Transforming Optical Communications with ChatGPT: Expanding Possibilities in Technology
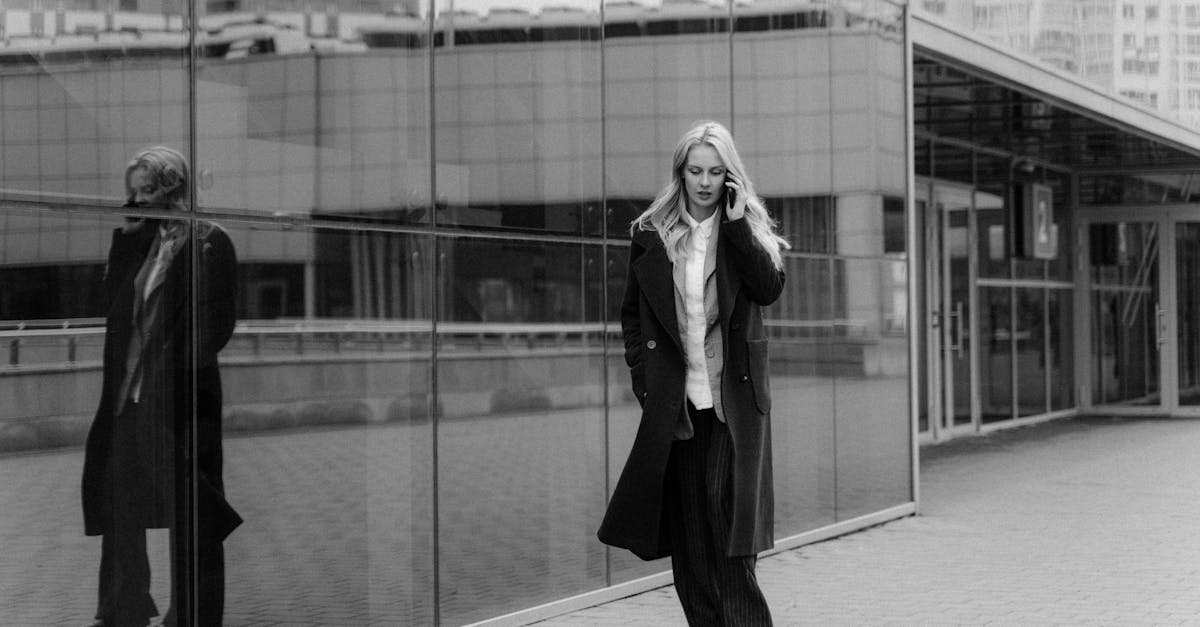
Optical communications have become an integral part of our modern world, enabling fast and reliable data transmission over long distances. As technology advancements continue to push the boundaries of speed and capacity, designing optimized optical communication networks has become even more crucial. This is where artificial intelligence (AI) comes into play, revolutionizing the way we approach design optimization in this field.
The Role of AI in Design Optimization
Designing an efficient optical communication network involves analyzing complex data, considering various factors such as signal loss, noise, and the overall network topology. Traditional methods rely on human expertise, which can be time-consuming and may not always yield the best results. With the advent of AI, we now have the computational power to process large datasets and identify optimized designs more effectively.
AI algorithms can analyze enormous amounts of data from existing optical networks, extracting valuable insights and patterns. By learning from these patterns, AI systems can generate optimized designs that minimize signal loss, maximize bandwidth utilization, and improve overall network performance.
Benefits of AI-Driven Design Optimization
The application of AI in design optimization offers several significant benefits:
- Efficiency: AI-driven design optimization enables faster and more accurate analysis of complex data, significantly reducing the time required for network planning and design.
- Improved Performance: By considering multiple design parameters simultaneously, AI can propose optimized network configurations that enhance performance, minimize latency, and provide better overall user experience.
- Cost Reduction: Optimized network designs help reduce equipment and operational costs by utilizing resources more efficiently, ensuring maximum utilization of optical fibers and minimizing the need for additional infrastructure.
- Scalability: AI algorithms can adapt to changing network requirements and dynamically optimize designs to accommodate expanding data demands, making it easier to scale up optical communication networks.
