The Disruptive Potential of Gemini in the Technological Proteomics Landscape
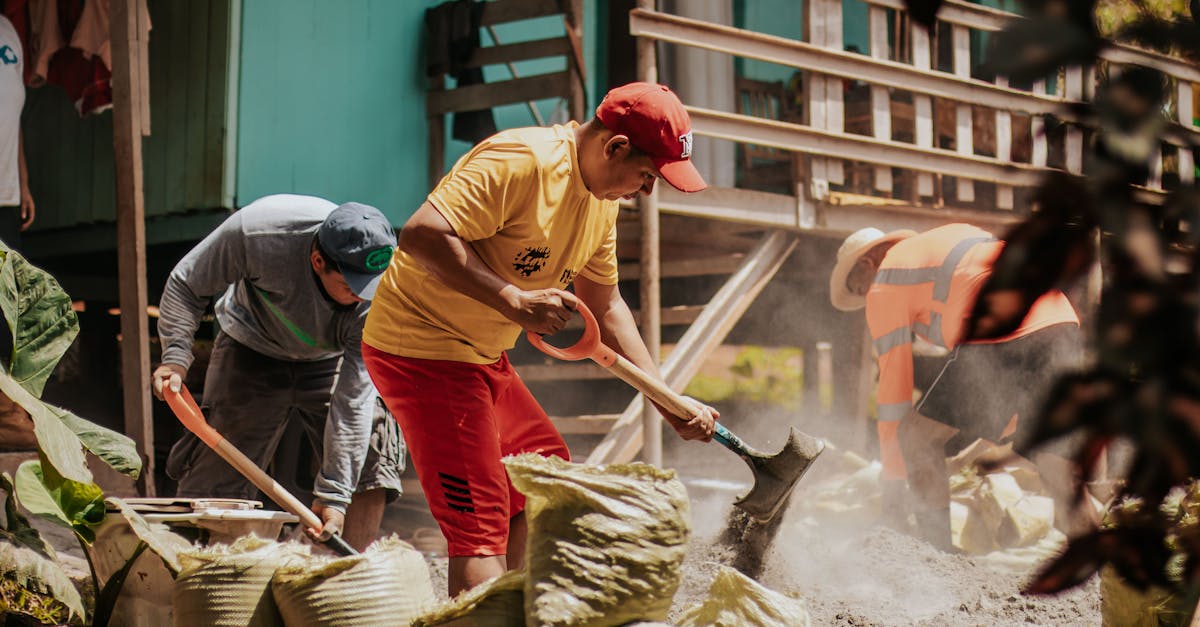
Technological proteomics is a rapidly evolving field that aims to study the complete set of proteins in an organism, tissue, or cell. With recent advancements in artificial intelligence (AI) and natural language processing (NLP) technologies, the potential for transformative innovation in this field has greatly increased. One such innovation is Gemini, an advanced language model developed by Google.
What is Gemini?
Gemini is a language model developed by Google that is capable of generating human-like responses given a prompt or a series of prompts. It is powered by the LLM (Generative Pre-trained Transformer) architecture and has been fine-tuned on a vast amount of internet text. Gemini has shown impressive performance in various language-based tasks, including question-answering, dialogue generation, and creative writing.
The Potential Applications in Technological Proteomics
Gemini has the potential to disrupt the technological proteomics landscape in several ways:
- Automated Protein Analysis: Gemini can assist researchers in analyzing protein sequences and structures, predicting protein-protein interactions, and identifying potential drug targets. By leveraging its ability to understand and generate natural language, Gemini can provide valuable insights and recommendations to researchers in real-time.
- Data Interpretation and Visualization: With the increasing amount of data generated by next-generation sequencing technologies and mass spectrometry, there is a pressing need for efficient data interpretation and visualization tools. Gemini can help researchers navigate through large datasets, interpret complex proteomic data, and generate visual representations for better understanding.
