Unleashing the Power of ChatGPT: An Innovative Problem Solver for Data Analysis
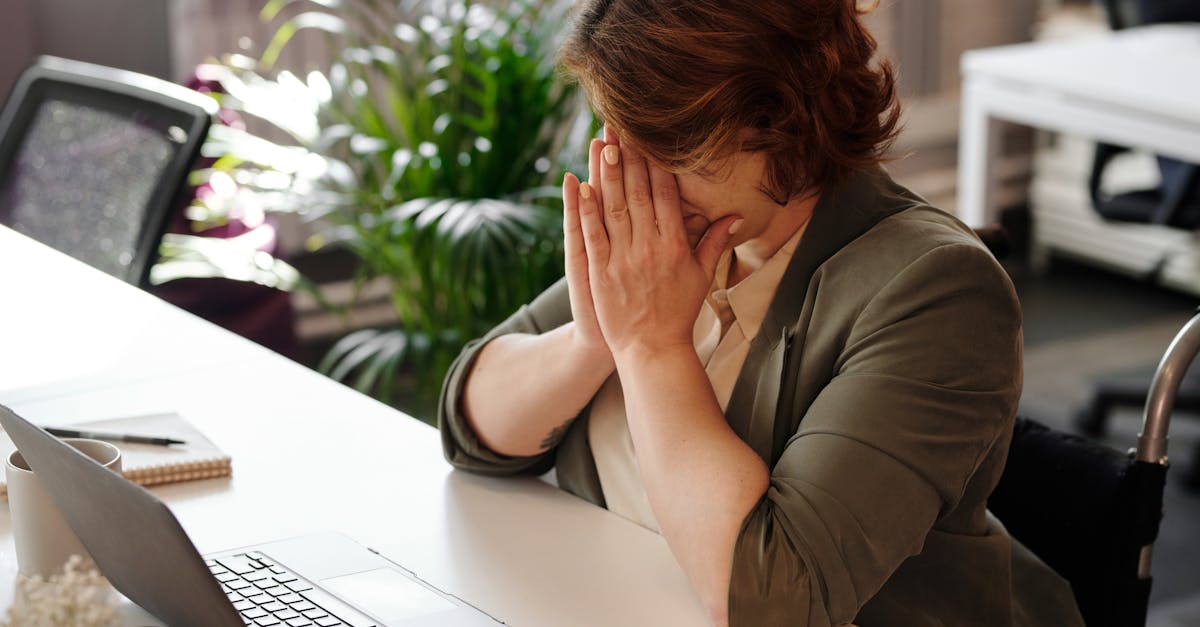
Welcome to the world of innovative problem solving in the field of data analysis. Today, we will explore how ChatGPT-4 can revolutionize the way data is analyzed, insights are generated, and reports are generated.
Introducing ChatGPT-4
ChatGPT-4 is an advanced natural language processing (NLP) model developed by OpenAI. It is built on the success of its predecessors and utilizes state-of-the-art techniques to understand and generate human-like responses. This technology has gained significant traction in various domains, including data analysis.
The Power of Data Analysis
Data analysis plays a crucial role in decision-making processes across industries. It involves inspecting, cleaning, transforming, and modeling data to discover useful information, draw conclusions, and support decision-making. Proper data analysis can provide valuable insights, help identify patterns and trends, and uncover hidden relationships within large datasets.
ChatGPT-4 in Data Analysis
With its advanced capabilities, ChatGPT-4 can aid in the data analysis process in several ways. Firstly, it can assist in data cleansing by identifying and correcting errors or inconsistencies in the dataset. This ensures that the data is accurate and reliable for further analysis.
Moreover, ChatGPT-4 can generate descriptive statistics and perform complex statistical analysis. It can identify data outliers, calculate measures of central tendency and dispersion, and conduct hypothesis testing. This allows data analysts to quickly obtain valuable information without the need for manual calculations or writing complex scripts.
Additionally, ChatGPT-4 can help in visualizing data through the generation of charts, graphs, and plots. By understanding user queries, it can generate appropriate visual representations that aid in understanding patterns and trends within the data.
Insights and Report Generation
One of the key strengths of ChatGPT-4 is its ability to generate meaningful insights from data and compile them into comprehensive reports. By analyzing patterns, correlations, and trends, it can extract valuable information that may not be immediately apparent. These insights can then be summarized and presented in a well-structured report format.
The reports generated by ChatGPT-4 are highly customizable, allowing users to specify the desired level of detail and the format of presentation. Whether it is a concise executive summary or a detailed technical report, ChatGPT-4 can meet the specific requirements of data analysts, decision-makers, and stakeholders.
Conclusion
The integration of ChatGPT-4 in the field of data analysis opens up new possibilities for innovative problem solving. Using its advanced natural language processing capabilities, it can assist in data cleansing, statistical analysis, data visualization, and generate insightful reports. With ChatGPT-4 as a valuable tool in the toolbox of data analysts, the process of data analysis becomes more efficient, accurate, and accessible.
