The Impact of Gemini in Neuroimaging: Unlocking New Insights in Technology
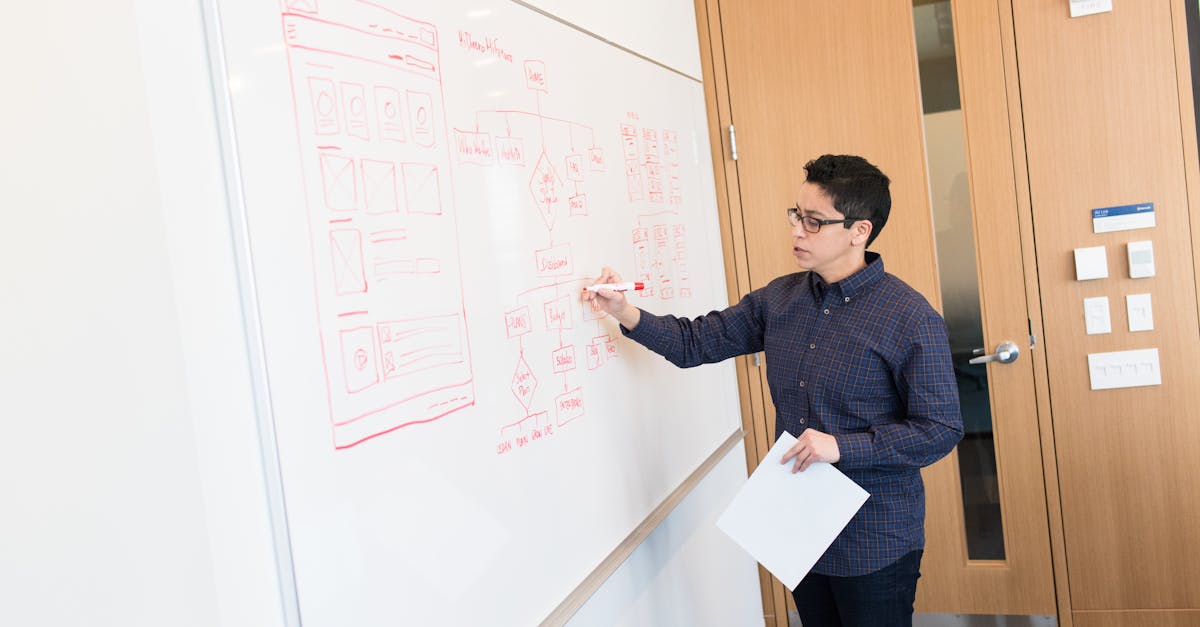
Introduction
Advancements in technology have revolutionized the field of neuroimaging, empowering researchers and medical professionals to explore the human brain like never before. One such technology that has generated significant attention is Gemini – a powerful language generation model trained by Google. This article will examine the impact of Gemini on neuroimaging and discuss how it has unlocked new insights in technology.
Gemini and Neuroimaging
Gemini, built upon the LLM model, utilizes deep learning techniques to generate human-like responses to text prompts. This has proved to be invaluable in the field of neuroimaging as it allows researchers to interact with the model and gain insights into complex brain imaging data. By providing a conversational interface, Gemini enables professionals to explore neuroimaging results more intuitively and efficiently.
Advantages and Applications
One of the fundamental advantages of Gemini in neuroimaging is its ability to aid in data analysis and interpretation. Through the model's conversational nature, researchers can ask targeted questions, refine their hypotheses, and receive detailed explanations. This interaction allows for a deeper understanding of the complex neural patterns and structures detected in neuroimaging scans.
Moreover, Gemini serves as a valuable tool for knowledge consolidation and sharing in the neuroimaging community. Researchers can tap into the vast knowledge stored within the model by asking questions about specific imaging techniques, methodologies, or neurobiological concepts. This democratization of information accelerates research progress and facilitates collaboration among experts in the field.