- Expanding Knowledgebase: The rapid pace of technological advancements in proteomics often leads to a substantial amount of new information being generated. Gemini can be utilized as a knowledgebase, continuously updated with the latest research findings and insights. Researchers can query Gemini to get accurate and up-to-date information on proteins, pathways, and experimental techniques.
- Virtual Collaboration and Education: Gemini can act as a virtual collaborator, aiding researchers in brainstorming ideas, discussing experimental protocols, and troubleshooting issues in real-time. It can also assist in educating and training the next generation of proteomics researchers, helping them learn complex concepts and techniques through interactive conversations.
- Enhancing Accessibility: Proteomics research traditionally requires expertise in bioinformatics and computational analysis. Gemini can bridge this gap by making proteomics more accessible to researchers from diverse backgrounds. Its user-friendly interface and conversational approach can enable researchers with limited computational skills to navigate and utilize proteomic tools effectively.
Conclusion
The development of Gemini has opened up new possibilities for transforming the technological proteomics landscape. Its ability to understand and generate human-like responses can revolutionize protein analysis, data interpretation, knowledge expansion, collaboration, and accessibility. However, it is important to acknowledge the limitations of Gemini and ensure that it is used responsibly by considering the ethical implications and potential biases associated with AI models. With further advancements and refinements, Gemini can contribute significantly to unraveling the complexities of the proteome and driving innovative discoveries in the field of technological proteomics.
Comments:
Great article, Brittany! Gemini definitely has the potential to disrupt the technological proteomics landscape. The ability to generate human-like responses and understand context will be game-changing for various industries.
I completely agree, Michael. Gemini's potential applications seem unlimited. From customer service to content generation, it could revolutionize how businesses interact with users and save time by automating repetitive tasks.
While Gemini's potential is impressive, I worry about the risks of relying too heavily on AI for important tasks. Human oversight and ethical considerations will be crucial in keeping it in check and ensuring it aligns with our values.
Hi Leonard, that's a valid concern. Ensuring ethical usage of AI is indeed important. Google, the organization behind Gemini, acknowledges the importance of safety and is actively working on improvements to address potential risks.
Absolutely, Leonard. We have to be cautious and avoid blindly trusting AI, especially when it comes to sensitive information or decision-making. Human judgment and intervention should complement AI tools.
I'm excited about the potential of Gemini, but we also need to discuss the biases and limitations it might have. AI models can inadvertently reinforce existing biases present in the training data. This should be addressed to ensure fairness and inclusivity.
You're absolutely right, Mason. Bias mitigation is an important aspect. Google is actively working to address biases and improve calibration so that the model is unbiased and reliable in providing helpful and accurate responses.
I can see how Gemini can be a useful tool, but I also worry about potential job displacement. If AI can automate tasks traditionally done by humans, what will be the impact on employment?
Valid concern, Olivia. While AI may automate certain tasks, it also has the potential to create new job opportunities. We need to adapt our skills and focus on tasks that require human creativity, problem-solving, and emotional intelligence.
I agree with Michael. AI can augment our abilities rather than replace us entirely. By automating repetitive tasks, it frees up time for humans to focus on more complex and meaningful work.
Gemini sounds promising, but how well does it handle domain-specific knowledge? In technological proteomics, accuracy and understanding of complex scientific concepts are crucial.
Hi Daniel, Gemini performs well in various domains, including science and technology. However, domain-specific knowledge is an ongoing focus area for improvement. Google plans to enable users to easily customize the model to suit specific domains.
I wonder about the potential privacy concerns when using Gemini. What measures are in place to prevent misuse or unauthorized access to sensitive user data?
Privacy is a top priority, Natalie. Google is committed to protecting user data and plans on providing clear guidelines and policies to prevent misuse. They are actively exploring ways to allow users to have more control over their data.
While the potential of Gemini is exciting, what about its limitations? Are there scenarios where it may not be suitable or produce inaccurate results?
