Streamlining Material Selection in Design for Manufacturing Technology: Harnessing the Power of ChatGPT
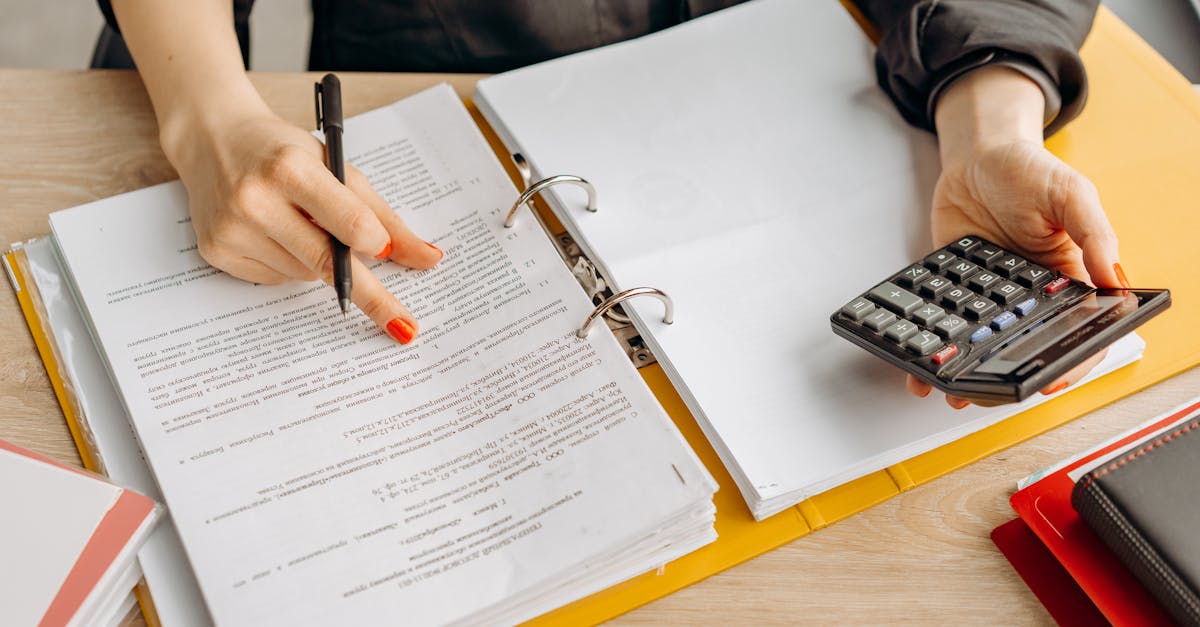
In the world of manufacturing, choosing the right materials for the production process is crucial. Material selection plays a significant role in determining the success of a design, as it directly impacts the product's functionality, durability, and overall performance. Design for Manufacturing (DFM) focuses on optimizing the manufacturing process, and one aspect of DFM is material selection.
The Importance of Material Selection
Material selection is a critical decision that engineers and designers have to make early in the product development process. The choice of materials can affect various factors, such as cost, product quality, manufacturing efficiency, and environmental impact. It is essential to consider the properties of different materials and match them to the specific design requirements.
Challenges in Material Selection
Identifying the most suitable material for a design can be a complex task. There are numerous factors to consider, including mechanical properties, thermal properties, chemical resistance, electrical conductivity, and more. Additionally, it is vital to assess factors like availability, cost, and environmental sustainability.
DFM aids can provide valuable assistance in the material selection process. By incorporating design constraints into the aid, it offers suggestions for materials that align with the specific parameters of the manufacturing process and product design.
Benefits of DFM in Material Selection
Integrating DFM technology into material selection offers several benefits:
- Streamlined Process: DFM tools help streamline the material selection process by providing a database of materials and their properties. This allows designers to explore various options quickly and efficiently.
- Improved Product Quality: Proper material selection based on design constraints ensures that the final product meets the desired quality standards. By considering factors like strength, stiffness, and corrosion resistance, the DFM tool helps in choosing the right material for the job.
- Cost Optimization: DFM tools take into account material costs and availability, allowing designers to choose cost-effective materials without compromising on product performance.
- Efficient Manufacturing: By selecting materials that are compatible with the manufacturing process, the DFM tool can help minimize production issues, reduce waste, and improve overall manufacturing efficiency.
- Environmental Sustainability: DFM tools also consider the environmental impact of different materials, enabling designers to select materials that are more sustainable and eco-friendly.
Conclusion
Design for Manufacturing offers valuable guidance in the material selection process for manufacturing. By incorporating design constraints into the aid, it assists designers and engineers in making informed decisions that optimize product quality, manufacturing efficiency, and cost-effectiveness. Material selection is a crucial step in the manufacturing process, and with the help of DFM technology, designers can choose the most suitable materials to bring their designs to life.
Comments:
Thank you all for reading my article on streamlining material selection in design for manufacturing technology! I hope you found it insightful.
Great article, Sam! Material selection can indeed be a complex process. ChatGPT sounds promising for assisting in this area.
I agree, Emily. The ability to leverage AI-powered technologies like ChatGPT can definitely streamline the material selection phase, saving time and resources.
Absolutely, Michael. The efficiency improvements brought by technologies like ChatGPT can give designers more time to focus on other critical aspects of the manufacturing process.
I've been working in the design for manufacturing field for years, and this article resonates with my experiences. Looking forward to exploring the potential of ChatGPT.
As an engineer, I'm always looking for ways to improve the design process. Exciting to see AI stepping in to assist with material selection.
Indeed, Alex. The combination of human expertise and AI capabilities can lead to more informed decisions when it comes to choosing the right materials.
Sam, thanks for shedding light on the potential of AI in material selection. Are there any specific use cases you've explored where ChatGPT has made a significant impact?
