Transforming Medical Image Analysis in Life Sciences: Harnessing the Power of ChatGPT
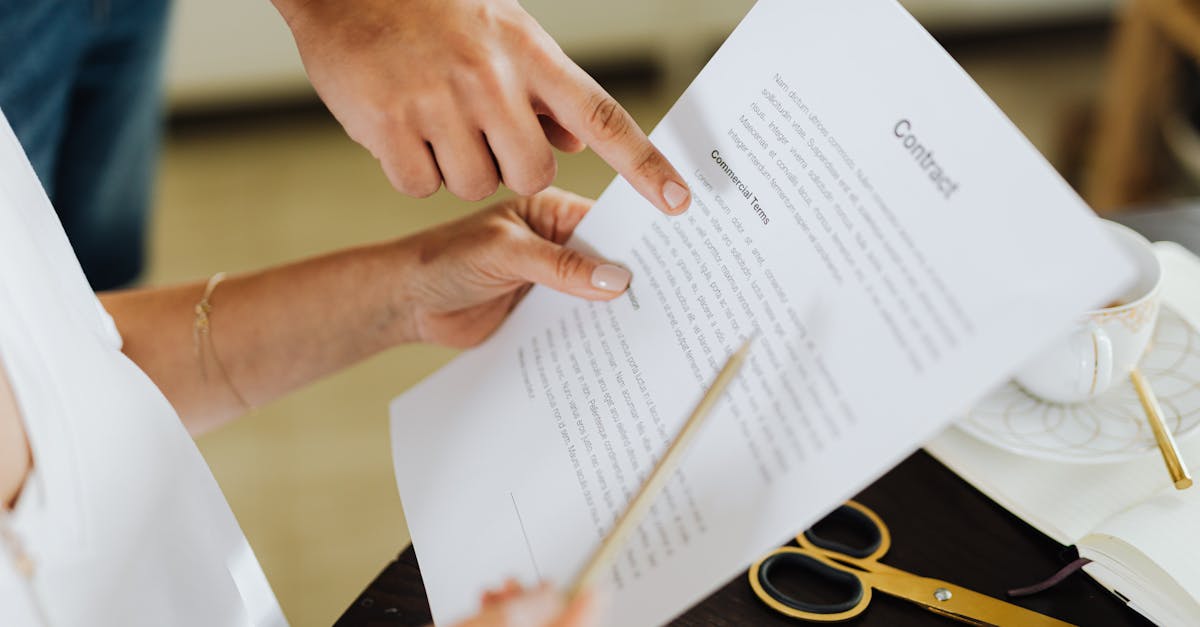
Medical Image Analysis is a rapidly growing field in Life Sciences that utilizes advanced technology to assist in the analysis of medical images such as X-rays, MRI scans, and histopathology slides for disease detection and diagnosis. This field combines the expertise of medical professionals and computer scientists to develop algorithms and techniques for extracting meaningful information from these images, ultimately aiding in accurate disease diagnosis and providing personalized treatment plans.
Technology
The technology used in medical image analysis involves a combination of image processing, machine learning, and computer vision techniques. These technologies enable the extraction of features from medical images, visual representation, and quantitative analysis of the data captured by different imaging modalities.
Area: Medical Image Analysis
Medical Image Analysis primarily focuses on developing algorithms and tools for the interpretation and understanding of various medical images. It involves a range of tasks including image segmentation (identifying the regions of interest), classification (identifying the presence or absence of a disease or condition), registration (aligning multiple images or images from different modalities), and visualization (enhancing visibility and understanding of the structures within the image).
Usage
Medical Image Analysis has numerous applications and benefits in the field of healthcare:
- Disease Detection and Diagnosis: Medical Image Analysis algorithms can assist in the early detection and accurate diagnosis of various diseases or conditions. For example, in lung cancer diagnosis, these algorithms can help identify suspicious nodules or tumors in chest X-rays, providing valuable information to radiologists and oncologists.
- Treatment Planning and Monitoring: Medical images, when analyzed using advanced algorithms, can aid in treatment planning and monitoring. By visualizing the internal structures and abnormalities captured by MRI scans, physicians can precisely plan radiotherapy or surgical interventions while minimizing the risk of complications.
