Transforming Data Center Management with ChatGPT: Exploring AI Ops for Enhanced Efficiency and Operational Excellence
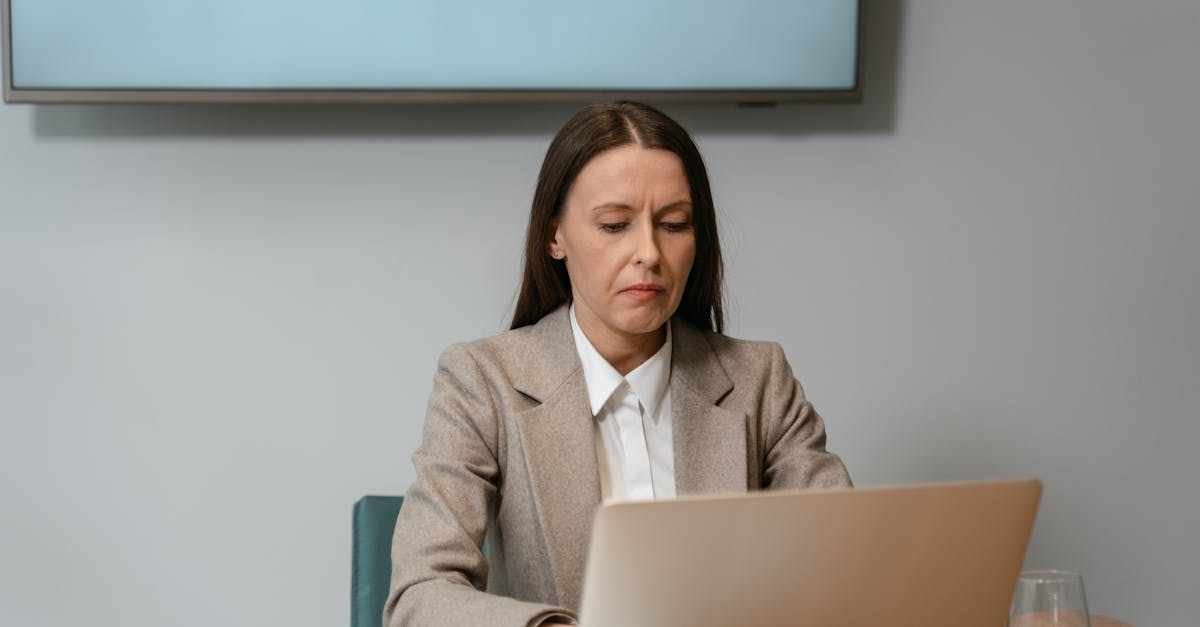
In the rapidly evolving field of technology, managing data centers efficiently and reliably has become increasingly challenging. To tackle this, the application of artificial intelligence (AI) in IT operations, known as AI Ops, has gained significant importance. AI Ops leverages the power of AI to automate and enhance IT operations, leading to improved efficiency, reduced downtime, and enhanced overall performance. As AI continues to advance, one particular technology that stands out is ChatGPT-4, which can revolutionize the way AI Ops is implemented in data center management.
What is AI Ops?
AI Ops refers to the application of artificial intelligence and machine learning techniques to IT operations. It involves using AI algorithms and models to automate, analyze, and predict various IT processes, including monitoring, event correlation, root cause analysis, and incident management. By leveraging AI, organizations can streamline their operations, reduce manual effort, and gain valuable insights to make informed decisions. This ultimately enables data center administrators to proactively manage and maintain their infrastructure with increased efficiency and reliability.
The Role of ChatGPT-4
ChatGPT-4, the latest iteration of OpenAI's chatbot model, has the potential to significantly facilitate AI Ops in data center management. With its advanced natural language processing (NLP) capabilities, ChatGPT-4 can understand and process complex queries, providing accurate responses and insights to data center administrators. Whether it is monitoring the overall health of the data center, troubleshooting issues, or predicting potential problems, ChatGPT-4 can offer real-time assistance and support.
Benefits of ChatGPT-4 in AI Ops
The integration of ChatGPT-4 in AI Ops brings several benefits to data center management:
- Efficient Troubleshooting: ChatGPT-4 can assist in diagnosing and resolving IT issues by analyzing the available data and providing relevant solutions. Its ability to understand context and ask clarifying questions makes it an ideal tool for efficient troubleshooting.
- Automated Alerts and Notifications: ChatGPT-4 can continuously monitor the data center's performance and generate automated alerts in case of any anomalies or issues. This proactive approach enables timely intervention, reducing the risk of downtime.
- Predictive Analytics: By leveraging historical data and machine learning techniques, ChatGPT-4 can provide predictive insights into potential problems that may arise in the data center. This allows administrators to take proactive measures to prevent such issues before they occur.
- Improved Decision Making: ChatGPT-4 can provide data center administrators with valuable recommendations based on data analysis and insights. These recommendations can help in making informed decisions regarding resource allocation, infrastructure enhancements, and capacity planning.
