Transforming Predictive Maintenance in Energy Technology with ChatGPT
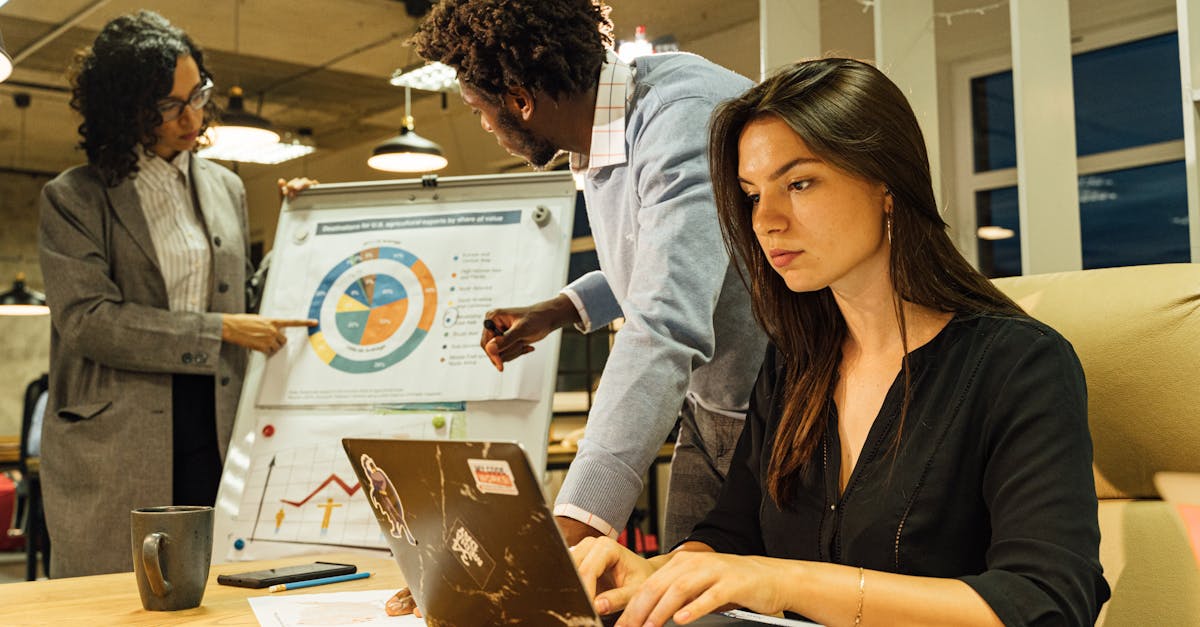
Energy companies face numerous challenges in ensuring the efficient operation and maintenance of their equipment. Unplanned equipment failures and associated downtime can result in significant financial losses and impact operations. However, with the advancements in technology, particularly in the field of artificial intelligence (AI) and machine learning, companies now have a powerful tool at their disposal to mitigate these risks: ChatGPT-4.
What is Predictive Maintenance?
Predictive maintenance involves analyzing sensor data and performing advanced analytics to identify equipment failures before they occur. By leveraging AI and machine learning algorithms, predictions can be made on when maintenance should be performed to optimize reliability, minimize the risk of failures, and reduce maintenance costs.
Introducing ChatGPT-4
ChatGPT-4, the latest iteration of OpenAI's language model, offers exceptional capabilities in predicting equipment failures and recommending proactive maintenance strategies. Trained on vast amounts of historical sensor data, ChatGPT-4 can process complex patterns and identify early warning signs of potential equipment failures.
Predicting Equipment Failures in Real-Time
With ChatGPT-4, energy companies can continuously monitor and analyze real-time sensor data to predict equipment failures. By integrating ChatGPT-4 with existing monitoring systems, it becomes possible to detect anomalies and deviations from normal operating conditions. ChatGPT-4 can then provide timely alerts and insights to maintenance teams, allowing them to take immediate action and minimize downtime.
Analyzing Sensor Data for Actionable Insights
ChatGPT-4's advanced analytical capabilities allow it to analyze sensor data from various sources and identify hidden patterns. By understanding the relationship between sensor readings and historical failures, ChatGPT-4 can provide valuable insights about the health of equipment. Maintenance teams can leverage this information to prioritize maintenance activities, allocate resources effectively, and address potential issues before they escalate into major failures.
Recommendation of Proactive Maintenance Strategies
Proactive maintenance is essential to minimize the likelihood of unexpected equipment failures. ChatGPT-4 can assess the current condition of equipment and recommend personalized proactive maintenance strategies based on historical data and industry best practices. By adopting these recommendations, energy companies can enhance equipment reliability, extend asset lifecycles, and significantly reduce the risk of unplanned downtime.