- Research and Development: Medical Image Analysis plays a crucial role in research and development, allowing scientists to study diseases, understand their progression, and evaluate the effectiveness of novel therapies or drugs. It assists in generating quantitative measurements and biomarkers, providing valuable insights into disease mechanisms and treatment outcomes.
- Automated Screening: With the help of automated medical image analysis, large-scale screenings can be performed efficiently. For instance, in mammography, the algorithms can analyze mammograms to identify potential breast abnormalities, enabling early detection of breast cancer and reducing mortality rates.
Medical Image Analysis has the potential to greatly impact the field of healthcare by improving diagnostic accuracy, enabling personalized treatment plans, and enhancing research capabilities. With advancements in technology, the algorithms and techniques utilized in medical image analysis are continuously evolving, contributing towards better patient care and outcomes.
Comments:
Thank you all for joining this discussion on transforming medical image analysis! As the author of the blog post, it's great to see so many people interested in the potential of ChatGPT in life sciences. I'm excited to hear your thoughts and perspectives.
Excellent article, Taren! The advancements in medical image analysis are indeed fascinating. With the power of ChatGPT, I can envision more accurate diagnoses and faster decision-making. This could greatly benefit healthcare professionals and patients alike.
Thank you, Oliver! I completely agree. The potential for ChatGPT to assist medical professionals in analyzing and interpreting complex medical images is immense. It can help save time, improve accuracy, and ultimately enhance patient outcomes.
I find the idea of using ChatGPT for medical image analysis very intriguing. However, do you think it's robust enough to handle the nuances and complexities of different medical conditions? I'm concerned about potential misdiagnoses.
That's a valid concern, Eleanor. While ChatGPT shows promising capabilities, it's crucial to ensure that the system is thoroughly trained on diverse datasets representing various medical conditions. Additionally, it should always be used as a tool to assist human experts rather than replacing their expertise and judgment.
I'm curious about the ethical considerations when implementing ChatGPT for medical image analysis. How can we address issues of privacy and data security, especially when dealing with sensitive patient data?
Ethical considerations are essential, Sophia. Implementing measures such as secure data storage, anonymization, and strict access controls are crucial to protect patient privacy. Adhering to relevant regulations, like HIPAA, is vital to maintain a high standard of data security and privacy.
Taren, great write-up! How do you see the integration of ChatGPT in medical image analysis workflows? How can it seamlessly fit into existing systems and processes?
Thank you, Nathan! Integrating ChatGPT into existing systems can be done through APIs or software development kits (SDKs). By providing easy-to-use interfaces, developers can incorporate ChatGPT into medical image analysis workflows without requiring significant changes to the existing infrastructure.
The potential of ChatGPT in transforming medical image analysis is remarkable, but do you think there will be challenges in gaining acceptance from healthcare professionals who may question the reliability of AI-based diagnoses?
Certainly, Liam. Building trust among healthcare professionals is crucial. Transparent communication about the limitations, continuous validation against clinical data, and collaboration between AI experts and healthcare providers can help address concerns and demonstrate the reliability and value of AI-assisted medical image analysis.
I can see the potential benefits of ChatGPT in medical image analysis, but what about the costs associated with implementing such technology? Will it be accessible to healthcare institutions with limited budgets?
Cost considerations are important, Victoria. However, with advancements and increasing adoption, the costs of implementing AI technologies like ChatGPT are reducing over time. Open-source alternatives and collaborative efforts between academia and healthcare institutions can also contribute to making these technologies more accessible and cost-effective.
I wonder if ChatGPT can also be used to assist in training medical professionals. It could potentially provide support during medical education or as a reference tool for up-to-date information. What are your thoughts?
Absolutely, Mason! ChatGPT has the potential to be a valuable educational tool. It can assist in medical training by providing access to vast amounts of medical literature and acting as a reference tool for the latest research and best practices, ultimately helping medical professionals stay updated and enhance their knowledge.
While the idea of using AI to improve medical image analysis is interesting, we must not overlook potential biases within the training data that could lead to biased or discriminatory outcomes. How can we ensure fairness and address bias in AI systems like ChatGPT?