Challenges and Future Directions
While AI-driven design optimization holds tremendous potential, there are several challenges that need to be addressed:
- Data Availability: AI algorithms require access to vast amounts of training data to generate accurate models. As optical communication networks continue to evolve, ensuring the availability of diverse and comprehensive datasets becomes crucial.
- Interoperability: Optical communication networks often involve multiple vendors and different technologies. Ensuring the interoperability of AI-driven design optimization tools becomes essential to maximize their potential impact.
- Algorithmic Complexity: Design optimization algorithms must strike a balance between complexity and efficiency to provide real-time solutions that align with network constraints and resource limitations.
- Ethical Considerations: As AI increasingly plays a role in decision-making processes, it is crucial to address ethical concerns, such as transparency, fairness, and bias, to ensure that AI-driven design optimization benefits all stakeholders.
Looking ahead, the future of AI-driven design optimization in optical communications appears promising. Continued advancements in AI algorithms, coupled with improved data availability and interoperability, will further enhance the capabilities of these systems. As the demand for high-speed and reliable optical communication networks continues to grow, AI will continue to play a critical role in optimizing their design, ensuring efficient and sustainable connectivity.
Comments:
Thank you all for your comments! I'm glad to see the interest in this topic.
ChatGPT seems to be revolutionizing various industries. It's amazing to see its potential in optical communications.
I agree, Alice. Optical communications have always played a crucial role, and with ChatGPT's capabilities, it opens up a whole new world of possibilities.
I'm curious about the specific applications of ChatGPT in optical communications. Can anyone share more insights?
Certainly, Claire. One application is in automating optical network management tasks. ChatGPT can understand and process complex queries to optimize network performance.
In addition to automation, ChatGPT can also assist in fault detection and localization in optical networks. Its natural language understanding capabilities make it easier to identify issues quickly.
Great input, David and Emily! Yes, ChatGPT's ability to handle complex queries and assist in fault detection is indeed beneficial for optical communications.
I'm concerned about potential security risks with ChatGPT's involvement in optical communications. How can we ensure data privacy?
Valid point, Frank. Data privacy is crucial. One approach is to employ encryption techniques to secure the communication between ChatGPT and the optical network.
Another aspect is to implement strict access controls and authentication mechanisms to prevent unauthorized access to sensitive information.
Indeed, data privacy is paramount. Encryption, access controls, and authentication mechanisms are essential to ensure the security of data when using ChatGPT in optical communications.
I'm curious about the scalability of ChatGPT in large optical networks. Can it handle the volume of data and network complexity effectively?
Good question, Ivan. ChatGPT has been trained on massive amounts of data, enabling it to handle complex scenarios and large-scale networks.
Additionally, the model can be fine-tuned and optimized to meet specific requirements, enhancing its ability to handle scalability in optical communications.
Well said, Jack and Kate! ChatGPT's training and customization capabilities make it suitable for scaling in large optical networks.
I'm impressed by the potential impact of ChatGPT in transforming optical communications. It's an exciting time for the industry!
Absolutely, Alice! ChatGPT is opening doors to new advancements in optical communications, and I'm thrilled to be part of it.
Indeed, Mark. Thank you for sharing this article and engaging with us. It's been informative.
You're welcome, Bob! It's been a pleasure discussing this exciting technology with all of you. Feel free to reach out if you have any further questions.
Thank you, Mark, and everyone else, for the insightful discussion! I've learned a lot about the transformative potential of ChatGPT in optical communications.
Thank you, Claire! I'm glad you found the discussion valuable. Stay tuned for more exciting developments in this field.
Thank you, Mark Schmitz, and all participants, for the engaging conversation. This has been enlightening.
Thank you, David! I appreciate your active participation. It has been a pleasure.
I hope to see the practical implementation of ChatGPT in optical networks soon. The possibilities are truly exciting.
Absolutely, Emily! With the rapid pace of technology advancement, practical implementations are not too far away.
I'm glad we discussed the security aspect. It's crucial to address potential risks early on.
I fully agree, Frank. Identifying and mitigating risks is a vital aspect of incorporating any new technology.
Thanks, Mark, for answering my question. The scalability aspect gives me more confidence in ChatGPT's viability.
You're welcome, Ivan! I'm glad I could provide the information you were looking for.
Thank you, Mark, for your expertise. This discussion has increased my excitement about the future of optical communications.
