Transforming Research Publication: Leveraging ChatGPT for Advanced Powder X-ray Diffraction Studies
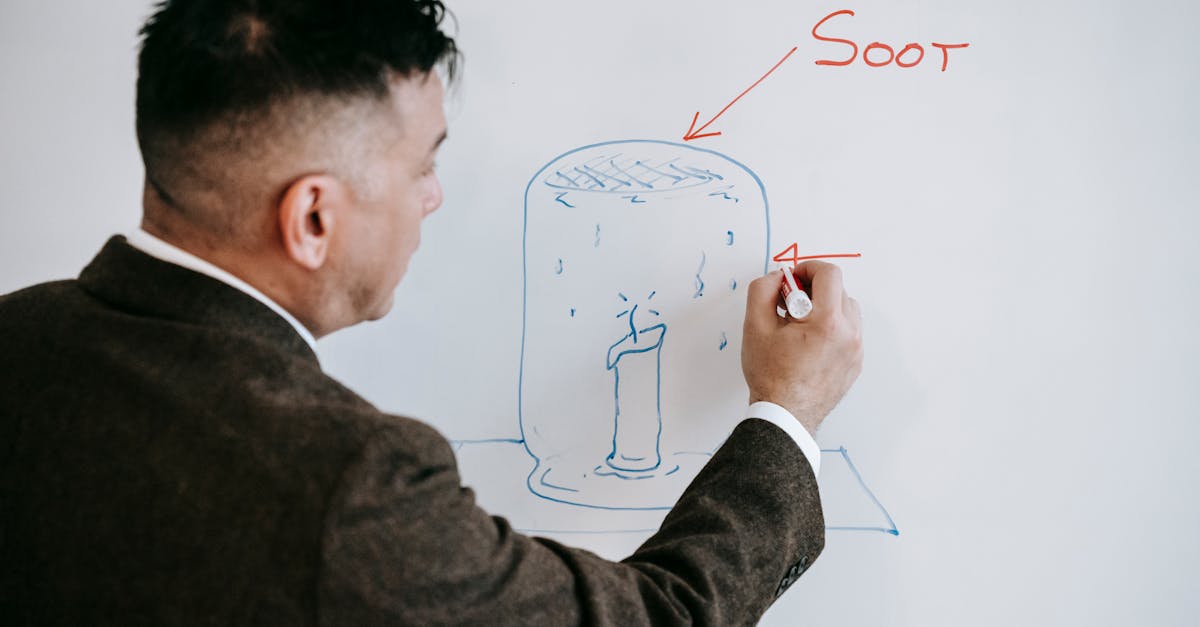
Powder X-ray Diffraction (PXRD) is a powerful analytical technique used in research publications for studying the crystal structure of materials. It provides valuable insights into the atomic arrangement and composition of various substances.
PXRD technology utilizes the phenomenon of X-ray diffraction, where X-rays are directed onto a powdered sample. The X-rays interact with the atoms in the sample, causing them to diffract in a distinctive pattern. By carefully analyzing this diffraction pattern, researchers can determine the crystal structure and properties of the material under investigation.
Research publications extensively utilize PXRD to investigate a wide range of materials, including minerals, pharmaceuticals, polymers, and metals. The technique is particularly useful in fields such as chemistry, materials science, geology, and pharmaceutical research.
One of the significant benefits of employing PXRD in research publications is its ability to provide accurate and detailed information on the crystal structure of a material. This information is crucial for understanding a material's properties, behavior, and potential applications. PXRD data helps researchers validate their hypotheses, establish relationships between structure and properties, and make informed decisions about the development and optimization of various materials.
With the advancements in natural language processing and AI technology, tools like ChatGPT-4 can aid researchers in drafting and proofreading their research articles related to PXRD technologies. ChatGPT-4 is an advanced AI assistant that can understand and generate human-like text.
This AI assistant can assist researchers in various ways:
- Drafting Articles: ChatGPT-4 can help researchers in structuring their research articles related to PXRD technologies. It can provide information about the methodology, data interpretation, and significance of findings.
- Proofreading: ChatGPT-4 can proofread research articles to identify and correct grammatical errors, improve the flow of the text, and suggest better word choices. This can enhance the overall clarity and readability of the publication.
- Language Enhancement: ChatGPT-4 can help researchers improve the language and style of their articles. It can suggest alternative phrases, rephrase sentences, and offer language enhancements to make the content more engaging and impactful.
