Transforming Pharmacovigilance: Leveraging Gemini for Technological Surveillance
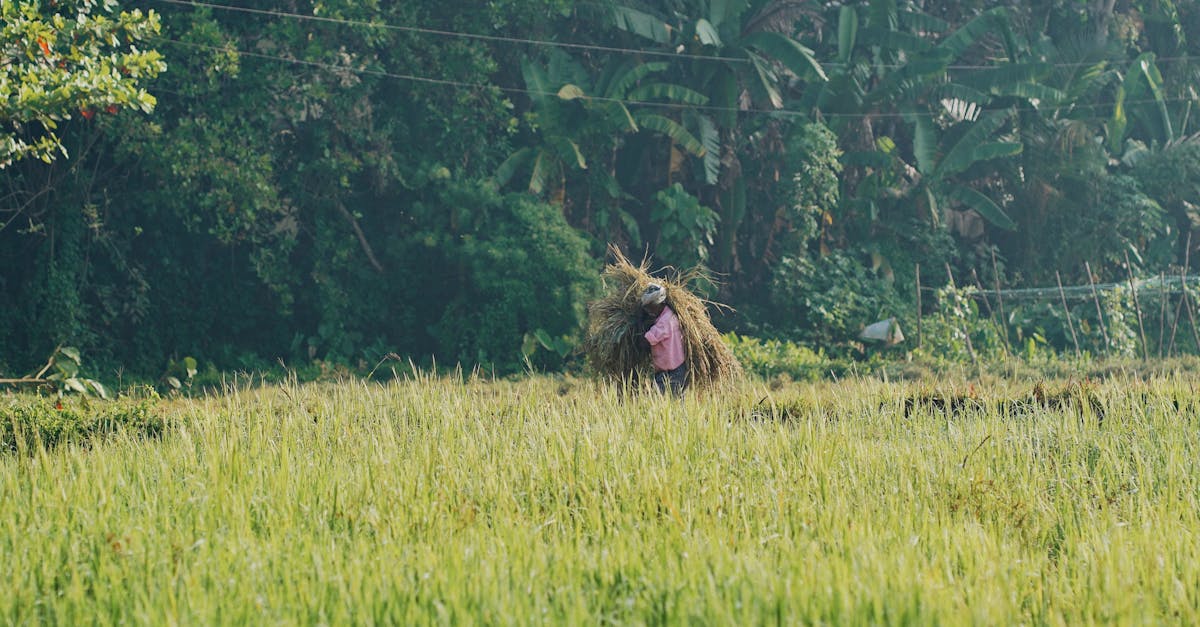
Pharmacovigilance, the science and activities related to the detection, assessment, understanding, and prevention of adverse effects or any other medicine-related problem, is a critical aspect of healthcare. As the pharmaceutical industry continues to grow, it is essential to find innovative ways to improve pharmacovigilance processes and ensure the utmost safety for patients.
With the emergence of Artificial Intelligence (AI) technologies, the field of pharmacovigilance has witnessed significant advancements. One such technology that holds great promise is Gemini, a language model developed by Google. Gemini utilizes Natural Language Processing (NLP) and machine learning algorithms to understand and generate human-like responses, making it an ideal tool for technological surveillance in pharmacovigilance.
The application of Gemini in pharmacovigilance allows for enhanced real-time monitoring of adverse drug reactions (ADRs) and potential drug interactions. By leveraging large datasets and learning from vast amounts of medical literature, Gemini can provide valuable insights and facilitate early detection of safety concerns. This technology has the potential to revolutionize pharmacovigilance by enabling proactive interventions and preventive measures.
One of the key advantages of implementing Gemini in pharmacovigilance is its ability to handle complex and unstructured data. Traditional methods often struggle with identifying and extracting relevant information from various sources, such as social media, patient forums, and medical literature. Gemini, on the other hand, excels in understanding context, nuances, and extracting meaningful insights from a diverse range of textual data.
