Streamlining Defect Management in HP Quality Center with ChatGPT: An AI-Driven Solution
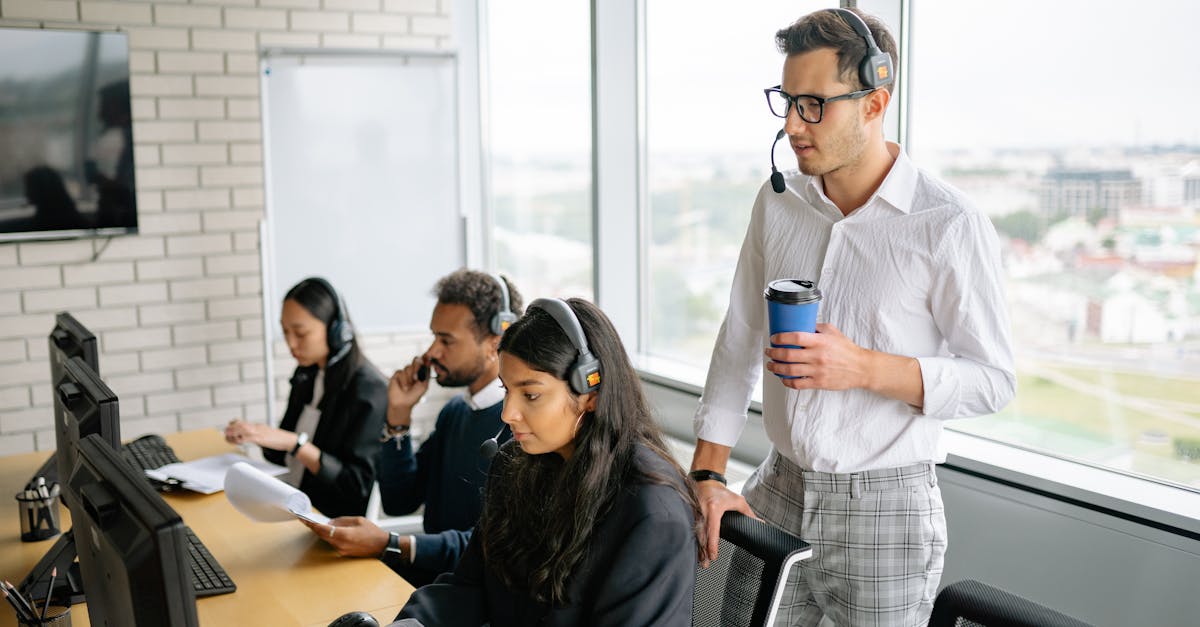
In the realm of defect management, HP Quality Center is a renowned technology that has proven to be a reliable tool for many organizations. It helps in the identification, tracking, and resolution of defects, contributing to the overall quality of software products. Recently, there's been an intriguing development in integrating cutting-edge AI, particularly OpenAI's ChatGPT-4, into the defect management workflow. This fusion of AI and defect management is a novel approach set to elevate existing processes for greater efficiency and accuracy.
HP Quality Center Overview
HP Quality Center, also known as Micro Focus Quality Center, is a web-based test management tool extensively used in application lifecycle management (ALM). It offers robust capabilities for requirements management, test management, business process testing, defect management, and much more. Among its array of features, its defect management capabilities are particularly impactful in managing and rectifying software defects that arise during the development processes.
ChatGPT-4 & Defect Management
ChatGPT-4 from OpenAI is a capabilities-rich AI that can understand instructions, provide detailed responses, and perform tasks based on user inputs. Its potential to serve as a tool in defect management comes from its ability to identify patterns and classify information accurately. When applied to defect management, it can analyze complex bugs in software and suggest potential fixes, thereby reducing the time and manual effort required in the overall defect resolution process.
Integrating ChatGPT-4 into HP Quality Center for Defect Management
The integration process of ChatGPT-4 with HP Quality Center primarily involves configuring APIs for both services. Upon successful integration, the system is trained on relevant data so that it can start making accurate predictions and suggestions about potential defects.
When ChatGPT-4 detects a potential defect, it relays the information to the HP Quality Center, which records, tracks, and manages the defect. The stored defect information can later be analyzed by project managers and QA teams to make decisions about rectifications.