By utilizing AI assistants like ChatGPT-4, researchers can save valuable time and effort in the writing and editing process. These tools can assist in creating high-quality research articles related to PXRD technologies, contributing to the advancement of scientific knowledge and facilitating effective communication within the research community.
In conclusion, Powder X-ray Diffraction is a fundamental technology extensively used in research publications to study the crystal structure of materials. With the help of AI assistants like ChatGPT-4, researchers can effectively draft and proofread their research articles related to PXRD technologies, leading to enhanced publications and scientific progress.
Comments:
Thank you all for taking the time to read my article on Transforming Research Publication using ChatGPT for advanced Powder X-ray Diffraction Studies. I'm excited to discuss this topic further. Please feel free to share your thoughts and comments!
Great article, Stephen! The potential of leveraging ChatGPT for advanced powder X-ray diffraction studies is fascinating. It's impressive how artificial intelligence can enhance research in various fields. Have you experimented with any other AI models for this type of study?
Thanks for your feedback, David! I have experimented with other AI models, but ChatGPT showcased promising results due to its ability to generate detailed interpretations in a conversational format.
Stephen, thank you for your response. It's interesting to hear that ChatGPT outperformed other AI models in this context. Did you utilize any specific pre-training techniques tailored to powder X-ray diffraction data?
Stephen, could you provide some insights into the specific techniques or methodologies you employed for training ChatGPT to handle powder X-ray diffraction data? Were there any significant adaptations needed?
David, ensuring the reliability of ChatGPT involves careful monitoring, bias mitigation, and cross-validation of results using established scientific methods. Regular iterations and refining of the model contribute to minimizing limitations and enhancing output quality.
David, the training process involved adapting the model to deal with the nuances of powder X-ray diffraction data by fine-tuning the architecture and applying transfer learning techniques. The initial adaptations were followed by fine-tuning iterations to improve output quality.
Stephen, regular iteration and refining of the model to mitigate limitations and enhance output quality make sense. Were there any specific techniques or approaches you found particularly effective in minimizing biases in ChatGPT-generated interpretations?
David, minimizing biases involved a combination of techniques such as training on diverse datasets, evaluating against multiple references, and being mindful of potential data biases during fine-tuning iterations. Iterative feedback from experts also played a significant role in identifying and addressing biases.
David, techniques like adversarial training, careful dataset curation, and employing interpretable AI techniques were effective in mitigating biases in ChatGPT-generated interpretations. Continuous monitoring and evaluation helped ensure unbiased and reliable results.
Stephen, thanks for the insights into minimizing biases in ChatGPT-generated interpretations. Adversarial training and careful dataset curation sound essential. How did you evaluate the effectiveness of bias mitigation techniques at different stages of model development?
David, to evaluate the effectiveness of bias mitigation techniques, we employed manual evaluation by experts focusing on identifying potential biases in the model's output. Additionally, we conducted continuous monitoring of results and analyzed user feedback to ensure biases were minimized across different stages of model development.
Stephen, it's great to hear that manual evaluation by experts and continuous monitoring were integral in mitigating biases. How did you ensure that the biases identified by experts were effectively addressed in the model's subsequent iterations?
David, when biases were identified by experts, we analyzed the specific biases in the model's output and used that information to refine the training process, including modifying the loss function, adjusting data augmentation techniques, and incorporating additional controls to minimize biases in subsequent iterations.
Stephen, combining manual evaluation with continuous monitoring and user feedback to address biases is commendable. How did you ensure that the biases identified by experts were effectively communicated to the AI model development team?
David, we established clear channels of communication between the domain experts and the AI model development team. Regular meetings and documentation of feedback ensured that the identified biases were effectively communicated and incorporated into the training process. This collaboration helped us refine the model's output and minimize biases over time.
Stephen, thanks for sharing your insights. The collaboration and communication between domain experts and the AI model development team were crucial in addressing biases. Overall, your research is groundbreaking and has the potential to revolutionize powder X-ray diffraction studies!
Stephen, your article shed light on how ChatGPT can transform research publication. The idea of using AI to aid in interpreting complex data from powder X-ray diffraction studies is remarkable. I'm curious about the challenges you encountered during this research. Could you share some insights?