Unlocking New Insights
Gemini's remarkable language generation capabilities have the potential to unlock new insights in neuroimaging. The model can assist in the identification of subtle brain abnormalities, aiding in the early detection and diagnosis of neurological conditions. Additionally, the exploration of different hypothetical scenarios through chat interactions with Gemini helps researchers investigate the potential implications of their findings and expand their understanding of brain function and dysfunction.
Conclusion
The integration of Gemini into neuroimaging workflows has had a profound impact on the field. By providing a conversational interface, the model enhances data analysis, facilitates knowledge sharing, and unlocks new insights into the intricacies of the human brain. As technology continues to advance, the role of language generation models like Gemini in neuroimaging research will become increasingly significant, fostering breakthrough discoveries and advancements in our understanding of this complex organ.
Comments:
Thank you all for your comments on my article! I'm glad to see such engaging discussions.
This article provides an interesting perspective on the impact of Gemini in neuroimaging. The possibilities of unlocking new insights in technology are exciting. I'm particularly curious about the specific applications in neuroimaging.
@Sarah Thompson Agreed! The potential applications of Gemini in neuroimaging are truly fascinating. Can you provide some examples of how this technology can be utilized in this field?
@Ryan Davis Sure! One example is using Gemini to aid in the analysis of brain imaging data. It can help researchers interpret complex patterns or identify relevant features that may be overlooked. This can potentially enhance diagnostic accuracy and provide valuable insights into neurological disorders.
I found the article insightful and well-written. It's amazing how AI technologies like Gemini are revolutionizing various fields, including neuroimaging.
@Sarah Thompson @Ryan Davis Indeed, the use of Gemini in neuroimaging can greatly speed up the analysis process and improve efficiency. It could assist in tasks like image segmentation, localization of abnormalities, and even predicting treatment responses.
The article highlights the potential of Gemini in neuroimaging, but I wonder about its limitations. How would Gemini handle noisy or incomplete imaging data?
@Michael Clark Great question! While Gemini can contribute to the analysis process, it's important to note that it relies on the quality of input data. Noisy or incomplete imaging data may result in less accurate or unreliable insights. Additional preprocessing steps and validation are essential in such cases.
I think the advancements in AI like Gemini are incredible, but we should still be cautious about over-reliance on such technology in crucial areas like neuroimaging. Human expertise and critical thinking should always be at the forefront.
@Anna Wilson Absolutely, AI technologies should be seen as complementary tools rather than replacements for human expertise. They can assist in analysis and provide new perspectives, but human critical thinking remains crucial.
As a neuroscientist, I'm thrilled to see the potential of Gemini in neuroimaging. It could be a game-changer not only for research but also in clinical settings, bringing more efficiency and accuracy to diagnosis.
@Sophia Lee Exactly! The integration of Gemini in clinical workflows has the potential to streamline diagnosis, improve treatment planning, and potentially reduce healthcare costs.
@Sarah Thompson That sounds very promising! Gemini's ability to identify relevant features and patterns can be valuable, especially considering the vast amount of data in neuroimaging studies. It could potentially uncover hidden correlations and provide deeper insights into brain function.
The implications of Gemini in neuroimaging go beyond just analysis. It could also aid in developing personalized treatment plans and monitoring treatment progress, leading to better outcomes for patients.
While the applications of Gemini in neuroimaging are promising, we should also consider the ethical implications. How do we address challenges related to data privacy and potential biases in the algorithms?
@David Turner Good point! Ethical considerations are crucial when implementing AI technologies. Rigorous safeguards should be put in place to protect patient data privacy, ensure algorithm transparency, and address biases that may arise. Ongoing monitoring and regulation are necessary to avoid any unintended consequences.
@Jorge Conde I appreciate your response! Indeed, robust data privacy measures and algorithmic transparency are crucial to ensure trust in AI technologies used in neuroimaging.
@David Turner Validating and reproducibility are key aspects in any scientific research, and the same rigor should be applied when utilizing Gemini in neuroimaging studies.
That's an exciting prospect, Jorge. Personalized medicine based on neuroimaging data could revolutionize how we approach neurological disorders.
