Revolutionizing Vehicle Autopilot: Harnessing the Power of ChatGPT and Neural Networks
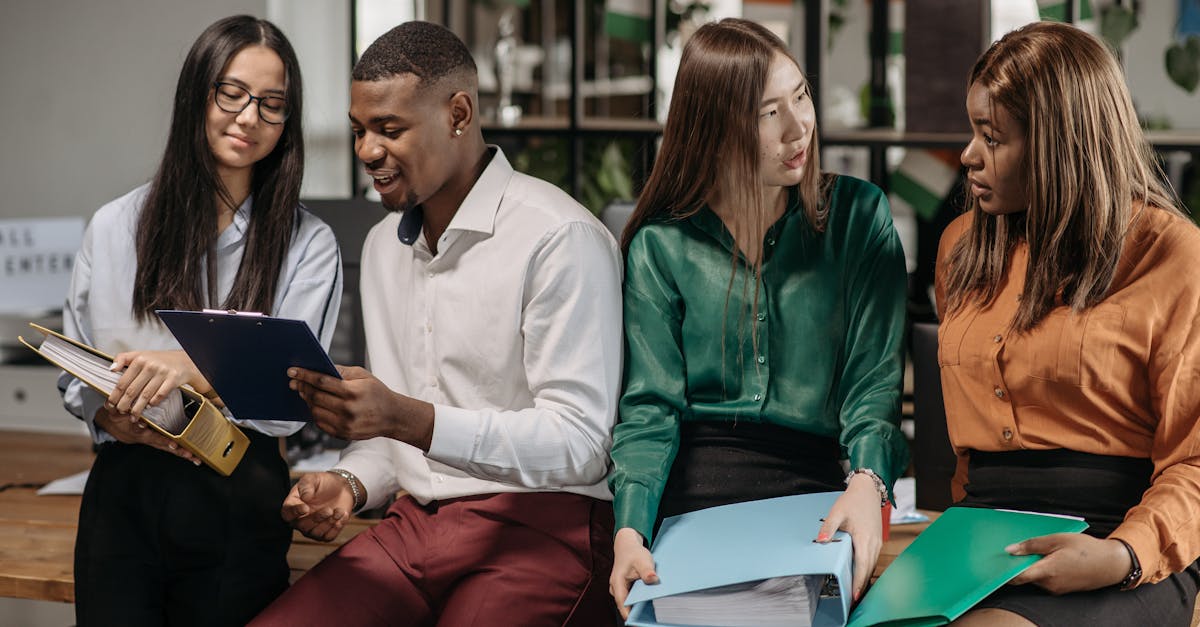
In recent years, self-driving cars have become increasingly prominent in the automotive industry. These vehicles rely on advanced technologies to navigate roads, recognize traffic signs, and make informed decisions in real-time. One such technology that has revolutionized the development of autonomous vehicles is neural networks.
What are Neural Networks?
Neural networks are a branch of artificial intelligence that aim to mimic the functioning of the human brain. They consist of interconnected nodes, or "neurons," that process and transmit information between each other. These networks are designed to learn and adapt from patterns and data, enabling them to perform complex tasks effectively.
Application in Vehicle Autopilot
The use of neural networks in vehicle autopilot systems has greatly enhanced their capabilities. These systems utilize neural networks to process vast amounts of real-time data from various sensors, such as cameras, lidar, and radar. By analyzing these inputs, neural networks enable self-driving cars to make informed decisions based on the current road conditions.
One of the critical aspects of autonomous driving is the ability to understand and interpret the environment accurately. Neural networks excel in this area by recognizing and classifying objects, such as pedestrians, other vehicles, and road signs. This allows the vehicle to respond accordingly, adjusting its speed, trajectory, and behavior to ensure safe navigation.
Improving Decision Making
The strength of neural networks lies in their ability to learn from prior information and adapt to new situations. In the context of vehicle autopilot, this means that as the network is exposed to more driving scenarios, it becomes better at making decisions. Neural networks can analyze patterns and correlations between different variables, such as weather conditions, traffic flow, and road layouts, to determine the most appropriate actions to take.
For example, when approaching an intersection, the neural network can consider factors such as the state of traffic lights, the presence of pedestrians, and the speed and position of other vehicles. Based on this information, it can make decisions such as when to yield, when to accelerate, or when to stop. Over time, the network's accuracy and efficiency in making these decisions improve, resulting in safer and more reliable autonomous driving.
Real-Time Inputs and Adaptability
The real-time nature of neural networks makes them particularly valuable in vehicle autopilot systems. These networks can process sensor data with minimal delay, allowing for quick and accurate decision making. This capability is crucial in situations where split-second decisions are required, such as avoiding obstacles or responding to sudden changes in traffic patterns.
Furthermore, the adaptability of neural networks ensures that they remain effective even in dynamic environments. Road conditions, traffic patterns, and other variables can change rapidly, and the network must be able to adjust its decision-making processes accordingly. Neural networks can continue learning and evolving, incorporating new information and adapting to evolving circumstances, ensuring optimal performance in all driving situations.