Good question, David. Gemini is impressive, but it has limitations. In some cases, it may generate incorrect or nonsensical answers. Google acknowledges this area for improvement and continues to make regular updates to enhance model performance.
This technology is fascinating, but what about potential security vulnerabilities? AI systems can be exploited by malicious actors. How do we ensure the safety and security of Gemini?
Hi Adam, security is vital. Google takes security seriously and employs measures to prevent unauthorized access or misuse. They actively encourage user feedback to identify and address potential vulnerabilities for a safer experience.
While Gemini shows promise, I worry about the environmental impact of these large AI models. The computational resources required for training and inference can have a significant carbon footprint.
You raise a valid concern, Sophia. Google aims to make AI models more efficient to reduce their environmental impact. They are actively researching and investing in methods to lower resource requirements without compromising performance.
I believe that with responsible development and continuous improvement, Gemini can bring numerous benefits. Its potential to transform industries is vast, and it's exciting to see how it will evolve over time.
I couldn't agree more, Michael. Gemini is a remarkable advancement in AI, and it will shape the way we interact with technology, paving the way for more efficient and intuitive systems.
While the potential is exciting, we must remember to proceed with caution. Striking the right balance between AI and human judgment will ensure the responsible and beneficial integration of technologies like Gemini.
Looking forward to seeing how Gemini develops. As with any new technology, it's important to address potential concerns and challenges early on to maximize the positive impact it can have.
I'm intrigued by the potential applications of Gemini. The ability to understand and generate human-like responses can truly revolutionize how we interact with technology. Exciting times ahead!
I appreciate the article's insights into Gemini's disruptive potential. It brings forth both excitement and valid concerns. As AI continues to advance, responsible development should be a priority.
It's great to see the ongoing efforts to address biases and privacy concerns in AI models like Gemini. Ethical considerations and user safety should always accompany technological advancements.
The limitations and potential inaccuracies of Gemini are important to consider. It's crucial to manage expectations and understand when and where AI models may fall short.
Security should be a top priority as AI becomes more prevalent. It's relieving to know that Google is proactive in addressing potential vulnerabilities and ensuring a safe user experience.
Addressing the environmental impact of AI models is essential. I'm glad to hear that Google is actively working on making AI more efficient and reducing its carbon footprint.
Thank you all for your insightful comments and concerns. It's encouraging to see such engaged discussions around the potential and challenges of Gemini. Your feedback and perspectives are valuable in shaping responsible AI development.
If you have any further questions or thoughts, please don't hesitate to share. I'm here to answer your inquiries and discuss this exciting topic further.
Thank you, Brittany, for providing us with this platform to exchange ideas. It's been an insightful discussion, and it's great to see your active engagement with the community.
Indeed, thank you, Brittany. This discussion has been valuable, emphasizing the importance of responsible AI development and the need to address concerns early on. Appreciate your thoughtful responses.
Thank you, Brittany Mason, for joining in and providing us with further information and insights. It's great to see your dedication to addressing the community's concerns.
You're all very welcome. I'm glad to have participated in this discussion with such insightful individuals. Let's continue to stay aware and involved in shaping the future of AI.
Thank you, Brittany, and everyone else for sharing your thoughts. This has been an enlightening discussion, highlighting both the excitement and responsibility that accompany technological advancements.
I appreciate the opportunity to engage in this discussion. It's crucial to consider the implications of new technologies like Gemini and promote responsible usage. Thank you, Brittany, for facilitating this conversation.
Thank you, Brittany, for your valuable responses and moderating this discussion. It's encouraging to see the diverse perspectives shared here, emphasizing the importance of ethics and safety in AI.
Thank you all once again for your active participation. As we navigate the integration of AI into various industries, responsible development and continuous improvement remain key. Stay curious and engaged!
Thank you all for reading my article on the disruptive potential of Gemini in the technological proteomics landscape. I'm excited to hear your thoughts and opinions!