I completely agree, David. AI-driven insights in personalized medicine have the potential to improve patient outcomes and optimize treatment strategies.
I'm intrigued by the applications of Gemini in neuroimaging research. It seems like it could facilitate collaboration among researchers by providing automated insights and aiding in the interpretation of complex imaging data.
@Ryan Davis Collaboration is indeed an important aspect. Gemini could assist in generating hypotheses and facilitating discussions among researchers, allowing them to explore new avenues in their neuroimaging studies.
The integration of Gemini in neuroimaging may also open up opportunities for cross-disciplinary collaborations between AI experts and neuroscientists, leading to even more innovation.
@Liam Johnson Absolutely! Collaborations between different fields can often lead to breakthroughs and novel approaches. The integration of AI in neuroimaging research offers exciting prospects.
One potential concern I have is the interpretability of Gemini's insights in neuroimaging. How can we ensure that the generated explanations are meaningful and reliable?
@Emily Parker Valid concern! The interpretability of AI models like Gemini is a hot topic. It's crucial to develop methods that provide meaningful explanations alongside the predictions. Techniques like attention maps and model-agnostic interpretability can help address this concern.
Jorge, thank you for shedding light on the impact of Gemini in neuroimaging. It's exciting to see how this technology can enhance our understanding of the human brain!
@Emily Parker Interpretability is a valid concern. Development of explainable AI models and methods is essential to ensure the reliability and trustworthiness of Gemini's insights in neuroimaging.
The potential of Gemini in neuroimaging is undeniable. However, it's important to strike a balance between automated analysis and the expertise of human neuroimaging researchers. Combining the strengths of both can lead to more innovative and reliable findings.
@Nathan Foster Absolutely agree! The synergy between AI technologies and human expertise is key. By using Gemini as a tool alongside expert knowledge, we can make significant advancements in the field of neuroimaging.
The wider adoption of Gemini in neuroimaging research would also require addressing challenges related to computational resources and potential biases in training data. Nevertheless, the prospects of this technology are promising.
Considering the ever-increasing complexity of neuroimaging data, the integration of AI technologies like Gemini can be a game-changer in unlocking new insights in technology and advancing our understanding of the human brain.
One interesting aspect is how Gemini can expand accessibility in neuroimaging research. By automating certain analysis tasks, it can potentially empower more researchers, even those with limited expertise in neuroimaging techniques.
The potential for Gemini to assist in personalized medicine by tailoring treatment plans based on neuroimaging data is truly remarkable. It could lead to more effective and targeted interventions.
Considering the growing importance of AI-backed technologies in healthcare, it's essential to ensure rigorous validation and reproducibility of findings when using Gemini in neuroimaging studies.
The strengths of AI technologies should be embraced, but we must also stay vigilant about their limitations. Regular evaluation and validation are crucial to ensure the reliability and accuracy of Gemini in neuroimaging.
Can Gemini be used to integrate data from multiple imaging modalities, such as MRI, fMRI, and EEG, to provide a more comprehensive analysis?
@Michael Clark Integrating data from multiple imaging modalities is an interesting possibility. While Gemini focuses on natural language understanding, it can assist in interpreting the results obtained from different modalities and help correlate findings across various techniques.
@Jorge Conde Integrating data from multiple imaging modalities with Gemini's assistance sounds valuable. It may enable researchers to gain a more comprehensive understanding of brain structure and function.
@Michael Clark That's an excellent point! Gemini could assist in handling noisy or incomplete imaging data by leveraging its capabilities to recognize patterns and fill in information gaps.
@Jorge Conde You're raising essential concerns, Michael. As with any AI-based technology, handling noise or incomplete data is a challenge. Preprocessing techniques and carefully designed algorithms can help mitigate these issues.
The potential applications seem vast, but we should also consider the challenges of bias in AI algorithms. It's critical to ensure diverse and representative training datasets to mitigate any potential biases.