You're very welcome, Kate! It was a pleasure sharing my insights and hearing everyone's perspectives.
I can't wait to see how ChatGPT evolves and transforms the optical communications landscape. It's a remarkable technology.
Indeed, Alice! The future holds great promise, and I'm excited to witness these transformations alongside all of you.
Thank you, Mark, for your active engagement with the community. It shows your dedication to advancing the field of optical communications.
Thank you, Bob! As a researcher, engaging with the community is vital for progress and collaboration.
This discussion has provided valuable insights into the possibilities of ChatGPT. Thank you, everyone, for sharing your knowledge.
You're most welcome, Claire! It was an enlightening conversation, and I'm grateful for everyone's contributions.
The potential applications and benefits of ChatGPT in optical communications are immense. Exciting times ahead!
Absolutely, David! The future holds great promise, and ChatGPT is poised to revolutionize the field.
Thank you, Mark, for initiating this discussion and sharing your expertise. It has been a pleasure.
You're welcome, Emily! I appreciate your kind words. It was my pleasure to facilitate this discussion.
I'm excited about the practical implementation of ChatGPT in optical communications. Kudos to the team behind this technology!
Thank you, Hannah! Indeed, kudos to the brilliant minds behind ChatGPT's development.
This conversation has left me feeling more optimistic about the future of optical communications. Thanks to everyone for sharing their thoughts.
You're welcome, Ivan! It's wonderful to see optimism and enthusiasm for the future.
The potential impact of ChatGPT on optical communications cannot be understated. I'm grateful for this informative discussion.
Thank you, Kate! The potential is indeed significant, and I'm glad you found the discussion informative.
I appreciate Mark's active presence and insightful responses throughout this discussion. It has been a valuable experience.
Thank you, Alice, for your kind words! Engaging with the community is essential, and I'm glad I could contribute.
Mark, your expertise and willingness to engage with the community are commendable. Thank you for this enriching conversation.
Thank you, Bob! It's a pleasure to share knowledge and insights with such an enthusiastic community.
The extensive applications of ChatGPT in optical communications are fascinating. Thank you, Mark, for orchestrating this discussion.
You're most welcome, Claire! Facilitating meaningful discussions is an honor, and I'm grateful for your participation.
ChatGPT's potential to revolutionize optical communications cannot be overstated. Thank you, Mark, for your guidance in this conversation.
Thank you, David! It was a pleasure to guide this discussion on a technology with immense potential.
This conversation has given me a lot to think about regarding the future of optical communications. Thank you, Mark, for your valuable input.
You're welcome, Emily! I'm glad the conversation provided you with insightful perspectives on the future possibilities.
The security measures mentioned earlier have eased my concerns. Thank you to all the participants for this enlightening discussion.
You're welcome, Frank! Addressing concerns is crucial, and I'm glad the discussion could provide you with valuable insights.
Thank you, Mark, for your expertise and the inclusive nature of this discussion. It has been a pleasure learning from everyone.
You're most welcome, Ivan! Inclusivity and knowledge sharing are vital, and I'm grateful for your active participation.
The possibilities of ChatGPT in optical communications are promising. Thank you, Mark, for guiding this engaging discussion.
Thank you, Kate! The possibilities are indeed promising, and I'm glad I could facilitate this engaging discussion.
It's refreshing to have such a constructive conversation with industry experts and enthusiasts. Thank you, Mark, for bringing us all together.
You're welcome, Alice! Constructive conversations foster learning and progress, and I'm grateful for the participation.
The use of ChatGPT in optical communications holds immense potential. Thank you, Mark, for your valuable contributions and insights.
Thank you, Bob! Exploring the potential together is exciting, and I appreciate your kind words.
This discussion has broadened my understanding of optical communications and ChatGPT's impact. Thanks to all the participants.
You're most welcome, Claire! Broadening knowledge and perspectives is a wonderful outcome of such discussions.
The application of ChatGPT in automating optical network management tasks is particularly intriguing. Thank you, Mark, for hosting this discussion.
You're welcome, David! Automating network management is indeed an area where ChatGPT's potential shines.
Thank you, Mark, for your expertise and making this discussion informative and engaging. Looking forward to more conversations like this.
