Transforming Operations Research in Technology: Harnessing the Power of ChatGPT
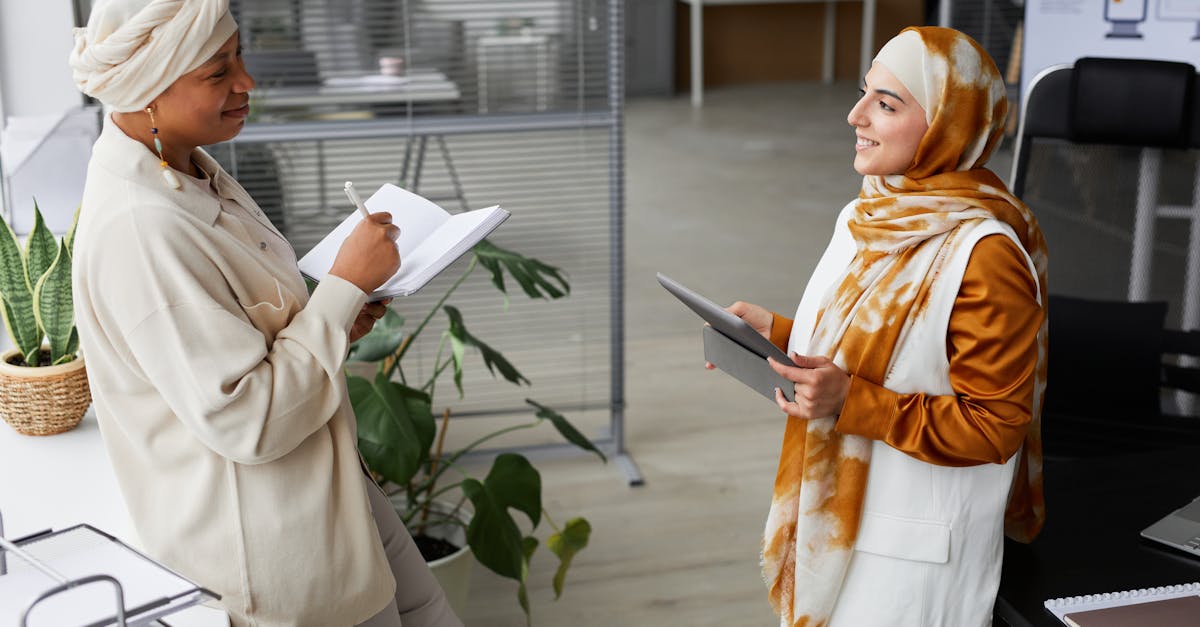
Over the years, Operations Research has fundamentally reshaped the world of Supply Chain Management. As the supply chain sector continues to advance, stakeholders are seeking emerging technologies to help them streamline their operations and improve efficiency. Of all the technologically innovative solutions available, chatbots have turned out to be one of the most effective. Chatbots, integrated with Operations Research methodologies, are proving to be indispensable assets in handling supply chain queries, supervising inventory levels, predicting logistics, automating various processes, and aiding decision-making based on data analytics.
A Brief Introduction to Operational Research and Chatbot Technology
Operations Research is a discipline that deals with skilful use of advanced analytical methods to help make better decisions. Initially emerging during World War II to enhance military operations, Operations Research now has an array of applications in diverse industries, including Supply Chain Management.
A chatbot, on the other hand, is a program driven by rules and artificial intelligence (AI) that simulates human conversation through voice commands, text chats, or both. It is a virtual assistant that understands user needs and performs appropriate tasks. For Supply Chain Management, chatbots can be used to handle diverse tasks such as responding to supply chain queries, maintaining inventory levels and even predicting logistics.
Chatbots in Supply Chain Management
In the essence of chatbot technology lies its ability to mimic human interactions creating an intuitive interface for users. For Supply Chain Management, chatbots can provide numerous benefits, from handling customer queries to automating tasks.
With AI and machine learning, query handling becomes more efficient. Chatbots are capable of performing various supportive tasks, such as providing information on shipment status, inventory status, and facilitating communication between different organizational departments. This can significantly reduce the workforce required to manage these tasks and can inherently improve response times and efficiency.
Inventory management is another task where chatbots, by leveraging Operations Research methodologies, can make a significant difference. They can monitor and report inventory levels in real-time, notify necessary stakeholders when supplies are low or forecast future inventory needs based on historical trends and current demand. This can lead to more effective inventory management, reducing the risk of overstocking or understocking.
In the logistics realms, chatbots can predict potential challenges based on factors such as weather patterns, geopolitical events, or even traffic congestion. By doing so, they enable businesses to prepare for unexpected circumstances, ensuring that their supply chains are not significantly disturbed.
Lastly, the automation of various processes via chatbots helps in reducing human errors and increases the efficiency and effectiveness of these processes. Chatbots can be programmed to perform tasks such as tracking shipments, managing stock, and even finalizing transactions.
Chatbots for Decision Making based on Data Analytics
Modern chatbots are capable of processing and interpreting large data sets, giving them a decisive role in making data-driven decisions. They can identify patterns and derive insights from historical data, which in turn can be used to make predictions about future trends. Such predictive analytics can facilitate strategic decision making in Supply Chain Management by enabling proactive actions and anticipation of future challenges.
Conclusion
The fusion of Operations Research and chatbot technology offers promising potential for reshaping operations in Supply Chain Management. From automating mundane tasks to facilitating strategic planning, the usage of chatbots is rapidly transforming how businesses manage their supply chains. Embracing this technology can lead companies to sync better with their supply chains, improve customer service, and gain a competitive edge in the market.
