Streamlining Efficiency: Leveraging Gemini in Technology Deduplication
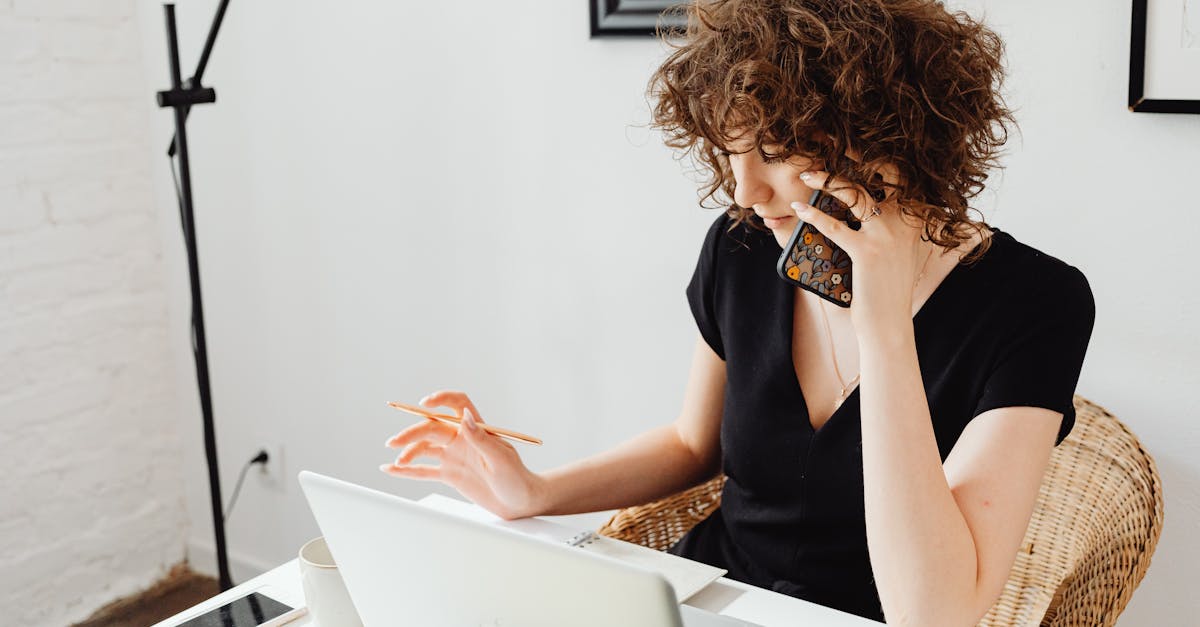
In today's rapidly evolving technological landscape, companies are constantly faced with the challenge of managing and organizing vast amounts of data. One particular issue that often arises is the identification and elimination of duplicate entries in technology databases. This is where leveraging the power of Gemini, an advanced language model developed by Google, can greatly streamline the deduplication process and enhance overall efficiency.
The Power of Gemini
Gemini is built upon the powerful LLM model and is designed to engage in natural language conversations with users. It has been trained on an extensive dataset, making it capable of understanding and generating human-like text based on given prompts.
When it comes to deduplicating technology databases, Gemini can be a valuable tool. It can effectively identify duplicate entries by comparing textual descriptions, product names, or any relevant information within the database. By analyzing the data and using its machine learning capabilities, Gemini can detect subtle differences and similarities that humans might easily miss.
Areas of Application
The application of Gemini in technology deduplication is vast. Here are some key areas where it can be applied:
Product Catalogs
E-commerce platforms often have vast catalogs of products, and the challenge of maintaining uniqueness in product listings is significant. Gemini can help identify duplicate entries within these catalogs by examining various attributes such as product descriptions, specifications, and images.
Software Repositories
For companies managing extensive repositories of software applications, avoiding duplicate entries becomes crucial. Gemini can compare software descriptions, functionality, and even code snippets to efficiently identify duplicates and prevent redundancy.
Research Databases
In research institutions or academic settings where large databases of scholarly articles and publications exist, deduplicating entries becomes critical. Gemini can be employed to analyze abstracts, titles, authors, or even full texts to ensure the integrity of the database.