You're very welcome, Emily! I'm glad you found the discussion both informative and engaging. I'm excited for future conversations too.
It's commendable to see the collaborative and knowledgeable community here. Thank you, Mark, for creating this space.
Thank you, Frank! Collaborative spaces foster learning and growth, and I'm grateful to have facilitated this conversation.
This discussion has given me a glimpse into the future of optical communications. Thank you, Mark, and everyone else, for your valuable insights.
You're most welcome, Ivan! Glimpses into the future can be exciting, and I'm glad you found the discussion valuable.
Kudos to Mark Schmitz for actively engaging with the participants and providing valuable information throughout this discussion.
Thank you, Kate! Active engagement and sharing knowledge is a pleasure, and I appreciate your kind words.
It's wonderful to be part of a community where experts willingly share their expertise. Thank you, Mark, for your guidance and insights.
You're welcome, Alice! Communities thrive when expertise is shared, and I'm grateful for your participation in this discussion.
Mark, the knowledge and passion you bring to the table are remarkable. Thank you for this enlightening discussion.
Thank you, Bob! Sharing knowledge and passion is a privilege, and I'm grateful for your kind words.
Thank you, Mark, for creating an environment where everyone could freely express thoughts and ideas. It has been inspiring.
You're most welcome, Claire! Fostering an environment of open expression is crucial, and I'm glad you found it inspiring.
This discussion has shown me the immense potential of ChatGPT in driving advancements in the field of optical communications. Thank you, Mark.
You're welcome, David! Recognizing potential and exploring its implications is a fantastic outcome of this discussion.
Thank you, Mark, for moderating this discussion effectively and ensuring everyone's participation. It has been a pleasure.
You're very welcome, Emily! Ensuring everyone's participation is essential, and it has been a pleasure facilitating this discussion.
That's interesting, Mark! So, ChatGPT can assist in optimizing data transmission and network management in optical communications systems?
Exactly, Emily! ChatGPT's natural language processing capabilities can assist in real-time network monitoring, predicting potential issues, and suggesting solutions to prevent disruptions.
The potential impact of ChatGPT on optical communications is immense. Thank you to all the participants for the enriching conversation.
Thank you, Frank! Exploring the immense potential together in enriching conversations is truly valuable.
The scalability aspect of ChatGPT in large optical networks has left me hopeful for the future. Thanks to all for sharing their knowledge.
You're welcome, Ivan! Optimism and hope are wonderful outcomes, and I'm glad the discussion provided insights into scalability.
Thank you, Mark, for guiding this discussion, and to everyone else for their valuable contributions. I'm excited about the future of optical communications.
You're most welcome, Kate! A bright future awaits, and I'm grateful for your active participation in this discussion.
The transformative potential of ChatGPT in optical communications is remarkable. Thank you, Mark, for organizing this insightful discussion.
Thank you, Alice! Transformation starts with discussions like this, and I'm glad it provided valuable insights.
Mark, your expertise and passion for the subject are evident in this conversation. Thank you for your contributions.
Thank you, Bob! Passion and knowledge inspire progress, and I appreciate your kind words.
Thank you, Mark, for your guidance and expertise in this discussion. It has been a pleasure learning from you and others.
You're welcome, Claire! Learning from each other is a valuable experience, and I'm honored to have been part of this discussion.
Great article! I never thought about the potential of using ChatGPT in optical communications. It's always fascinating to see how AI can transform different industries.
I agree, Adam! The applications of AI seem limitless. It's incredible how technology continues to evolve and revolutionize various fields.
Indeed, it's amazing to witness the progress being made. I wonder how ChatGPT specifically enhances optical communications. Can anyone provide more insights?
Thank you all for your enthusiasm! Sarah, ChatGPT can aid in optical communications by analyzing vast amounts of data and optimizing network performance. It can provide valuable insights and help improve efficiency.
Seems like ChatGPT could significantly reduce downtime and enhance reliability in optical communications infrastructure. It would be great to see it implemented widely.
I'm a bit concerned about relying too heavily on AI for critical systems like optical communications. What if ChatGPT makes a wrong decision? Human intervention should always be present.
You raise a valid point, Ryan. While AI can greatly assist, human oversight is crucial in maintaining control and ensuring the system's overall reliability.