Comments:
Thank you all for taking the time to read my article on 'Transforming Operations Research in Technology: Harnessing the Power of ChatGPT'. I look forward to hearing your thoughts and engaging in a fruitful discussion!
Great article, Everett! I found the concept of harnessing ChatGPT for operations research fascinating. It's definitely a powerful tool that can revolutionize the field. I'm curious to know how ChatGPT can address the challenges of real-time decision-making in dynamic systems. Any thoughts?
Thank you, Samantha! I appreciate your positive feedback. Regarding the challenges of real-time decision-making in dynamic systems, ChatGPT can handle such scenarios by utilizing a combination of reinforcement learning algorithms and continuous learning. By training on historical data and adapting to evolving circumstances, it can provide real-time insights and optimize decision-making. It's an exciting area of research!
I enjoyed reading your article, Everett! The potential of ChatGPT in operations research seems immense. However, do you think there might be limitations when it comes to integrating ChatGPT into existing systems? Compatibility could be a concern, especially in industries with legacy infrastructure.
Thank you, Mark! You bring up a valid point. Integrating ChatGPT into existing systems can pose challenges, particularly with legacy infrastructure. However, by using well-defined APIs and proper system integration practices, it is possible to bridge the gap. Adapting and upgrading existing systems to leverage the benefits of ChatGPT can lead to significant improvements in operations research outcomes.
I was also wondering about the integration challenges, Mark. Everett, do you think organizations need to invest in significant infrastructure updates to fully integrate ChatGPT into their existing systems?
Hi Rachel! Fully integrating ChatGPT into existing systems may require some infrastructure updates, particularly when dealing with legacy systems. However, it's not necessarily a requirement for significant investments. By using well-defined APIs and thoughtful system integration practices, organizations can gradually adapt their infrastructure to integrate ChatGPT's capabilities. This allows for a step-by-step approach that aligns with an organization's resources and priorities. The benefits of leveraging ChatGPT in operations research often outweigh the integration efforts involved.
Your insight on infrastructure updates is valuable, Rachel. Everett, how can organizations gradually align their infrastructure to integrate ChatGPT without disrupting existing operations?
Hello, Natalie! Organizations can gradually align their infrastructure to integrate ChatGPT without disrupting existing operations by following an iterative approach. This involves identifying specific use cases or areas where ChatGPT's capabilities can bring the most value, and focusing on integrating it within those domains first. By starting with a limited scope, organizations can evaluate the impact, learn from experiences, and refine the integration process. Iterative improvements, guided by strategic implementation plans, minimize disruption and allow for scalable integration, ensuring a smooth transition to leverage ChatGPT in operations research.
Thanks for addressing infrastructure updates, Natalie. Everett, how can an organization evaluate the impact of integrating ChatGPT into their existing systems during the iterative alignment process?
Hi Chloe! Evaluating the impact of integrating ChatGPT into existing systems during the iterative alignment process involves defining clear success metrics and conducting regular assessments. Organizations can monitor various factors like decision-making effectiveness, resource utilization, time savings, or any other relevant KPIs as defined for the integration. Comparing the outcomes before and after the integration, alongside feedback from stakeholders, helps measure the impact accurately. Conducting user surveys, focus groups, or usability testing sessions also aids in gathering qualitative feedback on the integration's impact. Combining quantitative and qualitative evaluation measures ensures a comprehensive assessment of the impact of integrating ChatGPT into existing systems.
Your comments on data privacy are crucial, Chloe. Everett, what are the key considerations organizations should address to ensure data compliance while leveraging ChatGPT?
Hello, Lucas! Ensuring data compliance while leveraging ChatGPT involves several key considerations. Organizations should assess the legal and regulatory landscape applicable to their operations and define clear data privacy policies compliant with these regulations. Transparency about how data is collected, used, and stored is vital. Organizations must establish robust security measures, including data anonymization, encryption, and access controls. Additionally, obtaining informed consent from users for data usage, providing options for data erasure, and enabling clear mechanisms for data subject rights allow compliance with data protection regulations. A proactive approach to addressing data compliance builds trust and safeguards user privacy in AI-powered operations research using ChatGPT.
Your comments on managing computational demands are insightful, Chloe. Everett, how can organizations explore online and incremental learning techniques for real-time adaptation with ChatGPT?
Hi Aaron! Exploring online and incremental learning techniques for real-time adaptation with ChatGPT involves several considerations. These techniques generally involve updating the model based on new data samples while minimizing the computational requirements. Organizations can design a data pipeline to continuously feed new relevant data to the model for ongoing learning. Efficient algorithms like stochastic gradient descent or optimizer-specific adaptive learning rate approaches can be employed to update the model's parameters effectively. Choosing appropriate techniques and adapting them to the specific operations research requirements allows ChatGPT to leverage real-time data effectively and adapt swiftly, enhancing its decision-making capabilities.
Hi Everett! Your article was thought-provoking. I believe ChatGPT can greatly enhance decision-making in operations research. However, I'm curious about its limitations when dealing with complex optimization problems involving large datasets. How does ChatGPT handle computational scalability?
Hello, Linda! I'm glad you found the article thought-provoking. ChatGPT's handling of complex optimization problems involving large datasets is a challenging aspect. While it performs well with substantial amounts of data, there are limitations in terms of computational scalability. To address this, we can explore techniques like data partitioning, distributed computing, and parallel processing to manage the workload efficiently. Some research efforts are also aimed at optimizing GPT architectures for better resource utilization. It's definitely an area for future improvements!
I'm glad you raised the issue of handling large datasets, Linda. Everett, are there ways to overcome computational scalability limitations when using ChatGPT for operations research involving vast amounts of data?