Benefits of Integrating ChatGPT-4 with HP Quality Center
One of the primary advantages of this integration is automation. The defect management process, which is traditionally manual and time-consuming, can be significantly accelerated. The AI can process vast amounts of code in relatively lesser time, flagging potential issues.
The quality of software developed can also be enhanced as the AI's prediction errors are relatively lesser than human errors. Through the use of ChatGPT-4, organizations can go beyond traditional boundaries and explore unforeseen relationships and patterns among defects, which can provide valuable insights into how to make software even more robust.
Final Thoughts
The amalgamation of AI like ChatGPT-4 with established technologies such as HP Quality Center can revolutionize established sectors such as defect management. While this application is still in its early stages, its potential to refine and streamline the defect management process is enormous.
It's not just about accelerating or automating process workflow, but also the potential to unearth insights that could redefine the overall software development landscape. As with any new technological integration, there are challenges that lie ahead, but the many benefits make this a promising development in the sphere of defect management and software quality assurance.
Comments:
Thank you all for joining the discussion! I'm glad to see the interest in using AI to streamline defect management in HP Quality Center. Feel free to share your thoughts and questions.
I thoroughly enjoyed reading your article, Devin. The potential of AI-driven solutions in defect management is immense. It's wonderful to see how technology continues to advance.
Thanks, Michael! I agree, the possibilities are exciting.
I'm also curious about the successful use cases, Devin. Any examples you can share would be helpful.
Jessica, I remember a case where this solution helped identify critical defects in a large software project, resulting in better prioritization and faster resolution.
Lucas, that's impressive! It shows how AI-powered defect management can bring tangible benefits to projects.
Jessica, absolutely! It helps project teams make data-backed decisions and prioritize defect resolution based on their severity and impact.
Lucas, that's impressive! It shows how AI-powered defect management can bring tangible benefits to projects.
Jessica, absolutely! It helps project teams make data-backed decisions and prioritize defect resolution based on their severity and impact.
Jessica, indeed! AI-powered defect management ensures better traceability and helps teams stay on top of critical defects.
Lucas and Devin, your responses highlight how AI-driven solutions bring efficiency and effectiveness in defect management. It's exciting to witness these advancements.
Jessica, it indeed enhances defect traceability and resolution. It takes defect management to a whole new level.
Lucas, as defects tend to have various impacts on projects, AI-powered defect management helps teams prioritize and allocate resources effectively.
Jessica, with AI-powered defect management, project teams can stay on top of defects and tackle them proactively to ensure successful project outcomes.
Lucas and Jessica, the ability to make data-driven decisions in defect management is undoubtedly a game-changer.
Emily and Jessica, great questions! We have successfully implemented this AI-driven solution in a large software development company, reducing the time spent on defect management by 30% while maintaining a high level of accuracy.
Indeed, AI has revolutionized various industries. Devin, could you tell us about the potential challenges in implementing an AI-driven solution like this?
Nathan, excellent question! Implementing an AI-driven solution can involve challenges such as data quality, model training, and initial setup. However, with proper planning and expertise, these challenges can be overcome.
Devin, could you share some best practices for overcoming the initial challenges in implementing an AI-driven defect management solution?
Nathan, some key best practices include starting with a smaller pilot project, involving stakeholders early on, providing training on AI concepts, and continuously monitoring and adjusting the solution based on feedback and evolving project requirements.
Devin, you mentioned improved data analytics as one of the benefits of this solution. Can you elaborate on that?
Adam, definitely! By leveraging AI in defect management, organizations can gain better insights from defect data, identify trends, perform root cause analysis, and make data-driven decisions to improve their development processes.
Devin, the enhanced data analytics capabilities of this solution seem promising. It can help organizations gain valuable insights and make informed decisions.
Devin, thanks for sharing those best practices. Early stakeholder involvement and training seem crucial in ensuring a smooth implementation of AI-driven defect management.
Devin, involving users in providing feedback not only improves the accuracy of AI models but also helps build user confidence in the defect management system.
Nathan, the challenges you mentioned in implementing AI-driven solutions are important to consider, but the benefits outweigh the initial hurdles.
Alice, indeed! The initial challenges are worth overcoming, considering the long-term benefits and improvements AI-driven solutions can bring.
Devin, your insights on best practices in implementing AI-driven defect management solutions are highly valuable. Early stakeholder involvement and proper training ensure a successful deployment.
