Transforming Hate Speech Detection in Criminology: Harnessing the Power of ChatGPT
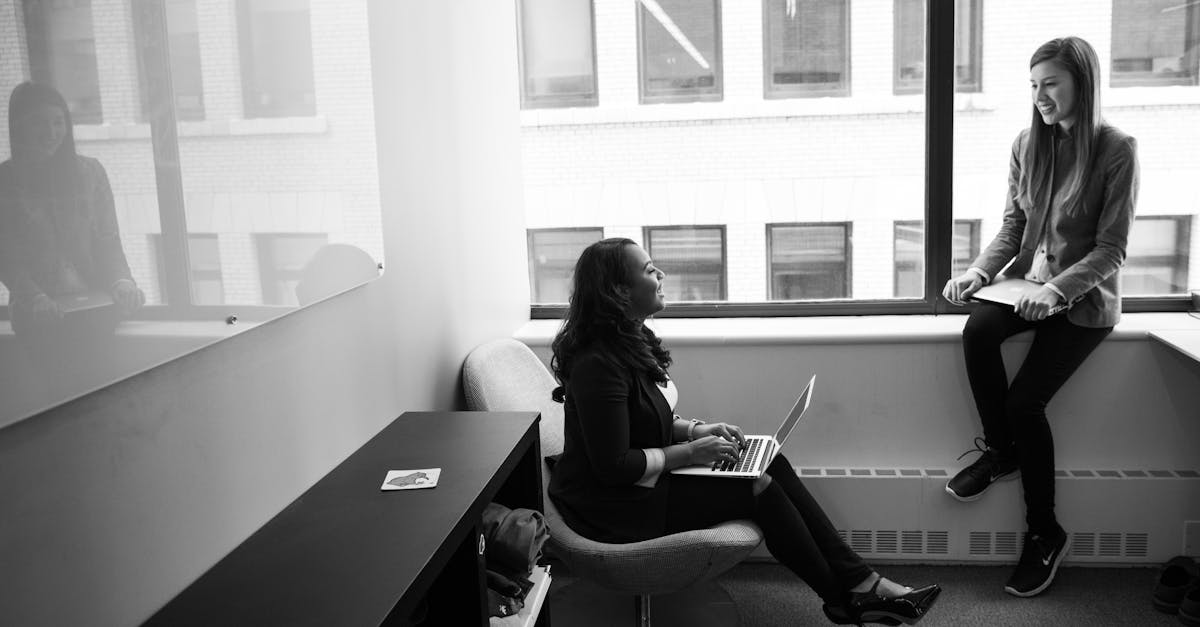
In the age of advancing technology and widespread internet access, hate speech and online harassment have become significant concerns in society. Criminology, the scientific study of criminal behavior, has now turned to the use of technology to address this issue.
One technological tool that has seen significant development in recent years is hate speech detection. This technology utilizes natural language processing techniques and machine learning algorithms to monitor online platforms for instances of hate speech and other forms of online harassment.
By analyzing large amounts of text data, hate speech detection algorithms can identify patterns and linguistic cues that indicate hateful or abusive language. These algorithms can be trained on datasets that have been manually labeled by human reviewers, allowing them to learn and improve over time.
The usage of hate speech detection technology has several benefits in the field of criminology:
- Early intervention: Hate speech detection enables early identification of individuals or groups engaging in hate speech, allowing law enforcement agencies or platform moderators to take appropriate action.
- Prevention of harm: By detecting hate speech on online platforms, this technology can help prevent the spread of harmful ideologies or violent behavior.
- Research and analysis: Criminologists can use the data collected through hate speech detection to gain insights into the prevalence and characteristics of hate speech, contributing to a better understanding of this criminal behavior and its impact on society.
- Public safety: Monitoring online platforms for hate speech can contribute to creating a safer online environment for individuals, particularly those who are vulnerable to targeted harassment.
However, the use of hate speech detection technology also raises ethical concerns. Determining what constitutes hate speech is a complex task that requires careful consideration of context and intent. There is a risk of false positives and the potential for censorship of free speech. Therefore, the deployment of hate speech detection algorithms must be accompanied by human oversight and a thorough understanding of cultural nuances.
In conclusion, hate speech detection technology has emerged as a valuable tool in the field of criminology. Its usage allows for the monitoring of online platforms, aiding in the identification and prevention of hate speech and online harassment. However, ethical considerations and human oversight are crucial to ensure the responsible and effective use of this technology. By combining the power of technology with human expertise, we can strive towards creating a safer and more inclusive digital space.
Comments:
Thank you all for your interest in this topic. I'm glad to see so many engaged in discussing hate speech detection and the potential of ChatGPT. Let's dive into the conversation!
This article is quite informative. The advancements in AI-driven technology like ChatGPT provide promising possibilities. But what about the ethical concerns related to relying solely on AI to detect hate speech? Humans have biases too, and an algorithm is only as good as the data it's trained on.