Hello, Robert! Overcoming computational scalability limitations is an ongoing research focus when using ChatGPT for operations research involving large datasets. Techniques like data partitioning, parallel processing, and distributed computing can help manage the workload effectively. Additionally, advances in hardware technology and optimizations in deep learning frameworks contribute to improved scalability. Researchers and engineers are actively exploring these avenues to enhance ChatGPT's computational scalability and bring its benefits to operations research on vast datasets.
Thanks for addressing the computational scalability, Robert. Everett, are there techniques or advancements that can further improve ChatGPT's computational scalability?
Hi Laura! Advancements to improve ChatGPT's computational scalability are actively researched. Techniques like model distillation, network pruning, and quantization aim to reduce the model's computational requirements while maintaining the desired performance. Adaptive computation time approaches dynamically adjust the amount of computation allocated to different parts of the input, improving efficiency. Distributed computing and leveraging hardware accelerators like GPUs and TPUs also contribute to enhanced computational scalability. Continuous research and engineering efforts focus on optimizing ChatGPT's performance in handling larger workloads more efficiently within operations research.
Your insight into scalability advancements is valuable, Laura. Everett, can you explain how model distillation can contribute to better computational scalability in ChatGPT?
Hello, Aiden! Model distillation contributes to better computational scalability in ChatGPT by reducing its overall complexity. It involves training a smaller, more lightweight model (student model) to mimic the behavior and knowledge of a larger, more complex model (teacher model), which is ChatGPT in this case. By distilling the teacher model's knowledge into the student model, we achieve a more efficient model that occupies less memory and requires fewer computations. This technique enables faster processing times and improved computational scalability without compromising the accuracy of ChatGPT's recommendations in operations research.
Interesting article, Everett! I believe ChatGPT has enormous potential in the field of operations research. However, there might be ethical concerns when it comes to using AI-powered decision-making systems. How can we ensure transparency, fairness, and accountability in the decision-making process?
Thank you, Gabriel! Addressing ethical concerns is crucial in AI-powered decision-making systems. To ensure transparency, decision outputs from ChatGPT can be logged and made auditable. Fairness can be achieved by training the model on diverse and unbiased datasets. Furthermore, incorporating explainability approaches, augmenting human decision-makers with AI systems instead of replacing them entirely, and subjecting the AI's recommendations to human review can enhance accountability. It's an ongoing challenge but one that must be tackled to build trustworthy AI systems!
Your comment on ethical concerns is crucial, Gabriel. Everett, what measures can be taken to avoid bias and ensure fairness in the decision-making process powered by ChatGPT?
Hi Sarah! Avoiding bias and ensuring fairness is of paramount importance when deploying AI systems like ChatGPT. One approach is to ensure training datasets are diverse, inclusive, and representative of the population they serve. Regularly monitoring and auditing the system for unintended biases is essential. Techniques like debiasing, fairness-aware training, and post-processing can also be employed to mitigate bias. Collaborating with experts in ethics and fairness is crucial to define and implement appropriate measures that align with societal values and promote fairness in decision-making processes powered by ChatGPT.
Your comments on bias reduction are essential, Sarah. Everett, how can organizations ensure accountability when implementing the fairness-aware measures you mentioned?
Hello, Lucia! Ensuring accountability when implementing fairness-aware measures is crucial. Organizations can establish clear accountability frameworks, with roles and responsibilities defined for monitoring and auditing the decision-making process powered by ChatGPT. External audits conducted by independent experts can provide an unbiased evaluation of the fairness and ethical implications. Involving relevant stakeholders, from data collection to decision-making, fosters transparency and shared responsibility. By having transparency reports and avenues for user feedback, organizations demonstrate their commitment to accountability and build trust in the fairness of operations research outcomes derived from ChatGPT.
Your comments on accountability are crucial, Lucia. Everett, how can organizations facilitate external audits to ensure fairness and ethical implications are evaluated effectively?
Hi Leah! Facilitating external audits to ensure fairness and ethical implications are effectively evaluated involves collaboration with independent auditors who specialize in AI ethics and fairness. Organizations can engage with these auditors to design audit processes that assess ChatGPT's performance, decision-making, and adherence to fairness standards. The auditors review relevant metrics, validate the training process, investigate potential biases, and conduct robust fairness assessments. The collaboration should be guided by transparent agreements, incorporating the expertise and perspectives of external auditors in defining fairness evaluation criteria and conducting thorough audits of the decision-making process powered by ChatGPT.
I appreciate your comment on transparency, fairness, and accountability, Gabriel. Everett, can you elaborate on how explainability approaches can be implemented to achieve transparency in ChatGPT's recommendations?
Certainly, Emma! Explainability approaches can address the transparency aspect of ChatGPT's recommendations. Techniques like attention mechanisms help identify which parts of the input data influenced the decision, providing valuable insights. Additionally, generating explanations alongside the recommendations can help domain experts understand the reasoning behind ChatGPT's suggestions. Explanation methods such as LIME (Local Interpretable Model-agnostic Explanations) and SHAP (SHapley Additive exPlanations) can be leveraged to highlight the contribution of different input features to the decision. These techniques contribute to a more transparent decision-making process, enabling better trust and understanding.
Great article, Everett! I'm curious to know if ChatGPT can handle uncertain and incomplete data in operations research scenarios. Any insights on that?