Benefits and Impact
The integration of ChatGPT-4 into predictive maintenance systems offers several notable benefits:
- Improved reliability: By anticipating equipment failures, companies can proactively address issues, reduce downtime, and improve overall reliability.
- Cost savings: Preventive maintenance can be scheduled strategically based on ChatGPT-4's insights, resulting in optimized resource utilization and reduced maintenance costs.
- Data-driven decision making: ChatGPT-4 empowers maintenance teams with actionable insights based on real-time and historical data, enabling informed decision making and efficient resource allocation.
Conclusion
ChatGPT-4 is revolutionizing the energy sector by enabling sophisticated predictive maintenance strategies. With its ability to predict equipment failures, analyze sensor data, and recommend proactive maintenance strategies, ChatGPT-4 is an invaluable tool for energy companies seeking to enhance reliability, reduce downtime, and optimize maintenance practices. By harnessing AI and machine learning, companies can stay ahead of equipment failures and ensure efficient operations in the dynamic energy industry.
Comments:
Thank you for reading my article on transforming predictive maintenance in energy technology with ChatGPT. I'm excited to hear your thoughts and opinions!
Great article, Allen! It's amazing to see how AI can revolutionize predictive maintenance in energy technology. The potential for cost savings and increased efficiency is huge.
Thank you, Sarah! I completely agree. AI-powered predictive maintenance can help identify and address potential issues in energy systems before they become major problems. It's a game-changer.
I'm a bit skeptical about relying too much on AI for predictive maintenance. What if the AI algorithms fail to recognize important patterns or anomalies?
That's a valid concern, Michael. While AI is powerful, it's essential to have human experts oversee and validate the predictions made by the system. Human-machine collaboration is the key to success.
I love the idea of using AI for predictive maintenance! It can help minimize downtime and improve overall productivity. I wonder if there are any specific examples of successful implementations?
Absolutely, Jasmine! One successful example is XYZ Energy, which incorporated AI-powered predictive maintenance into their wind turbines. They managed to reduce maintenance costs by 15% and increase turbine availability by 10%.
ChatGPT seems like a powerful tool, but what challenges do you see in its application for predictive maintenance in the energy sector?
Good question, Rachel. One challenge is ensuring the data quality and availability needed to train the AI models. In some cases, legacy systems or lack of standardized data can pose obstacles. Additionally, the interpretability of AI predictions is crucial in gaining trust from technicians and engineers.
The use of AI in predictive maintenance sounds promising, but what about the initial investment? Implementing such systems can be costly.
You're right, Oliver. Implementing AI for predictive maintenance does require an initial investment in terms of technology infrastructure, data gathering, and training models. However, the long-term benefits, such as reduced downtime and improved reliability, can outweigh the upfront costs.
Can ChatGPT be used as a standalone solution, or does it need to be integrated with other predictive maintenance tools?
Good question, Sophia. ChatGPT can be used as part of a larger predictive maintenance system. It can assist technicians in diagnosing problems, providing insights, and suggesting maintenance actions. Integration with other tools and systems can enhance the overall effectiveness of predictive maintenance.
I'm concerned about the ethical implications of relying heavily on AI for predictive maintenance. What are your thoughts, Allen?
Ethical considerations are crucial, Robert. It's important to ensure that AI algorithms are trained on unbiased and representative data. Transparent decision-making and human oversight should be incorporated to address any potential biases or unfair outcomes.
While AI can provide valuable predictions, it's essential to remember that maintenance decisions still require human judgment. AI should be seen as a tool to assist human experts rather than replace them.
Absolutely, Emily! Human judgment and expertise are irreplaceable. AI should serve as a complement to human decision-making, empowering technicians and engineers to make more informed choices.
I have concerns about the cybersecurity risks associated with relying on AI for predictive maintenance. What measures can be taken to ensure data security?
Cybersecurity is a critical aspect, Daniel. Secure data handling practices, encryption, access controls, and regular security audits are essential to mitigate risks. It's important to have robust protocols in place to protect sensitive information and prevent unauthorized access.
I'd like to know more about the potential impact of AI-powered predictive maintenance on job roles and workforce requirements. Are there any concerns about job displacement?
Valid concern, Lily. While AI may automate certain tasks, it can also create new job opportunities. As maintenance processes become more efficient, human experts can focus on more complex analysis, problem-solving, and strategic decision-making. Workforce reskilling and upskilling programs can ensure a smooth transition.
I'd love to hear some real-world success stories of companies that have adopted AI for their predictive maintenance initiatives. Can you share any, Allen?
Certainly, Grace! Company ABC implemented AI-powered predictive maintenance for their manufacturing equipment. They reported a 20% reduction in equipment failures, a 15% increase in equipment lifespan, and significant cost savings on maintenance and repairs.