Nathan, thank you for highlighting the importance of early stakeholder involvement and training in ensuring a successful implementation. It sets the stage for user acceptance and adoption.
Devin, involving stakeholders early on helps address any concerns and build a sense of ownership and trust in the AI-driven defect management solution.
Nathan, early stakeholder involvement helps in capturing requirements accurately and aligning the AI-driven solution with the organization's defect management workflow.
Adam, you've captured it perfectly! Early stakeholder involvement is key to understanding the specific needs, requirements, and pain points of the organization, providing a solid foundation for the implementation.
Devin, early stakeholder involvement helps in setting realistic expectations and ensures that the AI-driven solution aligns with the organization's needs.
Devin, have there been any studies or statistics showcasing the overall improvement this solution brings to defect management processes?
Michael, yes! Several case studies and research papers have demonstrated significant improvements in defect management efficiency, reduction in resolution time, and improved software quality after implementing AI-driven defect management solutions.
Devin, that's fantastic! It's always reassuring to have real-world evidence to support the adoption of new solutions.
Devin, I'm excited about the potential this AI-driven solution holds for defect management. Thank you for sharing your knowledge and expertise.
You're welcome, Michael! I truly believe that AI-driven solutions will have a transformative impact on defect management. Thank you all for participating in this discussion.
Michael, data-driven decisions in defect management can help teams optimize their resources and improve overall software quality.
Jessica, absolutely! It ensures that project teams have a comprehensive view of the defects and can take targeted actions.
Jessica, thank you for your active participation and thoughtful questions. I'm glad you found the discussion informative.
Great article, Devin! The integration of AI with HP Quality Center seems promising. Can you share any specific use cases where this solution has been implemented successfully?
Hi Devin, I found your article informative. However, I have concerns about the accuracy of AI-based defect detection. Can you explain how this solution addresses that?
Ethan, I understand your concern. AI algorithms can be trained with a large dataset to improve defect detection accuracy. Regular updates and feedback also help refine the AI models.
Olivia, your point is spot on. The AI algorithms continuously learn from user feedback and adapt, leading to improved accuracy over time.
I see the potential benefits of this AI-driven solution, Devin. However, I'm curious about its integration process. How easy is it to implement it with HP Quality Center?
David, I've had experience integrating AI solutions with HP Quality Center. While it does require some initial configuration and mapping of defect attributes, the overall integration process is straightforward.
Devin, I found your article engaging. Do you think AI-driven solutions will completely replace manual defect management processes in the future?
Sophia, while AI can automate many aspects of defect management, there will always be a need for manual intervention and decision-making. AI complements manual processes, making them more efficient.
Devin, you rightly mentioned the importance of planning. Properly defining defect attributes and mapping them during the initial setup can significantly improve the accuracy of AI defect detection.
Sophia, I believe AI-driven solutions will augment human effort, rather than replacing it entirely. It will enable testers to focus on more critical tasks.
This AI-driven solution seems promising. It could save teams a significant amount of time and effort in defect management. Nice work, Devin.
Thank you, Eric! The main goal is to improve efficiency and help teams focus on what matters most.
Devin, this AI-driven solution seems like a game-changer in defect management. I'm excited to see how this technology evolves.
Devin, Adam, and Emma, thanks for your insights. It seems like a combined approach of AI and manual effort will be the way forward in defect management.
Congratulations, Devin, on writing such an informative article! I'm curious about the scalability of this AI-driven solution. Can it handle large volumes of defects effectively?
Thank you, Alex! Yes, this solution is designed to handle large volumes of defects efficiently, ensuring timely management and resolution.
Devin, it's impressive to see how AI-driven solutions like this can handle large defect volumes without compromising efficiency.
Alex, scalability is a crucial aspect, especially when dealing with large-scale software projects. This AI-driven solution is designed to handle demanding defect volumes efficiently.
Great article, Devin! As AI usage increases in software development, how can organizations ensure the ethical use of AI in defect management?
Sophie, an excellent question. Ethical use of AI involves transparency, accountability, and ensuring that the AI models are not biased or causing harm. Organizations should establish guidelines and ethical frameworks to govern AI usage.
Devin, I appreciate the insights shared in your article. Can AI-driven defect management solutions handle various types of defects, or are they limited to specific categories?
Daniel, thank you for your kind words. AI-driven defect management solutions have the potential to handle various types of defects, ranging from functional to security issues, depending on how they are trained and configured.