Thank you, Sophie! ChatGPT can indeed handle uncertain and incomplete data in operations research scenarios. It has the ability to leverage probabilistic models and handle uncertain inputs. In the case of incomplete data, ChatGPT can make reasonable assumptions based on the available information. However, it's important to note that the quality and completeness of the data play a significant role in the reliability of the insights provided. Addressing data uncertainty and incompleteness is an important area of research within operations research and AI.
The topic of handling uncertain and incomplete data is crucial, Sophie. Everett, do you think there are any potential advancements that can further enhance ChatGPT's performance in dealing with such data scenarios?
Hello, Harper! Advancements to further enhance ChatGPT's performance in dealing with uncertain and incomplete data are actively pursued. Techniques like Bayesian neural networks can provide a probabilistic framework for handling uncertainty more effectively. Additionally, research in active learning aims to enable ChatGPT to seek further information to reduce uncertainty and make more informed decisions. Another direction of improvement lies in handling missing data through techniques like imputation. By continually pushing the boundaries of research, we can enhance ChatGPT's capabilities in handling various data scenarios within operations research.
Your comments on dealing with data uncertainty are insightful, Harper. Everett, what are the research directions focused on reducing uncertainties in operations research, specifically with ChatGPT?
Hello, Isabella! Reducing uncertainties in operations research, specifically with ChatGPT, is an active research area. Ongoing efforts are focused on exploring Bayesian models to provide better uncertainty quantification and propagate it through the decision-making process. Research on active learning aims to develop techniques to identify the most valuable data for reducing uncertainties, leading to more informed decisions. Additionally, research in efficient handling of missing data and imputation techniques seeks to minimize uncertainties arising from incomplete information. Through these research directions, we aim to enhance ChatGPT's performance and reliability in operations research scenarios with uncertainties.
Fantastic article, Everett! ChatGPT's potential in operations research is exciting. I'm wondering about the privacy implications when dealing with sensitive data. How can we ensure data privacy while utilizing ChatGPT?
Thank you, Adam! Ensuring data privacy is crucial when dealing with sensitive information. By implementing privacy-aware protocols and adopting techniques like federated learning, data anonymization, and differential privacy, we can protect users' privacy while utilizing ChatGPT. It's important to have robust privacy measures in place and comply with relevant data protection regulations to maintain user trust and confidentiality.
Thanks for addressing data privacy concerns, Adam. Everett, how can organizations ensure that ChatGPT's recommendations do not compromise data privacy, especially when dealing with sensitive information?
Hi Eva! Ensuring data privacy is crucial, especially when dealing with sensitive information. Organizations can implement privacy-aware protocols like data anonymization, encrypting sensitive data, and access controls. Techniques like federated learning enable training ChatGPT with data distributed across different organizations, without sharing the raw data. Additionally, differential privacy can help preserve privacy by adding noise to the data. By adopting a layered approach incorporating technical measures, legal compliance, and robust privacy practices, organizations can protect data privacy while leveraging ChatGPT's capabilities in operations research.
Thanks for explaining data privacy measures, Eva. Everett, how can organizations ensure compliance with data protection regulations while using ChatGPT for operations research?
Hi Sophia! Ensuring compliance with data protection regulations while using ChatGPT for operations research involves several measures. Organizations can establish comprehensive privacy policies that address the use of AI systems like ChatGPT and adhere to applicable data protection regulations. Employing anonymization techniques, encrypting sensitive data, and implementing access controls help protect user privacy. Additionally, providing transparency to users about how their data is used, obtaining appropriate consent, and allowing users control over their data contribute to compliance. Collaboration with legal experts and maintaining awareness of evolving regulations is essential to ensure compliance in data-driven operations research.
Hi Everett! Your article sheds light on the potential of ChatGPT in operations research. Do you think there might be concerns about overreliance on AI systems and their impact on human decision-making skills? How can we strike a balance?
Hello, Lucas! Overreliance on AI systems can indeed raise concerns about the impact on human decision-making skills. Striking a balance involves augmenting human decision-makers with AI systems rather than completely replacing their role. By combining human expertise with AI insights, we can benefit from the efficiency and accuracy of AI while ensuring that human judgement and critical thinking are not undermined. AI systems should be viewed as decision support tools rather than autonomous decision-makers. It's crucial to foster a collaborative partnership between humans and AI in operations research.
I agree, Lucas! Everett, can you share any best practices on how to ensure a harmonious collaboration between humans and AI systems in operations research?
Certainly, Sophie! Ensuring a harmonious collaboration between humans and AI systems involves several best practices. First, organizations should foster a culture of collaboration and educate stakeholders about the capabilities and limitations of AI systems. It's crucial to involve domain experts in the decision-making process, where the AI system's recommendations are subject to human review and final decisions are made collectively. Encouraging ongoing feedback, refining the AI's capabilities based on user experiences, and conducting regular performance evaluations all contribute to building trust and realizing the full potential of collaborative operations research between humans and AI.
Your comment on collaborative operations research is insightful, Sophie. Everett, how can organizations actively foster a culture of collaborative decision-making between humans and AI systems?
Hello, Alexander! Actively fostering a culture of collaborative decision-making between humans and AI systems involves multiple aspects. Organizations can encourage interdisciplinary teams comprising domain experts, AI specialists, and decision-makers to work together. Transparent communication, knowledge sharing, and regular meetings help build common ground and mutual understanding. Encouraging open feedback and facilitating discussions on the collaboration's impact and challenges foster a learning environment where both humans and AI systems can improve. By recognizing and rewarding contributions from both human and AI decision-makers, organizations reinforce the value of collaborative operations research and pave the way for its continued success.
Your comments on fostering a learning environment are valuable, Alexander. Everett, how can organizations encourage ongoing feedback from stakeholders to improve collaborative decision-making?
