Revolutionizing Video Compression: The Power of ChatGPT
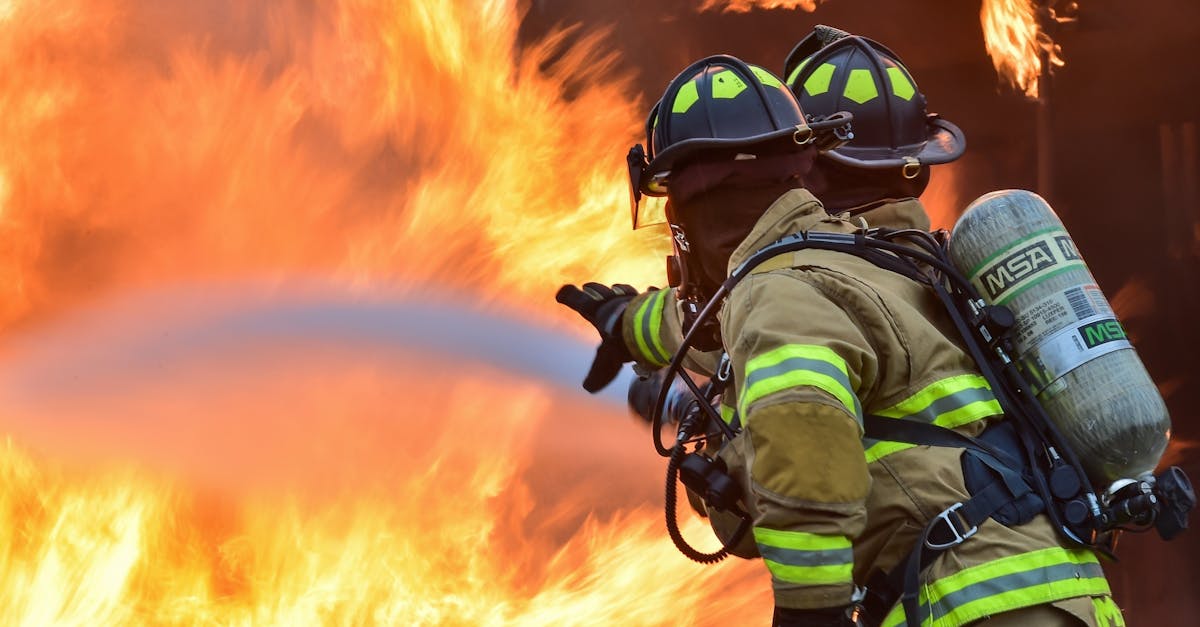
Video compression is a staple technology in today's digital world. Much of the media we consume, whether it be on YouTube, Netflix, or other streaming platforms, would not be possible without this technology. Video compression works by reducing and removing unnecessary file content, thereby making the file smaller without a significant loss in the quality of the video. While this technology has been improved significantly over the years, quality analysis remains a critical feature that should be continually enhanced and refined.
Quality analysis involves examining the video compression to decipher whether it maintains the same quality as the original or not. This is an essential aspect because it ensures that the end user gets an almost exact quality like the original video, despite the reduction in file size. Quality analysis could involve checking for pixelization, loss of detail, blurred images, or any other issue that could degrade the video content.
In an era where algorithms are getting smarter, many organizations have started utilizing AI technologies to automate video compression quality analysis. One of these AI models is OpenAI's ChatGPT-4. This AI model could be used to analyze video compression quality by parsing feedback and complaints from various users.
ChatGPT-4 and Video Compression Quality Analysis: A New Approach
ChatGPT-4 model provides a unique and novel approach to video compression quality analysis. Unlike traditional methods that rely on manual checks and balances, ChatGPT-4 uses an algorithm to parse user feedback and comments about the video. This provides a faster and more precise quality evaluation.
The foundation of this method starts with collecting user feedback or comments about a video's quality. These comments or complaints can be obtained from various sources such as customer emails, chat transcripts, social media comments, and other user-generated content. ChatGPT-4 is then utilized to analyze this data.
When parsing through the feedback, ChatGPT-4 searches for keywords and phrases associated with video quality complaints. These could range from phrases like "The video is pixelated" or "the resolution is blurred." By picking up these complaints, the AI can identify patterns and common complaints, thus providing an accurate analysis of video compression quality.