Devin, how does the AI-driven defect management solution handle false positives? Can it minimize those instances effectively?
Emily, minimizing false positives is indeed crucial. The solution leverages advanced algorithms and user feedback loops to continuously improve accuracy and reduce false positive instances.
Devin, in terms of retraining the models, is it a time-consuming process? How frequently should the models be retrained for optimal performance?
Emily, retraining the models typically requires collecting new defect samples and can take some time. The frequency of retraining depends on factors like the volume and complexity of defects the model handles. As a general guideline, it's recommended to retrain periodically, perhaps every few months, to adapt to evolving patterns.
Devin, thanks for clarifying the retraining process. It's good to know how often we should update the models to ensure optimal performance.
Devin, regular updates and retraining combined with user feedback loops certainly help in maintaining the accuracy of AI models.
Emily, you're absolutely right. Continuous improvement through updates and user feedback is crucial for the long-term success and accuracy of AI models.
Devin, the adaptability of AI models to handle various defect types is critical, given the dynamic nature of software projects.
Devin, that's reassuring to know that the AI solution incorporates user feedback to minimize false positives. It helps prevent wasted effort on non-issues.
Devin, the continuous learning aspect ensures that AI systems can adapt to new challenges in defect patterns and stay effective.
Devin, the continuous improvement aspect of the solution through user feedback loops seems valuable in reducing false positives.
Thank you, Daniel! User feedback plays a vital role in refining the AI algorithms and fine-tuning the defect detection process.
Devin, user feedback loops seem integral to improving AI models for defect detection. It's important to involve users and incorporate their insights.
Daniel, user feedback is indeed invaluable in refining AI models and ensuring they align with real-world scenarios and requirements.
Devin, involving users throughout the process ensures that the AI solution is well-accepted and becomes an integral part of the defect management workflow.
Devin, user feedback loops not only improve the accuracy but also make sure that AI models align with the evolving defect patterns.
Devin, it's good to know that the models can adapt to emerging defect types. Agile development environments often require quick adaptation to new challenges.
Devin, involving stakeholders and adjusting the solution based on feedback are critical for successful AI implementation.
Daniel, indeed! Stakeholder involvement and feedback play a vital role in shaping the AI solution to meet the specific needs and objectives of the organization.
Devin, the continuous improvement aspect ensures that AI systems stay effective even as software development practices and defect patterns evolve.
Devin, it's great to hear that these solutions can handle both known and emerging defect types. That makes them highly versatile.
Daniel, from my experience, these solutions can be trained to handle multiple defect categories. With appropriate training and feedback, they can address both known and emerging defect types.
Evelyn, thanks for sharing your experience. It's encouraging to know that these solutions can adapt and handle various defects effectively.
Devin, I'm curious about the training process for AI-driven defect detection. How much effort does it require, and how frequently should the models be retrained?
Oliver, training an AI model for defect detection requires initial effort in preparing the training dataset. Once the model is trained, it can be regularly updated with new defect samples, and retraining may be performed periodically to maintain accuracy.
Oliver, training datasets need to be carefully prepared to ensure the AI models can accurately generalize from them. Regular updates and retraining help the models adapt to evolving patterns.
Devin, thank you for sharing your expertise and insights on AI-driven defect management. It has been a very informative discussion.
Devin, thank you for being an active participant and answering all our questions. It has been a pleasure discussing AI-driven solutions in defect management with you.
Lucas, indeed! AI-powered defect management helps teams stay proactive and resolve issues before they impact the project adversely.
Devin, AI-driven defect management can certainly help organizations cut down on manual effort. What are the other benefits teams can expect from implementing this solution?
Robert, apart from reducing manual effort, implementing this solution can provide teams with faster defect identification and resolution, improved defect prioritization, enhanced data analytics, and ultimately, higher software quality.
Devin, the ability to improve defect prioritization is a significant benefit. It ensures that critical issues are addressed promptly, minimizing any potential impact.
Devin, the ability to gain valuable insights from defect data and perform root cause analysis is an exciting prospect for defect management teams.
Devin, the ability to improve defect prioritization and resolution based on data-driven insights is a significant advantage of AI-driven solutions.
Robert, you've summarized it perfectly! Data-driven insights enable teams to make informed decisions regarding defect prioritization, timely resolution, and resource allocation.