@Emma Martinez Absolutely! Bias mitigation is crucial in AI development. Ensuring diverse and representative training datasets, along with continuous monitoring and evaluation, can help minimize biases and create more equitable AI technologies.
I believe Gemini can also assist in automating repetitive image analysis tasks, freeing up time for researchers to focus on more complex and critical aspects of neuroimaging research.
@Ryan Davis Using Gemini to analyze large-scale neuroimaging datasets can potentially speed up research and aid in discovering novel biomarkers for various neurological conditions.
The integration of AI in neuroimaging research not only enhances analysis capabilities but also offers potential benefits in training future neuroscientists by facilitating access to advanced analysis techniques and insights.
Considering the rapid advancements in AI technologies, it's exciting to think about the possibilities that lie ahead. Integrating Gemini in neuroimaging is just the beginning of a transformative journey.
One area where Gemini can be particularly useful is in data exploration. It can help researchers identify patterns, generate hypotheses, and guide future experiments in neuroimaging.
@Oliver Harris That's a great point! The exploratory power of Gemini combined with neuroimaging data can unlock new avenues of research and deepen our understanding of brain structure and function.
@Oliver Harris Absolutely! Gemini's capability to find patterns and generate hypotheses can be a valuable asset in uncovering hidden insights within complex neuroimaging datasets.
@Emma Martinez Indeed, the potential for AI technologies like Gemini to revolutionize various fields, including neuroimaging, is truly remarkable. Exciting times ahead!
@Emma Martinez Agreed! The advancements we're witnessing are just the tip of the iceberg. The future of neuroimaging research, with the integration of technologies like Gemini, looks incredibly promising.
@Emma Martinez The accessibility aspect is exciting. AI technologies like Gemini can democratize access to neuroimaging tools and insights, enabling more researchers from diverse backgrounds to contribute to the field.
Thank you all for taking the time to read my article on the impact of Gemini in neuroimaging. I'm excited to hear your thoughts and engage in a discussion!
Great article, Jorge! I find it fascinating how Gemini can provide new insights in technology. It's amazing how AI has advanced in recent years.
I agree with Lisa, Jorge. The potential for Gemini in neuroimaging is immense. It can definitely help speed up the analysis process and reveal new patterns in brain images.
I'm not convinced about the reliability of Gemini in neuroimaging. AI algorithms can introduce biases and inaccuracies. How do we address these concerns?
Sarah, you raise a valid point. Bias in AI algorithms is a critical concern. It's important to ensure diverse and representative training data to mitigate biases.
Indeed, Peter. Addressing bias in AI algorithms is crucial for their effective and ethical use in neuroimaging. We need robust evaluation and mitigation strategies.
Gemini seems promising, but I'm curious about its limitations. Are there any specific challenges in applying it to neuroimaging? Great article, by the way!
Thank you, Olivia! There are indeed challenges with using Gemini in neuroimaging. One limitation is its reliance on training data availability. Robust datasets are crucial for accurate results.
Great article, Jorge! The integration of AI in neuroimaging is opening up new possibilities for research and diagnosis.
I completely agree, Mark. AI can revolutionize the field of neuroimaging and help us uncover insights that were previously hidden.
Another challenge is the interpretation of the results produced by Gemini. Sometimes the AI might generate explanations that are difficult to validate or understand.
Valid concerns, Lisa. AI-generated interpretations need to be carefully scrutinized to avoid misleading conclusions or false correlations.
Valid point, Sophia. Human oversight and critical analysis are essential to ensure the reliability and validity of the insights derived from AI algorithms.
I appreciate the discussion on addressing biases and limitations in AI algorithms for neuroimaging. It's crucial to ensure the technology is used ethically and responsibly.
Agreed, Sarah. Ethical considerations should always be at the forefront when developing and deploying AI technologies like Gemini in neuroimaging.
Absolutely, Sarah and Daniel. Responsible development and usage of AI is key to prevent unintended consequences and potential harm.
