Taking Predictive Analytics in the Semantic Web to the Next Level with ChatGPT
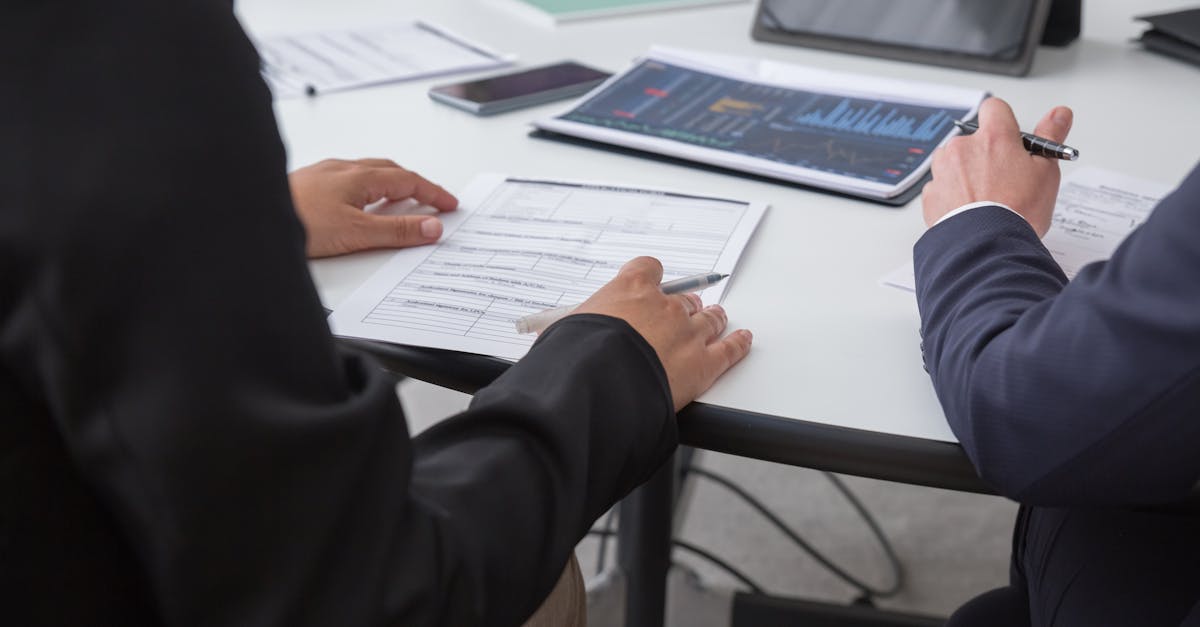
Introduction
Predictive analytics is a field that utilizes historical and current data to predict future outcomes. It has revolutionized various industries such as finance, healthcare, and marketing by enabling businesses to make data-driven decisions.
The Need for Semantic Web
As the amount of data continues to grow exponentially, it becomes increasingly important to extract meaningful insights from this vast pool of information. Traditional predictive models often rely on structured data and predefined relationships, which can limit their ability to capture complex relationships and patterns.
This is where the Semantic Web comes into play. The Semantic Web is an extension of the World Wide Web that aims to facilitate machine understanding of information. It allows data to be linked and interpreted in a meaningful way by assigning semantic relationships between different data points.
Enhancing Predictive Models
The Semantic Web can greatly enhance predictive models in predictive analytics by providing a deeper understanding of the data. By leveraging semantic relationships, predictive models can capture hidden patterns and associations that might go unnoticed using traditional methods.
For example, in the healthcare industry, predicting the risk of a certain disease requires analyzing various factors such as patient demographics, medical history, lifestyle choices, and genetic predispositions. By applying semantic relationships between these data points, predictive models can identify complex interactions and correlations that contribute to the disease risk.
Benefits of Semantic Web in Predictive Analytics
1. Improved Accuracy: Semantic Web enables predictive models to capture intricate relationships between data points, resulting in more accurate predictions.