Streamlining Efficiency
By leveraging Gemini in technology deduplication, companies can achieve significant efficiency gains. Manual deduplication processes can be time-consuming, error-prone, and subject to human bias. Gemini, on the other hand, can automate the process, greatly reducing the time and effort required.
Additionally, Gemini can adapt and learn from new data, continuously improving its deduplication capabilities over time. This ensures a more accurate and effective deduplication process, minimizing the risk of false positives or false negatives.
Conclusion
As technology continues to advance, the management and organization of data become increasingly complex. Leveraging innovative technologies like Gemini can provide a solution to one of the key challenges faced by companies – technology deduplication. Its ability to analyze and compare textual data in a vast database is a game-changer, streamlining efficiency and improving overall data quality.
With Gemini, companies can confidently navigate the intricacies of technology deduplication, saving valuable time, resources, and ensuring accurate decision-making based on reliable data.
Comments:
Great article, Samir! I found your insights on leveraging Gemini in technology deduplication very interesting. It's amazing how AI can streamline efficiency in such complex processes.
I agree, Sarah! The potential of Gemini in technology deduplication is immense. It can save so much time and effort by automating the process. Do you think it's reliable enough for production environments?
Good point, Michael! While Gemini has shown promising results, I believe a thorough evaluation and testing would be necessary before implementing it in production. We need to ensure its accuracy and reliability.
I enjoyed reading your article, Samir! It's fascinating how natural language processing techniques like Gemini can be applied to technology deduplication. Are there any specific challenges you faced during the implementation?
Thank you, Emily! One of the main challenges we encountered was the need to ensure that Gemini can handle the technical jargon and specific domain knowledge required for technology deduplication. We had to fine-tune the model and provide it with relevant training data.
Samir, your article is enlightening! It's impressive how Gemini can assist in technology deduplication. However, what are the potential limitations or risks of relying solely on AI for this process?
Thank you, Peter! While AI can greatly enhance efficiency, it's important to acknowledge its limitations. One potential risk is the possibility of false positives or false negatives in the deduplication process. Human oversight and validation are still crucial to ensure the accuracy of results.
Interesting article, Samir! I can see how leveraging Gemini can make technology deduplication more efficient. Have you compared its performance against other existing deduplication methods?
Thank you, Rachel! Yes, during our evaluation, we compared Gemini's performance against traditional rule-based methods and other machine learning approaches. Gemini showcased competitive results, especially in scenarios where the deduplication rules required flexibility.
Great read, Samir! I'm curious about the scalability of using Gemini for technology deduplication. Can it handle large datasets efficiently?
Thank you, David! Gemini's scalability depends on various factors, such as the available computational resources and the size of the dataset. With adequate resources, it can be trained on large datasets and handle the deduplication process efficiently.
Samir, your article sheds light on an exciting application of AI in technology deduplication. How do you foresee the future advancements in this field?
Thank you, Lucy! The future of technology deduplication lies in the continuous improvement and evolution of AI models like Gemini. As natural language processing techniques advance, we can expect even more accurate and efficient deduplication processes.
Great article, Samir! I can imagine the tremendous impact Gemini can have in reducing duplicate technology records. Do you think it can be adapted to other domains as well?
Thank you, Adam! Absolutely, Gemini can be adapted to other domains with similar deduplication challenges. By providing it with appropriate training data and fine-tuning, the model can be utilized in various applications beyond technology deduplication.
Samir, your article brings attention to the potential of AI in technology deduplication. How would you address concerns about privacy when utilizing such AI models?
Thank you, Natalie! Privacy concerns are vital when leveraging AI models. In our implementation, we took steps to ensure compliance with data protection regulations and implemented privacy-preserving techniques. It's essential to strike a balance between utilizing AI's capabilities and safeguarding user privacy.
Great job, Samir! I'm curious to know if the model's performance varies when dealing with different types of technology deduplication scenarios.
