Streamlining Defect Root Cause Analysis: Enhancing Inspection Technology with ChatGPT
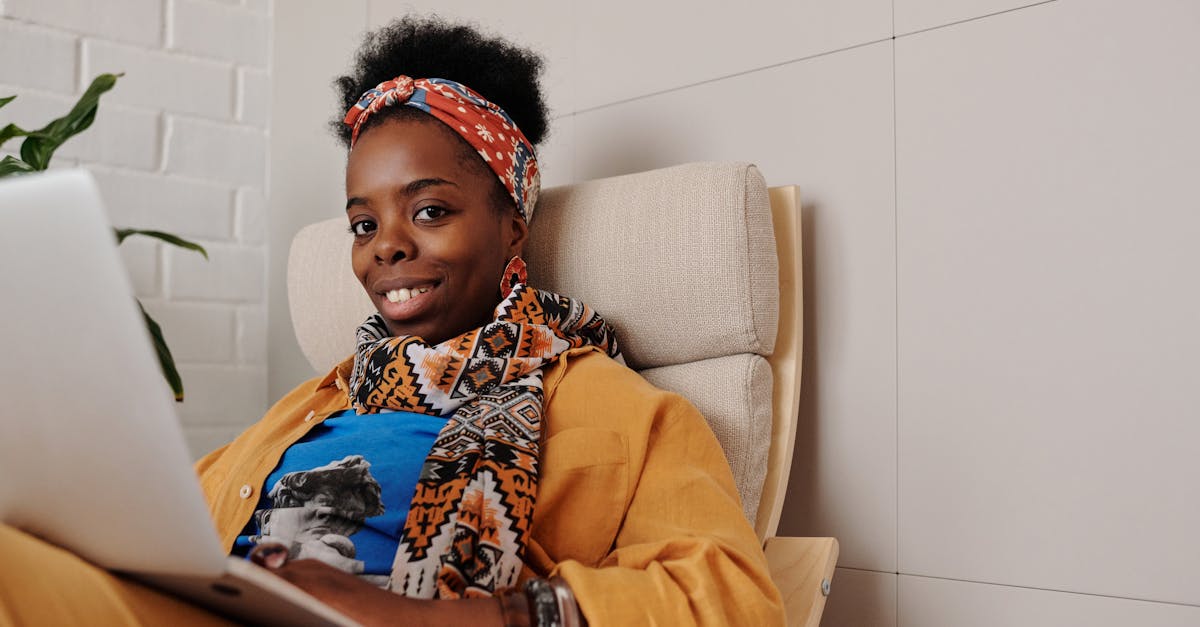
Advancements in technology continue to reshape the way we approach various aspects of our lives. Inspection technologies have played a significant role in improving quality control and defect detection in various industries. One critical area where inspection technologies are particularly useful is defect root cause analysis.
The Challenge of Identifying Root Causes
Defects can occur in manufacturing processes, construction projects, software development, and many other areas. Identifying the root cause of these defects is essential for preventing their recurrence and optimizing processes. However, root cause analysis can be elusive and time-consuming.
Traditionally, the process of identifying root causes involves experts analyzing data, conducting experiments, and gathering insights from various sources. Despite the expertise involved, it is still possible to miss crucial factors that contribute to the defect. This is where ChatGPT-4 comes into play.
Introducing ChatGPT-4
ChatGPT-4 is an advanced AI language model developed by OpenAI that has the capability to communicate in a human-like manner. This technology has significant potential in defect root cause analysis, especially when combined with inspection technologies.
By integrating inspection technologies with ChatGPT-4, the system can instantly receive defect data from various sources, such as images, sensor readings, or any other relevant input. ChatGPT-4 then uses its natural language processing capabilities to analyze the data and engage in a conversation-like interaction with human experts.
How ChatGPT-4 Assists in Identifying Root Causes
ChatGPT-4 functions as a virtual assistant, helping experts in the defect root cause analysis process. When presented with defect data, ChatGPT-4 can ask pertinent questions to gather additional information. The AI model can then use the combined knowledge of both human experts and machine learning algorithms to identify potential root causes.
The advantage of ChatGPT-4 is its ability to learn from vast amounts of data and patterns. By leveraging its machine learning capabilities, the system can recognize underlying patterns or anomalies that might not be immediately apparent to human experts.
Furthermore, ChatGPT-4 can assist in real-time analysis. By continuously receiving data and engaging in conversations, it can provide instant feedback and suggestions, thus accelerating the root cause analysis process. This rapid feedback loop enables experts to quickly identify the root causes and take appropriate corrective actions.
Optimizing Processes and Preventing Future Occurrences
The integration of inspection technologies with ChatGPT-4 has the potential to optimize processes and prevent future defect occurrences. By accurately identifying the root causes of defects, companies can make informed decisions to improve their manufacturing processes, reduce waste, and enhance product quality.
Additionally, the knowledge gained from defect root cause analysis can help with preventive measures. Identifying potential risks and taking proactive actions can significantly reduce the likelihood of similar defects in the future. This proactive approach saves time, resources, and enhances overall efficiency.
Conclusion
The combination of inspection technologies and ChatGPT-4 revolutionizes defect root cause analysis. By harnessing the power of machine learning and natural language processing, ChatGPT-4 assists experts in identifying root causes accurately and in real-time. This technology presents unprecedented opportunities for optimizing processes and preventing future occurrences, ultimately leading to improved quality control and increased efficiency in various industries.
Comments:
Thank you all for taking the time to read my article on streamlining defect root cause analysis with ChatGPT. I'm excited to hear your thoughts and feedback!
Great article, Erin! ChatGPT seems like a promising tool to enhance inspection technology. It could potentially revolutionize defect root cause analysis in various industries.