This use of AI for quality analysis in video compression shows how the application of artificial intelligence can streamline and optimize many processes in the tech industry, especially areas that require customer feedback to assess the quality of services.
Conclusion
Video compression is critical technology given the heavy reliance on video content in the digital age. As such, the quality of compressed videos is a priority, and leveraging artificial intelligence to this end, specifically ChatGPT-4, offers a promising solution.
The use of ChatGPT-4 to parse through user comments and feedback for quality analysis makes it possible to identify issues with compression quickly, allowing service providers to act promptly and correct issues faster. This not only enhances the users' experience but also pushes the boundaries for further artificial intelligence applications in quality analysis.
Comments:
Thank you all for reading my article on Revolutionizing Video Compression: The Power of ChatGPT. I'm excited to hear your thoughts and opinions!
Great article, David! Video compression is such an important aspect in today's digital world. I'm curious, how does ChatGPT specifically contribute to revolutionizing video compression?
Thanks, Rebecca! ChatGPT has the ability to understand contextual information and generate human-like responses. By utilizing ChatGPT, we can improve video compression algorithms to better retain the quality of videos while reducing file sizes.
I believe video compression has come a long way, but there's still room for improvement. Excited to learn more about ChatGPT's role in this!
Absolutely, Michael! Traditional video compression techniques often lead to loss of quality. ChatGPT can help in identifying and retaining important details in videos while compressing them effectively.
It's fascinating how AI is influencing various domains. I wonder how ChatGPT tackles challenges like preserving video details during compression without sacrificing too much quality.
Great question, Laura! ChatGPT uses techniques like contextual understanding and adaptive algorithms to effectively balance compression ratios without compromising significant video details.
I'm always amazed by how technology continues to advance. ChatGPT's potential in video compression sounds promising. Will it be accessible for everyone to use?
Certainly, Eric! Our aim is to make ChatGPT accessible and user-friendly for various applications, including video compression. We want to empower users and developers by providing reliable and efficient tools.
This article opened my eyes to the possibilities of ChatGPT in video compression. It would be interesting to see some concrete results or comparisons with traditional compression methods.
Absolutely, Sophia! In the near future, we plan to conduct extensive research and comparisons to showcase the advantages of using ChatGPT in video compression. Stay tuned!
David, do you envision any challenges or limitations ChatGPT might face in video compression?
Great question, Sophia! While ChatGPT offers tremendous potential, it may face challenges in understanding highly complex scenes or unfamiliar content. It's crucial to continuously improve its training and ensure diverse and extensive datasets.
Thanks for your response, David. Overcoming those challenges will be crucial for widespread adoption. Continuous improvement of ChatGPT's training sounds like the way forward!
Impressive insights, David! I can see a bright future for ChatGPT in the video compression domain. Can't wait to see how it reshapes the industry!
Thank you, Alex! It's an exciting time for advancements in video compression, and I'm thrilled about the potential impact of ChatGPT. Together, we can reshape the industry!
I've been following the development of ChatGPT, and it's impressive to see its applications expanding. Are there any limitations or challenges when using ChatGPT for video compression?
Very true, Patrick! One challenge is the computational resources required to process and compress videos in real-time. We are actively working on optimizing the algorithms to mitigate this limitation.
I'm glad to see continuous advancements in video compression technology. How does ChatGPT handle different video formats and resolutions?
Good question, Rachel! ChatGPT is designed to be flexible and adaptable to various video formats and resolutions. It can intelligently optimize compression techniques based on specific video characteristics.
David, with the rapid growth of 4K and even 8K video content, can ChatGPT help address the challenges of compressing such high-resolution videos?
Absolutely, Rachel! ChatGPT's ability to understand and recognize patterns in high-resolution videos makes it well-suited for tackling the challenges of compressing 4K and 8K content. It can improve efficiency while preserving quality.
That's fantastic, David. I'm impressed by the versatility of ChatGPT in handling different resolution videos. It'll be interesting to witness its potential in action!
David, how does ChatGPT handle the trade-off between compression efficiency and computational requirements? Is it resource-intensive?
Good question, Jessica! While ChatGPT does require computational resources, advancements in hardware and optimization techniques can effectively manage the trade-off. The potential benefits it brings to video compression outweigh the resource considerations.
Thanks for clarifying, David. It's crucial to strike a balance between efficiency and resource utilization. Excited to witness the positive impact ChatGPT can have in real-world scenarios!
