The Gemini Revolution: Enhancing 'in Situ Hybridization' Technology through Conversational AI
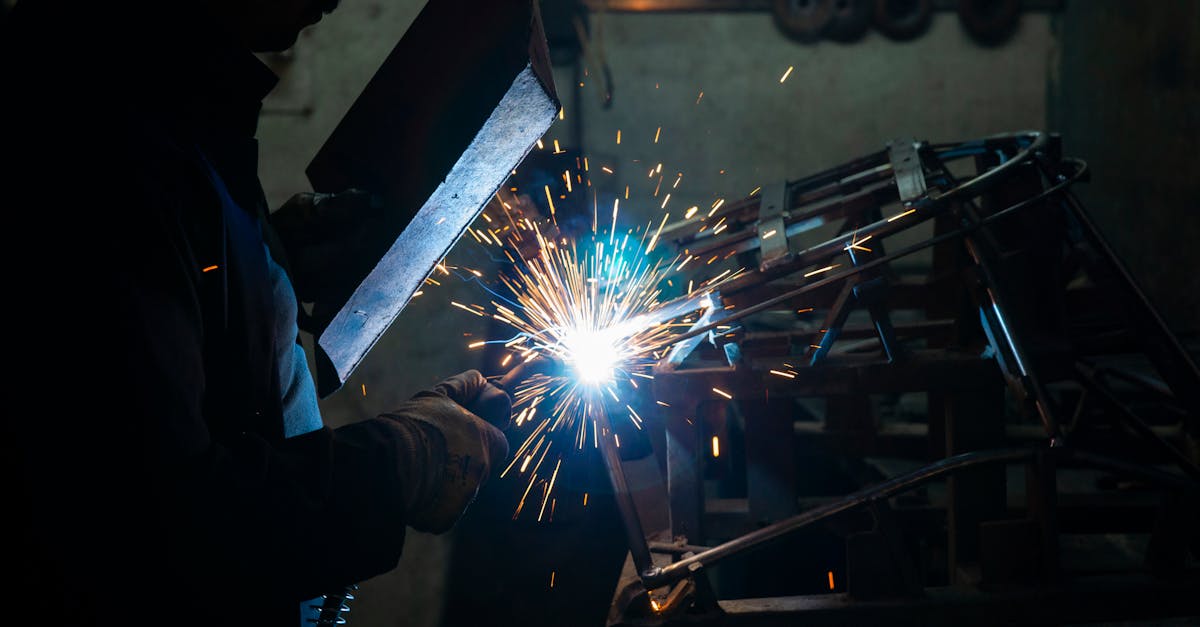
In situ hybridization is a powerful technology used in molecular biology to visualize and localize specific nucleic acid sequences within tissues or cells. It has revolutionized our understanding of gene expression patterns and cellular functions. However, like any technology, it has its limitations.
Enter Conversational AI, a cutting-edge technology that has the potential to enhance the capabilities of in situ hybridization. With the recent advancements in language models, such as Google's Gemini, researchers can leverage the power of natural language processing to improve the processes involved in this technique.
One of the major challenges in in situ hybridization is the design and optimization of probes. These short nucleic acid sequences are labeled with a detectable molecule and are used to target specific RNA or DNA sequences. Designing effective probes requires a deep understanding of the target gene, its variants, and potential cross-reactivity. This is where Conversational AI can play a vital role.
By interacting with a language model like Gemini, researchers can discuss their target gene, seek advice on probe design strategies, and receive real-time feedback. The model can analyze vast amounts of literature, databases, and experimental data to provide valuable insights into probe design. This not only saves time but also enhances the accuracy and efficiency of the process.
Moreover, Conversational AI can assist in interpreting in situ hybridization results. Analyzing and ascribing biological meaning to the patterns of gene expression within tissues or cells can be complex. Researchers often encounter ambiguous or contradictory results, making the interpretation challenging. However, by engaging in a conversation with a language model, researchers can obtain suggestions, hypothesis explanations, and explore alternative interpretations.