Thank you, Oliver! The model's performance can vary based on different deduplication scenarios, data quality, and the complexity of the deduplication rules. Fine-tuning and dataset preparation play crucial roles in ensuring optimal performance across diverse scenarios.
Samir, your article highlights the potential of Gemini in technology deduplication. Have you encountered any limitations or challenges specific to implementing Gemini in this context?
Thank you, Isabella! One challenge we faced was the model's tendency to generate incorrect or nonsensical suggestions. We addressed this through rigorous fine-tuning and carefully crafting the training data to ensure accurate and meaningful deduplication suggestions.
Samir, I enjoyed reading your article. How do you see the adoption of AI models like Gemini impacting the future of technology management?
Thank you, Jason! The adoption of AI models like Gemini has the potential to revolutionize technology management. By streamlining processes like deduplication, it frees up valuable time for IT professionals, enabling them to focus on more strategic and high-value tasks.
Impressive work, Samir! What are the key steps involved in implementing Gemini for technology deduplication?
Thank you, Sophia! The key steps in implementing Gemini for technology deduplication involve data preparation, fine-tuning the model using appropriate techniques, validating the results, and integrating it into the existing deduplication workflow. Continuous monitoring and refinement are also crucial.
Samir, your article is thought-provoking! How do you see Gemini and similar AI models evolving to address more complex deduplication scenarios in the future?
Thank you, Ryan! As AI models evolve, we can expect them to advance in understanding and handling more complex deduplication scenarios. Their capabilities could be enhanced by incorporating additional contextual information and leveraging multi-modal learning techniques.
Interesting article, Samir! I'm curious about the training process of Gemini for technology deduplication. Can you share any insights?
Thank you, Liam! The training process involved providing Gemini with a dataset of duplicate and non-duplicate technology records. We fine-tuned the model using supervised learning, leveraging techniques like focal loss and data augmentation. The model learned to generate deduplication suggestions based on the input records.
Samir, your article showcases the potential of AI in deduplicating technology records. How do you ensure the model's suggestions align with the preferences of the users?
Thank you, Hannah! To align the model's suggestions with user preferences, we incorporated user feedback loops during the training process. The feedback helped refine the model's understanding of what constitutes a good deduplication suggestion, leading to improved alignment with user preferences.
Great insights, Samir! Have you explored using Gemini in conjunction with other AI models or techniques for technology deduplication?
Thank you, Ethan! While our focus was primarily on Gemini, incorporating other AI models or techniques, such as entity recognition or keyword extraction, can potentially enhance the overall deduplication process. It's an avenue worth exploring.
Samir, your article provides valuable insights into the role of AI in technology deduplication. How do you envision the collaboration between AI systems and human experts in this domain?
Thank you, Chloe! Collaboration between AI systems and human experts is crucial for technology deduplication. AI can automate and accelerate the process while human experts provide oversight, domain knowledge, and ensure the accuracy of deduplication decisions. It's a symbiotic relationship.
Impressive article, Samir! Do you have any insights into how Gemini can handle deduplication scenarios involving unstructured or semi-structured data?
Thank you, Emma! Gemini can handle deduplication scenarios involving unstructured or semi-structured data to some extent. However, the accuracy and performance may vary depending on the complexity and quality of the data. Fine-tuning and experimenting with different techniques can help improve results.
Great work, Samir! How do you measure the effectiveness of Gemini in technology deduplication?
Thank you, Grace! We measured the effectiveness of Gemini by comparing its deduplication suggestions against manually validated ground truth. Metrics like precision, recall, and F1 score were used to evaluate its accuracy and overall performance. Continuous monitoring and evaluation are necessary for maintaining effectiveness.
Samir, your article unveils an interesting application of AI in technology deduplication. Is Gemini capable of learning from user feedback during the deduplication process?
Thank you, Jack! Gemini's learning capabilities can be enhanced by incorporating user feedback during the deduplication process. This iterative feedback loop helps improve the model's suggestions and align them with the user's preferences, leading to more accurate deduplication results.
Fascinating article, Samir! How does the performance of Gemini compare when dealing with non-English or multilingual technology records?