Hi Ella! Encouraging ongoing feedback from stakeholders to improve collaborative decision-making involves creating an environment that values input from all parties. Organizations can establish feedback mechanisms like suggestion boxes, periodic surveys, or dedicated channels for stakeholder engagement. Regularly conduct feedback sessions and focus groups to gather insights, suggestions, and concerns. Actively incorporating the feedback into decision-making processes fosters a sense of ownership and investment among stakeholders. Clear communication on how the feedback impacts decision-making, the rationale behind decisions, and the improvements ensuing from the feedback further motivate stakeholders to participate and engage in collaborative decision-making effectively.
Great read, Everett! I'm curious about the potential applications of ChatGPT in industries beyond technology. Can it be adapted for operations research in healthcare, for example?
Thank you, Emily! Absolutely, ChatGPT has the potential for applications beyond technology. It can be adapted for operations research in various industries, including healthcare. By utilizing healthcare-specific data and training the model accordingly, ChatGPT can provide valuable insights and support decision-making in healthcare operations research, ranging from resource allocation optimization to personalized treatment recommendations. Adapting the model to specific domains is an exciting direction for expanding its applicability!
Your comment on the adaptability of ChatGPT to healthcare is intriguing, Emily. Everett, how can ChatGPT be trained and fine-tuned for healthcare-specific operations research?
Hi Megan! ChatGPT can be trained and fine-tuned for healthcare-specific operations research by utilizing healthcare-specific datasets. These datasets can include electronic health records, clinical trials data, medical literature, and more. By focusing the fine-tuning process on healthcare-related objectives and using data that captures the nuances of healthcare decision-making, ChatGPT can learn to provide valuable insights specific to the healthcare domain. Tailoring the model to the healthcare context helps unlock its potential in supporting various operations research aspects within the healthcare industry.
Insightful article, Everett! I'm interested to know about the interpretability of ChatGPT's decision-making in operations research. How can we ensure that the model's reasoning is explainable and understandable to domain experts?
Thank you, Timothy! Ensuring interpretability and explainability in ChatGPT's decision-making is important. Techniques like attention mechanisms can help identify key elements in the input data that contribute to the model's reasoning. Additionally, incorporating rule-based systems and generating explanations alongside the recommendations can aid domain experts in understanding the rationale behind the model's decisions. Interpretable AI models play a crucial role in building trust and adoption in operations research.
I appreciate your explanation on achieving interpretability, Timothy. Everett, how can rule-based systems be integrated with ChatGPT to enhance transparency?
Hello, Jason! Integrating rule-based systems with ChatGPT enhances transparency by providing explicit rules that guide decision-making. These rules can be formulated by domain experts and incorporated alongside ChatGPT's recommendations. When the rules influence the model's output, it becomes clearer how the decision aligns with the established guidelines. By combining the strengths of ChatGPT's AI capabilities with the interpretability and explicitness of rule-based systems, we can strike a balance between complex reasoning and transparent decision-making within operations research.
Your insight into balancing reasoning complexity and transparent decision-making is valuable, Jason. Everett, how can rule-based systems be developed to coexist naturally with the suggestions provided by ChatGPT?
Hello, Jack! Developing rule-based systems to coexist naturally with ChatGPT involves creating comprehensive rules that holistically align with the organization's objectives. By involving both rule-based systems and ChatGPT's suggestions in informing decisions, organizations can harmonize the strengths of each approach. The combination should aim for synergy, where the rules guide the decision-making within predefined boundaries, while ChatGPT provides insights and highlights possibilities that might be overlooked solely by rule-based approaches. It's crucial to foster collaboration between stakeholders to define and refine these rules, allowing their coexistence to augment operations research outcomes effectively.
I appreciate your insight into explainability approaches, Timothy. Everett, can you explain how attention mechanisms help in identifying key elements in ChatGPT's decision-making?
Hi Maria! Attention mechanisms in ChatGPT help identify key elements by assigning importance weights to different parts of the input data. The model learns to allocate higher attention to relevant words or phrases in the context, highlighting their influence on the decision-making process. By analyzing the attention weights, we can identify which parts of the input data the model relied upon to make its recommendation. Attention mechanisms provide valuable insights into the inner workings of ChatGPT's decision-making process, aiding interpretability and transparency within operations research.
Your comments on attention mechanisms are valuable, Maria. Everett, can attention mechanisms of ChatGPT be adapted or customized based on the specific requirements of operations research fields?
Hello, Emma! The adaptability and customization of attention mechanisms in ChatGPT can be explored to cater to the specific requirements of operations research fields. By applying domain-specific attention mechanisms, we can focus the model's attention on relevant aspects aligned with the specific objectives and decision factors within a particular operations research field. Customizing attention mechanisms based on the unique characteristics of the data and the context helps improve the relevance and interpretability of ChatGPT's decision-making process. Exploring attention mechanism adaptations in operations research fields is an exciting research direction that can lead to tailored decision support systems.
Hi Everett! Your article was insightful. I'm wondering about the limitations of ChatGPT when it comes to handling real-time streaming data. Can it keep up with the speed and volume of data generated by modern systems?
Hello, Alicia! Handling real-time streaming data is a challenge for ChatGPT due to its sequential nature and high computational requirements. While it may face limitations in keeping up with the speed and volume of data generated by modern systems, there are ongoing efforts to improve GPT architectures for more efficient processing. Techniques like online and incremental learning can also be explored to adapt ChatGPT to real-time scenarios. Balancing model performance and computational complexity is crucial in such applications.
Great point about real-time data handling, Alicia. Everett, what are the possible strategies to improve ChatGPT's performance in real-time scenarios?