@Emily Brown, you make a fair point. While AI can help streamline hate speech detection, we should definitely be cautious about placing full reliance on it. A human-in-the-loop approach, where AI aids human moderators, could be a more balanced way to address the biases and limitations.
I agree with both @Emily Brown and @David Carter. AI can assist in efficiently monitoring a vast amount of user-generated content, but combining it with human oversight is crucial to avoid potential pitfalls. Human judgment and contextual understanding are essential elements in determining hate speech, which AI alone might struggle with.
@Sarah Thompson, I agree that humans play a vital role in identifying and understanding hate speech. But AI can act as a valuable tool to alleviate the burden on human moderators who deal with massive amounts of user-generated content daily. It can prioritize content for review, flag potential issues, and detect patterns that may not be immediately apparent to humans.
@Peter Adams, you're right. AI has the potential to augment human efforts, not replace them. By assisting moderators, AI can help increase efficiency and reduce response times, allowing for more effective moderation of user-generated content.
@Emily Brown, I completely agree. Holistic strategies are key. Combining AI tools, education, and a supportive online environment is the best approach to tackle hate speech comprehensively.
@David Carter, well said. AI is a powerful tool, but it cannot replace the essential human touch. By combining AI algorithms with human expertise, we can effectively combat hate speech while respecting freedom of speech and maintaining a healthy online environment.
@Sarah Thompson, well put. Balancing AI automation with human judgment ensures a nuanced and fair approach. Moderators can provide the necessary context and evaluate the intent behind the speech, which is challenging for AI algorithms alone.
@Sarah Thompson, AI can indeed help moderators prioritize their efforts, especially when dealing with large quantities of content. By highlighting potential issues, AI enables humans to focus on nuanced areas where their expertise is most valuable.
@Sarah Thompson, I agree. AI can be an invaluable tool in prioritizing content efficiently and bringing attention to potential issues for a human moderator's final assessment. Collaboration between AI and human judgment is necessary for effective moderation.
@Sarah Thompson, precisely. AI can help identify potential cases, but it is essential to have human moderators making informed decisions, considering the nuances and contextual details that AI algorithms can struggle with.
@Emily Brown and @David Carter, I appreciate your concerns. You're right that collaboration between AI and human expertise is essential. A combination of human and machine intelligence can lead to better outcomes in hate speech detection, minimizing both false positives and negatives.
@Peter Adams, you're right about the burden on human moderators. AI can help alleviate some of that burden by flagging potential issues, allowing moderators to focus their efforts where they're most needed.
@Emily Brown, I couldn't agree more. AI should be seen as an ally, aiding human moderators, rather than as a replacement for human judgment. By working together, we can enhance hate speech detection while respecting the complexities of the task.
We should also consider that hate speech can evolve and adapt quickly. AI models need continuous retraining to keep up with the changing landscape. It's an ongoing effort that requires constant updates and collaboration between AI developers and human experts.
Absolutely, @Michael Johnson. The dynamic nature of hate speech makes it challenging to create a universally accurate model. Continuous improvement and adapting to new patterns are vital to effectively combat hate speech in real-time.
@Sophia Miller, you mentioned the importance of adaptability. Combating hate speech requires staying ahead of its ever-evolving nature. Regular updates and collaboration between AI developers and experts can help in promptly addressing new trends and patterns.
While AI can help identify explicit hate speech, it might struggle when dealing with more subtle forms, like microaggressions. Detecting the tone, intent, and context of such instances can be difficult for AI algorithms. So, human involvement remains crucial.
@Connor Harris, you raise an important point. Microaggressions are complex and require nuanced understanding. Although AI still has limitations in this area, it can act as a useful first filter, helping human moderators focus on more challenging cases. It's about finding the right balance between automation and human judgment.
I have concerns about potential biases in AI models when it comes to detecting hate speech. If the training data isn't diverse enough, the model could inadvertently amplify existing biases or fail to recognize new manifestations. Ensuring inclusivity and diversity in the training process is crucial.
@Emma Rodriguez, you're correct. Bias in AI models is a significant concern. It's crucial to curate diverse training data and incorporate rigorous evaluation processes to minimize bias as much as possible. Combining diverse datasets and involving people from different backgrounds in the training phase are steps in the right direction.
@Thomas Canaple, regular evaluation and improvement indeed play a critical role. Transparency in model development and involving external audits can help address potential biases and enhance the overall performance and reliability of AI-driven hate speech detection systems.
@Emma Rodriguez, comprehensively analyzing and recognizing microaggressions in various forms across different linguistic and cultural contexts is undoubtedly a complex task. Continuous training and human involvement are crucial to improving AI models' accuracy in detecting such subtleties.
@Sophie Evans, precisely. Hate speech is not confined to certain cultures or languages. Embracing inclusivity in AI models is paramount to ensure a shared understanding and addressing hate speech effectively.