Thank you, Mia! Gemini's performance in deduplicating non-English or multilingual technology records heavily relies on the availability of appropriate training data and fine-tuning in those languages. With sufficient training and resources, it can be adapted to handle diverse language scenarios.
Well-written article, Samir! How do you handle cases where the model suggests incorrect deduplication results?
Thank you, Evelyn! When the model suggests incorrect deduplication results, it's important to have mechanisms in place to capture user feedback and enable manual verification. This feedback loop helps identify and rectify any inaccuracies, continuously improving the model's performance over time.
Samir, your article highlights the potential of AI in technology deduplication. Are there any ethical considerations or potential biases to be aware of when using Gemini in this context?
Thank you, Aaron! Ethical considerations and biases are essential when using AI models like Gemini. We closely monitored the training data, implemented bias mitigation techniques, and ensured fairness and transparency in the deduplication process. Regular audits and ongoing efforts to improve fairness are necessary.
Samir, your article provides valuable insights into the potential of AI in technology deduplication. How do you see the future collaboration between AI models and human experts evolving in this field?
Thank you, Madeline! The future collaboration between AI models and human experts in technology deduplication will likely involve increasing synergy and trust. AI models will take on more routine tasks, allowing human experts to focus on complex decision-making and ensuring the overall accuracy and quality of the deduplication process.
Great insights, Samir! How do you handle cases where Gemini doesn't provide a clear recommendation for deduplication?
Thank you, Gabriel! In cases where Gemini doesn't provide a clear recommendation, we designed our system to prompt the user for additional input or redirect the scenario to human experts. It's crucial to have mechanisms in place to handle such cases and ensure accurate deduplication decisions.
Thank you all for taking the time to read my article on 'Streamlining Efficiency: Leveraging Gemini in Technology Deduplication'. I'm excited to hear your thoughts and insights!
Great article, Samir! I found the concept of leveraging Gemini in technology deduplication fascinating. It seems like it has the potential to significantly improve efficiency and accuracy in the field. Have you personally used Gemini for this purpose?
Thank you, Anna! Yes, I have used Gemini in technology deduplication projects, and it has proven to be quite effective. The model's ability to understand context and generate relevant responses makes it a valuable tool in identifying duplicate technologies.
Interesting read, Samir. I can see how leveraging Gemini could streamline the deduplication process. It would be helpful to know more about the specific challenges or limitations you encountered while implementing this approach.
Thank you, Michael! While Gemini is powerful, it does have limitations. One challenge is the risk of generating incorrect responses due to context misunderstanding. It requires careful fine-tuning and monitoring to minimize such errors. Additionally, the model's lack of awareness of external datasets can sometimes limit its ability to identify less common technologies.
Samir, your article provides valuable insights into leveraging language models like Gemini for deduplication. I wonder how this approach compares to traditional rule-based deduplication algorithms in terms of accuracy and efficiency.
Thank you, Emily! Traditional rule-based deduplication algorithms have been effective, but they often require manual rule creation and may not adapt well to evolving data. Gemini can offer higher accuracy and efficiency by automatically learning patterns from data. However, it still requires human oversight to ensure quality and minimize false positives/negatives.
An important aspect to consider is the computational resources required when leveraging language models like Gemini. Did you face any challenges in terms of model size or computational limitations?
That's a valid point, David. Gemini can be resource-intensive, especially when using larger models or processing large datasets. It's crucial to strike a balance between model size and available computational resources to ensure efficient utilization. In some cases, model optimization techniques or distributed computing can help overcome these challenges.
Samir, I'm curious about the sources of data that Gemini relies on for technology deduplication. How does it handle identifying technologies that are not widely known?
Great question, Sophia! Gemini relies on a large corpus of text data, including online sources, to learn patterns and understand technologies. However, its knowledge is limited to what it has been trained on, so identifying lesser-known technologies can be challenging. This is where human expertise and curation of external datasets become crucial to enhance the model's effectiveness.
Samir, I appreciate your article on leveraging Gemini in technology deduplication. One concern I have is the potential bias in the model's responses. How do you ensure fairness and mitigate biases in the deduplication process?