David, how does ChatGPT handle different types of video content, like action-packed sequences or slow-paced scenes?
Good question, Ethan! ChatGPT can adapt to different video content by learning from diverse examples during training. It can identify and retain relevant details in action-packed sequences while efficiently compressing slow-paced scenes, ensuring optimal video quality.
That's impressive, David! The ability to handle different types of video content effectively adds another layer of value to ChatGPT. Exciting times for video compression!
I'm impressed by the potential of ChatGPT in video compression. However, are there any privacy or security concerns associated with using this AI technology?
Valid concern, Daniel! We prioritize privacy and security in all our AI applications. While using ChatGPT, data protection measures and secure implementations will be enforced to ensure user confidentiality.
David, considering the ever-evolving nature of video technologies, how can ChatGPT continuously adapt to emerging trends and future requirements?
Excellent question, Daniel! ChatGPT can be trained on up-to-date datasets that incorporate emerging trends and future requirements. Additionally, continuous research and development will enable it to adapt to the evolving landscape of video technologies.
That's fantastic, David! Keeping pace with emerging trends is key in a fast-evolving field like video technologies. Looking forward to witnessing ChatGPT's adaptability firsthand!
David, I'm impressed with ChatGPT's ability to optimize compression based on individual preferences. It promises a personalized viewing experience for users. Exciting times ahead!
David, considering the vast amount of video content produced daily, how can ChatGPT handle the scaling requirements of efficient video compression?
Excellent question, Daniel! ChatGPT can benefit from parallel computing and distributed systems to efficiently handle the scaling requirements of video compression. By utilizing modern technologies, it can tackle the demand for processing large video datasets effectively.
That's fascinating, David! The ability to scale efficiently is crucial in modern video processing. I'm excited to see how ChatGPT can handle this demand!
Video compression is crucial for streaming platforms. With ChatGPT's involvement, will we see significant improvements in streaming quality?
Absolutely, Sarah! By leveraging ChatGPT for video compression, streaming platforms can deliver higher quality streams while optimizing bandwidth usage. Users can expect a better streaming experience!
ChatGPT's potential in revolutionizing video compression is fascinating. What is the estimated timeline for incorporating ChatGPT into existing video compression infrastructure?
Great question, Ryan! While it's challenging to provide an exact timeline, we are actively working on integrating ChatGPT into video compression infrastructure. We anticipate significant progress in the next couple of years.
I'm excited about the future of video compression with AI technology like ChatGPT. Will this lead to better video streaming on mobile devices with limited bandwidth?
Absolutely, Emily! ChatGPT's advanced compression techniques can greatly benefit mobile devices with limited bandwidth. Users will experience smoother streaming and reduced buffering.
It's intriguing to see AI contributing to video compression advancements. Will ChatGPT's algorithm continuously improve itself based on user feedback and usage patterns?
Certainly, Melissa! Feedback and usage patterns play a crucial role in the continuous improvement of ChatGPT's algorithm. The more data it receives, the better it becomes at optimizing video compression.
This article gave me a new perspective on video compression. How can developers and researchers contribute to the advancements of ChatGPT in this domain?
Wonderful question, Jason! Developers and researchers can contribute by collaborating, sharing their insights, and helping us gather more data. We believe in the power of community-driven advancements.
Congratulations on the article, David! This AI-based approach to video compression indeed piques my interest. Do you foresee any challenges in the adoption of ChatGPT for this purpose?
Thank you, Nancy! One challenge lies in the integration of ChatGPT with existing video compression workflows and standards. We are actively working towards overcoming this hurdle to ensure a smooth adoption process.
Video compression is vital in various fields. How does ChatGPT fare when it comes to real-time applications, such as online video conferences?
Excellent question, Matthew! Real-time applications like video conferences pose unique challenges due to latency requirements. We are optimizing ChatGPT to handle real-time compression efficiently, catering to such applications.
I'm thrilled about the potential impact of ChatGPT in video compression. Will it be compatible with existing video codecs or require a completely new framework?
Great question, Ava! ChatGPT can complement existing video codecs by enhancing their compression capabilities. It's designed to be adaptable to different frameworks, making integration smoother.
Video compression is an ever-evolving field. How does ChatGPT tackle the trade-off between compression ratio and video quality?
Indeed, George! ChatGPT leverages advanced algorithms to find an optimal balance between compression ratio and video quality. It aims to maximize efficiency while minimizing quality degradation.