Thanks for the question, Oliver. ChatGPT has proven to be particularly useful in scenarios where designers are looking to optimize material properties for specific performance requirements.
For instance, it can help identify materials that offer a balance between strength and flexibility, or thermal conductivity and weight. By generating suggestions, it accelerates the decision-making process.
Sam, how does ChatGPT handle the vast amount of material data for analysis? Is it capable of processing and offering insights on all available materials in the market?
That's a great point, Nathan. ChatGPT leverages large material databases and can analyze a wide range of materials, including metals, polymers, and composites. It provides insights based on existing data.
However, it's important to note that the quality and accuracy of data can impact the reliability of suggestions. So, while ChatGPT can handle a large number of materials, ensuring data accuracy is crucial.
Sam, I appreciate your article focusing on the practical benefits of ChatGPT. Can you share any user feedback or success stories from implementing this technology in material selection processes?
Certainly, Lisa! We've received positive feedback from users who have stated that ChatGPT led them to consider materials they hadn't previously explored, resulting in improved product performance.
One user reported that ChatGPT suggested a novel composite material that met their project requirements, leading to a significant reduction in weight without compromising strength.
It's important to note that while ChatGPT can provide valuable insights, it should be used as a tool alongside domain expertise. Human judgment is still crucial in final material selection.
Sam, what are the challenges or limitations of using ChatGPT for material selection? Are there specific scenarios where it may not be as effective?
Good question, David. ChatGPT relies on large amounts of data to generate suggestions, so if there's limited existing data on certain materials or specific performance parameters, its effectiveness might be reduced.
Additionally, ChatGPT may overlook unique material properties or manufacturing constraints that require human expertise to consider. It's important to strike the right balance between AI and human input.
Sam, thank you for emphasizing the collaboration between AI and human experts. What steps can designers take to integrate ChatGPT into their existing material selection workflows?
You're welcome, Sophia. Integrating ChatGPT into existing workflows involves training the model on relevant material data and performance requirements so that it can offer more tailored suggestions.
Designers should also validate the AI-driven recommendations through physical testing or simulations, which can further refine the material selection process.
Ultimately, the goal is to see ChatGPT as a supporting tool that complements human expertise, rather than a standalone decision-maker.
I've been curious about the potential impact of AI in the manufacturing industry. Sam, what do you think the future holds for AI-driven material selection?
Great question, Megan. I believe AI-driven material selection will continue to evolve and become more sophisticated. With advancements in machine learning, models like ChatGPT can offer increasingly accurate suggestions.
We might see AI systems integrating real-time material performance feedback from sensors in the future, allowing for adaptive material selection based on dynamic conditions.
Ultimately, the goal is to optimize material selection to such a degree that it enables the creation of innovative, high-performance products and reduces manufacturing costs.
Sam, I'm curious about the limitations of ChatGPT in understanding complex design constraints. For instance, how would it handle conflicting requirements or intricate geometries?
That's a valid concern, Emily. ChatGPT's ability to handle such constraints is limited, especially when it comes to intricate geometries or conflicting performance requirements.
In these cases, it's essential to involve human experts who can consider various trade-offs and make informed decisions that go beyond the scope of AI-driven suggestions.
However, ChatGPT can still narrow down the range of potential materials and prompt designers to explore alternative options that may have been overlooked.
Sam, how accessible is ChatGPT to designers who may not have extensive AI expertise? Is it relatively user-friendly and easy to implement?
Good question, Michael. The developers behind ChatGPT have focused on making it user-friendly and accessible to a wide range of users, including those without extensive AI expertise.
The implementation process involves training the model on relevant data and performance requirements, which might require some technical expertise. However, user-friendly interfaces are being developed to simplify the process.
Sam, I'm curious about the ethical considerations surrounding AI-driven material selection. How can we ensure unbiased recommendations and prevent unintended consequences?
Ethical considerations are crucial, Emma. Bias and unintended consequences can arise from the data used to train AI models. Careful curation of the training data and continuous monitoring/testing can help reduce biases.
Transparency is also essential. Users must have visibility into the decision-making process of AI systems to understand and verify the recommendations it provides.
Additionally, collaboration between interdisciplinary teams and involving diverse perspectives can help identify and address potential biases or unintended consequences.
Sam, I appreciate the discussion around AI in material selection. It's an exciting field with immense potential. Can't wait to see how it evolves further!
Thank you for your kind words, Jennifer. I share your excitement and belief in the future of AI-driven material selection. It was a pleasure to have this discussion.
Indeed, Sam. Your insights have provided valuable perspectives on the topic. Looking forward to more articles from you in the future.
I truly appreciate the feedback, David. I'll definitely keep exploring and sharing insights in this rapidly evolving field. Thank you for engaging in this conversation.
Sam, thank you for taking the time to address our questions and engaging in this discussion. It has been enlightening.
You're very welcome, Sophia. I'm glad you found the discussion enlightening. Thank you for your active participation and thought-provoking questions.
Thank you, Sam, for sharing your expertise and insights. It's been a valuable discussion, and I look forward to future developments in this area.
Thank you, Nathan. I'm delighted that you found the discussion valuable. Exciting times lie ahead, indeed.
Thank you, Sam. Your article and this discussion have provided valuable insights into the potential of AI in material selection. Best of luck with your future endeavors!
Thank you so much, Sarah! Your kind words mean a lot. Best of luck to you as well. Keep exploring the possibilities in this field!
Thank you, Sam, for sharing your knowledge and thoughts with us. This discussion has given me a lot to think about. Excited to see where AI takes us in material selection.
You're most welcome, Megan. I'm thrilled that you found the discussion thought-provoking. The future of AI in material selection holds immense potential. Let's stay curious and explore together!