Great article, Brittany! I agree that Gemini has the potential to revolutionize the technological proteomics landscape. The ability to generate human-like responses opens up possibilities for more interactive and personalized experiences.
I found the article very informative. It's fascinating to see how AI applications like Gemini can impact various fields, including technological proteomics. The potential for faster analysis and discovery is promising.
While I see the potential, I have concerns about the accuracy of the output from Gemini. How can we ensure that the generated responses are reliable in the context of analyzing complex proteomics data?
Thank you for your comment, David. Validating the accuracy of Gemini's responses is an important aspect. Researchers are exploring methods to verify the reliability of AI-generated outputs, including comparing them with existing data and involving domain experts in the evaluation process.
Thank you for addressing my concern, Brittany. Validating the accuracy of AI-generated responses through expert evaluation is essential for reliable proteomics analysis.
Thank you for addressing my concern, Brittany. Collaborative efforts from researchers and AI models can help in improving the reliability of proteomics analysis.
I believe Gemini can be a valuable tool in the technological proteomics landscape, but we should also consider potential bias in the AI model's training data. Bias can lead to skewed results and potentially hinder progress. It's crucial to address this issue.
Absolutely, Riley. Bias in AI models is a pressing concern. It's crucial to ensure diverse and representative training datasets to mitigate any unintended biases. Continual monitoring and improvement of Gemini's training process can help address this challenge.
I'm excited about the potential of Gemini in the field of technological proteomics. It could greatly assist researchers in exploring complex relationships between proteins and accelerating the discovery process.
Indeed, Sophia! Gemini has the capacity to complement researchers' work by providing automated insights and assisting in faster exploration of protein data. It's an exciting time for technological proteomics.
I can see how Gemini can be advantageous, but how can we ensure the privacy and security of sensitive proteomics data when employing AI models like Gemini? Any thoughts on this?
That's an important concern, Connor. When deploying AI models like Gemini, it's crucial to implement strong security measures and adhere to strict privacy protocols. Ensuring data encryption, restricted access, and anonymization techniques can safeguard the sensitive proteomics data used during analysis.
Thank you for your response, Brittany. Ensuring data privacy and security measures are in place will help build trust in AI utilization for proteomics analysis.
Absolutely, Connor. Privacy and security protocols must be in place to protect sensitive proteomics data when utilizing AI models like Gemini.
I completely agree with you, Brittany. Gemini's interactive capabilities can enhance user experiences and analysis in the field of technological proteomics.
I agree with your concern, Connor. Ensuring data privacy and security is of utmost importance, especially when dealing with sensitive proteomics data. Encryption and strict access controls can help address these concerns.
I'm curious about the computational resources required to run Gemini effectively in the context of technological proteomics. Are there any limitations or challenges in that regard?
Great question, Ethan. Running Gemini can require significant computational resources, especially when dealing with vast proteomics datasets. However, advancements in cloud computing and distributed systems can help mitigate these challenges and make Gemini more accessible.
Thanks for providing insights, Brittany. Advancements in cloud computing and distributed systems will undoubtedly play a crucial role in making AI models like Gemini more accessible in the field of technological proteomics.
Thank you, Brittany. Advancements in cloud computing and distributed systems will certainly make running AI models like Gemini more feasible in the field of proteomics.
Indeed, Brittany. Advancements in cloud computing and distributed systems will play a significant role in making AI models like Gemini more accessible.
While the potential is exciting, we must also consider ethical implications that arise when using AI models like Gemini. How do we ensure responsible AI usage in the technological proteomics field?
Ethical considerations are crucial, Natalie. Responsible AI usage can be ensured through transparent and accountable practices, regularly reviewing and addressing potential biases, and involving stakeholders in decision-making processes. Open dialogue and collaboration among researchers, policymakers, and industry experts are essential.