Comments:
Thank you for taking the time to read my article on 'Unleashing the Power of ChatGPT: An Innovative Problem Solver for Data Analysis'. I'm excited to discuss any questions or thoughts you may have!
Great article, Germain! ChatGPT seems like a powerful tool for data analysis. Do you have any suggestions for best practices when using it?
Thank you, Sarah! When using ChatGPT for data analysis, it's important to provide clear instructions and context to get accurate results. It can be helpful to start with a few example interactions related to your data analysis task to guide the system.
ChatGPT seems like a great tool, but I'm concerned about biases in the generated responses. How does OpenAI address this issue?
Valid concern, Mark. OpenAI uses a two-step process to help reduce biases. Firstly, they train ChatGPT on a mixture of licensed data, data created by human trainers, and publicly available data. Secondly, they provide guidelines that explicitly instruct trainers not to favor any political group. They aim to improve the system's clarity on this topic as well.
This article is really informative, Germain. I'm curious, how does ChatGPT handle complex and technical questions during data analysis?
Thank you, Alice! ChatGPT can handle complex and technical questions to some extent, but it performs best on questions related to its training data. If the system isn't confident in an answer, it may ask clarifying questions or provide a general response. It's always a good practice to review and verify the generated outputs.
I'm interested in the scalability of using ChatGPT for large datasets. Can it efficiently handle analysis tasks involving millions of records?
Good question, Robert. ChatGPT is designed to handle various tasks, but its performance may degrade with larger datasets. For scalability, it's recommended to break down the analysis into smaller, manageable parts. You can interact with the model multiple times, each time focusing on a subset of the data.
Germain, what are the limitations of using ChatGPT for data analysis, and what alternative approaches do you suggest?
Great question, Emily! ChatGPT has a few limitations. It may generate plausible-sounding but incorrect answers, it doesn't always ask for clarifications when it should, and its responses may vary. For alternative approaches, you can consider rule-based systems, specialized data analysis software, or custom code tailored to your specific needs.
How does ChatGPT handle missing or incomplete data? Can it help in imputing missing values during analysis?
Good question, Daniel. ChatGPT doesn't have built-in imputation capabilities. It can provide suggestions or help explore potential approaches to handle missing or incomplete data. However, it's always advisable to consult domain experts and use established imputation techniques when dealing with missing values.
I'm interested in understanding how ChatGPT handles privacy and security when dealing with sensitive datasets. Can you shed some light on this, Germain?
Absolutely, Grace! OpenAI takes privacy and security seriously. ChatGPT conversations are logged and may be used to improve the system, but they are subject to privacy policies. When handling sensitive data, it's crucial to ensure compliance with relevant regulations, perform a privacy impact assessment, and take any necessary precautions to protect the data.
I'm impressed with the capabilities of ChatGPT for data analysis. Are there any specific domains where it has shown outstanding performance?
Thank you, Natalie! ChatGPT has shown promise in a variety of domains, including natural language understanding, summarization, and translation. However, its performance can vary depending on how well it was trained for a specific domain. It's always a good idea to evaluate and fine-tune the model for your particular use case, if possible.
Germain, thanks for sharing this informative article. Can ChatGPT be used for real-time data analysis, or is it more suitable for offline tasks?
You're welcome, Oliver! ChatGPT can be used for real-time data analysis, but it's important to remember the limitations of response times and model capacity. For mission-critical or time-sensitive tasks, it might be more suitable to use traditional or specialized real-time analytics solutions.
I'm curious, Germain, how does ChatGPT handle different types of data sources? Can it handle structured, unstructured, or semi-structured data equally well?
Great question, Liam! ChatGPT can handle different types of data sources, including structured, unstructured, or semi-structured data. However, it's important to provide clear instructions and context specific to the data source to guide the model effectively.
As someone new to data analysis, I find ChatGPT fascinating. Are there any resources or tutorials you would recommend for beginners?
Certainly, Ella! OpenAI provides resources like documentation and tutorials to get started with ChatGPT. You can also explore online learning platforms and communities that offer tutorials and courses on data analysis. Practice with smaller tasks and gradually work your way up to more complex analysis.
What is the expected development roadmap for ChatGPT in the future? Are there any upcoming features or improvements we can look forward to?
Good question, William! OpenAI is actively working on refining and expanding ChatGPT based on user feedback. They are planning to introduce improvements to the model's capabilities, safety mitigations, and API availability. You can expect more updates and features as they continue to iterate and enhance the system.
How does ChatGPT handle numerical analysis tasks? Can it perform regression, forecasting, or other statistical analyses effectively?
Good question, Sophia! ChatGPT is not specifically designed for numerical analysis tasks or statistical analyses. While it can provide guidance or suggest potential approaches, it's not a replacement for specialized statistical software or coding libraries that are better suited for these tasks.
