Revolutionizing Wildlife Observation: Enhancing Machine Vision with ChatGPT
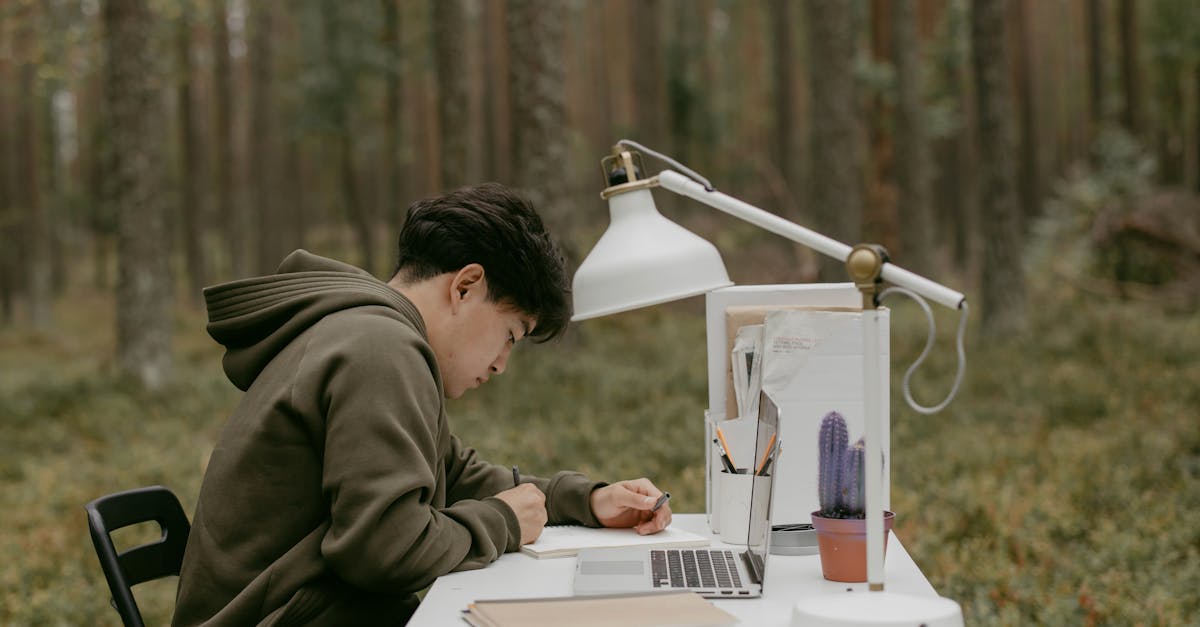
In recent years, technological advancements have greatly improved our ability to study and conserve wildlife. One such technology is Machine Vision, which has proved to be a valuable tool in wildlife observation, especially when it comes to identifying species from camera trap images. This innovative technology, combined with the powerful AI capabilities of the latest natural language processing model ChatGPT-4, opens up new opportunities for biodiversity studies and conservation efforts.
Overview of Machine Vision
Machine Vision, also known as computer vision, is a field of technology that enables computers to interpret and understand visual information from images or videos. It involves the development of algorithms and techniques to extract meaningful data from visual inputs, just like humans do with their eyes and brain. These algorithms can recognize objects, shapes, and even specific features within an image.
Application in Wildlife Observation
Camera traps are widely used in wildlife research and conservation projects. They help capture images and videos of animals in their natural habitats, allowing researchers to monitor animal populations, behavior, and distribution patterns. However, manually analyzing the vast amount of data collected from camera traps can be time-consuming and labor-intensive.
This is where Machine Vision comes in. By leveraging its algorithms, researchers can automate the identification process of wildlife species in camera trap images. With the integration of ChatGPT-4, a language model that excels in understanding natural language prompts, it becomes even easier to analyze and categorize camera trap images. The combination of these technologies provides a more efficient and accurate approach to wildlife observation.
Promoting Biodiversity Studies
The ability to automatically identify species from camera trap images using Machine Vision and ChatGPT-4 opens up new possibilities for biodiversity studies. Researchers and conservationists can process large datasets of wildlife images faster than ever before, allowing for more comprehensive studies on species distribution, population dynamics, and ecological patterns.
Moreover, the use of Machine Vision and AI can help in identifying rare or elusive species that are difficult to spot in the field. This aids in understanding the biodiversity of the studied area more effectively and allows for targeted conservation efforts. By accurately identifying species and monitoring their populations, conservation strategies can be tailored accordingly, ensuring the preservation of vulnerable and endangered species.
Conservation Efforts
Machine Vision in wildlife observation not only aids biodiversity studies but also plays a vital role in conservation efforts. The automated identification of species from camera trap images enables early detection of illegal activities, such as poaching or habitat destruction. Conservation organizations can utilize the technology to develop real-time monitoring systems, alerting authorities in cases of potential threats to wildlife.