Hello, Maxwell! Improving ChatGPT's performance in real-time scenarios is an ongoing research challenge. Strategies include optimizing the model for faster processing, developing techniques for online and incremental learning to adapt to changing data in real-time, and exploring hardware accelerators like GPUs and TPUs to speed up computations. Techniques such as caching and precomputing relevant information can also improve response times. By combining advancements in model architecture, hardware, and processing techniques, we can enhance ChatGPT's performance in handling real-time streaming data.
Your comments on improving ChatGPT's real-time performance are insightful, Maxwell. Everett, how can the computational demands be managed to ensure real-time handling of streaming data?
Hi Sophie! Managing computational demands to ensure real-time handling of streaming data with ChatGPT involves multiple strategies. One approach is optimizing the model architecture and computations to reduce the overall computation required while maintaining an acceptable level of accuracy. Leveraging hardware accelerators like GPUs and TPUs can significantly improve processing speed. Applying caching and precomputing techniques to minimize repetitive computations can also enhance response times. Additionally, techniques like online and incremental learning enable the model to adapt and fine-tune its recommendations in real-time. Combining these strategies helps strike a balance between computational efficiency and real-time streaming data processing in operations research.
Your explanation of research directions is valuable, Sophie. Everett, how can active learning techniques help improve ChatGPT's decision-making in the presence of uncertainties?
Hi James! Active learning techniques can help improve ChatGPT's decision-making in the presence of uncertainties by selecting the most valuable data to reduce these uncertainties. These techniques enable ChatGPT to actively seek information by posing queries, refining its understanding of the problem, and adapting to reduce uncertainties. By iteratively labeling and incorporating new data points that are most informative, the model can make more informed decisions, reducing uncertainties in operations research scenarios. Active learning offers an avenue to enhance ChatGPT's performance and improve decision-making and adaptability even for complex and uncertain situations.
Your insight on fine-tuning adjustments is valuable, James. Everett, how can organizations refine the fine-tuning process based on the domain-specific requirements of their operations research?
Hello, Clara! Refining the fine-tuning process based on domain-specific requirements involves tailoring it to the unique aspects of the operations research. Organizations should conduct careful analysis of domain-related objectives and decision factors. By customizing hyperparameters like learning rates, regularization techniques, or transfer learning approaches, organizations can align the fine-tuning process with specific operations research requirements. Conducting attuned evaluation using relevant metrics tailored to the domain ensures the fine-tuning process's effectiveness. Further, iteratively refining the fine-tuning process based on insights gained from user feedback and real-world application helps evolve and optimize ChatGPT's decision-making abilities for domain-specific operations research.
The potential for healthcare applications is fascinating, Alicia. Everett, what are the challenges in training ChatGPT for healthcare-specific operations research?
Hi Ava! Training ChatGPT for healthcare-specific operations research comes with challenges. The availability of large-scale, high-quality healthcare datasets can be a limitation. Obtaining appropriate access to sensitive healthcare data while ensuring privacy and complying with regulations can be complex. Additionally, the incorporation of domain expertise and ethics in training to handle critical healthcare scenarios requires careful curation of training data. Overcoming these challenges involves collaborations between researchers, healthcare providers, and AI experts, striving for comprehensive, representative, and privacy-preserving healthcare datasets tailored for training ChatGPT in operations research.
Your comments on training challenges are insightful, Ava. Everett, how can organizations ensure ethical considerations are integrated into healthcare-specific ChatGPT training?
Hello, Grace! Ensuring ethical considerations are integrated into healthcare-specific ChatGPT training involves collaborating with healthcare professionals and stakeholders who are well-versed in ethical guidelines. Organizations must curate training datasets that are representative, unbiased, and conform to established ethical standards. Involving ethics review boards or committees can help assess the ethical implications of utilizing sensitive healthcare data during the training process. Considering the privacy, confidentiality, informed consent, and potential biases during model training ensures that the resulting ChatGPT is aligned with ethical principles. Collaborative efforts between AI experts, healthcare professionals, and ethical experts are essential to ensure ethical considerations are integrated throughout healthcare-specific ChatGPT training.
Your insights on coexistence between rule-based systems and ChatGPT are valuable, Jackson. Everett, how can organizations ensure that the rules and ChatGPT's suggestions are effectively combined to achieve optimal outcome in operations research?
Hello, Liam! Achieving an optimal outcome in operations research by effectively combining rules and ChatGPT's suggestions involves a systematic approach. Organizations should cultivate an environment of collaborative decision-making, encouraging input from domain experts and key stakeholders who can define the rules. It's crucial to prioritize transparency and clearly communicate the boundaries set by the rules to avoid conflicts with ChatGPT's suggestions. Building upon ChatGPT's insights while respecting the predefined rules requires regular evaluation, ongoing communication, and iterative adaptation of both components. By fostering an environment that encourages mutual learning and flexibility, organizations can achieve an optimal outcome by effectively combining ChatGPT's suggestions with rule-based systems within operations research.
Interesting article, Everett! I'm curious about the training process of ChatGPT. How do you ensure the model's accuracy and reliability?
Thank you, Olivia! Training ChatGPT involves a two-step process: pre-training and fine-tuning. In pre-training, the model learns from a large corpus of publicly available text, acquiring language understanding. Fine-tuning follows, where the model is further trained on a specific dataset and task to refine its performance. Ensuring accuracy and reliability involves using high-quality datasets, careful fine-tuning, and rigorous evaluation metrics. Continuous monitoring and updates of the model with real-world feedback also contribute to improving its accuracy and reliability over time.