Furthermore, Gemini can assist healthcare professionals in categorizing and prioritizing ADR reports based on severity and urgency. By automating this process, pharmacovigilance teams can focus their efforts on analyzing the most critical cases and responding promptly. This not only saves time but also ensures a more efficient allocation of resources.
Another significant benefit is the potential to improve patient outcomes through personalized medicine and tailored treatment plans. Gemini can analyze patient-specific data, including medical history, genetic profiles, and current medications, to identify potential risks and suggest personalized interventions. This individualized approach enhances patient safety and minimizes the possibility of adverse reactions or negative interactions.
While Gemini proves to be an invaluable asset in technological surveillance for pharmacovigilance, it is crucial to acknowledge certain limitations. As an AI model, Gemini relies on the data it has been trained on, and it may not be aware of the most recent medical advancements or rare/unknown adverse effects. Therefore, continuous monitoring, validation, and updates are essential to ensure accuracy and efficacy.
In conclusion, the integration of Gemini in pharmacovigilance opens up new avenues for improving patient safety and healthcare outcomes. By harnessing the power of AI and NLP, we can enhance real-time monitoring, early detection, and personalized interventions, transforming the way we conduct pharmacovigilance. However, it is vital to strike a balance between AI-driven automation and human expertise to overcome limitations and provide the best possible care for patients.
Comments:
Thank you all for taking the time to read my article! I'm excited to discuss this topic with you.
Great article, Eric! Pharmacovigilance is indeed an important aspect of drug safety. Leveraging AI and chatbots could significantly enhance surveillance. Do you think there are any challenges in implementing this technology?
Sarah, regarding your question about challenges, one potential challenge I see is the need for continuous training and updating of the chatbot to keep up with new drugs and adverse event reporting guidelines. It requires ongoing efforts from developers and experts.
Oliver, I agree. The dynamic nature of drug information and guidelines requires continuous updates to ensure the chatbot provides accurate and up-to-date responses. Collaboration between pharmacovigilance experts and developers is essential for successful implementation.
Olivia, close collaboration with pharmacovigilance experts, along with continuous training, validation, and feedback loops, can ensure the chatbot remains up to date and trustworthy.
I agree, Sarah. While AI-based surveillance systems can greatly streamline the process, there might be some concerns about the accuracy and reliability of the chatbot responses. Eric, any thoughts on this?
Robert, I believe having a robust validation process in place can address concerns about accuracy. Regular monitoring and fine-tuning can help ensure the chatbot's responses align with expert judgments.
Kevin, I agree. Proper validation against expert judgments and continuous training can ensure the chatbot performs consistently and meets the required accuracy standards in pharmacovigilance.
Robert, I agree that accuracy and reliability are critical. It would be helpful to have strict protocols in place to validate the AI's outputs against expert judgments and continuously evaluate its performance.
Rachel, adopting robust protocols for validation and evaluation can help minimize any bias and ensure the chatbot aligns with expert judgments, enhancing the reliability of its responses.
Sarah and Robert, you both bring up valid points. Implementing AI in pharmacovigilance does come with challenges. It's necessary to ensure the chatbot's training and validation processes are robust to maintain accuracy and reliability. Regular updates and monitoring can help address these concerns.
I'm impressed with the potential of AI in pharmacovigilance. It could greatly improve adverse event reporting and analysis. However, privacy and data protection are major concerns. How can these be addressed?
That's an important concern, Lisa. Privacy and data protection should be given utmost priority. The data collected must comply with applicable regulations, and measures, such as encrypting sensitive information and implementing access controls, can be taken to protect patient privacy. Transparency about data usage is also crucial.
Lisa, to address privacy concerns, the system could use anonymized data wherever possible and ensure strict access controls. Implementing strong encryption during data transmission and storage is crucial. Users should also be informed and given control over their data usage.
Addressing privacy concerns is crucial, Lisa. To mitigate risks, transparency about data collection, storage, and usage should be maintained. Implementing strong security protocols and obtaining user consent can help gain trust and ensure data protection.