ChatGPT's potential in video compression is exciting. Are there any specific use cases where it has shown remarkable results?
Absolutely, Olivia! ChatGPT has shown remarkable results in scenarios where videos have complex scenes with high levels of detail. Its adaptive algorithms excel in retaining quality while compressing such videos.
I'm intrigued by ChatGPT's involvement in video compression. What criteria do you consider when determining the effectiveness of video compression algorithms?
Good question, Andrew! The effectiveness of video compression algorithms is evaluated based on criteria like compression ratio, video quality, encoding speed, and compatibility with existing formats.
This article sheds light on the potential future of video compression. Will ChatGPT's integration require significant computational power?
Valid concern, Emma! While ChatGPT's integration requires computational power, we are actively optimizing and exploring techniques to ensure efficient compression without excessively high resource requirements.
I'm thrilled to see advancements in video compression technology. Does ChatGPT support parallel processing for faster compression?
Absolutely, Joshua! ChatGPT can leverage parallel processing techniques to accelerate video compression. This ensures faster compression times and improved processing efficiency.
I'm fascinated by the potential impact of ChatGPT in video compression. What are your thoughts on the future of video codecs in relation to AI advancements like ChatGPT?
Great question, Lily! The future of video codecs involves closer collaboration with AI advancements like ChatGPT. We can expect smarter codecs that provide superior compression while preserving video quality.
ChatGPT's role in video compression holds promising possibilities. Are there any specific challenges you faced during its development for this particular use case?
Certainly, Isaac! One challenge was training ChatGPT on video-specific data due to its sequential nature. We had to carefully design the training process to ensure effective utilization for video compression.
This article highlights how AI can revolutionize video compression. How does ChatGPT handle audio compression alongside video?
Good question, Grace! While ChatGPT's main focus is video compression, it can incorporate audio compression techniques alongside video to provide an integrated approach and enhance the overall compression efficiency.
It's fascinating how AI is transforming various fields. How does ChatGPT deal with minimizing artifacts introduced during video compression?
Indeed, William! ChatGPT uses advanced algorithms to minimize artifacts by capturing and preserving essential details while adapting the compression techniques to reduce visual distortions effectively.
Video compression is crucial for storage and transmission. Will ChatGPT's involvement lead to significant advancements in both areas?
Absolutely, Victoria! ChatGPT's advancements in video compression will benefit both storage and transmission. Users can store more videos without sacrificing quality, and networks can transmit videos more efficiently.
I'm curious to know about the target audience for ChatGPT's video compression capabilities. Is it more geared towards consumers, professionals, or both?
Good question, Aiden! ChatGPT's video compression capabilities can be valuable for both consumers and professionals. It will empower individuals to compress and store videos effectively, while professionals can enhance their workflows.
Video compression plays a significant role in the entertainment industry. How do you see ChatGPT contributing to enhancing the streaming experience for viewers?
Spot on, Hailey! ChatGPT can enhance the streaming experience by delivering high-quality streams that require less bandwidth. Viewers will enjoy smoother playback, reduced buffering, and improved overall quality.
This article was an interesting read, David! How does ChatGPT handle real-time video compression for live broadcasts or sports events?
Thank you, Ella! Real-time video compression for live broadcasts is an exciting area. ChatGPT's adaptive algorithms and parallel processing techniques are designed to optimize compression in such scenarios effectively.
AI's impact on video compression is fascinating. Does ChatGPT work well with low-resolution videos, or does it primarily benefit high-resolution content?
Great question, Ashley! ChatGPT can enhance both low and high-resolution videos. It aims to find the right balance for each, ensuring efficient compression while preserving crucial details important for the respective resolutions.
Video compression has become a necessity on various platforms. Does ChatGPT provide any control to users regarding the level of compression they prefer?
Certainly, Aaron! ChatGPT can offer control to users in determining the level of compression. Users can have preferences regarding specific video qualities and the desired balance between file size and video quality.
I'm interested in the practical applications of ChatGPT in video compression. Any insights on its potential impact in industries like healthcare or surveillance?
Excellent question, Claire! ChatGPT can have significant applications in healthcare and surveillance industries. It can aid in compressing and storing medical imaging videos or optimizing bandwidth in surveillance systems, among others.
The potential of ChatGPT in video compression is intriguing. Are there specific hardware requirements to leverage its capabilities optimally?
