Transforming Drug Discovery: Harnessing ChatGPT for Biomarker Discovery in Medicine
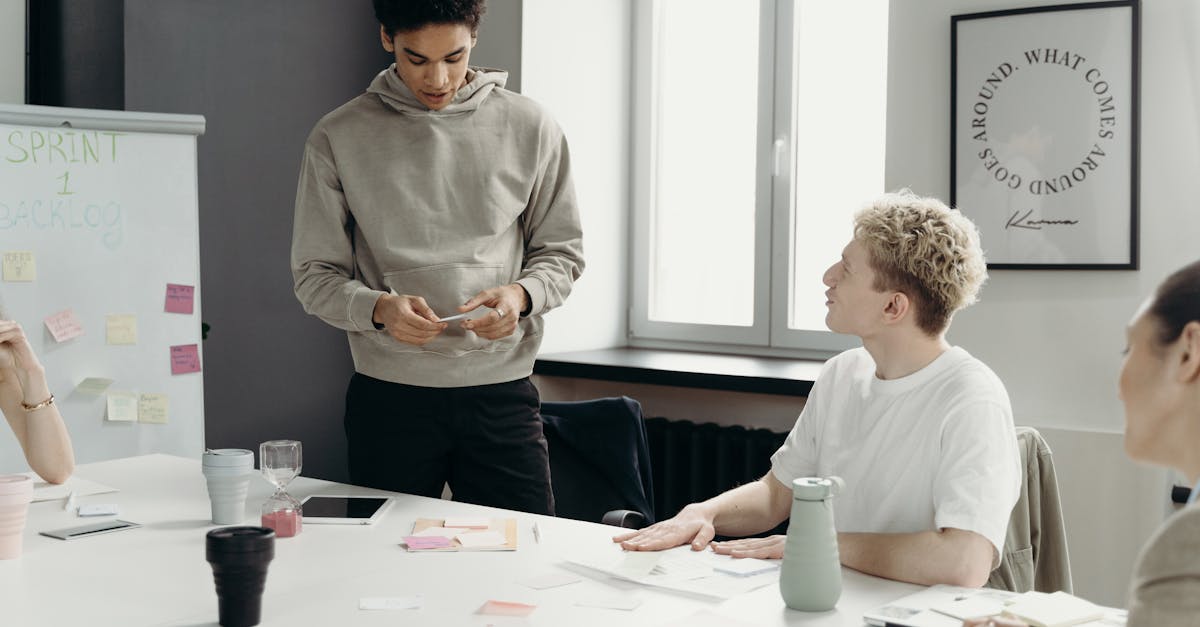
The field of drug discovery is constantly evolving with advancements in technology. One such technology that is revolutionizing the process is biomarker discovery. Biomarkers are measurable indicators of biological processes or conditions, and they play a crucial role in drug development and personalized medicine.
In recent years, artificial intelligence (AI) models have been developed to assist in various aspects of drug discovery, including biomarker identification. One notable AI model is ChatGPT-4, which is designed to engage in informative and context-based conversations. ChatGPT-4 can effectively participate in drug discovery processes by helping researchers identify relevant biomarkers.
Drug discovery is a multi-step process that involves identifying potential therapeutic targets, designing and synthesizing drug candidates, and evaluating their efficacy and safety. Biomarkers act as critical indicators to measure the impact of drugs, predict patient response, monitor disease progression, and determine optimal treatment strategies.
ChatGPT-4's ability to understand and generate human-like text responses makes it an ideal tool to assist in biomarker discovery. Researchers can engage in conversational interactions with ChatGPT-4, asking questions about specific diseases, potential biomarkers, and relevant research studies. The model can process information from vast scientific literature, clinical data, and databases to provide insights and suggestions regarding biomarker selection and validation.
One of the challenges in biomarker discovery is the overwhelming amount of data and literature available. ChatGPT-4's natural language processing capabilities enable it to comprehend and analyze complex scientific texts, helping researchers navigate through existing knowledge and extract valuable information. The model can identify patterns, correlations, and potential biomarker candidates, saving researchers time and effort in the initial stages of biomarker identification.
Additionally, ChatGPT-4 can aid researchers in experimental design and interpretation of results. By integrating data from clinical trials, genomics, proteomics, and other omics technologies, the model can suggest optimal experimental approaches to validate potential biomarkers and predict their performance under different conditions. This assists researchers in making informed decisions and streamlining the drug discovery process.