Conclusion
Neural networks have emerged as a powerful tool in the development of self-driving car technologies. Their ability to process real-time inputs, learn from prior information, and make informed decisions has significantly contributed to the advancement of vehicle autopilot systems. As these networks continue to evolve and improve, we can expect even safer and more capable self-driving cars on our roads in the future.
Comments:
Thank you all for reading my article on revolutionizing vehicle autopilot! I'm excited to hear your thoughts and engage in a discussion.
Great article, Breaux! The potential of using ChatGPT and neural networks to enhance vehicle autopilot systems is truly fascinating. I'm curious to know what specific improvements these technologies can bring.
Thanks, Peter! Using ChatGPT and neural networks can improve various aspects of vehicle autopilot. For example, they can enhance object recognition, improve decision-making algorithms, and enable more advanced driver-assistance systems. These advancements aim to achieve safer and more autonomous driving experiences.
Thanks for clarifying, Breaux! It's exciting to think about the possibilities. Better object recognition and decision-making would indeed make autonomous vehicles more reliable and safe, potentially reducing accidents caused by human error.
You're welcome, Peter! Indeed, reducing accidents caused by human error is one of the key motivations for advancing vehicle autopilot systems. By leveraging AI technologies like ChatGPT and neural networks, we can aim for reliable and efficient autonomous driving experiences.
Thanks, Breaux! It's an exciting prospect to see how ChatGPT and neural networks can enhance vehicle autopilot systems. I can't wait to witness the future of autonomous driving.
I agree, Peter! The potential for ChatGPT and neural networks in revolutionizing vehicle autopilot is really exciting. I can imagine a future where vehicles can communicate with each other to collectively optimize traffic flow and reduce accidents. It could lead to a whole new era of transportation.
Hi Breaux, thank you for sharing your insights! As an AI enthusiast, I believe integrating ChatGPT and neural networks into vehicle autopilot has the potential to greatly enhance safety and efficiency. However, I wonder if there are any concerns about relying too heavily on AI systems in critical driving situations.
Hi Laura, that's a great point! Safety is a critical aspect when it comes to autonomous driving. While AI-powered systems can improve safety, it's necessary to have robust fail-safe mechanisms and constant oversight to prevent any potential issues. AI should be seen as a supportive tool rather than a complete replacement for human judgement.
Absolutely, Breaux! A strong human oversight and backup systems are crucial to mitigate risks associated with AI-controlled driving. It's also important to consider ethical considerations, such as data privacy and the potential for algorithmic bias.
Definitely, Laura! Ethical considerations and responsible implementation of AI in autonomous vehicles are critical. As for the communication between vehicles, it can greatly enhance traffic efficiency, allowing for reduced congestion and improved overall transportation systems.
I'm on the same page, Emma! Traffic optimization and reduced congestion would greatly benefit our daily commuting experiences. It'd be fantastic to have a future where travel is both efficient and environmentally friendly!
Absolutely, Sara! Environmentally friendly transportation is becoming increasingly important. AI-based autonomous vehicles can contribute to reducing emissions and optimizing energy usage, making them a vital part of sustainable transportation.
Hi Emma, I completely agree! Traffic optimization and reduced congestion would not only save time but also have a positive impact on the environment. It's exciting to think about the positive changes AI can bring in this domain.
Absolutely, Oliver! AI-driven traffic optimization can lead to more efficient use of existing infrastructure, reduce fuel consumption, and minimize the environmental impact of transportation. It's a win-win situation for both commuters and the planet.
I totally agree with you, Sara! The potential benefits of AI-based traffic optimization go far beyond just saving time. It can contribute to reducing carbon emissions and creating more sustainable urban environments.
Indeed, Oliver! The positive impact on the environment and quality of life in urban areas cannot be overlooked. AI presents opportunities to optimize traffic flow, reduce congestion, and make cities more livable.
Definitely, Oliver! AI technologies can play a significant role in shaping a more sustainable future, and traffic optimization is just one of the aspects where these technologies can create positive change.
Hello Breaux, I enjoyed reading your article. These emerging technologies indeed have the potential to transform the way we travel. However, I'm curious about the timeline for the widespread implementation of such advanced autopilot systems. What are your thoughts?
Hello John! I appreciate your feedback. The implementation timeline depends on various factors such as technological advancements, regulatory frameworks, and public acceptance. While there are ongoing trials and limited deployments, a widespread implementation of advanced autopilot systems could still be several years away.
Thank you for your response, Breaux. Indeed, it's a complicated process, and public acceptance is crucial for widespread implementation. I hope to see the advancements in autopilot systems become a reality in the near future.
You're welcome, Breaux! I share your optimism for the future of autonomous driving. The potential benefits are immense, and it's exciting to witness the progress in this field.
I'm a bit skeptical, Breaux. While the advancements in autopilot systems are impressive, I worry about the potential for hacking or system malfunctions that could put lives at risk. How can we ensure the security of these AI-driven autopilot systems?
