Supercharging Software Testing Life Cycle: Leveraging ChatGPT for Automated Testing
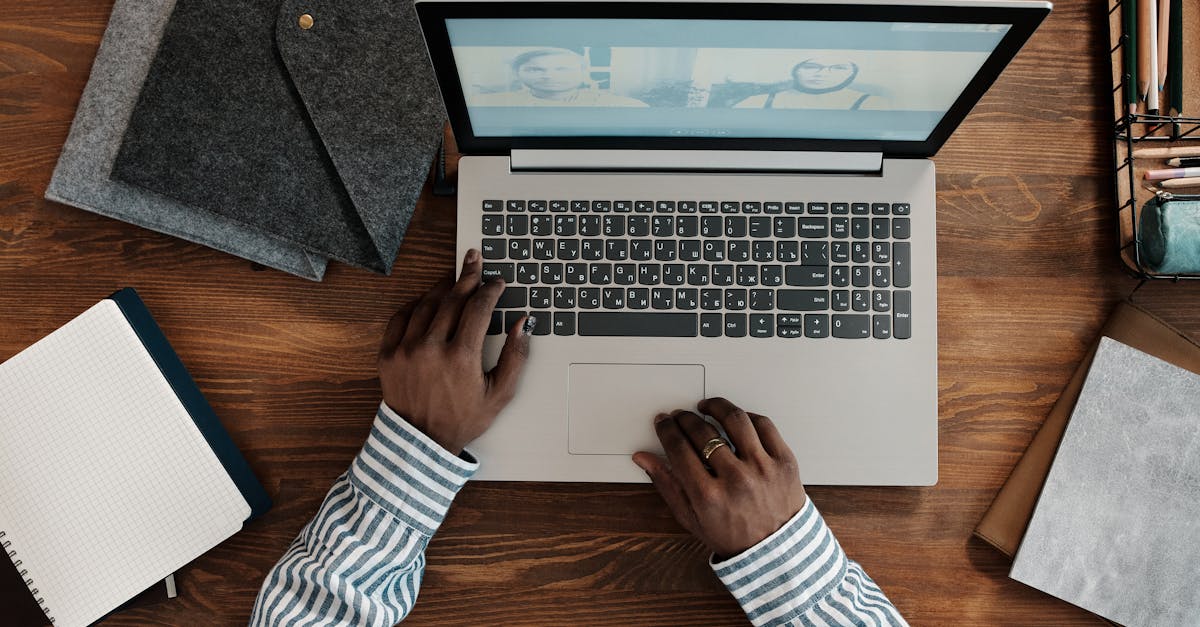
Automated testing plays a crucial role in the Software Testing Life Cycle (STLC) by enabling the execution of comprehensive testing scenarios and scripts with minimal effort. It revolutionizes the testing process by reducing the manual effort involved and ensuring the integrity and reliability of the software being tested.
Understanding the Software Testing Life Cycle
The Software Testing Life Cycle (STLC) is a systematic approach to software testing that ensures the software meets the desired quality standards. It involves planning, designing, executing, and monitoring the testing activities throughout the development process.
Automated testing, as a part of the STLC, focuses on using software tools to automate the execution of test scenarios and scripts. This allows for quicker and more efficient testing, ensuring that all critical scenarios are covered and reducing the potential for human error.
The Importance of Automated Testing
Automated testing has gained popularity in recent years due to the numerous benefits it offers. Here are some key reasons why automated testing is crucial in the software development process:
- Time and Cost Efficiency: Automated testing reduces the overall testing time, as tests can be executed in parallel without requiring human intervention. This leads to cost savings by optimizing resources and reducing the need for manual testing.
- Improved Test Coverage: Automated testing allows for the execution of a large number of test cases, including complex scenarios and edge cases, which may be impractical to perform manually.
- Enhanced Accuracy: Automated testing eliminates the possibility of human errors, such as missing a step or making calculation mistakes, ensuring accurate test results.
- Reliable Regression Testing: Regression testing is essential to ensure that changes or enhancements in the software do not break existing functionality. Automated testing enables efficient and thorough regression testing, ensuring the stability of the software.
Models for Comprehensive Automated Testing
In order to achieve effective automated testing, it is important to follow appropriate testing models. Here are two commonly used models:
- Keyword-Driven Testing: This model focuses on separating the test scripts from the test data and test execution logic. It uses keywords and actions to represent the desired functionality, making it easy to create and maintain test scripts. This model is highly flexible and allows for reusability of test scripts.