I see the potential for AI to automate the monitoring process, but can chatbots truly replace human vigilance? Human judgment and experience play a significant role in recognizing subtle patterns and understanding complex cases.
You raise an important point, David. AI and chatbots can assist human vigilance, but they should not replace it entirely. The goal is to enhance pharmacovigilance by automating repetitive tasks and aiding experts in data analysis, allowing them to focus on critical cases that require human judgment and experience.
Eric, your response makes sense. Human expertise and AI should work hand in hand for effective pharmacovigilance. Collaborative efforts can leverage the strengths of both approaches.
David, while chatbots can't replace human vigilance, they can augment it. By analyzing large volumes of data quickly, they can provide early warnings and indicators to human experts, enabling faster and more proactive pharmacovigilance.
Elizabeth, early warning systems powered by AI can provide valuable insights to healthcare professionals, enabling them to identify adverse events and potential safety concerns before they escalate.
Jason, precisely. The ability of AI systems to process large amounts of data quickly can enable healthcare professionals to intervene early and prevent potential harm.
Elizabeth, exactly. Early identification of adverse events can prevent harm and improve patient safety. AI can enhance pharmacovigilance by providing healthcare professionals with timely insights and alerts.
Elizabeth, proper encryption is crucial to protect sensitive data. Additionally, strict authorization controls should be implemented to limit access to patient information and prevent unauthorized use.
Sophia, I completely agree. Ensuring transparency and giving users control over their data can help build trust and confidence in AI-based pharmacovigilance systems.
I can see the benefits of leveraging AI and chatbots, but what about potential biases in the data used for training? How can we ensure that the surveillance system doesn't amplify existing biases or overlook certain demographics?
Valid concern, Amy. Bias in data can lead to biased outputs. When training the chatbot, it is crucial to use diverse and representative datasets to minimize bias. Regular audits and reviews can help identify and address any unintended biases in the system. Maintaining transparency and involving experts from various backgrounds in the development process can also help mitigate this issue.
Amy, ensuring the data used for training is representative of different demographics is vital. By actively seeking diverse data sources and involving experts from various backgrounds, we can minimize biases and make the surveillance system more inclusive and equitable.
Ryan, involving diverse experts not only helps minimize biases but also allows for a broader perspective in analyzing adverse events and recognizing patterns.
Ryan, inclusivity is crucial to avoid biased surveillance. AI and chatbot developers should actively seek data from underrepresented populations to ensure the system caters to all demographics effectively.
Amy, addressing biases in training data is crucial to prevent amplification of existing biases. Regular audits and external evaluations can help identify and rectify any biases that may arise during the system's operation.
I appreciate the potential of AI in pharmacovigilance, but how do you see this technology impacting the work of healthcare professionals in terms of workload and job responsibilities?
Great question, Michelle. AI can alleviate the workload of healthcare professionals by automating routine tasks, allowing them to focus on more critical aspects like decision-making and patient care. They can benefit from the insights and analysis provided by AI systems, aiding in faster and more informed decision-making. Collaboration between AI technology and healthcare professionals is key to achieving optimal outcomes.
Eric, with AI handling routine tasks, healthcare professionals may have more time for patient interaction and personalized care. It has the potential to enhance their role rather than replace it.
Eric, your article was insightful, and it's great to see the potential of AI in transforming pharmacovigilance. I appreciate your valuable input on the challenges and considerations involved in implementing this technology.
Sarah, another challenge could be user acceptance and trust in AI-based systems. Educating healthcare professionals and patients about the benefits, limitations, and ethical considerations of AI in pharmacovigilance is crucial for widespread adoption.
Sarah, continuous training and updating are essential to keep up with the fast-paced advancements in pharmacovigilance. A collaborative approach between developers, regulators, and healthcare professionals can help address this ongoing challenge.
Oliver, collaboration between developers and experts is indeed crucial. Regular evaluation and feedback from healthcare professionals can help improve the chatbot's performance and keep it aligned with evolving pharmacovigilance guidelines.