Emily, excellent question! One of the main challenges was training the model to accurately handle the complexities of powder X-ray diffraction data and generate meaningful interpretations. Calibration and verification were critical to ensure reliable results.
Stephen, thanks for sharing your insights! Calibration and verification are indeed crucial for reliable results. Did you face any challenges during the calibration process? How did you ensure that the model's interpretations align with established scientific knowledge?
Stephen, I completely agree. Collaboration between AI and domain experts is crucial to ensure AI-generated interpretations do not compromise scientific rigor. How do you envision this collaboration happening in practice?
Stephen, I'm intrigued by the challenges faced during training. Powder X-ray diffraction data can be complex. How did you optimize the training process to handle and interpret the intricacies of this type of data?
Stephen, I'm interested in understanding how you ensured that the model's interpretations align with established scientific knowledge. Were there any specific validation steps or reference datasets used during the process?
Emily, collaboration with domain experts is vital. In practice, it involves continuous feedback from experts regarding the accuracy of interpretations, incorporating suggestions, and ensuring that the model captures the expected scientific knowledge while delivering efficient results.
Emily, optimizing the training process involved a combination of techniques like fine-tuning the model architecture, using appropriate loss functions, and incorporating domain-specific data augmentation methods. This allowed the model to effectively handle the complexities of powder X-ray diffraction data.
Emily, the validation steps included comparing ChatGPT-generated interpretations with established scientific literature, verifying against reference datasets, and soliciting feedback from domain experts. Continuous refinement ensured alignment with scientific knowledge while accounting for specific nuances of the data.
Stephen, thanks for your response. The iterative process of incorporating expert feedback seems crucial. It ensures that the model captures the domain-specific knowledge and adapts effectively over time. Did you face any challenges in coordinating with experts during this collaborative process?
Stephen, the combination of techniques used for optimizing training seems comprehensive. How did you address the challenge of generalization to ensure that the model's interpretations could be applied to a wide range of powder X-ray diffraction studies?
Emily, in coordinating with experts, challenges arose in aligning schedules and ensuring effective communication channels. Regular meetings, clear guidelines, and pre-defined evaluation metrics were key to overcoming these challenges and maintaining productive collaboration.
Emily, tackling the challenge of generalization involved collecting diverse and representative powder X-ray diffraction data from different sources. The model was trained on this varied dataset to develop a robust understanding of the nuances present in a wide range of studies.
Stephen, aligning schedules and optimizing communication channels are indeed critical aspects of fruitful collaboration. Were there any specific strategies or tools you employed to facilitate the collaboration process with domain experts?
Emily, we used a combination of communication tools to facilitate collaboration, including video conferences, chat platforms, and shared document repositories. Additionally, setting clear expectations, sharing regular progress updates, and maintaining an open line of communication were key to ensuring a smooth collaborative process.
Stephen, the use of various communication tools and establishing clear expectations seems highly effective. How did you manage the integration of expert feedback into the model's training process in order to improve the quality of ChatGPT interpretations?
Emily, the integration of expert feedback was done through an iterative process. We collected feedback on model output from domain experts and identified areas of improvement. This feedback was then incorporated into the training process through fine-tuning iterations, resulting in enhanced quality and alignment with domain-specific knowledge.
Stephen, the iterative process of incorporating feedback into the training process seems effective. How frequently did you engage with domain experts throughout the iterative model development? Did their engagement change over time?
Emily, we engaged with domain experts regularly throughout the iterative model development process. Initially, the engagement was more frequent to establish a strong baseline understanding. As the model evolved and improved, the frequency of engagement shifted to more focused discussions and refining specific aspects of the model.
Impressive work, Stephen! Your article raises an important question - how can the use of ChatGPT for advanced powder X-ray diffraction studies impact the reproducibility of experiments? Are there any concerns regarding AI-generated interpretations of experimental data?
Hi Alexandra! Valid concern. AI-generated interpretations should be treated as guidance and reviewed by domain experts. It is crucial to maintain a balance between leveraging AI capabilities and validating the accuracy of results through established scientific methods.
Stephen, I appreciate your response. Validating AI-generated interpretations through scientific methods sounds essential. Could you briefly explain what steps you took to validate the accuracy and reliability of the ChatGPT-generated results?
It's impressive that ChatGPT provided detailed interpretations in a conversational format. Did you face any limitations or potential biases in the model's output? How did you mitigate these challenges to enhance reliability?