Your explanation of the training process is insightful, Olivia. Everett, how do you ensure the balance between pre-training and fine-tuning to achieve optimal accuracy in ChatGPT?
Hi Victoria! Achieving the balance between pre-training and fine-tuning is a crucial factor in optimizing ChatGPT's accuracy. A significant amount of pre-training enables the model to capture general language understanding, while fine-tuning on a specific dataset focuses it on the target task. Determining the optimal balance often requires experimentation and validation with evaluation metrics. By assessing the model's performance and iteratively adjusting the fine-tuning process, we can strike the right balance to ensure optimal accuracy and reliability in ChatGPT's decision-making abilities.
Thanks for highlighting the importance of the balance, Victoria. Everett, how can the fine-tuning process be adjusted to enhance ChatGPT's accuracy during operations research?
Hello, Emily! Adjusting the fine-tuning process can enhance ChatGPT's accuracy during operations research. Fine-tuning adaptations can include experimenting with different hyperparameters, such as learning rates, batch sizes, or regularization techniques to guide the model's convergence. Conducting ablation studies to identify more effective subsets of the training data and domain-specific optimization approaches can also contribute to higher accuracy. By iteratively refining the fine-tuning process and evaluating the model's performance against relevant evaluation metrics, we can achieve better accuracy in ChatGPT's recommendations for operations research use cases.
Great job, Everett! ChatGPT has the potential to revolutionize operations research. I wonder if there are any plans to make it accessible to non-technical users, enabling them to benefit from AI-based decision support?
Thank you, Daniel! Making ChatGPT accessible to non-technical users is indeed an important goal. Efforts are being made to develop user-friendly interfaces, simplify AI adoption, and hide the complexity of the underlying model. By building intuitive visualization tools, incorporating natural language interfaces, and facilitating seamless integration into existing software, we aim to empower non-technical users to leverage the benefits of AI-based decision support in operations research. Increasing accessibility is crucial for widespread adoption and impact!
I share Samantha's curiosity about real-time decision-making with ChatGPT, Everett. Thanks for addressing that! In addition, how does ChatGPT handle uncertainties in dynamic systems where new factors can emerge and change the decision landscape?
Hello, Monica! Handling uncertainties in dynamic systems is a significant aspect of ChatGPT's capabilities. As new factors emerge and impact the decision landscape, ChatGPT can adapt by refining its insights through reinforcement learning and continuous learning. It can learn from historical data and incorporate new information to provide up-to-date recommendations. The ability to handle evolving circumstances is essential in dynamic operations research scenarios, and ChatGPT's continuous learning approach enables it to address such challenges effectively.
Thanks for addressing the accessibility aspect, Daniel. Everett, how can the user-friendly interfaces of ChatGPT be designed to cater to non-technical users effectively?
Hello, Andrew! Designing user-friendly interfaces for ChatGPT involves understanding the needs and expertise of non-technical users. Intuitive visualizations, interactive dashboards, and natural language interfaces can make the system more accessible. The interfaces should focus on presenting the insights and recommendations provided by ChatGPT in a clear and understandable manner, avoiding technical jargon and complex visualizations. User testing, feedback, and iterative design cycles help refine the interfaces to cater to a wider range of users, ultimately making AI-based decision support more accessible to non-technical users in operations research.
Thanks for addressing Monica's question, Everett. I'm also interested to know how ChatGPT handles uncertainties in dynamic systems. Can it adapt to sudden changes swiftly?
Hi Owen! ChatGPT's ability to handle uncertainties in dynamic systems includes adapting to sudden changes swiftly. However, the extent of its adaptability depends on the availability and quality of data representing the sudden changes. If the data adequately captures the new factors influencing the decision landscape, ChatGPT can adapt and refine its insights accordingly. The continuous learning aspect ensures that as new data becomes available, the model keeps improving and can handle unforeseen factors more effectively. Swift adaptation is vital in dynamic operations research scenarios, and ChatGPT's design accounts for this requirement.
Your insights on adapting to sudden changes are insightful, Owen. Everett, how does ChatGPT handle evolving circumstances with limited historical data available?
Hello, Madison! When handling evolving circumstances with limited historical data available, ChatGPT relies on its ability to generalize from the existing data while incorporating new information. Although limited historical data may present challenges, ChatGPT can utilize transfer learning, leveraging knowledge accumulated from pre-training on extensive corpora. By incorporating new information iteratively and continuously refining its insights through reinforcement learning, ChatGPT's recommendations can adapt to evolving circumstances even without an extensive historical record. The adaptability provides valuable insights in operations research, enabling decision-makers to navigate uncertain scenarios with limited historical data.
Your insights on user-friendly interfaces are valuable, Andrew. Everett, how can organizations ensure that the AI-based decision support provided by ChatGPT aligns with the specific needs of non-technical users?
Hi Jacob! Aligning the AI-based decision support provided by ChatGPT with the specific needs of non-technical users involves user-centered design principles. Organizations must engage with non-technical users throughout the design and development process, incorporating their input, needs, and feedback. Conducting user research, usability testing, and iterative design cycles help understand and address users' pain points and preferences. Customizing the interface based on user profiles, allowing personalization, and providing clear explanations of the system's outputs contribute to ensuring that AI-based decision support caters to the specific requirements of non-technical users in operations research.
Thank you all for taking the time to read my article! I hope you find it interesting.
Great article, Everett! I agree that ChatGPT has the potential to revolutionize Operations Research in technology.
Thank you, Rachel. I believe that ChatGPT can automate the decision-making process in various areas such as supply chain optimization, resource allocation, and scheduling.