Indeed, Peter. Developers should actively collaborate with neuroscientists and ethicists to ensure AI algorithms are well-aligned with the needs of the field.
Collaboration between experts is vital to strike a balance between the potential benefits and ethical implications of AI in neuroimaging.
AI algorithms like Gemini are tools that can greatly aid neuroimaging research. However, human expertise and validation are still essential.
Excellent observation, Julia. AI is a powerful tool, but it should complement and be guided by human intelligence, not replace it.
Collaboration should also involve patients, ensuring their consent and privacy are respected when using AI-driven neuroimaging technologies.
You're absolutely right, Sarah. Involving patients in the decision-making process and upholding their privacy rights are crucial elements of responsible AI implementation.
Thanks for your support, David. Patient-centered AI is the way forward to ensure the technology benefits everyone without compromising their rights and well-being.
David, I completely agree. The responsible use of AI in neuroimaging goes beyond technical considerations and must prioritize patient-centricity.
David, you mentioned speeding up the analysis process with Gemini. Can you elaborate on how it achieves that?
Sure, Michelle! Gemini can analyze large volumes of neuroimaging data more quickly than traditional methods. This enables researchers to process and interpret data more efficiently.
I'm fascinated by the potential of Gemini in detecting abnormalities in brain scans. This could significantly improve early detection of neurological disorders.
Indeed, Michelle! Gemini's ability to identify subtle patterns and anomalies in neuroimaging data holds promise for more accurate and timely diagnoses.
Jorge, do you see any potential for Gemini to assist in personalized medicine or treatment planning based on neuroimaging data?
Absolutely, Sophia! Gemini's insights can potentially assist in tailoring treatment plans to individuals based on their unique neuroimaging characteristics, leading to more personalized and effective interventions.
Jorge, I have a question regarding data privacy. How can we ensure the sensitive neuroimaging data used by Gemini remains confidential and secure?
Valid concern, Lisa. When applying Gemini to neuroimaging, it's crucial to establish robust data privacy protocols, including encryption, anonymization, and secure storage to protect sensitive information.
In addition, Gemini's ability to generate automated reports and summaries saves time and allows researchers to focus on analyzing the most critical aspects of the data.
It's heartening to see the potential impact of AI in improving healthcare outcomes. Attention to ethical considerations will be key to realizing its full potential.
Collaboration between neuroscientists and AI experts is crucial to unlock the full potential of Gemini in neuroimaging. Together, they can drive innovation and address challenges.
Agreed, Emily. Interdisciplinary collaborations would allow for a holistic approach to AI-driven neuroimaging research, promoting accuracy and advancing the field.
Neuroimaging research can greatly benefit from cross-disciplinary partnerships. It brings together diverse perspectives and expertise, fostering innovation and enhancing results.
Absolutely, Peter. Collaborative efforts are instrumental in addressing the challenges, reducing biases, and maximizing the positive impact of AI in neuroimaging.
Collaboration between experts is necessary to ensure AI algorithms are developed and used responsibly. It's a complex field that requires collective efforts.
Data privacy and security must be prioritized to build trust in the use of AI algorithms. Strict adherence to privacy regulations and guidelines is essential.
I agree, Daniel. Data privacy should be at the forefront of AI development, ensuring that patients' rights and confidentiality are respected.
Daniel, you highlighted the importance of human intelligence guiding AI algorithms. It's crucial to maintain the right balance for optimal results in neuroimaging.
Indeed, Olivia. AI algorithms can augment human capabilities, but human expertise and judgment remain paramount in neuroimaging research and clinical decision-making.
In addition to robust protocols, thorough informed consent processes should be established to ensure individuals understand the data usage and are comfortable with it.
Human interpretation and critical analysis are pivotal in evaluating AI-generated insights, as they allow for context-specific validation and help prevent potential errors.
I couldn't agree more, Emily. The integration of AI in neuroimaging should not replace the need for human judgment but rather enhance it.
Well said, Lisa. A collaborative approach, where AI and human expertise complement each other, promises to yield the most impactful and accurate insights.