Another area where Conversational AI can revolutionize in situ hybridization is in data analysis. High-throughput techniques generate massive amounts of data, and extracting meaningful insights from this data can be a daunting task. Language models can aid researchers in navigating through the data, identifying patterns, and finding hidden relationships. The model can guide researchers in data preprocessing, statistical analysis, and data visualization, making the entire process more efficient.
While Conversational AI has incredible potential in enhancing in situ hybridization, it is important to note that it is not a replacement for domain expertise. Researchers must exercise caution and critically evaluate the suggestions provided by the language model. Additionally, the ethical and privacy concerns surrounding the use of AI in the research field should not be overlooked.
In conclusion, Conversational AI, powered by language models like Gemini, offers promising opportunities to enhance the capabilities of in situ hybridization. By leveraging natural language processing, researchers can optimize probe design, interpret results, and analyze data more effectively. While challenges and ethical considerations exist, the integration of Conversational AI with in situ hybridization technology has the potential to revolutionize molecular biology research.
Comments:
This article on enhancing 'in Situ Hybridization' technology through Conversational AI sounds fascinating! I've been following the Gemini revolution closely and I'm excited to see how it can improve scientific research.
I agree, Jessica! The potential applications of Conversational AI in scientific research are tremendous. It can save researchers a lot of time and effort, leading to faster advancements.
As a biologist, I'm intrigued to learn more about how Conversational AI can enhance 'in Situ Hybridization' technology. It could revolutionize our understanding of gene expression!
I agree, Maria! 'in Situ Hybridization' is a powerful technique, and combining it with Conversational AI can enhance its accuracy and make data interpretation easier.
Thank you all for showing interest in the article! I believe Conversational AI can indeed have a significant impact on scientific research. It's time to explore its potential in 'in Situ Hybridization'.
I'm curious to know how exactly Conversational AI can enhance the 'in Situ Hybridization' technology? Can anyone elaborate on that?
Sure, Daniel! Conversational AI can assist researchers in analyzing gene expression patterns more efficiently. It can process vast amounts of data, identify patterns, and help researchers make sense of complex information.
I'm excited about the potential of Conversational AI in improving scientific research, but I also have concerns. How do we ensure the reliability and accuracy of the AI-generated results?
Emily, you raise a valid concern. To ensure reliability, it's essential to validate the AI-generated results against existing manual methods. Close collaboration between AI systems and human experts is necessary for accurate interpretation.
@Bill Mendello | Thank you for addressing our questions! 'In Situ Hybridization' combined with AI indeed seems like a powerful combination that can transform the field and open up new avenues for research and applications.
I can see the benefits of using Conversational AI in scientific research, but we should be cautious about overreliance on AI. It should assist researchers, not replace them.
I agree, Gregory. AI can be a powerful tool, but it's important to maintain a balance between human expertise and AI assistance. Researchers should leverage the strengths of both.
The advancements in Conversational AI are impressive, but we also need to address ethical concerns. How do we prevent biases in AI models from skewing research outcomes?
John, you bring up a critical point. Addressing biases in AI models requires careful data selection, algorithm development, and continuous evaluation. Ethical considerations should always guide our use of AI in research.
@Bill Mendello | Thank you for explaining the potential applications of Gemini. It's fascinating to see how AI can revolutionize 'in Situ Hybridization'. I'm excited about the possibilities!
@Bill Mendello | Has the technology reached a stage where AI can assist in designing optimal 'in Situ Hybridization' experiments based on given datasets and specific research questions?
@John Harper | We are getting closer to the stage where AI can assist in designing 'in Situ Hybridization' experiments. While it may not be fully autonomous decision-making, AI can provide valuable guidance based on previous datasets, experiment objectives, and constraints.
@Bill Mendello | That's amazing to hear! Exciting times ahead for 'in Situ Hybridization' researchers. Thank you for your insights!