Furthermore, the data obtained through Machine Vision algorithms can provide valuable insights into the impact of environmental changes on wildlife populations. By understanding how species are responding to ecological shifts, conservationists can implement effective measures to mitigate negative impacts and protect habitats.
Conclusion
Machine Vision, in combination with natural language processing models like ChatGPT-4, is revolutionizing wildlife observation and conservation efforts. The ability to automatically identify species from camera trap images promotes biodiversity studies and empowers researchers and conservationists to protect wildlife more efficiently. As technology continues to advance, we can expect even greater contributions from Machine Vision in uncovering the secrets of the natural world and preserving its magnificent diversity.
Comments:
Thank you all for joining the discussion on my article! I'm excited to hear your thoughts on revolutionizing wildlife observation.
Great article, Nell! The concept of enhancing machine vision with ChatGPT sounds fascinating. Can you provide more details on how this integration could revolutionize wildlife observation?
I'm curious too, Sarah. Nell, could you explain how machine vision and ChatGPT together enhance wildlife observation?
Certainly, Sarah and Mark. Machine vision combined with ChatGPT provides an innovative approach to wildlife observation. ChatGPT can process and analyze real-time video feeds from cameras installed in natural habitats, while also generating textual descriptions of the observed wildlife. This integration enables researchers to gather more detailed information about animal behavior, population dynamics, and interactions.
That sounds promising, Nell! Could you elaborate on how the textual descriptions from ChatGPT are generated? How accurate can they be?
Great question, Emma. The textual descriptions are generated using natural language processing algorithms. ChatGPT analyzes the video frames from the cameras and generates descriptions based on learned patterns and contextual understanding. However, it's important to note that since the system is trained on large amounts of data, the accuracy of textual descriptions might vary depending on the context and quality of the video feed.
Nell, I agree that this technology has the potential to benefit conservation efforts. By providing more accurate data, it could help prioritize conservation actions and allocate resources effectively.
I completely agree, Nell. Accurate and more detailed data can assist conservation organizations in creating targeted initiatives, which could be more impactful in preserving wildlife and their habitats.
Nell, this integration seems like it could significantly improve wildlife research. How do you think it would impact conservation efforts?
That's an excellent point, Daniel. Enhancing wildlife observation with machine vision and ChatGPT can have several positive impacts on conservation efforts. Firstly, it allows researchers to gather more data about wildlife behavior and habitat conditions, which can inform conservation strategies more accurately. Furthermore, the technology can help in identifying endangered species, monitoring their movements, and detecting potential threats to their habitats.
Nell, I appreciate your response. The potential impact on conservation efforts makes this integration exciting. I look forward to seeing it implemented on a larger scale.
I'm curious about the practical application of this integration. Are there any ongoing projects or organizations implementing this technology?
Absolutely, Samantha. Several ongoing projects are utilizing this technology to enhance wildlife observation. For example, the Wildlife Conservation Society is currently testing this integration in their study on primate behavior in the rainforests. It has shown promising results in identifying different primate species, understanding their social interactions, and monitoring changes in their habitats.
I appreciate your insights, Nell. The ethical implications, privacy concerns, and accuracy of wildlife identification are indeed important aspects that must be carefully addressed in implementing this technology.
Nell, what are the potential limitations or challenges that researchers might face when implementing this integration?
Good question, Robert. Implementing this integration does come with some challenges. One significant challenge is the identification and classification of wildlife in diverse and complex environments accurately. Despite advancements, machine vision systems can still face difficulties in differentiating closely related species or species with similar physical features. Additionally, ensuring the privacy of wildlife and addressing ethical concerns related to video monitoring are important aspects to consider.
Thank you for addressing the potential challenges, Nell. It's essential to overcome these obstacles to ensure the successful implementation of this technology and its positive impact on wildlife conservation.
This integration sounds incredibly promising for wildlife research and conservation. Nell, do you think it could also be utilized for studying marine life and underwater ecosystems?
Absolutely, Oliver! This integration holds great potential for studying marine life and underwater ecosystems as well. By deploying underwater cameras and integrating machine vision with ChatGPT, we can gain valuable insights into the behavior, distribution, and health of marine species. It could aid in assessing the impact of human activities, such as fishing or pollution, on underwater ecosystems.
That's fantastic, Nell! The ability to study marine life and underwater ecosystems more effectively could lead to better protection and conservation efforts in these vulnerable environments.
This article raises interesting questions about the future of wildlife conservation. In addition to the benefits, what are the potential risks associated with relying heavily on technology for gathering wildlife behavioral data?
You're absolutely right, Rachel. While the integration of technology brings numerous benefits, there are also risks to consider. Heavy reliance on technology for data gathering may reduce the interaction between researchers and natural habitats, potentially leading to the oversight of important observations that automated systems may miss. It's crucial to find a balance between using technology as a tool and maintaining direct engagement with wildlife to ensure comprehensive and accurate conservation efforts.