@Emma Rodriguez, transparency in AI models is crucial. Open-sourcing the models and involving external audits can help identify biases and make necessary improvements to ensure fair and accurate hate speech detection.
@Michael Johnson, awareness of potential biases is key. AI developers should actively work to debias the models and involve experts from various domains for a comprehensive approach.
@Michael Johnson, regular updates are critical to stay ahead. Hate speech is continually evolving, with perpetrators finding new ways to express their harmful views. AI developers and experts should collaborate closely to adapt the detection algorithms accordingly.
@Thomas Canaple, external audits and independent evaluations can indeed play a vital role in ensuring AI models used for hate speech detection are fair, transparent, and effective.
@Emma Rodriguez, I completely agree with your concern. Without proper representation in the training data, AI models can perpetuate existing biases or fail to recognize certain forms of hate speech. An inclusive dataset, encompassing diverse perspectives, is vital to ensure fairness and accuracy.
The potential of ChatGPT in hate speech detection is undoubtedly fascinating. But what about false positives and negatives? How reliable can we expect AI algorithms to be in such complex tasks?
@Oliver Wilson, that's a valid concern. Achieving perfect accuracy is challenging, and false positives and negatives are unavoidable to some extent. However, AI tools can continuously learn from the feedback of human moderators, reducing errors over time. Regular evaluation and improvement based on real-world performance are crucial.
It's important to note that hate speech is not limited to one language or culture. The training data must incorporate diverse languages and contexts to be effective on a global scale. Otherwise, the AI algorithms might be biased toward certain regions or communities.
@Sophie Evans, absolutely! Building multilingual and culturally sensitive models is crucial to address hate speech comprehensively. Expanding the training data to cover diverse languages and regions will enhance the model's effectiveness across different contexts.
@Thomas Canaple, you're right about finding the balance. AI can help improve efficiency, especially in detecting explicit hate speech. When combined with human judgment, the system becomes more effective, addressing both the clear-cut cases and subtler, nuanced instances of hate speech.
@Sophia Miller and @Thomas Canaple, indeed, collaboration between AI and human judgment is key. AI can assist in flagging potential cases, but human moderators should retain the final say, ensuring decisions are carefully evaluated through a broader lens.
@Connor Harris, you make a valid point. Subtle forms of hate speech can be challenging to detect, even for humans. Training the AI models to recognize such microaggressions accurately will be essential, alongside human moderation.
@Thomas Canaple, incorporating diverse datasets is essential, but we should also be careful about potential biases present within those datasets. Bias mitigation techniques, like debiasing methods and transparency in model development, can help create fairer and more reliable models.
@Michael Johnson, I couldn't agree more. Hate speech is not static, and our models should adapt accordingly. Regular updates and collaboration will enable the detection algorithms to keep up with new trends and emerging forms of hate speech.
@Oliver Wilson, while AI algorithms might not achieve perfect accuracy, they can gradually improve with continual feedback and fine-tuning. Striving for improvement and learning from mistakes can help increase the reliability of these algorithms over time.
@Isabella Clark, inclusivity in training data is key. It helps avoid biases, minimizes false positives, and ensures fair treatment across different demographics. AI models should continuously learn from a diversified dataset and promote inclusivity rather than amplifying discrimination.
@Oliver Wilson, collaboration between AI developers and experts across various disciplines will lead to models that are more robust, inclusive, and less prone to biases.
@Liam Watson, I completely agree. Prevention is the best solution in the long run. Promoting inclusive, respectful online behavior and fostering understanding among users are crucial to tackling hate speech at its core.
@Sophie Evans, you bring up an essential point about including diverse languages in the training data. It's vital to ensure that AI models can effectively detect hate speech across different linguistic and cultural contexts, making the internet a safer space for everyone, regardless of their background.
@Maria Sanchez, precisely! The internet connects people from diverse backgrounds and cultures, and our hate speech detection models should reflect that diversity to ensure accurate identification of harmful content.
I'm glad to see the cautious optimism here. AI can certainly augment hate speech detection, but it should be part of a broader strategy that encourages responsible online behavior, educates users, and fosters inclusion. We need a multi-pronged approach to tackle the root causes as well.
Well said, @Emily Brown. AI is just one piece of the puzzle. Combining it with education, community engagement, and effective moderation policies can create a safer and more inclusive online environment.
Hate speech detection is undoubtedly important, but we must not forget the significance of proactive measures. Emphasizing tolerance, inclusivity, and fostering respectful online discussions can help prevent hate speech from appearing in the first place.
@Susan Lee, proactive measures are key in creating a safe online space. Combating hate speech requires a holistic approach, involving users, platform policies, and even formal education on promoting respectful and inclusive online interactions.
@Liam Watson, you're absolutely right. Collaboration between AI experts, domain experts, and human moderators will help keep the hate speech detection systems up-to-date and effective, constantly adapting to new ways hate speech can manifest.