Sarah, Eric did a great job highlighting the challenges involved in implementing this technology. It's crucial to address these issues for the successful integration of AI in pharmacovigilance.
Eric, your point about regular updates and monitoring is crucial. It ensures the AI system remains accurate and reliable, adapting to changes in adverse event reporting guidelines and incorporating new insights in drug safety.
Robert, I agree. Regular monitoring and recalibration can help ensure the chatbot remains in alignment with expert judgments and maintains a high level of accuracy.
Eric, by automating routine tasks, healthcare professionals can focus on personalized care and deepening patient engagement. The collaboration between AI and healthcare professionals can lead to better health outcomes.
Caroline, encryption and access controls provide layers of security, safeguarding sensitive patient data. User consent and transparency regarding data usage can foster trust in AI-powered pharmacovigilance.
Eric, continuous updates and monitoring are critical to ensure the AI system remains effective in capturing emerging drug safety concerns. As the field evolves, staying up to date with best practices is key.
Robert, updates and monitoring play a crucial role in ensuring AI systems can capture emerging drug safety concerns and adapt to the changing landscape of pharmacovigilance.
Robert, I couldn't agree more. Regular updates and monitoring help AI systems stay relevant and effective in the dynamic field of pharmacovigilance.
Eric, with AI handling routine tasks, healthcare professionals can focus on more complex cases and optimizing patient care. It's a win-win situation that can positively impact both healthcare professionals and patients.
Michelle, AI can help healthcare professionals by providing them with data-driven insights. With more information readily available, healthcare professionals can make better informed decisions and improve patient outcomes.
Michelle, in addition to workload alleviation, AI can enhance the accuracy of diagnosis and treatment recommendations, potentially reducing medical errors and improving overall healthcare quality.
Michelle, AI has the potential to assist healthcare professionals in diagnosing and prescribing treatments more accurately by leveraging vast amounts of medical knowledge and patient data.
I'm glad the article touched upon both the potential benefits and challenges of leveraging AI in pharmacovigilance. It's important to have a balanced perspective.
Great article! I found it really interesting how chatbots can be leveraged for pharmacovigilance.
Yes, it's amazing how AI can help improve drug safety monitoring.
I have some concerns about relying solely on chatbots for pharmacovigilance. Human oversight is still necessary, in my opinion.
I agree with Chris. While chatbots can assist, experienced professionals are crucial to ensure accurate reporting and analysis.
David, you're right. Let's use chatbots to support human professionals, enhancing their capabilities instead of replacing them.
Tina, chatbots can handle repetitive tasks, allowing professionals to focus on complex analyses and decision-making, which is invaluable in pharmacovigilance.
Thank you, Alice and David, for your comments! While chatbots can automate certain tasks, human expertise remains essential in pharmacovigilance.
Chatbots might complement human involvement, but they should not replace it entirely. People's lives are at stake here.
Eva, I see chatbots as tools to assist professionals, making the process more efficient. Trusting AI blindly is never the solution.
Agreed, Eva. It's important to integrate AI tools intelligently while maintaining human vigilance.
Exactly, Frank. Pharmacovigilance is a complex field, and collaboration between humans and AI can yield the best results.
Henry, I couldn't agree more. Humans bring critical thinking, while AI can handle repetitive tasks, increasing productivity.
Jamie, agreed. AI can provide valuable insights that humans might overlook, but it shouldn't replace the human judgment in critical decision-making.
Natalie, you're right. Combining human judgment and AI capabilities can lead to better-informed decisions, ultimately benefitting patient safety.
Alice, I couldn't agree more. The collaboration between AI and human experts offers a promising future for pharmacovigilance.
Alice, absolutely! Combining the strengths of humans and AI allows us to leverage technology's potential while ensuring the highest level of patient safety.
Grace, well said. Continuous collaboration between experts is essential to overcome the challenges of implementing AI in pharmacovigilance.
AI can assist in detecting patterns and trends that humans might overlook, but the final decision should always rest with humans.