Future Potential and Challenges
While ChatGPT-4 shows immense potential in facilitating AI Ops in data center management, there are certain challenges that need to be addressed. Ensuring the accuracy and reliability of the responses produced by the model is crucial, as incorrect recommendations can have significant consequences. Additionally, maintaining data privacy and security is of utmost importance when integrating AI chatbots into data center environments.
Nonetheless, as AI technologies continue to evolve and improve, the future of AI Ops looks promising. With advancements in NLP and machine learning, chatbots like ChatGPT-4 will become even more powerful, offering seamless support and insights for efficient data center management.
Conclusion
ChatGPT-4 has the potential to revolutionize AI Ops in data center management. Its ability to understand and respond to complex queries, provide automated alerts, and offer predictive analytics makes it a valuable tool for data center administrators. By leveraging AI chatbots like ChatGPT-4, organizations can enhance the efficiency and reliability of their IT operations, leading to improved performance and reduced downtime.
Comments:
Thank you all for joining the discussion! I'm glad to see the interest in using AI Ops for data center management. Feel free to share your thoughts and questions.
Great article, Brian! AI Ops definitely has the potential to transform data center management. I'm particularly interested in how it can enhance operational efficiency. Can you provide some examples?
Absolutely, David! One major benefit is predictive analytics, where AI algorithms analyze data to identify patterns and anticipate issues before they occur. This helps in proactive maintenance planning and reducing downtime.
Predictive analytics sound promising, Brian. But how accurate can AI Ops be in identifying potential issues? Are there any limitations or challenges?
Great question, Sarah! AI Ops relies on historical data and learning from previous incidents. However, it may face challenges with rare or unforeseen events that haven't been encountered before. Continuous improvement and human oversight are crucial to address these limitations.
I agree, Brian. AI Ops can bring significant improvements, but human expertise should never be overlooked. There will always be scenarios where human intervention and judgment are necessary.
Well said, Mark. The goal of AI Ops is to augment human capabilities, not replace them. Combining the power of AI with human experience and decision-making is essential for successful data center management.
I'm curious about the implementation process. How complex is it to integrate AI Ops into existing data center management systems?
Good question, Jennifer. The complexity depends on various factors such as the existing infrastructure, data sources, and AI models used. Generally, it requires careful planning, data integration, model training, and continuous refinement. Working with experienced professionals can streamline the implementation process.
Brian, what about the ethical implications of AI Ops? How can organizations ensure ethical use of AI in data center management?
Good point, Jennifer. Organizations should establish clear ethical guidelines and frameworks for AI Ops. Transparency in decision-making, accountability, and regular audits can help ensure ethical use. Additionally, involving diverse perspectives and avoiding biased data inputs are essential considerations.
Brian, do you have any recommendations for organizations planning to adopt AI Ops? What steps should they take to ensure a successful transition?
Certainly, Carlos. Organizations should start by clearly defining their goals and understanding the specific data center management challenges they want to address. It's important to involve key stakeholders, assess data readiness, and gradually pilot and scale AI Ops initiatives. Regular evaluation and feedback loops are crucial for continuous improvement.
I see the potential benefits, but what about the potential risks? Are there any security concerns associated with AI Ops in data center management?
Excellent point, Emma. Security is a top concern when it comes to AI Ops. Implementing proper data security measures, robust authentication mechanisms, and regular vulnerability assessments are essential to mitigate potential risks. Security should always be an integral part of AI Ops strategies.
I believe AI Ops can also play a significant role in optimizing resource allocation within data centers. It can help identify underutilized resources and make recommendations for efficient utilization. This could bring cost savings as well. What are your thoughts, Brian?
Absolutely, Robert! AI Ops can analyze resource usage patterns and provide recommendations for optimal resource allocation. By avoiding overprovisioning and ensuring efficient utilization, organizations can reduce costs and maximize the value they get from their infrastructure.
Brian, how can organizations address security concerns while ensuring data accessibility and availability for AI Ops?
I'm intrigued by the potential of AI Ops. Are there any real-world examples of organizations that have successfully implemented it for data center management?
Definitely, Joanna. Many organizations across various industries have embraced AI Ops for data center management. A notable example is Google, which utilizes AI algorithms to optimize power usage and cooling systems in their data centers. Their efforts have resulted in significant energy savings and improved efficiency.
Brian, are there any specific industry sectors that you believe will benefit the most from AI Ops in data center management?
Joanna, while AI Ops can benefit organizations across industries, sectors with large and complex data center infrastructures, such as finance, healthcare, and technology, are likely to see significant advantages. The ability to optimize resource utilization, ensure high availability, and proactively address potential issues is particularly valuable in those sectors.
Brian, what kind of skill sets are required to implement and manage AI Ops effectively? Are there any specific roles or expertise an organization needs to focus on?
That's a great question, Victor. Organizations should have skilled professionals who understand both data center operations and AI technologies. Data scientists, AI engineers, and system administrators with experience in machine learning and data management are essential for effective implementation and ongoing management of AI Ops.