@John Harper | Indeed, we are witnessing exciting advancements in 'in Situ Hybridization' research. Pairing AI and domain expertise can have tremendous potential in unraveling the complexities of gene expression spatially within tissues.
@Bill Mendello | Thank you for highlighting the importance of data privacy and responsible use of AI in 'in Situ Hybridization'. These aspects must be taken seriously to maintain public trust and ethical standards.
@Bill Mendello | Thank you for taking the time to engage with us and provide valuable information about the integration of Gemini into 'in Situ Hybridization'. Exciting possibilities lie ahead!
I think Conversational AI can greatly improve collaboration among researchers. It can facilitate knowledge-sharing and enable experts to connect and learn from each other's findings.
Absolutely, Julia! Conversational AI platforms can create virtual environments where researchers can exchange ideas, share data, and collectively work towards scientific breakthroughs. Collaboration is key!
The integration of Conversational AI with 'in Situ Hybridization' could also enhance the accessibility of scientific research. It can help bridge the gap between complex scientific findings and non-expert audiences.
I agree, Michelle! Making scientific research more accessible to the general public is crucial. Conversational AI can assist in simplifying scientific findings and conveying them in a more understandable manner.
While Conversational AI shows promise, we should consider the potential limitations and risks. Security and privacy concerns must be addressed to ensure the safe use of AI in scientific research.
Anna, I completely agree. Maintaining data privacy and security is of utmost importance when integrating Conversational AI into scientific research. Proper safeguards and protocols need to be in place.
Could Conversational AI eventually lead to automating the entire 'in Situ Hybridization' process? It would be a game-changer if researchers could save time and improve efficiency.
Interesting point, Sarah! While complete automation might not be feasible in the near future, Conversational AI can certainly streamline various aspects of the 'in Situ Hybridization' process and make it more efficient.
I wonder if the integration of Conversational AI can also help identify new insights and patterns in 'in Situ Hybridization' data that might be overlooked by manual analysis?
Jessica, that's a great point! Conversational AI has the potential to identify hidden patterns and correlations in large datasets, leading to novel discoveries in 'in Situ Hybridization' research.
While I'm excited about the benefits of AI in scientific research, we should pursue rigorous validation and peer review processes to ensure the quality and replicability of AI-generated results.
Michael, I couldn't agree more. Rigorous validation and peer review remain essential steps in upholding scientific integrity, regardless of the tools or technologies used.
I see great potential in Conversational AI for cross-disciplinary collaborations in 'in Situ Hybridization'. It can bring together experts from different fields to collectively tackle complex research questions.
Emily, you're absolutely right! By connecting researchers from various disciplines, Conversational AI can facilitate the exchange of ideas, fostering innovative approaches to 'in Situ Hybridization' research.
What are the challenges and limitations researchers might face while integrating Conversational AI with 'in Situ Hybridization' technology? I'm curious to know more about potential hurdles.
Daniel, some challenges include interpreting complex AI-generated results, addressing biases, ensuring data privacy, and training researchers to effectively use AI tools. Overcoming these hurdles will require ongoing efforts.
I think it's essential for researchers to collaborate closely with AI developers and data scientists while integrating Conversational AI into 'in Situ Hybridization'. An interdisciplinary approach is crucial for success.
I believe that Conversational AI has the potential to empower researchers by automating repetitive tasks, allowing them to focus on higher-level analysis and interpretation.
Julia, you're absolutely right! By automating time-consuming tasks, researchers can allocate more time and effort towards critical thinking, hypothesis generation, and advancing scientific knowledge.
I hope the integration of Conversational AI in 'in Situ Hybridization' will also accelerate the pace of discoveries in the field of gene expression, leading to breakthroughs that can benefit many.
While Conversational AI presents exciting opportunities, researchers need to be mindful of potential biases encoded in AI algorithms. Ensuring fairness and inclusivity in AI findings should be a priority.
Anna, I completely agree with you. Addressing biases in AI algorithms is crucial to avoid perpetuating disparities and to ensure that AI-driven advancements benefit everyone.
