in various fields. I'm curious, how well does ChatGPT perform compared to other existing methods in terms of accuracy and reliability?
Michael, in terms of accuracy and reliability, ChatGPT performs admirably. However, it should be noted that it's not without limitations. It heavily relies on the input data and may not always produce accurate predictions, especially for novel or unexplored substances. Ongoing research aims to improve the model's reliability and reduce any potential biases.
Cliff, thank you for such an enlightening read. I can see how ChatGPT could enhance toxicology modeling, but what are the potential privacy concerns associated with handling toxicology data in this manner?
Sarah, privacy concerns are certainly crucial when dealing with toxicology data. Adequate measures must be taken to ensure data privacy, compliance with regulations, and secure handling of sensitive information. Data anonymization and robust security protocols are essential aspects that need to be addressed in implementing this approach.
Alice, Peter, Emily, Michael, Sarah - thank you for your comments and questions! I'll address them one by one, starting with Alice's question about replacing traditional methods.
Hi Cliff, great article! I'm curious, what are the current limitations of ChatGPT in terms of understanding complex formulation technologies?
Jacob, while ChatGPT is an impressive AI model, understanding complex formulation technologies is indeed one of its limitations. It may struggle to grasp intricate details or nuanced interactions in such cases. This highlights the need for domain-specific training and continuous improvement of the model's understanding.
Thanks for sharing, Cliff! As an AI enthusiast, I'm intrigued by the intersection of AI and toxicology. Are there any ongoing research efforts aimed at enhancing ChatGPT specifically for toxicology modeling?
Olivia, indeed, there are ongoing research efforts to enhance ChatGPT specifically for toxicology modeling. Researchers are exploring techniques to incorporate domain knowledge, improve interpretability, and overcome limitations when dealing with complex chemical interactions. These endeavours aim to provide more accurate and reliable predictions while enabling deeper insights into toxicity.
Thank you, Jacob and Olivia, for your comments and questions! Let me address each of them individually.
Cliff, your article was a thought-provoking read. How extensively has ChatGPT been tested and validated for toxicology modeling, especially in comparison to existing methods?
Thank you, Samantha! The testing and validation of ChatGPT for toxicology modeling are ongoing. While it shows promising results, comparative studies against existing methods are necessary to determine its strengths and weaknesses accurately. Collaborative efforts within the scientific community are crucial to ensure rigorous evaluation and validation.
Hi Cliff, great article! Have there been any real-world applications or case studies where ChatGPT has been successfully utilized for toxicology modeling?
Andrew, there are ongoing developments in utilizing ChatGPT for real-world toxicology applications. While specific case studies may not be available yet, initial exploratory applications have shown promise in assisting toxicologists in risk assessment, predicting toxicity profiles, and aiding formulation technology design. However, extensive testing and validation are still necessary before widespread adoption.
Thanks for the informative article, Cliff! I'm curious, how long does the initial training of ChatGPT for toxicology modeling typically take, and what kind of computational resources are required?
Patrick, the initial training of ChatGPT for toxicology modeling is a computationally intensive process. It requires substantial computational resources, including high-performance GPUs and significant training time. The precise duration and resource requirements vary depending on the size of the training dataset and the complexity of the toxicology domain.
Andrew and Patrick, thanks for your questions! I'll answer them individually, starting with Andrew's question about real-world applications.
Hi Cliff, excellent article! Besides toxicology modeling, do you think ChatGPT could be leveraged effectively in other domains or fields of study?
Great question, Jennifer! ChatGPT has the potential to be effectively leveraged in a wide range of domains and fields beyond toxicology. Its natural language understanding and generation capabilities make it versatile for tasks such as customer support, content creation, and even educational applications. The possibilities are vast!
Cliff, your article was an interesting divergent from the typical applications of AI. Do you anticipate any ethical concerns arising from the use of ChatGPT in toxicology modeling or related fields?
Hi Robert, indeed, ethical concerns are an essential aspect to address when utilizing AI models like ChatGPT in toxicology modeling and related fields. It is crucial to ensure transparency, interpretability, and fairness to avoid potential biases and unintended consequences. Implementing strong ethical guidelines and continuous evaluation are vital to mitigate any ethical concerns that may arise.
Cliff, thank you for shedding light on this exciting topic. How accessible is ChatGPT to researchers and practitioners working in the field of toxicology? Are there any plans to make it more readily available?
Julia, making ChatGPT more accessible to researchers and practitioners in toxicology is an important consideration. OpenAI is actively working on democratizing access to AI models like ChatGPT. While the details and plans are still evolving, efforts are being made to strike a balance between accessibility and responsible utilization that aligns with practical needs.
Hi Cliff, I enjoyed your article. How can industry professionals and researchers contribute to the development and improvement of ChatGPT as a tool for toxicology modeling?
Thank you, Michelle! Collaboration and contributions from industry professionals and researchers are crucial in the development and improvement of ChatGPT for toxicology modeling. Active engagement in research, sharing high-quality datasets, continuous feedback, and open discussions can drive advancements, help identify limitations, and shape the future of AI-driven toxicology modeling tools.