Valid concern, Joseph! While leveraging ChatGPT can benefit from higher compute resources, we aim to make it accessible across various hardware setups. Optimization techniques will ensure optimal capabilities on different systems.
I'm excited about ChatGPT's potential in video compression. How does it handle videos with dynamic scenes or rapidly changing content?
Great question, Anna! ChatGPT's intelligent algorithms can adapt to videos with dynamic scenes or rapidly changing content. They can optimize compression accordingly to reduce artifacts and ensure high-quality output.
Video compression has influenced various industries. Can ChatGPT optimize compression techniques based on specific industry needs, like e-learning or video surveillance?
Absolutely, Samuel! ChatGPT's flexibility allows it to optimize compression techniques based on specific industry needs. It can adapt to e-learning platforms requiring smaller file sizes or surveillance systems prioritizing certain video details.
I'm impressed by ChatGPT's potential in video compression. How can users ensure the security of their compressed videos while using this technology?
Valid concern, Hannah! We prioritize user security. Implementing secure data transfer protocols, encryption techniques, and permission-based access controls can ensure the security of compressed videos when utilizing ChatGPT.
AI advancements like ChatGPT open up new possibilities. How can ChatGPT help in reducing bandwidth constraints for video streaming platforms?
Great question, Nathan! ChatGPT's intelligent compression algorithms can optimize video streaming platforms by reducing file sizes without compromising quality. This, in turn, reduces bandwidth constraints and ensures smoother streaming experiences.
I'm fascinated by the potential of ChatGPT in video compression. Will it be compatible with different operating systems and platforms?
Certainly, Alice! ChatGPT's compatibility extends to different operating systems and platforms. We aim to make it easily integrable and accessible across a wide range of setups.
David, can ChatGPT optimize the compression of different video genres like movies, sports, or documentaries? Curious to know!
Great question, Alice! ChatGPT can indeed optimize compression for various video genres. By learning from a diverse range of examples, it can understand the unique characteristics and adapt the compression algorithms accordingly.
That's impressive, David! Being able to adapt compression to different genres ensures viewers can enjoy optimized video quality regardless of what they're watching. Amazing!
Video compression is crucial for efficient digital workflows. Will ChatGPT's integration introduce any specific software requirements for users?
Good question, Tom! While ChatGPT's integration may introduce some software requirements, we aim to minimize them and provide user-friendly software solutions for seamless integration into existing workflows.
I'm excited to see how ChatGPT transforms video compression. How does it handle videos with large file sizes or longer durations?
Spot on, Madison! ChatGPT's intelligent compression techniques can effectively handle videos with large file sizes or longer durations. It aims to optimize compression while retaining quality, regardless of video length.
This article emphasizes the significance of efficient video compression. How does ChatGPT address the issue of long encoding times in traditional compression methods?
Good question, Robert! ChatGPT utilizes parallel processing and optimization techniques to reduce encoding times. It aims to provide faster video compression without sacrificing the quality achieved by traditional methods.
David, when can we expect to see ChatGPT's impact on video compression in real-world applications? Can you share any upcoming developments?
Robert, while I cannot provide specific timelines, I'm optimistic about the potential of ChatGPT in the real-world applications of video compression. Ongoing research and collaborations aim to bring these advancements to the industry soon!
Thanks for the response, David. The potential impact of ChatGPT in real-world video compression is exciting. I'll be eagerly following its progress!
AI advancements can reshape industries. What's the approach of ChatGPT regarding patents and open-source contributions?
Great question, Evelyn! We believe in a balance between patents and open-source contributions. While protecting innovation through patents, we also encourage open-source collaborations to drive community-driven advancements.
The potential of ChatGPT in video compression sounds promising. Are there any specific video genres or content types where it can demonstrate exceptional performance?
Certainly, Isabella! ChatGPT excels in various video genres and content types. It can demonstrate exceptional performance in areas like action-packed videos, artistic content with intricate details, or even videos with complex visual effects.
The impact of AI in video compression is evident. How does ChatGPT handle specific challenges unique to virtual reality (VR) or augmented reality (AR) videos?
Good question, Sophie! VR or AR videos come with their own set of challenges. ChatGPT is designed to adapt to these challenges, optimize compression techniques for immersive video experiences, and retain crucial details despite the unique characteristics.
Thank you all for your wonderful questions and valuable insights on the potential of ChatGPT in video compression. It's inspiring to see the enthusiasm around this topic!