I wonder, can Conversational AI models be trained specifically to analyze 'in Situ Hybridization' data? Tailoring the models for specific scientific domains might lead to even better results.
Jessica, that's a great point! By training AI models on domain-specific data, we can improve their accuracy, efficiency, and overall performance in analyzing 'in Situ Hybridization' data.
I'm fascinated by the potential of Conversational AI to accelerate scientific breakthroughs by assisting researchers in data analysis and interpretation. It can truly transform how we conduct research.
Michael, I share your fascination! The fusion of Conversational AI and 'in Situ Hybridization' technology holds tremendous promise for advancing our understanding of gene expression and its implications.
Thank you all for engaging in this discussion! Your insights and questions highlight the importance of harnessing the potential of Conversational AI in a responsible and collaborative manner. Let's keep exploring!
Thank you all for your comments on my article! I'm excited to join this discussion and answer any questions you may have.
Great article, Bill! The potential of Gemini to enhance 'in Situ Hybridization' technology is fascinating. How do you see AI being integrated into this field in the near future?
@Mary Thompson | AI can revolutionize 'in Situ Hybridization' by assisting in data analysis and interpretation. It can streamline the process by detecting patterns, localizing signals, and even suggesting experimental designs based on previous datasets.
@Bill Mendello | That sounds promising! Can you provide any real-world examples where Gemini has already been successfully incorporated into 'in Situ Hybridization'?
@Mary Thompson | While Gemini is a recent technology, there have been successful applications of other AI models in 'in Situ Hybridization'. For instance, convolutional neural networks have been used to automatically segment and quantify gene expression signals in stained tissue sections.
@Bill Mendello | Thank you for providing an example. These advancements truly have the potential to transform 'in Situ Hybridization'. Looking forward to seeing Gemini being integrated into this field!
@Bill Mendello | Do you anticipate any ethical or privacy concerns that may arise with the use of AI in 'in Situ Hybridization'?
@Bill Mendello | It's truly remarkable how AI has the potential to shape the future of 'in Situ Hybridization'. Thank you for sharing your insights and expertise with us!
I'm not sure about AI's role in 'in Situ Hybridization' technology. It seems like a completely different domain. Could you explain how they can work together?
@David Martinez | AI can bridge the gap between data generation and analysis. It can help analyze complex datasets, detect patterns, and potentially assist in identifying target genes more efficiently.
@David Martinez | 'in Situ Hybridization' generates vast amounts of image and gene expression data. AI can help analyze this data more efficiently, finding patterns that humans might miss, and potentially enabling new discoveries.
@David Martinez | AI can also assist in data integration by combining 'in Situ Hybridization' results with other omics data, enabling comprehensive analysis and uncovering novel biological insights.
@Paul Lewis | That's an excellent point! AI can help bridge the gap between different levels of genetic information, constructing a more holistic view of gene expression and regulation.
@Paul Lewis | AI can also facilitate the identification of novel biomarkers by correlating 'in Situ Hybridization' results with clinical outcomes or other relevant data sources. This can aid in developing targeted therapies.
@Sharon White | Absolutely! AI can help uncover novel biomarkers that can be utilized in diagnostics or personalized treatment strategies. This highlights the potential of 'in Situ Hybridization' in precision medicine.
I'm curious to know if Gemini can assist in automating parts of the 'in Situ Hybridization' process. Would be a great time-saver!
@Sophia Wright | Yes, Gemini can indeed assist in automating certain aspects of 'in Situ Hybridization'. For example, it can help in automated image analysis, accurately quantifying signals, and reducing manual effort significantly.
@Bill Mendello | Automating image analysis would be amazing. It can save so much time and effort for researchers involved in 'in Situ Hybridization'. Exciting times!
@Bill Mendello | That would be fantastic! Automating signal quantification can reduce subjectivity and potentially improve the reproducibility of 'in Situ Hybridization' experiments.