How does ChatGPT handle uncertainty and variability in data, Everett?
Rachel, ChatGPT can handle uncertainty by incorporating probabilistic models and methods to account for variability in data and provide robust and reliable recommendations.
That's impressive, Everett. Thanks for the explanation.
I'm curious about the practical applications of ChatGPT in Operations Research. Can you provide some examples, Everett?
This sounds promising, Everett! Do you think ChatGPT can effectively handle large-scale optimization problems?
Sarah, that's a valid concern. ChatGPT's performance with large-scale problems depends on the underlying optimization algorithms it employs. With advanced algorithms, it can handle complex optimization tasks.
Thanks for clarifying, Everett. I see the potential in using ChatGPT for optimization tasks now.
You've eased my reservations, Everett. I can see the value in leveraging ChatGPT for optimization.
I understand the potential benefits of using ChatGPT, but how do you ensure the accuracy and reliability of its recommendations?
Emily, excellent question. The accuracy and reliability of ChatGPT's recommendations heavily rely on the quality and relevance of the input data. Proper validation and testing procedures are key to ensure trustworthy results.
I'm concerned about the interpretability of ChatGPT's recommendations. Will it be transparent enough to explain the reasoning behind its decisions?
Mark, interpretability is indeed an important aspect. While ChatGPT itself might not provide explicit explanations, efforts can be made to design post-processing techniques to enhance transparency and interpretability.
Everett, have you encountered any challenges while implementing ChatGPT in real-world Operations Research scenarios?
Julia, one of the challenges is handling domain-specific constraints effectively. It requires fine-tuning the AI models and incorporating the necessary knowledge to ensure practical usability.
Thanks, Everett. The application of ChatGPT in supply chain optimization seems particularly intriguing.
Thank you, Everett. I appreciate your insights.
Thank you, Everett. Addressing interpretability concerns post-processing techniques could be an interesting research area.
As an OR practitioner, I'm excited about the potential of integrating ChatGPT into our decision-making processes. It could bring new insights and enhance efficiency.
I have concerns about the ethical implications of relying on AI models like ChatGPT for critical decision-making. What steps can be taken to address this?
Linda, ethics is a crucial aspect. Implementing proper governance, transparency, and accountability frameworks can help address the ethical concerns associated with AI decision-making.
How scalable is ChatGPT? Can it handle real-time decision-making in high-demand environments?
Daniel, ChatGPT's scalability depends on factors like computational resources and optimization algorithms used. With proper infrastructure, it can handle real-time decision-making in high-demand environments.
Do you think ChatGPT can replace human decision-making in Operations Research, or is it better seen as a decision support tool?
Sophia, ChatGPT should be seen as a decision support tool. Human expertise and judgment are still important, but ChatGPT can assist in generating insights and exploring potential solutions.
I'm curious about the implementation challenges when integrating ChatGPT into existing OR systems. Can you share any experiences related to this, Everett?
Oliver, integrating ChatGPT into existing OR systems can involve challenges such as data integration, model compatibility, and system architecture. It's crucial to ensure seamless integration and performance.
What are the potential risks associated with implementing ChatGPT in Operations Research, Everett?
Michelle, potential risks include overreliance on AI recommendations without human validation, biases in data leading to biased decisions, and system failures or vulnerabilities. Proper risk assessment and mitigation strategies are necessary.
I appreciate your insights, Everett. Mitigating potential risks is essential to ensure responsible AI adoption.
Michelle, absolutely. Responsible AI adoption should be a priority in all decision-making processes.
Are there any specific industrial sectors where ChatGPT has shown promising results in OR, Everett?
Ella, ChatGPT has shown promising results in sectors like logistics, manufacturing, transportation, and healthcare, to name a few. Its versatility allows for applicability across various industries.
What are the limitations we should be aware of when adopting ChatGPT in Operations Research, Everett?
Ryan, limitations include the need for large amounts of quality training data, potential biases in data leading to biased recommendations, and limitations in scalability and integration with existing systems.
Everett, what future developments or directions do you see for ChatGPT in OR?
Liam, future developments could focus on improving interpretability, addressing bias concerns, enhancing scalability, and integrating multiple AI models or algorithms to provide more robust decision support in complex OR domains.
How about the time and effort required to implement ChatGPT in real-world OR scenarios, Everett?
Sophie, implementing ChatGPT in real-world scenarios requires significant time and effort in data preparation, model development, integration, and validation. However, the potential benefits justify the investment.
Great article, Everett! I'm excited about the possibilities ChatGPT offers in the field of OR.
Thank you, Alex. The intersection of AI and OR indeed holds incredible potential.
ChatGPT seems like a powerful tool. What level of expertise is required to effectively utilize it in OR, Everett?
Freya, while expertise in Operations Research is beneficial, a deep understanding of the underlying AI concepts, data preparation, and system integration is crucial to effectively utilize ChatGPT in OR.
Everett, what are some of the existing OR tools that can be augmented by ChatGPT?
David, ChatGPT can augment existing OR tools like mathematical programming solvers, simulation software, and optimization libraries; enhancing the decision support capabilities by incorporating natural language interactions.
I'm interested in the impact of ChatGPT on human decision-makers. How can we ensure it complements human expertise instead of replacing it, Everett?
Sophia, proper framing and positioning of ChatGPT as a decision support tool, emphasizing the role of human expertise and judgment, and encouraging collaboration between AI and human decision-makers can ensure its complementarity instead of replacement.
Thank you all for an engaging discussion! Your questions and perspectives provided valuable insights. I'm glad to see the enthusiasm surrounding the potential of ChatGPT in Operations Research.