Thank you, Karen! Addressing bias is an important consideration when using models like Gemini. Training data should be carefully curated to avoid biases, and the model's outputs should be regularly audited and evaluated for fairness. By involving diverse human perspectives and continuously improving the training data, we can strive to make the deduplication process as fair and unbiased as possible.
Samir, your article highlights the potential of Gemini in technology deduplication. Are there any specific industries or domains where you see this approach being particularly beneficial?
Good question, Daniel! Gemini can be valuable in various industries where deduplication is necessary, such as technology research, patent analysis, and software development. Its versatility allows it to adapt to different domains and datasets, making it a promising tool in many sectors.
Samir, I enjoyed reading your article. Do you foresee any potential ethical concerns or risks associated with using language models like Gemini for technology deduplication?
Thank you, Julia! Ethical concerns do arise when using language models. It's essential to handle sensitive data with care, respect privacy, and be transparent about the technology's limitations and potential biases. By following ethical guidelines and frameworks, we can navigate the risks and ensure responsible usage of such models.
Samir, your article has shed light on an exciting application of language models. Have you considered incorporating other types of AI technologies, like computer vision, to enhance the deduplication process?
Great point, Hannah! Combining AI technologies like computer vision with language models can potentially boost the deduplication process. Visual analysis can help identify duplicate technologies based on visual attributes, complementing the language-based matching approach. This interdisciplinary integration holds promise for more comprehensive and accurate deduplication.
Samir, your article has provided valuable insights into leveraging Gemini for deduplication. What are the potential future advancements or research directions in this field?
Thank you, Ryan! The field of technology deduplication is constantly evolving. Future advancements could focus on improving model interpretability, reducing biases, and developing specialized domain-specific versions of language models. Additionally, incorporating user feedback and building knowledge graphs for sophisticated matching can expand the capabilities of deduplication systems.
Samir, your article provides valuable insights into the role of Gemini in technology deduplication. What advice would you give to organizations considering implementing this approach?
Thank you, Oliver! For organizations considering implementing Gemini in deduplication, I would advise starting with smaller-scale projects to understand the model's impact and challenges. Ensuring data quality, ongoing monitoring, and incorporating human expertise are key factors to maximize the effectiveness of the approach.
Samir, your article on Gemini in technology deduplication is insightful. How do you handle the trade-off between automation and human involvement in the deduplication process?
Thank you, Grace! The trade-off between automation and human involvement is crucial. While automation reduces manual effort and speeds up the process, human oversight is necessary to ensure accuracy and quality. Striking a balance by leveraging the model's efficiency while involving humans in critical decisions can lead to optimal and trustworthy deduplication outcomes.
Samir, your article offers an interesting perspective on leveraging Gemini for deduplication. How do you handle the potential risks of relying heavily on machine learning algorithms in this process?
Thank you, Peter! Relying heavily on machine learning algorithms does pose risks. It is important to be aware of the limitations of the algorithms and to regularly evaluate their performance. Implementing human-in-the-loop systems and continuously refining the training data can help mitigate risks and ensure a reliable and accurate deduplication process.
Samir, your article has highlighted the potential benefits of leveraging Gemini in technology deduplication. How do you handle cases where similar technologies have different names?
Good question, Isabella! Handling cases where similar technologies have different names can be challenging. Gemini can rely on context and additional information associated with the technologies to identify similarities, even if the names are different. However, human expertise is crucial to account for semantic differences and ensure accurate deduplication.
Samir, your article sheds light on an interesting application of Gemini. How do you measure the success of the deduplication process and assess the model's performance?
Thank you, Jonathan! Measuring the success of the deduplication process involves evaluating metrics like precision, recall, and F1 score. Human evaluation and comparison of the model's outputs against ground truth instances also help assess its performance. Continuous monitoring and feedback loops allow for systematic improvements to enhance the model's capabilities.
Samir, I really enjoyed reading your article. Considering the rapid evolution of language models, how do you keep up with the latest advancements and ensure your deduplication approach remains state-of-the-art?