I'm impressed by the potential impact of AI Ops, but what about the costs involved? Is it affordable for all organizations, including smaller ones?
Valid point, Alexandra. Implementing AI Ops does involve costs, including infrastructure, AI model development, and training. However, the benefits in terms of improved efficiency, reduced downtime, and optimized resource utilization can outweigh the investment. Smaller organizations can start with pilot initiatives and gradually scale up as they see positive outcomes.
Brian, how does AI Ops handle dynamic environments and rapidly changing workloads? Can it adapt quickly to such scenarios?
Great question, Chris. AI Ops is designed to handle dynamic environments by continuously monitoring and analyzing data. By quickly adapting to changes in workloads, it can provide real-time insights and recommendations. However, it's important to regularly calibrate models and retrain them to ensure accurate assessments in rapidly changing scenarios.
Hi Brian, does AI Ops support multi-cloud environments? Many organizations rely on multiple cloud providers, so it would be helpful to know if AI Ops can integrate and manage such setups.
Hi Jessica, AI Ops can indeed support multi-cloud environments. It can integrate data from various sources, including multiple cloud providers, and enable centralized monitoring and management. This allows organizations to gain a holistic view of their data center operations across different cloud environments.
Brian, what kind of AI models or algorithms are commonly used for AI Ops in data center management? Are there any industry-standard approaches?
Hi Liam, common AI models used for AI Ops include anomaly detection algorithms, regression models, and decision trees. Machine learning techniques like supervised and unsupervised learning are widely applied. However, the specific models and algorithms used can vary based on the organization's requirements, data availability, and desired outcomes.
I'm concerned about potential biases in AI Ops. How can organizations ensure fairness and avoid biased decision-making when implementing AI in data center management?
Valid concern, Jake. To ensure fairness, organizations should be mindful of the data used for training AI models. Biases can be mitigated by regularly assessing and auditing the models for disparate impacts and continuously refining them. Transparency, explainability, and ongoing evaluation are key to avoiding biased decision-making in AI Ops.
Brian, what steps can organizations take to ensure transparency and explainability of AI Ops decisions?
Brian, what are some key performance indicators (KPIs) that organizations should consider when evaluating the effectiveness of AI Ops in data center management?
Good question, Sophia. Organizations can consider KPIs like mean time to detect anomalies, mean time to resolve incidents, reduction in equipment failure rates, energy efficiency improvements, and overall cost savings. These metrics help assess the impact and effectiveness of AI Ops in data center management.
Brian, in terms of ongoing management and updates, how often should AI models be retrained to maintain accuracy in AI Ops?
Sophia, the frequency of retraining AI models depends on the specific use case, data characteristics, and the rate of change in the environment. In dynamic scenarios, models may need more frequent updates, while in stable environments, periodic recalibration can be sufficient. Regular evaluation and monitoring help determine the optimal retraining intervals.
Are there any regulations or compliance considerations that organizations should be aware of when implementing AI Ops in data center management?
Absolutely, Emma. Depending on the industry and the data involved, organizations may need to comply with regulations like GDPR, HIPAA, or PCI DSS. It's crucial to ensure that AI Ops implementations align with industry-specific regulations and comply with data privacy and security requirements.
Brian, what are your thoughts on the future of AI Ops in data center management? How do you see it evolving in the coming years?
Great question, Mike. The future of AI Ops looks promising. We can expect further advancements in AI algorithms, integration with edge computing, and the use of real-time streaming data for enhanced insights. As technology evolves, AI Ops will continue to play a crucial role in driving efficiency and operational excellence in data center management.
Brian, for organizations looking to train their staff in AI Ops, what are the key areas they should focus on?
Lauren, organizations should focus on providing training in areas like data analysis, machine learning algorithms, cloud computing, and programming languages used in AI Ops. It's important to develop a strong foundational understanding of AI concepts along with practical skills for data center management.
Brian, how can AI Ops improve disaster recovery processes in data centers?
Brandon, AI Ops can play a crucial role in disaster recovery. By continuously monitoring data center operations, it can quickly detect anomalies and issue alerts. This enables faster response times and helps mitigate potential damages. Additionally, AI Ops can automate recovery processes and provide recommendations for efficient restoration of services.
Brian, what kind of ROI can organizations expect from implementing AI Ops?
Emily, the ROI of implementing AI Ops can vary depending on factors such as the organization's size, infrastructure complexity, and specific goals. However, organizations can expect improved operational efficiency, reduced downtime, optimized resource utilization, and potential cost savings. It's essential to carefully measure and track the impact to assess the ROI specific to each organization.
I think organizations should focus on implementing robust access controls, encryption mechanisms, and secure data storage practices. Balancing security and accessibility is crucial, and organizations should ensure that proper protocols are in place while enabling necessary access for AI Ops.
To ensure transparency, organizations should focus on using interpretable AI models, documenting decision-making processes, and providing clear explanations for AI Ops recommendations or actions. Mechanisms like model documentation, logging, and audit trails can help achieve explainability. Additionally, involving domain experts in decision-making can enhance transparency.