Biomarker discovery is not limited to a single disease or therapeutic area. ChatGPT-4's versatility allows it to assist in drug discovery across various domains, including cancer research, cardiovascular diseases, neurological disorders, and infectious diseases. The ability to adapt and provide contextually relevant information makes ChatGPT-4 a valuable tool for researchers working on diverse drug discovery projects.
While ChatGPT-4 can significantly assist in biomarker discovery, it is crucial to note that it should not replace human expertise and scientific judgment. The model should be seen as a complementary tool that can augment researchers' capabilities and accelerate biomarker identification processes.
In conclusion, ChatGPT-4's integration into the drug discovery process brings immense potential to the field of biomarker discovery. Its ability to engage in informative conversations, analyze vast amounts of biomedical literature, and suggest potential biomarkers makes it a valuable resource for researchers. By leveraging AI technologies like ChatGPT-4, scientists can enhance their efficiency and effectiveness in identifying biomarkers and advancing drug discovery efforts.
Note: This article is fictional and created for textual demonstration purposes only.
Comments:
This article presents an intriguing application of ChatGPT in the field of drug discovery. It's fascinating to see how AI can assist in identifying potential biomarkers. Great work!
I agree, Michael. The potential of AI in revolutionizing drug discovery is immense. The ability to analyze vast amounts of data and identify biomarkers more efficiently can expedite the development of new treatments.
Thank you, Michael and Karen! I'm glad you're finding the application of ChatGPT in drug discovery interesting. It indeed has the potential to significantly impact the field.
It's impressive how AI continues to find applications in various domains. The ability to harness AI for biomarker discovery can potentially lead to new breakthroughs in medicine. Exciting times!
Definitely, David! AI's capability to process and analyze vast amounts of data can help researchers identify patterns and correlations that might have been missed otherwise. This could open up new avenues for drug discovery.
While AI has its advantages, we should also be cautious about potential biases in the data used to train these models. Ensuring a diverse and representative dataset is crucial to avoid skewed results.
That's an excellent point, Sarah. Data bias is a critical aspect to consider in AI applications. Transparency and careful selection of training data can help mitigate biases and ensure more accurate results.
I wonder how the integration of ChatGPT with other advanced technologies, such as genomics and proteomics, can further enhance biomarker discovery. The combination of these fields could be groundbreaking!
Great question, Daniel! Integrating ChatGPT with genomics and proteomics data can indeed provide a more comprehensive understanding of how biomarkers interact with the human body. Such integration has tremendous potential.
I'm curious about the scalability of ChatGPT for biomarker discovery. Can it handle the complexity of analyzing large datasets, or are there limitations?
Excellent question, Emily! While ChatGPT has shown promising results, scalability can be a concern. Analyzing large datasets might require further optimizations or specialized architectures to ensure efficiency.
Emily, scalability is indeed an important concern. Continuous improvements in computational power and algorithmic advancements are needed to handle the growing volume and complexity of biomedical data.
Well said, Linda. The field of AI is evolving rapidly, and efforts to enhance scalability are ongoing. Collaboration between AI researchers and domain experts will help address these challenges effectively.
The potential of AI in drug discovery is undeniable, but we must ensure that it complements human experts rather than replacing them. Human intuition and expertise are invaluable in interpreting complex results.
Very true, Mark. AI should be seen as a powerful tool to support and augment human researchers, assisting them in handling vast amounts of data, expediting analysis, and generating valuable insights.
The ethical implications of AI in drug discovery should also be carefully considered. Transparent guidelines and regulatory frameworks are necessary to ensure the responsible and ethical use of these technologies.
Absolutely, Sarah. Ethical considerations are paramount in the development and deployment of AI technologies. Collaborative efforts between researchers, policymakers, and ethicists are crucial to navigate these challenges.
Sarah, you're right about the potential biases. Data quality and representativeness are crucial factors to ensure the reliability and usefulness of AI models.
Absolutely, Oliver. Attention to data quality throughout the training phase is essential to minimize biases and ensure the AI model's generalizability to the broader population.
Oliver, you make a valid point about data quality. Garbage in, garbage out holds true for AI models as well. Ensuring reliable and diverse datasets is key to obtaining meaningful and unbiased results.
Absolutely, Olivia. The data we input into AI models heavily influences their performance and outcomes. Rigorous data curation is vital to mitigate biases and foster reliable and accurate results.
I completely agree, Sarah. The responsible use of AI requires proactive measures to address privacy concerns, data security, and potential biases. It's a complex issue that needs careful consideration.