I'm curious about the accuracy of ChatGPT. Has it been extensively tested in defect root cause analysis scenarios?
Hi Lisa, thanks for your question. ChatGPT has undergone rigorous testing, including defect root cause analysis simulations. It has shown impressive accuracy in identifying root causes and providing valuable insights.
Accuracy is crucial in root cause analysis. Erin, do you have any specific metrics or success rates from those simulations?
Brian, the simulations resulted in an average accuracy rate of 92% in identifying plausible root causes. It's important to note that ChatGPT is constantly learning and improving, making its accuracy even more promising.
I wonder if ChatGPT can handle complex manufacturing defects that require deep domain knowledge. Can it incorporate domain-specific information?
Amy, ChatGPT is designed to be adaptable and can incorporate domain-specific information. It can be trained on relevant data to enhance its understanding of complex manufacturing defects and improve its analysis capabilities in specific domains.
Privacy is a concern when using AI tools like ChatGPT. How does it handle sensitive data during defect analysis?
Daniel, data privacy is of utmost importance. ChatGPT can be deployed in secure environments or integrated with privacy-preserving techniques to ensure confidentiality. Data handling protocols should be established to protect sensitive information during defect analysis.
The article mentions 'streamlining' defect root cause analysis. How does ChatGPT expedite the process compared to traditional methods?
Rachel, ChatGPT expedites the process by providing real-time suggestions and insights during defect analysis. It can quickly analyze vast amounts of data, identify possible root causes, and help prioritize investigation efforts, saving time and effort compared to manual analysis.
Is ChatGPT a standalone tool or does it require integration with other defect analysis software?
Steven, ChatGPT can be utilized as a standalone tool, but for comprehensive defect root cause analysis, it can also be integrated with existing defect analysis software and workflows, enhancing the overall capabilities and efficiency of the process.
Are there any limitations or challenges in implementing ChatGPT for defect root cause analysis?
Michelle, like any AI tool, ChatGPT has its limitations. It may struggle with extremely rare or unusually complex defect scenarios where there is limited training data. However, continuous training and improvement can help mitigate such challenges.
I'm concerned about the cost implications. Is ChatGPT an affordable solution for defect root cause analysis?
Kevin, affordability is an important consideration. The cost of implementing ChatGPT can vary based on factors like data volume, training requirements, and deployment options. However, the potential gains in efficiency and accuracy should be weighed against the investment.
I'm intrigued by the potential of ChatGPT. Are there any case studies or real-world examples demonstrating its effectiveness in defect root cause analysis?
Emily, there are several case studies and real-world examples showcasing the effectiveness of ChatGPT in defect analysis. I can provide you with some references and specific examples to explore further if you're interested.
How is the feedback loop with ChatGPT managed? Can it learn from user input and improve its analysis over time?
Joan, ChatGPT has a feedback loop mechanism. User input, corrections, and feedback are utilized for fine-tuning models and improving the system's analysis capabilities over time. This iterative learning process helps enhance accuracy and adaptability.
How can organizations ensure proper training and knowledge transfer to effectively use ChatGPT for defect root cause analysis?
Sophia, organizations should invest in comprehensive training programs to familiarize their teams with ChatGPT's capabilities, limitations, and best practices for defect analysis. Knowledge transfer and sharing experiences within the organization can further improve the effective utilization of the tool.
What are the hardware and software requirements for deploying ChatGPT in an organization's infrastructure?
Robert, the hardware and software requirements depend on the specific deployment method and scale. ChatGPT can be hosted on cloud servers or integrated within an organization's existing infrastructure. The resource requirements should align with the expected workload and user interactions.
Are there any ethical considerations organizations should keep in mind when using ChatGPT for defect root cause analysis?
Sarah, ethical considerations are crucial. Organizations should ensure the responsible use of AI tools like ChatGPT, respecting data privacy, transparency, and fairness. Establishing clear guidelines for human-AI collaboration and maintaining accountability can help address ethical concerns.
Can ChatGPT be customized to fit the specific needs and requirements of different industries?
Thomas, absolutely! ChatGPT's adaptability allows for customization based on specific industry requirements. It can be trained on relevant datasets in different domains, making it a versatile tool for defect root cause analysis across industries.
What kind of user interface does ChatGPT offer for defect root cause analysis?
Olivia, ChatGPT can provide a user-friendly interface, allowing users to interact through a chat-like system. It can accept input, provide analysis suggestions, and generate insightful responses to support defect root cause analysis activities.
Does ChatGPT have multilingual capabilities? Can it support defect analysis in languages other than English?
Richard, ChatGPT has the potential for multilingual support. By training it on diverse datasets in different languages, it can extend its defect analysis capabilities beyond just English, enabling analysis in various languages based on the training it has received.
What are some potential challenges or risks organizations should be aware of when adopting ChatGPT for defect root cause analysis?
Christine, organizations should consider challenges such as potential biases in training data, user reliance on ChatGPT without critical thinking, and the need for continuous model updates as defect scenarios evolve. Strengthening the human-AI collaboration and maintaining a balanced approach can mitigate such risks.
How does ChatGPT handle uncertainty or cases where it cannot determine a clear root cause?
Alexa, ChatGPT can indicate uncertainty when it cannot determine a clear root cause. The system can provide alternative hypotheses and suggest further investigation or expert intervention to handle such cases responsibly.
It sounds like ChatGPT has significant potential. What further developments or advancements can we expect in the future for defect root cause analysis?
Patrick, the future holds exciting possibilities. We can expect further advancements in natural language processing, model interpretability, and deeper integrations with other defect analysis tools. Continuous research and development aim to enhance ChatGPT's analysis capabilities and address emerging challenges.