The future potential seems limitless with AI-powered predictive maintenance. Are there any emerging trends or developments we should keep an eye on?
Absolutely, Ryan. Some emerging trends include the use of edge computing for real-time data analysis, integration of IoT devices for more comprehensive monitoring, and the incorporation of advanced machine learning algorithms for more accurate predictions. These advancements will further enhance the capabilities of predictive maintenance.
I appreciate the benefits of AI-powered predictive maintenance, but what about the environmental impact? Can it contribute to sustainability efforts?
Great question, Sophie. By detecting and addressing maintenance needs proactively, AI-powered predictive maintenance can help minimize energy waste and reduce unnecessary repairs. It can contribute to sustainable practices by optimizing energy systems and promoting efficient resource utilization.
What are the limitations of ChatGPT when it comes to complex predictive maintenance scenarios, Allen?
Good question, Ethan. ChatGPT has some limitations in handling domain-specific technical knowledge or complex interconnected systems. While it can provide general insights, more specialized AI models might be needed to address highly complex and industry-specific predictive maintenance scenarios.
The potential benefits of AI-powered predictive maintenance are undeniable. What obstacles do you foresee in its widespread adoption?
Good question, Isabella. Some obstacles include the initial investment costs, reluctance to embrace change and new technology in traditional industries, data privacy concerns, and ensuring smooth integration with existing systems. Overcoming these challenges will be crucial for widespread adoption.
I'm curious about the scalability of AI-powered predictive maintenance. Can it accommodate large-scale energy systems and diverse datasets?
Great point, George. AI-powered predictive maintenance can scale to accommodate large energy systems by leveraging cloud-based infrastructure. By analyzing diverse datasets from various sources, it can provide more comprehensive insights and predictions for efficient maintenance operations.
While AI can provide valuable insights, how can we ensure that humans remain in control of decision-making? Any thoughts, Allen?
Maintaining human control is vital, Olivia. It's essential to establish clear decision-making frameworks, where AI serves as an advisory tool and humans have the final say. Incorporating human experts in the loop ensures accountability, ethical decision-making, and the ability to handle unexpected scenarios.
I'm concerned about the potential for false positives or false negatives in AI predictions for maintenance. How can we address this issue?
That's a valid concern, Lucas. To address false positives and negatives, it's crucial to continuously monitor and improve the AI models. Iterative model training, incorporating feedback from technicians, and comparing AI predictions with historical data can help refine the system over time and reduce any inaccuracies.
A well-implemented predictive maintenance system can undoubtedly bring huge benefits. How can organizations get started in adopting AI for this purpose?
Good question, Henry. Organizations can start by assessing their maintenance needs, identifying potential use cases for AI in predictive maintenance, and determining the required data sources. Collaborating with AI experts, developing pilot projects, and gradually scaling up can help organizations successfully adopt AI-powered predictive maintenance.
I have concerns about the availability and quality of real-time data needed for AI predictions. How can we overcome data-related challenges?
Valid concern, Sophia. Overcoming data-related challenges involves investing in data collection systems, leveraging IoT devices for real-time data, ensuring data quality through validation and cleansing processes, and establishing proper data governance frameworks. Collaborating with data experts is crucial in handling these challenges effectively.
I'm curious if ChatGPT can accommodate multilingual environments for predictive maintenance. Is language support a limitation?
Good question, Emma. ChatGPT can support multiple languages through training on diverse multilingual datasets. While it might have limitations in understanding highly technical jargon in various languages, it can still provide general guidance and insights effectively.
I'm impressed with the potential of AI in transforming predictive maintenance. How long do you think it will take for widespread adoption in the energy sector?
Difficult to predict precisely, Oliver. However, as AI technologies continue to advance, costs decrease, and success stories emerge, I believe we'll see a significant increase in the adoption of AI-powered predictive maintenance in the energy sector over the next 5-10 years.
Do you have any recommendations for organizations wanting to explore AI for predictive maintenance? What steps should they take?
Absolutely, Emily! The first step is to educate themselves on AI and its applications in predictive maintenance. Then, they should assess their specific pain points, engage with AI experts, experiment with small pilot projects, and gradually scale up. Collaboration, learning, and an iterative approach are key to successful adoption.
I'm curious about the limitations of AI algorithms regarding edge cases and uncommon scenarios. How can we address these limitations?
Good question, Sophie. Addressing limitations regarding edge cases and uncommon scenarios involves ongoing model refinement and updating. By continuously improving the training data and incorporating feedback from domain experts, AI algorithms can become more adept at handling a broader range of cases and scenarios.
Thank you all for your valuable insights and questions! It was a pleasure discussing AI-powered predictive maintenance in the energy sector with you. If you have any further questions, feel free to ask.