Streamlining Pressure Technology Processes: Leveraging ChatGPT for Optimization
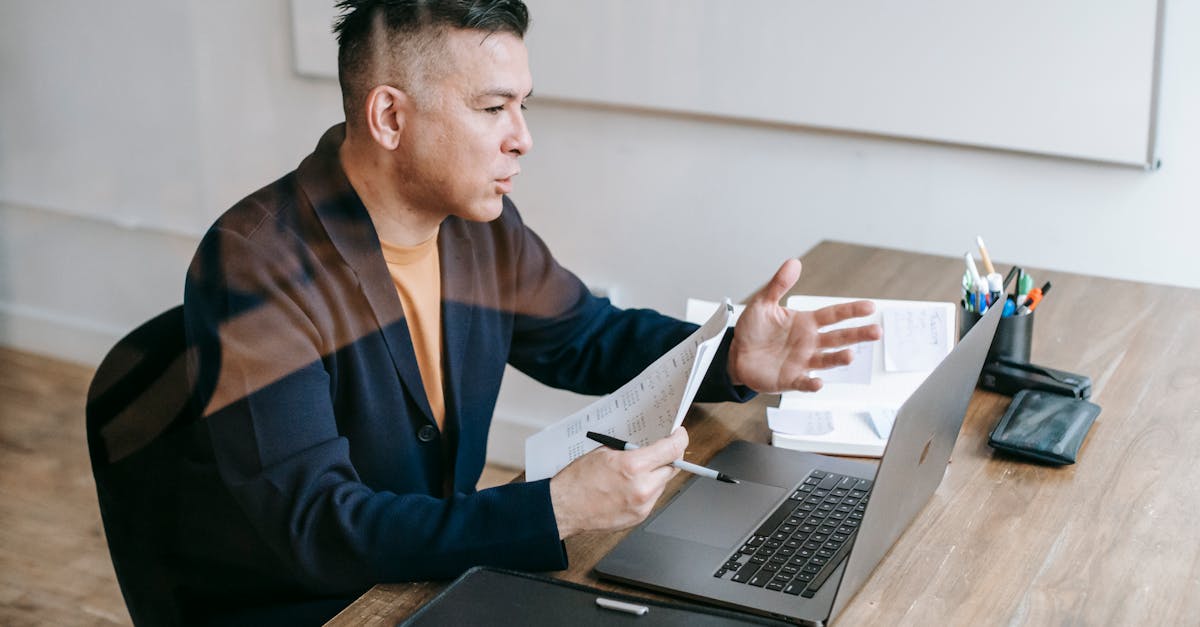
Pressure is a fundamental concept in numerous industries, playing a significant role in process optimization. Whether it is manufacturing, chemical processing, or oil and gas production, understanding and analyzing pressure system data and process parameters can lead to identifying opportunities for optimization. One such application of pressure analysis is in the powerful AI model, ChatGPT-4.
What is ChatGPT-4?
ChatGPT-4 is an advanced AI model developed by OpenAI. It is designed to generate human-like text responses and engage in dynamic conversations. This AI model utilizes natural language processing algorithms, machine learning techniques, and extensive training on vast amounts of data to understand and generate human-like responses.
Optimizing Industrial Processes with ChatGPT-4
Traditionally, process optimization in industries involved human expertise, data analysis, and complex mathematical models. However, with the capabilities of ChatGPT-4, the optimization process can be greatly enhanced. By analyzing pressure system data and process parameters, ChatGPT-4 can efficiently identify areas of improvement within industrial processes.
Reducing Waste
Waste reduction is crucial for industries striving for increased efficiency and sustainability. Excessive pressure in a system can lead to inefficiencies, leakage, and unnecessary energy consumption. ChatGPT-4 can analyze pressure data obtained from monitoring devices and identify opportunities to reduce waste by fine-tuning pressure settings. By doing so, industries can minimize material loss, energy consumption, and their impact on the environment.