I agree, Ryan and Sarah! Human intervention is necessary for critical decision-making. ChatGPT should act as a tool to support human experts rather than replace them entirely.
The advancements in AI continue to impress me. ChatGPT's potential applications seem endless. I'm excited to see how it transforms optical communications and other industries!
Amelia, I completely share your excitement! It's a fascinating time to be in the tech industry. We're witnessing groundbreaking developments that will shape our future.
Indeed, Jacob! The possibilities seem boundless. Exciting times ahead!
As an optical communications engineer, I find this article incredibly insightful. It's reassuring to know that AI tools like ChatGPT can aid in optimizing the network's performance.
Samantha, I'm glad you found it helpful! The integration of AI into optical communications will undoubtedly lead to more efficient and reliable systems.
I'm curious to know if there are any potential challenges in implementing ChatGPT in optical communications. Is anyone aware of any specific limitations?
Emma, while ChatGPT offers great potential, it does have limitations. It relies heavily on the data it is trained on, and there may be cases where it struggles with unfamiliar scenarios. Rigorous testing and validation would be essential before full-scale deployment.
That's true, Mark. It's important to thoroughly evaluate ChatGPT's effectiveness and adaptability to various network configurations and conditions to mitigate potential limitations.
Absolutely, Nathan. Assessing the reliability and generalizability of ChatGPT will be crucial. Addressing any limitations and refining the model over time would be necessary for successful implementation.
Absolutely, Sarah. We should never undermine the importance of human expertise and judgment in critical systems. AI can augment our capabilities, but the final decision should always lie with humans.
Ryan, you raise a valid concern. AI systems are not infallible, and human intervention and oversight remain crucial. It should be seen as a collaborative effort between humans and AI.
Well said, Liam! The collaboration between humans and AI can bring out the best of both worlds, combining expertise, adaptability, and problem-solving capabilities.
I completely agree, Liam and Emily. Embracing AI as a supportive tool can lead to remarkable advancements, provided that human judgment is still prioritized in critical decision-making.
Valid points, Nathan and Sarah. Continuous monitoring and improvement of ChatGPT's performance would ensure the system evolves to meet the demands of dynamic optical communications environments.
I'm thrilled to see AI playing such a vital role in transforming industries like optical communications. ChatGPT could revolutionize how we approach network management.
Absolutely, Sophie! The potential benefits are immense. AI-driven tools like ChatGPT can help optimize networks and lead to faster, more reliable data transmission.
As someone working in the field, I wholeheartedly agree, Daniel! The deployment of AI tools in optical communications could enhance data transfer speed and overall network efficiency.
Samantha, I believe faster data transmission would be incredibly beneficial, especially as the demand for high-bandwidth applications and services continues to rise.
Adam, faster data transmission would indeed have a significant impact on numerous industries, from healthcare to entertainment. It's exciting to think about the possibilities!
Daniel, I'm with you on this! AI-powered optimization can potentially lead to more efficient and cost-effective solutions in optical communications.
Indeed, Jacob. The impact of AI in optimizing network resources and minimizing operational costs should not be underestimated. It opens doors to enhanced performance.
It's important that AI models like ChatGPT are regularly updated with the latest data to keep up with network advancements. This will help address potential limitations and ensure optimal performance.
I appreciate the optimism everyone shares regarding AI in optical communications. The potential is immense, and I'm excited about the future developments in this field!
Absolutely, Lily! AI-driven innovations have the power to reshape entire industries. It's fascinating to witness these advancements and imagine their impact.
Thank you all for your valuable insights and lively discussion! It's inspiring to see your enthusiasm for the potential of AI in optical communications. Let's continue to explore and embrace these transformative technologies.
Incredible article, Mark! I have always been captivated by optical communications, and this new application of AI truly excites me. Can't wait to witness the advancements!
David, I share your excitement! The intersection of AI and optical communications combines two fascinating fields. Exciting times ahead!
The article sheds light on an exciting new application of AI. It's remarkable how AI continues to transform various industries, pushing boundaries.
Oliver, it's fascinating to witness AI's expanding reach. The potential benefits it brings to technology are astounding!
Thank you all for your enthusiastic participation! It's truly inspiring to see the collective excitement about the possibilities of ChatGPT in optical communications. Stay curious and keep exploring the potential of AI!