You raise a critical point, Ava. Ensuring fairness in AI systems is essential. It's imperative to carefully curate training data, mitigate biases, and continuously evaluate and improve the system's performance to avoid discriminatory outcomes. Transparency in AI system development is crucial to facilitating fairness and accountability.
I'm curious about the scalability of ChatGPT for medical image analysis. How well does it handle large datasets and high-resolution images? Are there any limitations to scalability?
Scalability is an important aspect, Sophie. ChatGPT's performance depends on the available computational resources. To handle large datasets and high-resolution images, it may require distributed computing setups or cloud infrastructure. While scalability is possible, it's necessary to consider the associated computational requirements and resource availability.
I think integrating ChatGPT into medical image analysis workflows has the potential to enhance collaboration between different healthcare professionals. It can help bridge the gap between radiologists, pathologists, and other specialists, leading to more comprehensive diagnoses. What do you think?
Great observation, Grace! Collaboration among healthcare professionals is crucial for comprehensive diagnoses, especially when dealing with complex cases. ChatGPT can facilitate interdisciplinary collaboration by providing a platform for efficient communication, knowledge sharing, and pooling of expertise across different specialties, ultimately leading to improved patient care.
What are the possible challenges in acquiring and preparing high-quality datasets for training ChatGPT for medical image analysis? Are there any specific requirements or recommendations?
Acquiring and preparing high-quality datasets can indeed be challenging, Leo. A diverse range of accurately annotated medical images is required for training ChatGPT. It's important to ensure data quality, maintain proper annotations, and address potential bias. Collaborating with healthcare institutions, utilizing open datasets, and incorporating expert annotations can contribute to building robust training datasets.
This technology sounds very promising. However, how can healthcare professionals keep up with the rapid advancements in AI-based medical image analysis to make the most of ChatGPT's potential?
Continuous learning and staying up-to-date is crucial for healthcare professionals, Isabella. Organizations can provide training programs, workshops, and conferences to educate and familiarize medical professionals with AI advancements like ChatGPT. Collaboration with AI experts and involving clinicians in the development process can also help bridge the knowledge gap.
The concept of using ChatGPT for medical image analysis is fascinating. Do you have any examples of successful real-world implementations or case studies that highlight its effectiveness?
Indeed, Daniel! While ChatGPT is still relatively new in medical image analysis, there are already promising advancements. Some real-world implementations have shown success in assisting radiologists with image interpretation, pathology analysis, and identifying subtle patterns that may lead to earlier disease detection. These implementations serve as early indicators of the potentials and benefits of ChatGPT in medical image analysis.
Considering the complexity of medical images and the time required for training AI models, how long do you think it will take before ChatGPT becomes widely adopted in medical image analysis?
Adoption timelines are hard to predict, Lucas. However, the increasing interest and ongoing advancements in the field indicate that ChatGPT and similar AI technologies will likely see a gradual adoption over the coming years. As trust, validation, and regulatory frameworks evolve, healthcare institutions will become more comfortable integrating AI into their image analysis workflows.
I'm interested in understanding the potential limitations of AI-based medical image analysis. What scenarios or conditions might be challenging for ChatGPT to handle effectively?
Great question, Lena! ChatGPT, like any AI system, has limitations. Complex cases, rare conditions, extremely subtle findings, or situations where multimodal data (such as combining images with clinical history) is crucial can pose challenges. Human expertise and judgment remain essential in such scenarios, and ChatGPT should be used as a helpful tool in conjunction with professional analysis.
This could revolutionize medical image analysis. However, what efforts are being made to ensure the explainability of ChatGPT's decisions? It's crucial for healthcare professionals to understand and trust the basis behind the system's interpretations.
Explainability is a vital aspect, Emily. Research and efforts are ongoing to enhance the explainability of AI systems like ChatGPT. Techniques such as attention mechanisms, saliency maps, and interpretable frameworks are being explored to provide insights into the basis for the system's decisions. Transparent reporting and clear communication of system capabilities and limitations are necessary to establish trust in AI-generated interpretations.
How do you envision the future integration of AI technologies like ChatGPT with other emerging fields, such as genomics or personalized medicine?