2. Uncovering Hidden Insights: By understanding the semantic relationships, predictive models can uncover hidden patterns and associations that were previously unknown.
3. Enhanced Decision-making: The insights gained from enhanced predictive models can help businesses make better-informed decisions, leading to improved outcomes and increased efficiency.
Conclusion
The Semantic Web plays a crucial role in enhancing predictive models in predictive analytics. Through a deeper understanding of the semantic relationships between various data points, predictive models can unlock hidden insights, improve accuracy, and facilitate better decision-making. As the volume and complexity of data continue to grow, the adoption of Semantic Web technologies becomes increasingly important in the field of predictive analytics.
Comments:
Thank you all for joining the discussion! I'm excited to hear your thoughts on the article.
Great article, Tiffani! I've been using predictive analytics for a while now, and the idea of combining it with ChatGPT sounds intriguing. Can you share some examples of how it could be applied?
Hi Sarah! Absolutely, combining predictive analytics with ChatGPT can be quite powerful. One example is enhancing customer service by using predictive analytics to anticipate customer needs and ChatGPT to provide personalized recommendations or resolutions.
This article got me thinking about the ethical implications. How do we ensure that the application of predictive analytics and ChatGPT is done responsibly?
Hi Michael! That's an important question. Responsible application of these technologies requires careful monitoring, transparency, and regular audits to ensure the algorithms don't perpetuate biases or discriminate against any group. Additionally, obtaining informed consent and being transparent about data usage are crucial steps.
I'm curious to know more about the technical challenges in implementing this combination. Are there any specific hurdles to overcome?
Hi Emily! Integration of predictive analytics and ChatGPT presents technical challenges such as data integration, ensuring real-time data flow, and optimizing performance to handle large-scale data. It requires expertise in data engineering, natural language processing, and machine learning to overcome these hurdles.
I see the potential of using this technology in healthcare, especially in predicting patient outcomes and assisting in medical diagnoses. However, privacy concerns arise when dealing with sensitive medical data. How can these concerns be addressed?
Hi David! Privacy is a crucial aspect when working with sensitive data, especially in healthcare. The concerns can be addressed through adopting rigorous data anonymization techniques, strict access controls, and complying with applicable privacy regulations such as HIPAA. Additionally, transparency in data usage and clear user consent mechanisms can help build trust.
The combination of predictive analytics and ChatGPT does sound promising. How does it handle situations where predictions might be incorrect or ChatGPT provides incorrect information?
That's a valid concern, Amy. It's important to acknowledge that no system is perfect, and errors can occur. In such cases, organizations should have mechanisms in place to handle incorrect predictions, provide human verification when needed, and continually improve the models through feedback loops.
Tiffani, I'm interested in the scalability of this combination. How well does it perform when dealing with large-scale datasets or high-volume interactions?
Hi Jonathan! Scalability is indeed a key consideration. With efficient infrastructure and distributed computing, such as leveraging cloud platforms and optimizing data pipelines, the combination can handle large-scale datasets and high-volume interactions with proper design and implementation strategies.
The integration of predictive analytics and ChatGPT sounds promising, but are there any limitations or potential risks we should be aware of?
Hi Lisa! Absolutely, it's important to be aware of the limitations and risks. Some limitations include the need for quality training data, potential biases in predictive models, and ChatGPT's tendency to generate plausible but incorrect responses. Organizations should invest in rigorous testing and validation to mitigate risks and be aware of any limitations in their specific domains.
I'm curious about the computational requirements. Would implementing this combination require significant computational resources?
Hi Mark! The computational requirements depend on factors like the volume of data, complexity of models, and desired real-time capabilities. While it may require resources, advancements in hardware and cloud computing have made it more accessible. Starting with smaller-scale deployments and gradually scaling up can be a good approach.
This combination can definitely revolutionize marketing strategies by providing more personalized experiences to customers. How can businesses get started with implementing predictive analytics and ChatGPT?