Valid concern, Daniel. Ensuring the security of AI-driven autopilot systems is crucial. Implementing robust security measures, such as encryption, authentication protocols, and continuous monitoring, can help mitigate the risks of hacking and system malfunctions. Additionally, regular vulnerability assessments and software updates are crucial for maintaining system integrity.
Thank you for addressing my concern, Breaux! Robust security measures, continuous monitoring, and prompt software updates indeed sound like effective ways to mitigate risks. It's comforting to know that these aspects are being considered.
Thank you for addressing my concern, Breaux! It's reassuring to know that these security aspects are being taken seriously. AI-driven autopilot systems have incredible potential, and it's exciting to see the continuous advancements.
Very true, Breaux! Data privacy is of utmost importance, especially when it comes to autonomous driving systems. Ensuring that personal information and vehicle data are securely handled is essential for building trust in these technologies.
Absolutely, Breaux! Trust plays a key role in the public acceptance of autonomous vehicles. Handling data securely and transparently will contribute to building trust in these AI-driven systems.
While security is definitely important, I wonder about the level of transparency in how these AI algorithms make decisions on the road. Should there be regulations or requirements to provide explanations for the decisions made by autonomous vehicles?
That's an interesting point, Megan! Explainability of AI algorithms is indeed a topic of discussion. Transparency can help build trust and understanding among users and regulators. Some regulatory frameworks are already emphasizing the need for explainable AI, especially in safety-critical domains like autonomous driving. It's an area that requires further exploration and research.
Great article, Breaux! I believe integrating ChatGPT and neural networks can also improve the accessibility of autonomous vehicles for people with disabilities. By enhancing adaptability and personalization, AI can help create inclusive transportation systems.
Thank you, Sarah! You're absolutely right. AI technologies can play a significant role in making transportation more inclusive and accessible for people with disabilities. Personalization and adaptability are key aspects that can have a positive impact in this regard.
I hadn't thought about the benefits for people with disabilities, Sarah. That's an important point! AI has the potential to make transportation more inclusive and help individuals with different abilities to experience greater freedom and independence.
Thanks for addressing my concern, Breaux! The topic of explainability is definitely complex, but as AI-powered systems become more prevalent, it's essential to strike a balance between transparency and preserving proprietary technology.
Absolutely, Megan! It's a delicate balance, and finding the right level of explainability while protecting sensitive aspects of the underlying technology is a challenge. Collaborative efforts involving researchers, industry experts, and regulators are essential to address this challenge.
I completely agree with you, Breaux! Finding a collaborative approach involving various stakeholders is crucial for addressing the challenges associated with AI explainability.
Finding the right balance between proprietary technology and explainability is certainly challenging, Breaux. I completely agree that collaborative efforts will be key in shaping regulations and standards for AI-driven autonomous vehicles.
Absolutely, Megan! The collaboration between different stakeholders is crucial for shaping the future of AI-driven autonomous vehicles. By involving various perspectives and expertise, we can work towards effective regulations and standards that address the complexities of this field.
I think finding the right balance between explainability and preserving proprietary technology is indeed crucial, Megan. It's a complex challenge, but one that needs to be addressed as AI continues to advance.
Thank you, Emily! It's indeed a complex challenge, but necessary for the responsible development and deployment of AI-driven technologies. Collaboration and continuous research can help strike the right balance and drive progress.
I'm glad you brought up transparency, Megan. Trust is crucial, and having a clear understanding of how AI algorithms make decisions on the road would definitely help in that regard.
Indeed, Samantha! Transparency goes hand in hand with trust. Explaining how AI algorithms make decisions in real-life scenarios can empower users to trust and adopt these systems more readily.
Well said, Breaux! Building a shared understanding and framework through collaboration can result in more effective regulations and policies regarding AI explainability.
I agree, Breaux and Samantha! Transparency fosters trust, and having a better understanding of AI decision-making can help address concerns and encourage wider acceptance of autonomous vehicles and their underlying technologies.
I'm glad we're discussing the importance of transparency and understandability in the decision-making of autonomous vehicles. It's not only about trust but also ensuring accountability and having a clear chain of responsibility.
You're absolutely right, Sarah! Transparency and understandability are essential not only for trust-building but also for establishing clear lines of responsibility and accountability. These aspects should be considered while designing and implementing AI-driven autonomous systems.
Well said, Breaux! Responsible development of AI-driven autonomous systems requires thoughtful consideration of not just technical aspects but also ethical, legal, and social implications.
I'm glad this discussion is happening! Transparency and accountability are crucial when deploying AI-driven technologies in real-world scenarios. It's important to ensure that AI decisions can be understood, audited, and, if needed, corrected.
Absolutely, David! Transparency and accountability are key principles in safely integrating AI-driven technologies. Being able to understand and audit AI decisions helps ensure that these technologies align with our societal values and function as intended.
I couldn't agree more, Breaux! Responsible development ensures that AI technologies serve as enablers and augmenters, ultimately benefiting society while mitigating potential risks. This discussion has been insightful!