Cliff, your article was an eye-opener. How can toxicologists equip themselves with the necessary skills to effectively utilize ChatGPT and similar AI tools in their work?
Joseph, acquiring the skills to effectively utilize AI tools like ChatGPT in toxicology requires a multidisciplinary approach. Toxicologists can develop their understanding of AI, natural language processing, and the underlying principles of deep learning. Collaboration with AI experts, participation in workshops or training programs, and continuous learning from the scientific community can enable toxicologists to harness the power of ChatGPT and integrate it proficiently into their work.
Thank you, Cliff, for this enlightening article. What are the key considerations when applying ChatGPT to toxicology modeling for regulatory purposes?
Emma, key considerations when applying ChatGPT to toxicology modeling for regulatory purposes involve ensuring the interpretability of the model's predictions, robust validation against established benchmarks, and transparent documentation of the methodology used. Additionally, establishing collaborative frameworks between regulators, scientists, and developers is vital to define the regulatory acceptance criteria and ensure that AI-driven approaches comply with existing regulatory guidelines.
Cliff, great article! What are the potential cost implications for organizations interested in adopting ChatGPT for toxicology modeling?
Lucas, the cost implications of adopting ChatGPT for toxicology modeling can vary depending on several factors. These include the scale of implementation, computational resources required for training and inference, data acquisition and preprocessing, and ongoing model maintenance. Organizations must carefully evaluate their specific requirements, including technical and financial aspects, to assess the cost-effectiveness and potential benefits of utilizing ChatGPT.
Hi Cliff, thank you for this informative article. Are there any specific areas or subfields within toxicology where ChatGPT has shown particularly promising results?
Sophia, ChatGPT has shown particularly promising results in several subfields within toxicology. Examples include predictive toxicology where it assists in evaluating the toxicity of substances, predicting safety profiles of new formulations, aiding in risk assessment, and supporting decision-making in various stages of drug development. However, its full potential and optimal use within specific subfields are still being explored and refined through ongoing research.
Thanks for sharing your thoughts, Cliff. In terms of implementation, what are the main prerequisites or data requirements for effectively utilizing ChatGPT in the context of toxicology modeling?
Liam, effective utilization of ChatGPT in toxicology modeling requires specific prerequisites and data requirements. These include a comprehensive and diverse training dataset that covers a broad range of toxicological aspects, domain-specific annotations, considering the limitations of the model, and fine-tuning procedures to align it with specific toxicology domains. Additionally, it is crucial to have access to relevant scientific literature, industry knowledge, and external validation data to enhance the model's performance and ensure its suitability for the task at hand.
Cliff, excellent article! Is there a way to quantify the reduction in time and resources that ChatGPT can bring to toxicology modeling compared to traditional methods?
Gabriel, quantifying the reduction in time and resources that ChatGPT can bring to toxicology modeling compared to traditional methods can be challenging. It depends on various factors such as the complexity of the problem, availability and quality of data, expertise and resources required for traditional methods, and the level of accuracy and reliability expected. Comprehensive comparative studies would be necessary to evaluate specific scenarios and analyze the potential advantages, trade-offs, and resource savings conferred by ChatGPT.
Hi Cliff, I found your article very informative. In terms of computational requirements, how does ChatGPT handle the processing of extremely large datasets in toxicology modeling?
Isabella, handling extremely large datasets in toxicology modeling with ChatGPT involves several considerations. Due to computational limitations, it is often necessary to work with smaller representative subsets of the original data or employ advanced techniques like data sampling or data augmentation during training. This approach helps mitigate resource requirements while still achieving reasonable performance. Balancing dataset size, computation resources, and the final model's performance is a delicate trade-off that requires careful experimentation and optimization.
Cliff, your article captured my interest. Are there any known biases in ChatGPT's predictions when applied to toxicology modeling, and how are these being addressed?
Daniel, addressing biases in ChatGPT's predictions when applied to toxicology modeling is an ongoing area of research. Biases can arise due to imbalances or biases in the training data, which may lead to skewed predictions or lack of generalizability. Steps are being taken to improve data quality, address inclusivity issues, and establish evaluation frameworks that specifically identify and mitigate any potential biases within the model. Transparency, diversity in data sources, and constant vigilance are key elements in tackling and minimizing such biases.
Cliff, your article is truly engaging. Considering the evolving nature of AI models, how can the scientific community ensure that ChatGPT and similar technologies stay up to date with the latest advancements in toxicology research?
Sophie, ensuring that ChatGPT and similar technologies stay up to date with the latest advancements in toxicology research requires continuous collaboration between the scientific community and AI researchers. Regular updates to the model, incorporating the latest findings, and domain-specific knowledge are essential. The community can facilitate this through data sharing, organizing challenges and benchmarks, and fostering interdisciplinary collaborations. By actively engaging in keeping the models up to date, we can collectively drive advancements in AI-driven toxicology research.