Thank you, David, for addressing all our queries and engaging with us. The potential of ChatGPT in video compression is indeed exciting, and we look forward to witnessing its advancements!
Great discussion! I learned a lot about ChatGPT's role in revolutionizing video compression. Thank you, David, and everyone else for sharing your thoughts and insights!
Indeed, Emily! This discussion provided valuable information on the impact of ChatGPT in the field of video compression. Thank you, David, and all the participants!
Thank you, David and everyone else, for such an insightful discussion. I'm looking forward to witnessing the advancements ChatGPT brings to video compression!
This discussion has been enlightening! Thank you, David, for providing valuable insights on ChatGPT's potential in video compression.
Thank you, David, for answering our questions. The potential of ChatGPT in video compression is indeed promising!
Thank you, David, for your detailed responses. It was great learning about ChatGPT's role in video compression!
Thank you, David, and everyone else, for the informative discussion. I'm excited about how ChatGPT will influence video compression!
Thank you, David, for providing us with insights into ChatGPT's potential in video compression. Looking forward to the future advancements!
Thank you all once again for your active participation and insightful questions. Your engagement fuels our motivation to continue pushing the boundaries of video compression with ChatGPT!
Thank you all for reading my article on Revolutionizing Video Compression: The Power of ChatGPT. I'm delighted to discuss this exciting topic with you!
Great article, David! Video compression has come a long way, and I'm intrigued to learn how ChatGPT can contribute to this field. Looking forward to your insights!
I've always been fascinated by video compression techniques, and this article caught my attention. Can't wait to see how ChatGPT can revolutionize the industry!
Emily, I've always been fascinated by how video streaming services handle compression. Do you think ChatGPT can improve the user experience in that regard?
Definitely, Michael! With ChatGPT's capabilities, video streaming services can optimize compression quality, reduce buffering, and deliver a seamless user experience. It has tremendous potential to enhance the streaming industry.
Thanks for the response, Emily. I'm excited to see the impact ChatGPT can have on the streaming industry. It's incredible how AI can reshape video technologies!
Michael, I agree with your enthusiasm. The potential impact of ChatGPT on the streaming industry is immense. Looking forward to witnessing its influence firsthand!
Hey Michael, I couldn't agree more. ChatGPT's potential to improve the user experience in video streaming is immense. Exciting times ahead!
Absolutely, Maria! Improved video compression can enhance the streaming experience and enable users to enjoy high-quality content even with limited bandwidth. The future is promising!
Michael, I completely agree. The streaming industry is set to benefit tremendously from ChatGPT's capabilities. Can't wait to experience the improvements firsthand!
Emily, as a content creator, I'm excited about the prospects of ChatGPT in video streaming. Faster encoding and improved quality will greatly benefit creators and viewers alike!
Hi David, as a videographer, I'm curious about the practical implications of using ChatGPT in video compression. Could you shed some light on that?
Peter, Emily, Lisa, thank you for your kind words and interest! ChatGPT has the potential to significantly enhance video compression algorithms. It can learn to analyze and understand complex visual scenes, improving encoding and compression efficiency.
Video compression has always been a challenge. How can ChatGPT help overcome current limitations of existing algorithms?
Good question, Susan! ChatGPT can assist by learning patterns, redundancies, and adaptive encoding schemes, promoting better compression quality with reduced bandwidth requirements.
David, I'm wondering if ChatGPT can optimize video compression based on individual preferences. Can it adapt to different user requirements?
Absolutely, Jacob! That's another exciting aspect of ChatGPT. By learning from user feedback, it can personalize compression algorithms, optimizing video quality, and reducing artifacts based on individual preferences.
David, how does ChatGPT handle different video formats and resolutions? Can it adapt to them efficiently?
Great question, Elizabeth! ChatGPT can indeed handle various formats and resolutions. It can learn from a comprehensive dataset to generalize knowledge across different video types, ensuring efficient adaptation.
That's impressive, David! Compatibility across formats and resolutions is crucial in video compression. Can't wait to see this technology in action!
That sounds impressive, David. I can see how that level of adaptability can be a game-changer for video compression. Exciting times ahead!
Susan, I couldn't agree more. The adaptability of ChatGPT in video compression can be truly game-changing. Exciting times!
Indeed, Steven! The potential that ChatGPT brings to enhance video compression and ultimately improve user experiences cannot be understated. Let's embrace the future!