Germain, what are the computational requirements for using ChatGPT for data analysis? Can it be run on personal computers, or is it more resource-intensive?
Great question, Isabella! ChatGPT can be resource-intensive, especially for large-scale analysis tasks. Training and utilizing the model at scale may require significant computational resources, such as powerful GPUs or cloud infrastructure. However, for smaller tasks, it's possible to run ChatGPT on personal computers using OpenAI's provided APIs.
Although ChatGPT is impressive, I wonder if it has any limitations when it comes to understanding domain-specific jargon or technical terms?
Valid concern, Henry. While ChatGPT has some understanding of a wide range of topics, it may not have specific knowledge of all domain-specific jargon or technical terms. It is more effective when provided with clear instructions and context relating to the specific terms or jargon being used.
Based on your experience, Germain, what are some common challenges one might face when using ChatGPT for data analysis, and how can they be overcome?
Great question, Sophie! Some common challenges include generating accurate results, avoiding biases, ensuring privacy and security, and handling complex or technical questions. These can be addressed by providing clear instructions, understanding the limitations of ChatGPT, reviewing and verifying outputs, and considering alternative approaches or expert guidance where necessary.
I'm curious, Germain, how does ChatGPT handle large volumes of text input for analysis? Are there any limitations in terms of input size?
Good question, Lucas. ChatGPT has a maximum token limit for input, which can vary depending on the API version and model configuration. For example, the base GPT-3 model has a limit of 4096 tokens. If your input exceeds this limit, you would need to truncate or preprocess the text to fit within the allowed token count.
Germain, can you share some real-world use cases or success stories where ChatGPT has been applied effectively to solve data analysis problems?
Certainly, Olivia! ChatGPT has been applied effectively in various real-world use cases. Some examples include text generation, content summarization, language translation, and even creative writing. While it may not be specialized for all data analysis problems, it can assist in various aspects and save time by automating certain tasks.
This article is really insightful, Germain. How frequently is ChatGPT updated with new data and improvements?
Thank you, Mia! OpenAI is continually working on improving ChatGPT. While they don't explicitly state the frequency of updates, they aim to incorporate user feedback and make regular model improvements. This iterative process allows them to address limitations, enhance performance, and introduce new features over time.
Germain, what are some potential risks or limitations of integrating ChatGPT into critical data analysis processes, and how can these risks be mitigated?
Great question, Riley! Some potential risks include reliance on potentially biased or incorrect answers, lack of transparency in decision-making, and security concerns when handling sensitive data. These risks can be mitigated by thorough testing and validation, using ChatGPT as a tool alongside human expertise, and implementing appropriate security measures to protect sensitive data during integration.
I'm fascinated by ChatGPT's capabilities for data analysis. Can it generate visualizations or charts based on the analyzed data?
Good question, Jack! ChatGPT focuses on text-based interactions and doesn't have built-in capabilities for generating visualizations or charts. However, it can provide guidance or suggestions on generating visualizations based on the analyzed data, which can then be implemented using specialized visualization libraries or tools.
In your opinion, Germain, what are the key benefits of using ChatGPT compared to traditional data analysis approaches?
Great question, Sophie! ChatGPT offers benefits in terms of automation, speed, and ease of use. It can save time by automating certain analysis tasks, provide quick insights through conversational interactions, and enable users without specialized coding knowledge to perform data analysis. However, it's important to consider its limitations and use it as a complementary tool to traditional approaches.
Germain, do you have any tips or recommendations for effectively integrating ChatGPT into existing data analysis workflows?
Certainly, Max! When integrating ChatGPT, it's advisable to start with smaller, well-defined tasks to understand its capabilities better. Provide clear instructions and context, review and validate outputs, and consider incorporating it as part of an iterative analysis process, combining ChatGPT's insights with human expertise. This way, it becomes a valuable tool alongside existing workflows.
Germain, thank you for this informative article. In your experience, what level of domain expertise is required to make effective use of ChatGPT for data analysis?
You're welcome, Isabelle! While ChatGPT can assist with data analysis tasks, having domain expertise can significantly enhance its effectiveness. Understanding the nuances of the data and analysis requirements allows users to provide better instructions, review outputs critically, and incorporate appropriate context. Domain expertise acts as a valuable complement to maximize the benefits of using ChatGPT in data analysis.
What are some potential trade-offs of using ChatGPT for data analysis, and how can users navigate these trade-offs effectively?
Good question, Ava! Some potential trade-offs include occasional incorrect or incomplete answers, possible biases, and the need for critical review. To navigate these trade-offs effectively, users should validate outputs, be aware of potential biases, and consider alternative approaches or expert guidance where necessary. Understanding the limitations and actively reviewing and refining results helps in striking a balance between automation and accuracy.