That's an exciting prospect, Grace! Integration with other emerging fields opens up new possibilities. ChatGPT and AI systems can complement genomics and personalized medicine by aiding in the interpretation of genetic data, analyzing genomic images, and assisting in treatment selection based on individualized patient characteristics. The synergy between these fields holds great potential for improving patient outcomes.
While ChatGPT can improve efficiency and diagnosis accuracy, how can we ensure that it doesn't lead to overreliance on AI and neglect the importance of human clinical judgement?
Valid concern, Olivia. Avoiding overreliance is crucial. The use of AI, including ChatGPT, should be seen as a supportive tool, providing assistance to healthcare professionals rather than replacing their expertise. Continuous education, promoting interdisciplinary collaboration, and establishing clear guidelines on the role of AI can help strike a balance between AI assistance and the importance of human clinical judgment.
I'm intrigued by the potential of ChatGPT in medical image analysis research. Are there any open datasets available for researchers to experiment and contribute to the advancement of this field?
Absolutely, Adam! There are several open datasets available for medical image analysis, which can be used by researchers to explore and contribute to the field. Datasets like the OpenI database, the MIMIC-CXR dataset, or the NIH Chest X-ray dataset provide valuable resources for training and testing AI models in medical image analysis.
Beyond medical image analysis, can ChatGPT be adapted to assist in other areas of healthcare, such as remote patient monitoring or telemedicine?
Absolutely, Caroline! ChatGPT's capabilities can extend beyond medical image analysis. It can be adapted to assist in remote patient monitoring by providing insights on recorded physiological measurements and symptoms. In telemedicine, ChatGPT can assist healthcare providers by answering patient queries, guiding self-assessments, and aiding in triage. The potential applications are broad and can enhance various aspects of healthcare delivery.
To ensure the wider adoption and success of AI-based medical image analysis, how can we address the challenges of integrating AI systems with existing healthcare IT infrastructure and ensure interoperability?
Integration challenges are important, David. Collaborative efforts between AI developers, healthcare IT professionals, and vendors can help address interoperability concerns. Ensuring compatibility with existing standards, such as DICOM for medical imaging, and leveraging established industry practices enable smooth integration of AI systems like ChatGPT with healthcare IT infrastructure.
How can AI technologies like ChatGPT contribute to reducing healthcare disparities, especially in underprivileged communities with limited access to expert medical resources?
AI technologies have the potential to narrow healthcare disparities, Chloe. By assisting in diagnostics, AI systems like ChatGPT can provide better access to expert insights and augment the capabilities of healthcare professionals serving underprivileged communities. Additionally, telemedicine applications can facilitate remote consultations and bring specialized expertise to areas with limited resources, ultimately improving healthcare outcomes and reducing disparities.
Taren, what do you think about the potential challenges in obtaining regulatory approvals for AI systems like ChatGPT in medical image analysis? Are there any specific guidelines to ensure patient safety and regulatory compliance?
Regulatory approvals are indeed a crucial aspect, Harper. Various regulatory bodies, such as the FDA, provide guidelines and frameworks for the evaluation and approval of AI systems in healthcare. Adhering to regulations, demonstrating safety, and conducting rigorous clinical validations are necessary to ensure patient safety, reliability, and regulatory compliance. Close collaboration between AI developers, healthcare providers, and regulatory authorities helps navigate this regulatory landscape.
I'm excited about the potential of ChatGPT in medical image analysis. How can researchers and developers work in collaboration to accelerate progress in this field?
Collaboration is essential, Amelia! Researchers, developers, and healthcare professionals can work together to share knowledge, expertise, and resources. Joint research projects, open-source initiatives, and industry-academia partnerships can accelerate progress. Engaging in discussions, attending conferences, and fostering interdisciplinary collaboration create an environment conducive to exploring new ideas, validating approaches, and driving advancements in medical image analysis with the help of technologies like ChatGPT.
Thank you all for your valuable comments and questions! It has been an insightful discussion, and I appreciate your active participation in exploring the potential of ChatGPT in medical image analysis. If you have any further thoughts or questions, please feel free to continue the conversation.