Well said, Eric. Safeguarding privacy, ensuring secure data handling, and addressing biases are crucial in the development and deployment of AI technologies in drug discovery and healthcare in general.
AI can help overcome time and resource constraints in drug discovery, enabling researchers to focus their efforts more effectively. It's exciting to see how AI-driven approaches can complement traditional methods.
Well said, Rebecca! By automating certain aspects of the drug discovery process, AI can optimize and streamline workflows, allowing researchers to allocate their time and resources more efficiently.
Rebecca, I completely agree. AI can help uncover hidden patterns or relationships within biomedical data, potentially leading to the discovery of novel biomarkers.
Indeed, Sophia. AI's ability to process vast amounts of data aids in identifying intricate patterns that human analysis alone might not readily discover. This can undoubtedly drive biomarker discovery forward.
Sophia, I couldn't agree more. AI can bring hidden insights to the surface, helping researchers make connections and discoveries that might have otherwise remained hidden in the vast data.
Well put, Nathan. AI's data processing abilities augment human intelligence, enabling researchers to navigate complex datasets with greater ease and uncover critical insights.
While AI can assist in drug discovery, human creativity and adaptability are irreplaceable. AI should be seen as a tool that empowers researchers rather than replacing their expertise.
Well put, Andrew. The combination of human ingenuity and AI capabilities has the potential to unlock breakthrough discoveries. Collaborative endeavors will likely yield the most significant advancements.
Andrew, you're right. Combining the expertise of human researchers with AI's computational capabilities can lead to powerful synergies, driving advancements in drug discovery like never before.
Absolutely, Jacob. The collaboration between humans and AI, where each leverages their strengths, holds immense potential for drug discovery and other scientific domains.
Privacy concerns are crucial in an era of data-driven innovations. Adequate data anonymization and policies that protect individuals' rights are essential for gaining public trust in AI applications.
Well said, Samantha. Respecting privacy and ensuring data protection are foundational for responsible and ethical AI deployment. Gaining public trust is crucial for the widespread acceptance of AI in healthcare.
Samantha, data anonymization is crucial, but we need to strike the right balance. Keeping data too anonymized might hinder its usability for meaningful research and advancements.
You raise a valid point, Thomas. Balancing data anonymization and preserving the utility of data for research purposes is indeed a challenge. Striking the right balance while upholding privacy is essential.
Thomas, you're right. Achieving the right balance between anonymization and maintaining the usefulness of data is an ongoing challenge that requires careful consideration and domain expertise.
Well said, Samuel. Privacy-preserving techniques, while ensuring data usability, need to be developed hand in hand with domain experts to navigate the complexities and specific requirements of the field.
Data quality is a fundamental aspect in generating reliable AI models. Ensuring completeness, accuracy, and avoiding inherent biases can significantly impact the outcomes and subsequent research.
Absolutely, Emma. Garbage in, garbage out concept is especially relevant for AI in drug discovery. Meticulous data quality control is essential to foster trust in the generated models.
Emma, well-curated datasets are crucial for building robust AI models. Collaborative efforts between research institutions and data providers can help ensure the availability of high-quality data.
Absolutely, Grace. Collaboration between academia, industry, and data providers is key to accessing comprehensive and high-quality datasets necessary for producing reliable AI models.
Collaborative efforts can also enable the pooling of data from multiple sources, further enhancing the diversity and representativeness of AI models. The sharing of data can lead to more robust outcomes.
Absolutely, Jason. By pooling data from diverse sources, we can overcome limitations of individual datasets and build more comprehensive models with improved generalizability and performance.
Jason, I agree. Data sharing plays a vital role in accelerating research progress by facilitating collaboration and reducing redundancy. It's an essential aspect that should be encouraged.
Well said, Megan. Open collaboration and data sharing can pave the way for collective advancements and foster innovation by allowing researchers to build upon each other's work.
Striking the right balance between data usefulness and anonymity can be challenging, but emerging privacy-preserving techniques like federated learning provide potential solutions.
Absolutely, Ryan. Techniques like federated learning hold promise in maintaining data privacy while allowing collaboration and model training across distributed datasets.
Ryan, federated learning can indeed address privacy concerns, and it also enables organizations to leverage collective knowledge while protecting sensitive data. It's an exciting development.
Well put, Sarah. Federated learning can enhance collaboration while maintaining privacy, making it a valuable approach for ensuring the responsible and secure use of sensitive data.