Unleashing the Power of ChatGPT: Advancing Time Series Analysis in Statistics Technology
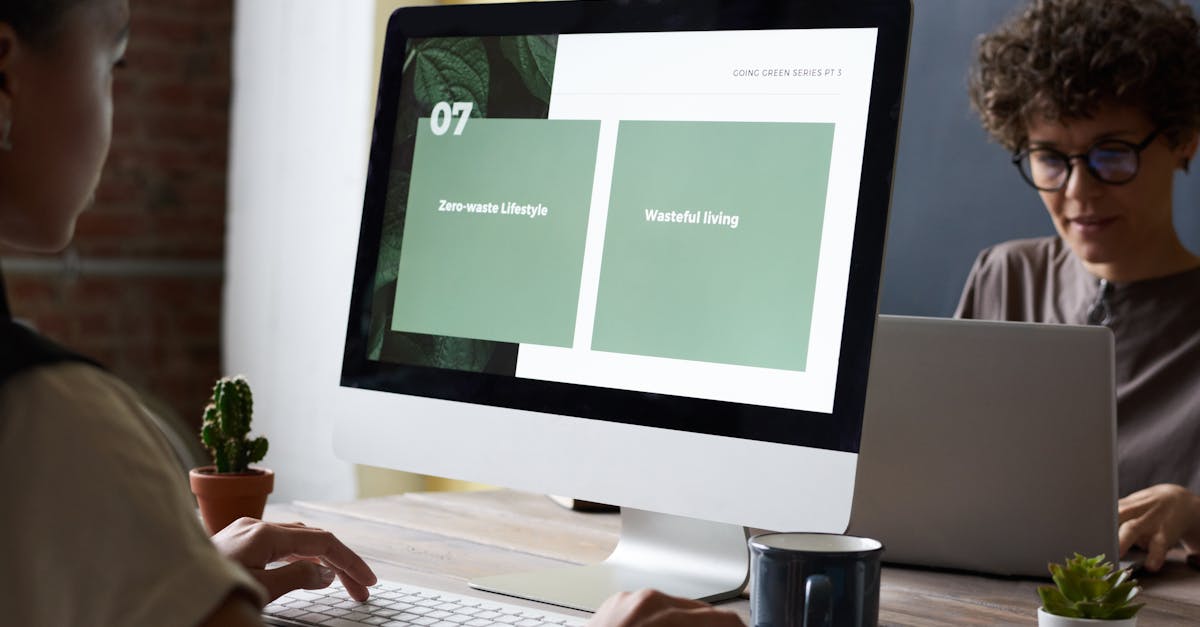
Time series analysis is a statistical technique used to analyze and interpret data points collected over time intervals. This analytical approach plays a crucial role in various fields, from finance and economics to climate and sales forecasting. With advanced technology like ChatGPT-4, the power of time series analysis can be harnessed more efficiently, opening new avenues for extracting insights and making informed decisions.
Autoregressive Integrated Moving Average (ARIMA)
One of the fundamental techniques in time series analysis is ARIMA modeling. ARIMA stands for Autoregressive Integrated Moving Average. It combines three components - autoregressive (AR), differencing (I), and moving average (MA) to model and forecast time series data.
The autoregressive component captures the relationship between an observation and a certain number of lagged observations. The differencing component helps in making the data stationary, which is a crucial assumption for many time series models. The moving average component considers the dependency between an observation and a residual error from a moving average model applied to lagged observations.
Forecasting
Time series forecasting is a valuable application of time series analysis. It involves predicting future data points based on historical patterns and trends in the data. Forecasting techniques, such as ARIMA, can be used to provide insights into future developments and help in making proactive decisions.
Seasonality and Trend Analysis
Seasonality refers to patterns that repeat at regular intervals within a time series. Identifying and understanding seasonality can be crucial for businesses to align their strategies, especially in industries affected by seasonal fluctuations, such as retail and tourism. Time series analysis can help uncover seasonality patterns and incorporate them into forecasting models.
Trend analysis involves studying the general direction and magnitude of a time series over an extended period. Understanding trends allows businesses to identify long-term patterns and extrapolate future developments, aiding in strategic planning and decision-making.
ChatGPT-4: An Advanced Time Series Analysis Tool
ChatGPT-4 is an advanced language model that can assist in various aspects of time series analysis. Leveraging its natural language processing capabilities, ChatGPT-4 can understand and respond to queries related to time series analysis techniques, helping users gain valuable insights.