Nell, studying underwater ecosystems using this integrated technology could be groundbreaking, considering the challenges of marine research. It has the potential to expand our knowledge and contribute to more effective conservation strategies.
Nell, what kind of computational resources are required to implement this integration on a large scale? Could it be feasible for smaller research organizations with limited budgets?
Valid concern, Liam. Implementing this integration on a large scale does require substantial computational resources. However, with advances in cloud computing and the availability of machine learning platforms, it is becoming more accessible, even for smaller research organizations. Additionally, collaborations between research institutions and technology companies can help overcome cost barriers and make the technology more widely available.
I can see how this integration can benefit wildlife conservation efforts greatly, Nell. What are the next steps in further developing and refining this technology?
Thank you, Emily. The next steps involve enhancing the accuracy and reliability of machine vision algorithms by continually training them with high-quality data. Research and development efforts are underway to address the challenges faced by current systems, such as species identification accuracy and ethical concerns. Additionally, user feedback and collaboration between experts in the fields of wildlife observation, machine learning, and conservation will play a crucial role in advancing this technology.
Nell, integrating machine vision with ChatGPT not only provides in-depth analysis of wildlife behavior but also offers researchers a powerful tool to efficiently process vast amounts of video data. This technology opens new avenues for wildlife observation and conservation research.
Thank you for elaborating, Nell. This integration seems like a game-changer in the realm of wildlife observation. It has the potential to significantly improve our understanding of animal behavior and support conservation efforts worldwide.
Finding the right balance between technology and hands-on research is crucial. Ensuring that human observers are still involved can help capture comprehensive data and maintain a multifaceted approach to wildlife conservation.
Collaborations between research organizations and technology companies seem like a promising way to make this technology more accessible. It would be great to see these partnerships grow to support smaller research initiatives as well.
It's interesting to learn about ongoing projects utilizing this technology. The study on primate behavior sounds fascinating. I hope to see similar initiatives focusing on other species as well.
Conservation efforts could greatly benefit from the combination of advanced technology and wildlife observation. It has the potential to create a comprehensive understanding of ecosystems and aid in strategic decision-making.
The accessibility of this technology to smaller research organizations is crucial. It would help enhance biodiversity monitoring and conservation efforts in areas where resources might be limited.
While technology can be a powerful tool, it's important to acknowledge that it cannot replace the value of firsthand experience and direct engagement with wildlife. Balancing the use of technology and field research is vital for comprehensive conservation approaches.
Indeed, Rachel. Although technology can enhance efficiency, it should complement, not replace, the invaluable knowledge that field researchers gain through direct observation and continuous monitoring.
Absolutely, Liam. Combining on-the-ground research with technological tools can lead to a comprehensive understanding of wildlife ecosystems, empowering conservation organizations to act more efficiently and make a larger impact.
Addressing the challenges of accurate species identification and privacy concerns associated with video monitoring is crucial. Overcoming these obstacles will pave the way for more successful implementations of this technology.
Marine life and underwater ecosystems face unique conservation challenges. Utilizing this technology to gain insights into these habitats can aid in their preservation and support wider marine conservation efforts.
Continuous collaboration and feedback from various stakeholders, including researchers, conservationists, and technologists, will be crucial in refining this technology. Ensuring its development aligns with real-world requirements and effectively addresses conservation challenges.
I'm excited to see the continuous improvement of this technology. The adaptation of machine learning and natural language processing for wildlife observation holds immense potential and could redefine our approach to conservation research.
Rachel, I agree. The responsible use of technology in wildlife research must be accompanied by a holistic approach that includes interdisciplinary collaboration and a sound understanding of the natural complexities of ecosystems.
Indeed, Rachel. Both technology-driven and boots-on-the-ground approaches have their merits. By combining them effectively, we can gain valuable insights that contribute to evidence-based conservation decision-making.
Balancing technological advancements with traditional research methods will indeed be key in leveraging this integration's potential effectively. It's a step towards more data-driven and informed decision-making in conservation.
The utilization of this technology for studying marine life can help shed light on the mysteries of our oceans and support global efforts to conserve and protect underwater ecosystems.
It's promising to hear about ongoing projects. Implementing this technology in diverse ecosystems will provide valuable insights into various species and habitats, contributing to a more comprehensive understanding of wildlife globally.
Marine conservation requires extensive research, but the vastness and complexity of underwater environments pose significant challenges. The integration of machine vision with ChatGPT could be a game-changer in expanding our knowledge of marine life.
Developing this technology further by incorporating user feedback is crucial. It ensures that the resulting systems align with the diverse needs and challenges faced by wildlife researchers and conservationists.
Collaborations between research institutions and technology companies can not only help overcome cost barriers but also ensure the development of user-friendly and accessible tools, benefiting researchers and conservation practitioners worldwide.
This technology has the potential to revolutionize conservation efforts, enabling more efficient and comprehensive monitoring of wildlife populations and habitats. Exciting times ahead!