- Data-Driven Testing: In this model, test cases are separated from the test data. The test scripts are designed to read test data from external sources, such as spreadsheets or databases. This allows for the testing of multiple data sets without the need for separate test scripts.
Both of these models help to create comprehensive and maintainable automated test suites, ensuring effective test coverage and flexibility in testing scenarios.
Conclusion
Automated testing is an integral part of the Software Testing Life Cycle (STLC) and is crucial for ensuring the quality and reliability of software. It offers numerous benefits such as time and cost efficiency, improved test coverage, enhanced accuracy, and reliable regression testing. By following appropriate testing models, such as keyword-driven testing and data-driven testing, comprehensive and maintainable automated test suites can be developed. Embracing automated testing will undoubtedly lead to better software quality and improved overall efficiency in the software development process.
Comments:
Great article! I found it really insightful and informative.
Sarah, I'm glad you found the article helpful. I'm excited about the potential of AI in software testing.
I agree, Sarah! The potential of leveraging ChatGPT for automated testing is massive.
Indeed, AI-powered testing could revolutionize software development.
Anna, I agree that AI-powered testing can boost productivity and improve software quality.
Julian, absolutely. AI can reduce human error and speed up the testing process.
Anna, exactly! AI can augment human testers, making software testing more efficient.
I'm curious about the limitations of using ChatGPT for testing. Has anyone encountered any challenges?
Hi David, one limitation is that ChatGPT might struggle with understanding complex requirements or domain-specific scenarios.
Interesting, Emily. So it may not be suitable for all types of testing.
David, I understand your concerns. ChatGPT has its limitations, particularly in complex scenarios.
Thanks for your comments, everyone! I appreciate your feedback.
I believe ChatGPT could be a valuable tool for regression testing. It can generate test cases automatically.
I wonder how well ChatGPT handles dynamic web applications that rely heavily on JavaScript.
Definitely, John. ChatGPT may struggle with dynamically changing elements.
Laura, that's a valid concern. JavaScript-driven apps can be challenging.
Laura, agreed. ChatGPT may not handle dynamically generated content well.
Laura and Emily, thanks for clarifying. It's important to consider these limitations before adopting AI for testing.
John, indeed. Complex interactions may require human understanding and testing.
Emily and Laura, I appreciate your insights. It's crucial to strike a balance between AI and manual testing.
Absolutely, John. Both AI-powered testing and manual testing play important roles.
As Aaron mentioned, it's essential to consider the limitations and use AI testing judiciously.
Anna, indeed. We should leverage AI to enhance our testing capabilities.
Julian, absolutely! AI and humans working together can lead to better testing outcomes.
Anna, collaborative testing can identify issues that AI or humans alone may overlook.
Julian, that's a great point. AI and human teamwork can enhance testing coverage.
Exactly, Laura. The collaboration ensures comprehensive and accurate software testing.
You're welcome, John! Finding the right balance ensures better software quality.
John, it's all about selecting the right approach for each testing phase.
John, that's true. Humans provide contextual insights that AI may miss.
Absolutely, Laura. Humans bring unique skills and intuition to the testing process.
Melissa, Laura, I completely agree. Collaborating AI with human testers is the way forward.
Indeed, John. A balanced approach ensures comprehensive testing and delivers quality software.
Laura, Anna, and John, I appreciate your insights. Balancing AI and human intelligence seems crucial for success.
Agreed, David. The right balance ensures effective testing while leveraging AI's strengths.
David, striking that balance is indeed challenging, but it can lead to optimal outcomes.
Laura, John, I'm glad we agree on the importance of collaboration. It enhances the overall testing process.
Absolutely, Julian. Collaboration brings together diverse strengths for better test coverage.
David, well said! Collaboration maximizes our ability to deliver high-quality software.
AI-powered testing can help us focus on more critical tasks, making automation a huge boon.
That's correct, Melissa. Automation frees up time for more valuable activities.
Melissa, I agree. AI can handle repetitive testing, allowing humans to focus on creativity.
AI is a powerful tool, but it cannot replace human expertise and intuition.
It's great to see such a lively discussion on AI-powered testing. Collaboration is key!
Collaboration is key in any testing approach. Different perspectives lead to robust outcomes.
Well said, John. Combining inputs from AI and humans minimizes the risk of missing important defects.
Collaboration is not only important in testing but in all aspects of software development.
John, you're absolutely right. Collaboration fosters better communication and teamwork.
Well summarized, Anna. Collaboration is crucial for driving success in any software project.
Indeed, Anna and Laura! Collaboration fuels innovation and helps us deliver exceptional software.