With ChatGPT-4, users can inquire about ARIMA modeling parameters, such as autoregressive order (p), differencing order (d), and moving average order (q). The model can provide guidance on selecting appropriate orders based on the characteristics of the time series data at hand.
Furthermore, ChatGPT-4 can assist in forecasting by answering queries related to future data predictions. Users can query the model about the expected values of time series data at specific time points or inquire about the likelihood of certain trends or patterns in the future.
Additionally, ChatGPT-4 can help users identify and analyze seasonality within time series data. By considering historical patterns, the model can suggest appropriate approaches to incorporate seasonality components into forecasting models or detect irregularities that deviate from regular seasonal patterns.
When it comes to trend analysis, ChatGPT-4 can provide users with insights into long-term patterns and trends exhibited by time series data. Whether it's identifying upward or downward trends or estimating the magnitude of future changes, ChatGPT-4 can assist in making data-driven decisions.
Conclusion
Time series analysis, with its techniques like ARIMA, forecasting, seasonality, and trend analysis, has become more accessible and powerful with advancements in technology like ChatGPT-4. Leveraging the natural language processing capabilities of ChatGPT-4, users can obtain valuable insights into time series data and make informed decisions based on accurate predictions and analysis.
Comments:
This article on ChatGPT's application in time series analysis is fascinating! It's amazing to see how AI is advancing in the field of statistics technology.
I agree, Gregory! The potential of ChatGPT in time series analysis is promising. It can greatly assist in analyzing complex data patterns and making accurate predictions.
Emily, the combination of ChatGPT's natural language processing abilities and time series analysis can be a game-changer. It opens up avenues for intelligent interactive data exploration.
Michael, absolutely! The ability to interact with ChatGPT using natural language makes data exploration more intuitive, especially for non-technical users.
Good point, Daniel! ChatGPT can bridge the gap between data experts and domain experts, enabling a more collaborative and user-friendly approach to time series analysis.
Daniel, absolutely! The intuitive nature of ChatGPT can democratize time series analysis and make it more accessible to a wider range of users.
Sarah, democratizing time series analysis would be a significant step forward. It can empower various stakeholders, including business users, researchers, and policymakers.
Emily, with the application of ChatGPT in time series analysis, it can also facilitate forecasting and predictive modeling, enabling better decision-making in various industries.
Absolutely, Michael. AI models like ChatGPT have immense potential to improve decision-making by providing accurate predictions and insights for driving business strategies.
Adam, mental health analysis can indeed benefit from ChatGPT's capabilities. However, careful consideration of potential biases and the ethical aspects of using AI in this sensitive domain is paramount.
David, you bring up an important point. While AI can offer great value in mental health analysis, ethical guidelines and rigorous validation processes are essential to ensure responsible and safe deployment.
Virginia Barnett, kudos on this article! The way ChatGPT can uncover insights and trends from time series data is remarkable. I wonder what other domains it could be applied to?
Thank you, Robert! Indeed, ChatGPT can have applications beyond statistics technology. It has the potential to revolutionize fields like finance, economics, weather forecasting, and more.
Virginia, do you think ChatGPT can handle large-scale time series datasets? As data volume increases, scalability becomes a crucial factor.
Emma, scalability is indeed essential. With the right infrastructure and efficient algorithms, ChatGPT can handle large-scale time series datasets. The computational resources should be considered.
Emma, ChatGPT can handle large-scale time series datasets with adequate resources and optimized algorithms. Distributed computing and algorithmic optimizations can alleviate scalability concerns.
Robert, I'm excited to see how ChatGPT can be used in healthcare analytics. It could potentially help in analyzing patient data, predicting disease trends, and improving medical treatments.
Sophie, you raise an interesting point! Healthcare analytics can greatly benefit from AI-powered models like ChatGPT. It may enhance diagnostics, drug discovery, and personalized medicine.
Robert, I'm excited about ChatGPT's prospects beyond statistics technology as well. Imagine the possibilities in marketing analytics, customer behavior prediction, and resource optimization!
I'm really curious about the accuracy and reliability of ChatGPT in time series analysis. How well does it perform compared to traditional statistical methods?