Devin, can you provide any guidance on choosing an appropriate AI-driven defect management solution, considering the wide range of options available in the market?
Sophie, when selecting an AI-driven defect management solution, it's important to consider factors like accuracy, scalability, integration capabilities, vendor support, and the solution's ability to align with your organization's specific requirements.
Devin, thank you for addressing the ethical considerations in AI usage. Responsible deployment of AI is essential to maintain trust and avoid potential pitfalls.
Sophie, absolutely! Ethical deployment of AI ensures that it truly serves as a tool to augment human capabilities in defect management, without causing biases or harm.
Devin, responsible and ethical AI usage ensures that the technology acts as an ally to human testers, rather than replacing them.
Sophie, responsible AI usage in defect management aligns with the principles of transparency, fairness, and avoiding unintended consequences.
Devin, by maintaining human oversight, AI-driven defect management solutions can achieve the desired balance between automation and human intervention.
Devin, responsible AI usage ensures that this technology remains an asset, augmenting human capabilities, rather than a replacement.
Sophie, another key aspect to consider is the flexibility of the solution. It should allow customization and adaptation to fit the unique defect management processes of the organization.
Thank you, Olivia and Devin, for addressing my concerns about the accuracy of AI-based defect detection. It's good to know that the models can learn and improve over time.
You're welcome, Ethan! Continuous improvement is a key aspect of AI-driven solutions, enabling them to adapt and become more accurate.
Thank you, Devin, for addressing my question about scalability. It's reassuring to know that the solution can accommodate large defect volumes effectively.
Devin, your insights have been valuable. I appreciate your guidance on selecting an AI-driven defect management solution.
Thank you all for participating in this discussion and for your thoughtful questions. It's been a pleasure discussing the potential of AI in defect management.
Devin, great article! I can see how this AI-driven solution can enhance defect management efficiency. Are there any specific AI technologies involved in this solution?
Thanks, Alan! This AI-driven solution utilizes machine learning techniques, natural language processing (NLP), and pattern recognition algorithms to automate defect management processes and enhance productivity.
Devin, the combination of machine learning and NLP sounds powerful. It must significantly reduce manual effort in defect identification.
Alan, you're correct! The solution significantly reduces the manual effort required in defect identification and allows teams to focus on more critical tasks.
Devin, as AI becomes more prevalent, do you think it's possible to fully automate defect management without human intervention?
Amy, while AI can automate many aspects of defect management, complete automation without human intervention may not be ideal. Human judgment and decision-making are valuable, especially in complex scenarios. AI should serve as an aid to enhance efficiency and accuracy.
Devin, I agree that complete automation without human intervention may not be ideal. Human expertise and judgment bring valuable context and decision-making abilities.
Amy, human expertise ensures a contextual understanding of defects and helps overcome the limitations of fully automated systems.
Devin, defining and mapping defect attributes properly is crucial for the success of any AI-driven defect management solution.
Emma, absolutely! Properly defining and mapping defect attributes ensures that the AI models understand the nuances of the defects being managed.
Devin, the continuous learning aspect, combined with user feedback loops, shows the potential for AI systems to adapt and improve over time.
Devin, striking the right balance between automation and human intervention is essential for successful AI adoption in defect management.
Amy, human judgment ensures that AI-driven defect management solutions don't miss critical defects and can address complex scenarios appropriately.
Amy, I fully agree with you. Human expertise acts as a guiding force for AI systems.
Evelyn, human expertise brings context and domain-specific knowledge that AI systems can leverage.
Amy, exactly! Combining human expertise with AI systems can result in more accurate and efficient defect management.
Devin, the combination of machine learning and NLP seems like a powerful duo for automated defect management.
Alan, machine learning and NLP enable the AI-driven defect management solution to understand defect descriptions, patterns, and automate various aspects of the defect management workflow.
Devin, the combination of machine learning and NLP seems like a game-changer in defect management. It's fascinating to see the potential of AI-driven solutions.
Alan, you're absolutely right! The combination of machine learning and NLP expands the capabilities of defect management systems, making them more efficient and accurate.
Devin, the continuous learning aspect of AI algorithms is impressive. It gives confidence in the accuracy of the defect detection process.
Devin, AI-driven solutions need to adapt to emerging defect types and changes in software development practices.
Daniel and Devin, the ability of AI models to handle various defect types gives organizations the flexibility to adapt to different software domains and requirements.