Hi Olivia! To get started, businesses can begin by defining clear objectives, identifying relevant data sources, and building a strong data foundation. They may need to collaborate with data scientists, natural language processing experts, and developers to implement predictive analytics and integrate ChatGPT based on their specific use case.
I wonder how end-users will perceive and interact with such a system. Are there any challenges in user acceptance and adoption?
Hi Jennifer! User acceptance and adoption are indeed critical for success. Challenges may include initial resistance from users, concerns about privacy and trust, and the need for clear communication regarding the capabilities and limitations of the system. User feedback and iterative improvements can help address these challenges.
The potential of combining these technologies is exciting, but how accessible are they to organizations with limited resources or technical expertise?
Hi Matthew! Initially, limited resources and technical expertise can be a challenge. However, as the technology evolves, it becomes more accessible. Organizations can partner with AI service providers or leverage cloud-based platforms that offer pre-built models and tools to kickstart their implementation without extensive technical knowledge.
I'm concerned about the potential biases that could be embedded in predictive analytics. How can we ensure fairness and avoid reinforcing existing biases?
Hi Andrew! Ensuring fairness is crucial in predictive analytics. It requires implementing fairness-aware machine learning techniques, regularly auditing and monitoring the models, and incorporating diverse perspectives during the development and testing phases. Organizations must actively strive to avoid reinforcing existing biases and work towards equitable outcomes.
The use of ChatGPT in predictive analytics brings exciting possibilities for personalized recommendations. Can you share an example of how a company has successfully utilized this combination?
Hi Sophia! One example is a travel booking platform that uses predictive analytics to analyze user preferences, combine it with historical data, and leverage ChatGPT to provide personalized trip recommendations. This has significantly improved the user experience and increased customer satisfaction.
What are the key skills and expertise needed to implement this combination successfully?
Hi Robert! Successfully implementing this combination requires skills in data engineering, machine learning, natural language processing, and cloud computing. Additionally, domain knowledge related to the specific use case is valuable to extract meaningful insights and develop effective models.
Are there any industry-specific challenges or considerations to keep in mind when implementing this combination?
Hi Emma! Industry-specific challenges may include compliance regulations in highly regulated sectors like finance or healthcare, the dynamic nature of customer preferences in retail, or the need for interpretable models in sectors where transparency is crucial. Understanding these considerations and tailoring the implementation accordingly is essential.
Can you elaborate on the role of human oversight in this combination of predictive analytics and ChatGPT?
Hi Anthony! Human oversight plays a vital role in validating predictions, ensuring ethical use, and managing system failures. It involves monitoring model performance, handling exceptions or edge cases, and having clear processes to escalate to human experts when necessary. Human oversight helps maintain accountability and mitigate potential risks.
What are some potential future developments or advancements we can expect in this field?
Hi Lily! The field of combining predictive analytics and ChatGPT is continuously evolving. We can expect advancements in areas like more accurate predictive models, improved natural language understanding, better integration with data sources, and increased interpretability to inspire confidence in predictions. Additionally, research on tackling biases and fairness in AI systems will likely continue to progress.
Considering the rapid evolution of technologies, how do you foresee the future impact of predictive analytics and ChatGPT in industries and society as a whole?
Hi Ryan! The future impact of these technologies is expected to be significant. Industries will be able to make more informed decisions, personalize user experiences, and improve operational efficiency. Society as a whole may witness advancements in areas like healthcare outcomes, targeted education, and enhanced accessibility to services. However, responsible development and deployment will be crucial to ensure the positive impact outweighs any potential risks.
How can organizations maintain transparency and build trust while using predictive analytics and ChatGPT?
Hi Grace! Organizations can maintain transparency by clearly communicating how data is being used, providing explanations for predictions, and describing the limitations and potential biases in the system. Building trust involves offering opt-in/opt-out mechanisms and involving end-users in the development process to address their concerns and preferences.
Thank you all for your insightful questions and comments! It was a pleasure discussing the potential of combining predictive analytics and ChatGPT with you. If you have any further questions, feel free to reach out.