Eric, from what I've read, ChatGPT has shown promising results in time series analysis. While it may not replace traditional methods entirely, it can offer valuable insights and assist in complex analysis tasks.
Eric, I believe ChatGPT can be a powerful tool in time series analysis, particularly for exploring the data and identifying patterns. However, we should consider the interpretability and potential biases introduced by AI models.
Eric, there might be cases where traditional statistical methods outperform ChatGPT, especially in situations where the underlying processes are well understood and modeled specifically.
Hannah is right. Careful consideration is needed when deciding between ChatGPT and traditional methods. The domain, data quality, and interpretability requirements play a significant role.
Interpretability is indeed a concern, Eric. ChatGPT and other AI models often lack transparency, making it challenging to understand the underlying decision-making process.
David, explainable AI methods are being actively researched to address this concern. It's essential to strike a balance between accuracy and interpretability in time series analysis.
Charlotte, I'm glad to know that research on explainable AI methods is ongoing. Transparency and interpretability are key to building trust in AI models across various industries.
Eric, while ChatGPT shows promise, it's worth exploring a hybrid approach using both ChatGPT and traditional statistical techniques to cross-validate the results and mitigate any limitations.
Linda, I agree. Combining the power of AI models like ChatGPT with established statistical methods can offer a more robust approach to time series analysis.
I wonder about the limitations of ChatGPT in time series analysis. Are there any specific challenges or types of data where it might struggle?
Evelyn, while ChatGPT is impressive, it might struggle with irregular or sparse time series data. It's best suited for well-structured datasets and time series with consistent patterns.
Sophie, I agree. ChatGPT might face challenges with incomplete or noisy time series. Preprocessing and handling outliers would be crucial to ensure accurate analysis and predictions.
Sophie and Oliver, I can see great potential for AI in mental health analysis using ChatGPT. It could assist in deciphering patterns and predicting potential mental health issues.
Handling outliers is crucial, Sophie. Outliers can significantly impact the analysis and predictions. Proper data preprocessing steps are necessary to ensure accurate results.
Mental health analysis is a promising area, Oliver and Sophie. Using ChatGPT can potentially detect patterns that humans may overlook, leading to improved early intervention.
Sophie, preprocessing techniques such as smoothing, interpolation, or outlier rejection can help deal with data irregularities before applying ChatGPT to time series analysis.
Correct, Sophie. Proper preprocessing steps and outlier treatment can significantly improve the quality of input data, leading to more reliable analysis and predictions using ChatGPT.
Although traditional methods excel in some cases, ChatGPT's potential to automate repetitive tasks and speed up analysis makes it an exciting addition to the time series analyst's toolkit.
Absolutely, Hannah. The synergy between ChatGPT and traditional methods can offer the best of both worlds, harnessing AI's power while leveraging the strengths of well-established techniques.
Daniel, democratizing time series analysis can drive innovation and discovery. The broader involvement of stakeholders can bring fresh perspectives and accelerate advancements in various domains.
Absolutely, Sarah! The broader adoption and democratization of AI-powered tools like ChatGPT have the ability to drive innovation across industries globally, benefiting society as a whole.
Emily, democratization helps break down barriers and encourages cross-pollination of ideas. It's an exciting time for AI in time series analysis and its positive impact.
Absolutely, Emily. A balanced approach that combines AI models like ChatGPT with human judgment can help mitigate the risks associated with biases and misinterpretations.
It's important, though, to ensure ethical considerations are in place when using AI in mental health analysis. Privacy and data security should be top priorities.
ChatGPT integration with predictive modeling tools would be a game-changer, allowing businesses to harness the power of AI for more accurate and timely forecasts.
Indeed, the natural language interface of ChatGPT promotes a more user-friendly and interactive data analysis process. It enhances communication between analysts and the system.
On the flip side, we need to be cautious about the risks of bias and unintentional misinterpretation when relying heavily on AI models like ChatGPT for crucial decision-making.
Thank you all for your valuable insights and engaging in this discussion. It's fantastic to see such enthusiasm and thoughtful perspectives on ChatGPT's potential in time series analysis!
While ChatGPT enhances user interaction and data exploration, the human analyst's expertise remains invaluable in discerning meaningful insights and contextualizing the results.
To leverage ChatGPT's potential fully, it's crucial to combine AI's capabilities with the domain knowledge and intuition of human experts.