@Sophia Wright | Absolutely! By automating quantification, AI can minimize human bias and the variability that may arise during manual signal quantification. This has the potential to improve the reliability and reproducibility of 'in Situ Hybridization' experiments.
@Bill Mendello | As data privacy and ethics are paramount concerns, proper protocols need to be in place to ensure secure handling of sensitive patient data. Transparency and guidelines should be established to address any potential ethical concerns.
@Bill Mendello | Agreed! Along with data privacy, informed consent and ensuring the responsible use of AI are crucial factors to consider, especially when dealing with human data in 'in Situ Hybridization' studies.
@Bill Mendello | Consistency and reproducibility in 'in Situ Hybridization' research are critical. AI-powered automation can potentially address these challenges by minimizing the influence of user-dependent factors.
@Bill Mendello | A reduction in human-dependent factors and improved reproducibility can lead to more reliable research outcomes and potentially translate into better clinical applications in 'in Situ Hybridization'.
@Bill Mendello | It sounds like AI can bring about a new era of efficiency in 'in Situ Hybridization'. Exciting possibilities lie ahead for researchers and scientists!
@Bill Mendello | AI-driven automation can also aid in reducing discrepancies between different laboratories by standardizing analysis approaches and ensuring consistent outputs in 'in Situ Hybridization'.
@Sophia Wright | Absolutely! AI has the potential to revolutionize 'in Situ Hybridization' and pave the way for new breakthroughs and advancements in various scientific fields.
@Bill Mendello | The ability to standardize analysis approaches in 'in Situ Hybridization' can also facilitate multi-center studies and collaborations, enabling more robust research outcomes and discoveries.
@Bill Mendello | Thank you for elaborating on the potential of AI in 'in Situ Hybridization'. It's becoming clearer how AI can complement and enhance this field with its data processing and analysis capabilities.
@Bill Mendello | It's an exciting time to be a part of 'in Situ Hybridization' research. AI advancements bring new opportunities for accelerating discoveries and unraveling the mysteries of gene expression!
@Bill Mendello | Standardization and collaboration enabled by AI in 'in Situ Hybridization' can foster sharing of knowledge, methods, and best practices among researchers, driving advancements and accelerating discoveries.
I wonder if there are any limitations or challenges in using AI for 'in Situ Hybridization'. Could it potentially introduce errors in the process?
@Emily Johnson | AI does have some limitations, one being the potential uncertainty in interpreting complex data and balancing false-positive/negative results. However, with proper training and validation, these challenges can be addressed.
@Emily Johnson | Another challenge with AI is explainability. It is important to develop transparent AI models that can provide interpretable results, especially in highly regulated fields like 'in Situ Hybridization'.
@Emily Johnson | Another challenge is the need for large and diverse training datasets to ensure the AI models can generalize well to different sample types and experimental conditions.
@Emily Johnson | AI models should be developed and validated with rigorous scientific methodologies to minimize errors and biases. The interpretability of AI-generated results should also be extensively studied.
@Emily Johnson | Data quality checks and proper validation processes should be implemented to identify and rectify any biases or inaccuracies introduced by AI algorithms in 'in Situ Hybridization'.
@Sophie Anderson | Agreed! The quality of data used to train AI models is crucial. It's important to ensure representatives samples and avoid biases that can impact the accuracy of AI-driven analysis in 'in Situ Hybridization'.
@Emily Johnson | AI models should also be tested on diverse datasets, representing different tissue types, experimental conditions, and populations to ensure robustness and generalizability in 'in Situ Hybridization' scenarios.
@Emily Johnson | Proper validation of AI models should involve comparing AI-generated results with expert interpretation and established industry norms to ensure accuracy and reliability in 'in Situ Hybridization'.
@Emily Johnson | Researchers should also be cautious about overreliance on AI-generated results and ensure that they are interpreted in conjunction with other experimental and clinical evidence in 'in Situ Hybridization'.
Great question, Emily! AI algorithms are only as good as the data they are trained on. It's crucial to have high-quality data to minimize potential errors and biases.