Improving Efficiency
Efficiency is a key metric in industrial process optimization. By evaluating pressure system data, ChatGPT-4 can identify inefficiencies within the production process. It can suggest adjustments to process parameters, such as optimal pressure levels, to enhance overall efficiency. This can lead to improved productivity, reduced downtimes, and cost savings.
The Role of Pressure Analysis
Pressure analysis plays a crucial role in the optimization process facilitated by ChatGPT-4. The AI model relies on accurate and real-time pressure data to provide intelligent recommendations. By monitoring pressure systems and capturing data points, industries can create a comprehensive dataset for ChatGPT-4 to analyze and learn from.
Conclusion
Pressure analysis is a vital component of process optimization, and ChatGPT-4 brings a new level of efficiency and intelligence to this field. By leveraging the capabilities of this advanced AI model, industries can harness pressure system data and process parameters to identify opportunities for improvement, ultimately leading to reduced waste, improved efficiency, and increased productivity.
Comments:
Great article, Hank! I found the use of ChatGPT for optimizing pressure technology processes fascinating.
Thank you, Sarah! AI technology has indeed opened up new possibilities for optimization.
Indeed, it's impressive how AI can be leveraged to improve efficiency in various industries.
I have a question regarding the implementation of ChatGPT. How long does it typically take to train the model for a specific process optimization?
Good question, Nicole. The training time can vary depending on the complexity of the process and the available data. It may take several hours or even days for more complex systems.
Thank you for the response, Hank. Considering the time investment, it seems crucial to ensure the training data is accurate and comprehensive.
Exactly, Nicole. Garbage in, garbage out. High-quality, representative training data is key.
The potential of AI in streamlining processes is enormous. It can greatly reduce costs and improve overall productivity.
Absolutely, Michael. The significant impact of AI on cost reduction should not be underestimated.
The article mentions reducing maintenance costs through better process optimization. Are there any specific examples where this has been successfully implemented?
Indeed, Daniel. In one case study, an industrial company optimized their maintenance schedules using ChatGPT and reduced their costs by 20% while maintaining the same level of reliability.
That's impressive, Hank! It highlights the real-world impact of leveraging AI for process optimization.
I'm curious about the limitations of ChatGPT in this context. Are there certain types of processes that it may not be suitable for?
Good question, Olivia. While ChatGPT is powerful, it may not be ideal for highly dynamic processes or those with complex interdependencies. Its effectiveness depends on the quality of available training data.
Thank you, Hank, for clarifying that. It's important to understand the limitations before implementing AI solutions.
Thank you for addressing my question, Hank. Understanding the limitations of AI tools is essential for responsible implementation.
In addition to AI's impact on cost reduction, it also enables predictive maintenance, minimizing downtime and maximizing efficiency.
I'm curious about the integration process. How difficult is it to integrate ChatGPT into existing pressure technology systems?
Integration can vary depending on the existing system, Emily. In some cases, it may require custom software development, data integration, and testing. It's crucial to have a strong understanding of the specific requirements.
Thank you for the response, Hank. It seems that proper planning and collaboration between the development team and end-users are necessary for a successful integration.
AI-driven optimizations can significantly improve overall system performance. It's exciting to see the advancements being made in this field.
What are the potential challenges in implementing AI for process optimization, and how can they be addressed?
One challenge is obtaining sufficient and representative training data. Addressing this requires careful data collection and curation processes. Additionally, proper evaluation and validation of the AI models are crucial.
Has ChatGPT been utilized in real-time optimization scenarios, or is it mainly used for offline analysis and decision-making?
ChatGPT is primarily used for offline analysis and decision-making, Nicole. Real-time optimization typically requires faster response times and specialized models.
How scalable is the use of AI in process optimization? Can it handle large-scale industrial systems effectively?
AI can be scaled to handle large-scale industrial systems, Daniel. However, it requires careful system design, sufficient computational resources, and scalable data management strategies.
It's worth mentioning that AI is not a silver bullet. It requires domain expertise and human oversight to ensure optimal results.
I agree, Robert. AI should be seen as a tool to augment human decision-making rather than replace it.
I'm curious about the potential risks and ethical considerations when implementing AI in process optimization.