Thank you, Jessica! Keeping up with the latest advancements in language models is a continuous effort. Actively participating in research communities, attending conferences, and collaborating with experts in the field help me stay updated. Regular experimentation and exploration of new pre-training techniques also allow me to refine and enhance the deduplication approach.
Samir, your article provides valuable insights into Gemini's role in technology deduplication. How do you handle cases where there is limited data available for certain technologies?
Thank you, Lucas! Limited data availability for certain technologies can be a challenge. In such cases, transferring knowledge from related technologies and leveraging external datasets can partially compensate for the lack of specific data. It requires carefully designing and implementing techniques to extrapolate information and make informed deduplication decisions.
Samir, your article on using Gemini for deduplication is informative. What are the main factors to consider when fine-tuning the model for this specific task?
Thank you, Lily! Fine-tuning the model for deduplication involves several key factors. Data quality and diversity play a crucial role, as do the choice of hyperparameters and careful crafting of the training objective to align with deduplication goals. Iterative experimentation and fine-tuning based on evaluation metrics help optimize the model's performance.
Samir, your article demonstrates the potential of Gemini in technology deduplication. How do you handle cases where duplicate technologies have different variations or versions?
Good question, Amy! Cases where duplicate technologies have different variations or versions require a detailed understanding of the technology landscape. Gemini can leverage contextual information and additional attributes to identify similarities across versions. However, human expertise is crucial to ensure accurate deduplication, especially when distinguishing major variations with distinct functionalities.
Samir, I appreciate the insights provided in your article. How do you handle cases where the duplicates are not exact matches but have slight differences?
Thank you, Nathan! Handling cases where duplicates have slight differences requires a nuanced approach. Gemini can use context and other attributes to identify similarities, even if the duplicates are not exact matches. However, human involvement is crucial to assess the significance of the differences and determine whether they stem from actual variations or other factors.
Samir, your article brings attention to an interesting use case for Gemini. Should organizations solely rely on Gemini for technology deduplication, or is it more effective as a tool to aid human experts in the process?
Good question, Sophie! While Gemini is a powerful tool, organizations should not solely rely on it for technology deduplication. Human experts bring domain knowledge and judgment, ensuring accurate and nuanced decisions. Gemini can aid experts by automating part of the process, reducing manual effort, and offering valuable suggestions, but it should be used in conjunction with human expertise.
Samir, your article sheds light on leveraging Gemini in technology deduplication. How do you address cases where the model lacks context or fails to recognize important information about a technology?
Thank you, Julian! Gemini's limitations in recognizing important information or lacking context can be addressed through techniques like prompt engineering and incorporating additional training examples. Fine-tuning the model using carefully designed prompts and rewarding desirable behavior helps enhance its understanding and recognition of important technology-related details.
Samir, I enjoyed reading your article on leveraging Gemini for deduplication. How do you overcome the challenge of generating machine-generated responses that seem plausible but are actually incorrect?
Thank you, Olivia! Overcoming the challenge of generating plausible yet incorrect responses requires ongoing monitoring and human review. Establishing feedback loops and incorporating human oversight help identify and correct such erroneous outputs. Careful model tuning, regular evaluation, and engaging domain experts can mitigate potential risks of incorrect responses.
Samir, your article provides valuable insights into the role of Gemini in deduplication. How do you handle cases where the model generates duplicate responses for different queries?
Thank you, Dominic! Handling cases where the model generates duplicate responses for different queries is important to avoid redundancy and improve efficiency. Techniques like response caching, deduplication of generated outputs, and incorporating query-specific signals during fine-tuning can help prevent such issues. Regular monitoring and iteration further ensure optimization of the model's responsiveness.
Samir, your article sheds light on using Gemini for deduplication. How do you handle cases where there are no duplications but the model incorrectly identifies similarities?
Thank you, Connor! Avoiding false positives where the model incorrectly identifies similarities is crucial. Human validation and fine-tuning the model using negative examples can reduce such errors. By involving domain experts and continuously refining the model's understanding, we can minimize false positives and improve the accuracy of the deduplication process.