Transparent and accountable practices are key, Brittany. The responsible use of AI models can ensure ethical AI practices in the field of technological proteomics.
I'm glad we're on the same page, Brittany. Responsible AI usage requires a collective effort from all stakeholders involved in the technological proteomics field.
Ethical implications cannot be ignored, Natalie. Implementing clear guidelines and regular audits to assess AI system behavior can safeguard against any ethical concerns in the technological proteomics landscape.
Continual improvement and monitoring are essential, Riley. Bias mitigation strategies should be implemented to ensure AI models like Gemini provide fair and unbiased results in the technological proteomics landscape.
Indeed, Olivia. Building diverse and representative datasets is vital to avoid perpetuating biases in the outcomes of AI models used in technological proteomics.
That's true, Olivia. Continuous monitoring and improvement of AI models like Gemini are essential to address biases in the technological proteomics landscape.
I agree, Riley. Transparent and inclusive practices are essential in ensuring the responsible deployment of AI models like Gemini in the technological proteomics landscape.
You're right, Riley. Establishing stringent guidelines and vetting processes for AI system behavior can prevent ethical concerns in technological proteomics.
I'm excited about the potential of Gemini in the technological proteomics landscape, but do you think AI models like this will replace human researchers altogether? I have mixed feelings about that.
It's an interesting question, Emily. I don't see AI models replacing human researchers entirely. Instead, tools like Gemini can augment the capabilities of researchers, providing assistance and generating insights to accelerate their work. Human expertise and critical thinking remain invaluable.
I appreciate your perspective, Brittany. The collaboration between AI tools and human researchers can certainly lead to powerful advancements in the field of technological proteomics.
I completely agree with you, Brittany. AI models like Gemini should always be seen as supportive tools for human researchers, not replacements.
I appreciate your viewpoint, Brittany. AI models like Gemini should be seen as tools to augment human researchers, not replace them.
I'm curious about the integration of Gemini with existing technological proteomics tools. How can we effectively combine AI models like Gemini with established software for comprehensive analysis?
Great point, Samuel. Integration is key. APIs and software libraries can facilitate the seamless integration of Gemini with existing technological proteomics tools, allowing researchers to leverage both the power of AI and the functionalities of established software for comprehensive analysis.
Thank you for addressing my query, Brittany. The integration of AI models with existing tools has the potential to provide a comprehensive analytical experience for researchers.
Thank you for your response, Brittany. APIs and software integration can enhance the capabilities of AI models in the technological proteomics landscape.
I see significant potential in Gemini, but I also worry about over-reliance on AI models. It's important to strike a balance and ensure human interpretation and judgment are not overlooked in the pursuit of automation.
You raise a valid concern, Jackie. Striking a balance is crucial, and human interpretation and judgment should never be replaced entirely. AI models like Gemini should be used as tools to assist and augment human researchers, not overshadow their expertise.
The potential applications of Gemini in the technological proteomics landscape are vast. How do you envision the future of AI-assisted proteomics research with the wide adoption of such models?
The future looks promising, Oliver. With wide adoption of AI models like Gemini, we can expect faster and more efficient analysis, enhanced discovery of protein relationships, and accelerated advancements in the field of technological proteomics. Collaboration between AI and human researchers will unlock exciting possibilities.
Absolutely, Brittany. Gemini's ability to generate interactive and personalized responses can significantly enhance the user experience in the field of technological proteomics.
I share your optimism, Brittany. The adoption of AI models like Gemini will pave the way for exciting advancements and discoveries in the field of technological proteomics.
The future integration of AI models like Gemini will undoubtedly lead to exciting breakthroughs and advancements in the field of technological proteomics. Collaboration between human researchers and AI tools is key.
Collaboration and coordination between human researchers and AI models like Gemini are crucial in leveraging the full potential of technological proteomics.
Definitely, Brittany. The ability to generate personalized responses can enhance user engagement and lead to more efficient analysis in technological proteomics.