One of the risks is overreliance on AI models. Human judgment and expertise are still essential to ensure ethical decision-making and mitigate biases.
I believe effective communication and collaboration between technical teams and process experts is crucial for successful implementation.
Absolutely, Emily. Bringing together experts from both domains can lead to more accurate AI models and better optimization outcomes.
Indeed, collaboration sparks innovation. It allows AI algorithms to be fine-tuned to specific industry needs.
It's exciting to see AI bridging the gap between technology and industry, unlocking new possibilities.
What are some potential future developments in AI-driven process optimization that we can expect to see?
Future developments may involve the integration of machine learning with physical modeling, enabling more accurate predictions and optimization in complex systems.
That sounds exciting, Hank! The combination of physical modeling and AI could unlock new frontiers in process optimization.
I couldn't agree more, Susan. The future of process optimization looks promising with ongoing advancements in AI.
How important is explainability in AI-driven optimization? Can we trust its decisions without understanding the underlying reasoning?
Explainability is crucial, David. Understanding the reasoning behind AI decisions builds trust and allows experts to validate the optimization outcomes.
Thank you for clarifying that, Hank. It's reassuring to know that explainability is considered in AI-driven optimization.
I agree, Hank. Ensuring the data used for training is accurate and representative is crucial for trustworthy optimization.
I appreciate your response, Hank. Transparency and explainability are important aspects of deploying AI systems.
Thank you, Hank. Understanding the rationale behind AI decisions is important for trust and accountability.
Absolutely, David. Trust and accountability are critical when implementing AI in mission-critical applications.
I couldn't agree more, Susan. Trust is essential, especially in scenarios where AI aids decision-making.
Indeed, David. AI is a tool that should be wielded responsibly and in collaboration with human experts.
Are there any known limitations or challenges in deploying AI models in resource-constrained environments?
Resource-constrained environments can pose challenges in terms of the available computational power and data accessibility. However, there are approaches to optimize AI models for such scenarios.
Collaboration also helps identify overlooked process improvements that can be discovered through the combination of domain expertise and AI insights.
Would it be possible to deploy ChatGPT as a real-time advisory system, providing recommendations to operators during process operations?
Real-time deployment of ChatGPT as an advisory system could be challenging due to the response time requirements. However, it's an interesting area for further exploration.
If AI is implemented correctly, it can add tremendous value to industries. However, it's important to address any potential biases that might exist in the training data.
Absolutely, Melissa. Bias mitigation and data ethics play a crucial role in ensuring fairness and avoiding unintended consequences.
Well said, Edward. Ensuring fairness and avoiding biases are vital considerations in AI implementation.
I assume that real-time optimization requires faster model retraining as new data becomes available?
Correct, Nicole. Real-time optimization often involves periodic retraining of AI models to adapt to changing conditions and incorporate new data.
Thank you again for clarifying that, Hank. It's been a insightful discussion on the potential and challenges of AI-driven optimization.
Thank you for answering my question, Hank. It's helpful to understand the real-time capabilities of ChatGPT.
Understood, Hank. Continuous model retraining ensures the optimization system stays relevant and effective.
Thank you for the insight, Hank. I now realize the critical role of both data quality and training time in the effectiveness of ChatGPT.
AI-driven process optimization can revolutionize industries by unlocking hidden potential and driving continuous improvement.
Well said, John. Continuous improvement is a key benefit of AI-driven optimization, enabling industries to stay competitive and adapt to evolving challenges.
Thank you, Hank. I'm excited to witness the ongoing transformation AI brings to various sectors.
Thank you for engaging with our comments, Hank. Your insights have been valuable.
You're welcome, Robert. I appreciate the engaging discussion and the opportunity to share insights.
Thank you for the clarification, Hank. Overcoming resource constraints can be crucial for AI adoption in various settings.
I understand the challenge, Hank. It would be interesting to explore the feasibility of using real-time recommendation systems in process operations.
Indeed, Hank. Identifying and mitigating potential biases should be a top priority when designing AI solutions.
Combining physical modeling and AI sounds like a promising frontier. It could revolutionize how we optimize complex systems.