Grace, I completely agree. AI-powered surveillance can streamline the identification of adverse drug reactions, but humans should provide the final assessment.
Quinn, striking that balance between AI assistance and human decision-making is the key to unlocking the full potential of pharmacovigilance.
Oliver, maintaining the human touch in pharmacovigilance is crucial to ensure the best outcomes for patient safety. AI should augment, not replace, human expertise.
I understand the concerns, but let's not forget that chatbots can offer scalability and efficiency, especially in handling large volumes of data.
Isabella, you have a point. With an ever-increasing amount of pharmacovigilance data, chatbots can help alleviate some of the burden.
However, we shouldn't forget the ethical aspects of pharmacovigilance. AI algorithms should be transparent and unbiased to ensure patients' safety.
Laura, ethics must be at the forefront while developing and implementing AI in pharmacovigilance. Patients' trust is paramount.
Uma, a multidisciplinary approach involving clinicians, data scientists, and ethicists is crucial to effectively address AI's integration challenges in pharmacovigilance.
Frank, I agree. An intelligent integration of AI into existing pharmacovigilance processes is necessary to leverage the advantages while mitigating risks.
I appreciate the thoughtful comments, Laura and Uma. Ethical considerations and maintaining patient trust are foundational in adopting AI in pharmacovigilance.
Absolutely, Eric. Responsible development and deployment of AI technologies should be a priority in the pharmaceutical industry.
Michael, responsible AI deployment is crucial to avoid unintended consequences and ethical dilemmas. It's reassuring to see industry professionals emphasizing its importance.
Laura, absolutely. Transparency and accountability must be ensured to build and maintain trust in AI-based pharmacovigilance systems.
Michael, AI and responsible ethics should go hand in hand in pharmacovigilance to protect patients and improve drug safety.
Eric, thank you for addressing our concerns regarding ethics and patient trust. Collaboration between experts in the field is crucial for successful implementation.
Eric, based on your experience, what do you think is the biggest challenge in integrating chatbots into pharmacovigilance processes?
Chris, one of the significant challenges is ensuring chatbots are trained on accurate and up-to-date data to provide reliable insights. Regular updates and monitoring are crucial.
Eric, thank you for highlighting the importance of ethics and trust in this context. Developing robust transparency and interpretability standards is crucial in AI adoption.
Paul, well-said. Establishing clear guidelines and regulations around AI in pharmacovigilance will help address concerns and ensure responsible use.
Paul, I agree. Chatbots can assist healthcare professionals, but human judgment and expertise are invaluable in ensuring patient safety.
Paul, exactly. Chatbots can assist healthcare professionals, but they should not be regarded as a substitute for their expertise and judgment.
Eric, besides accurate data, maintaining patient privacy and data security should also be top priorities in deploying chatbots for pharmacovigilance.
Rose, you're absolutely right. Patient privacy and data protection should be front and center when implementing AI systems in healthcare.
I believe AI has immense potential in enhancing drug safety, but Laura's point about ethics is crucial. We need responsible AI deployment.
The integration of AI in pharmacovigilance should be seen as a collaboration for better decision-making, not a replacement.
Well said, Oliver. AI is here to assist, not replace, the skilled workforce involved in pharmacovigilance.
Oliver, I fully agree. The human touch and experience are irreplaceable when it comes to patient safety and critical decision-making.
Isabella, chatbots can help identify potential risks and adverse events faster, but human experts should conduct in-depth analysis and make informed decisions based on that.
The key is finding the right balance between human expertise and technological advancements in pharmacovigilance.
The ability of chatbots to handle and analyze extensive data quickly is a game-changer in pharmacovigilance. It can enhance efficiency and timely identification of potential risks.
Thank you all for your valuable insights and engaging in this discussion. It highlights the importance of collaboration and cautious adoption of chatbots in pharmacovigilance for successful outcomes.
This discussion has been enlightening. It's clear that the collaboration between AI and human professionals is necessary to improve pharmacovigilance practices.